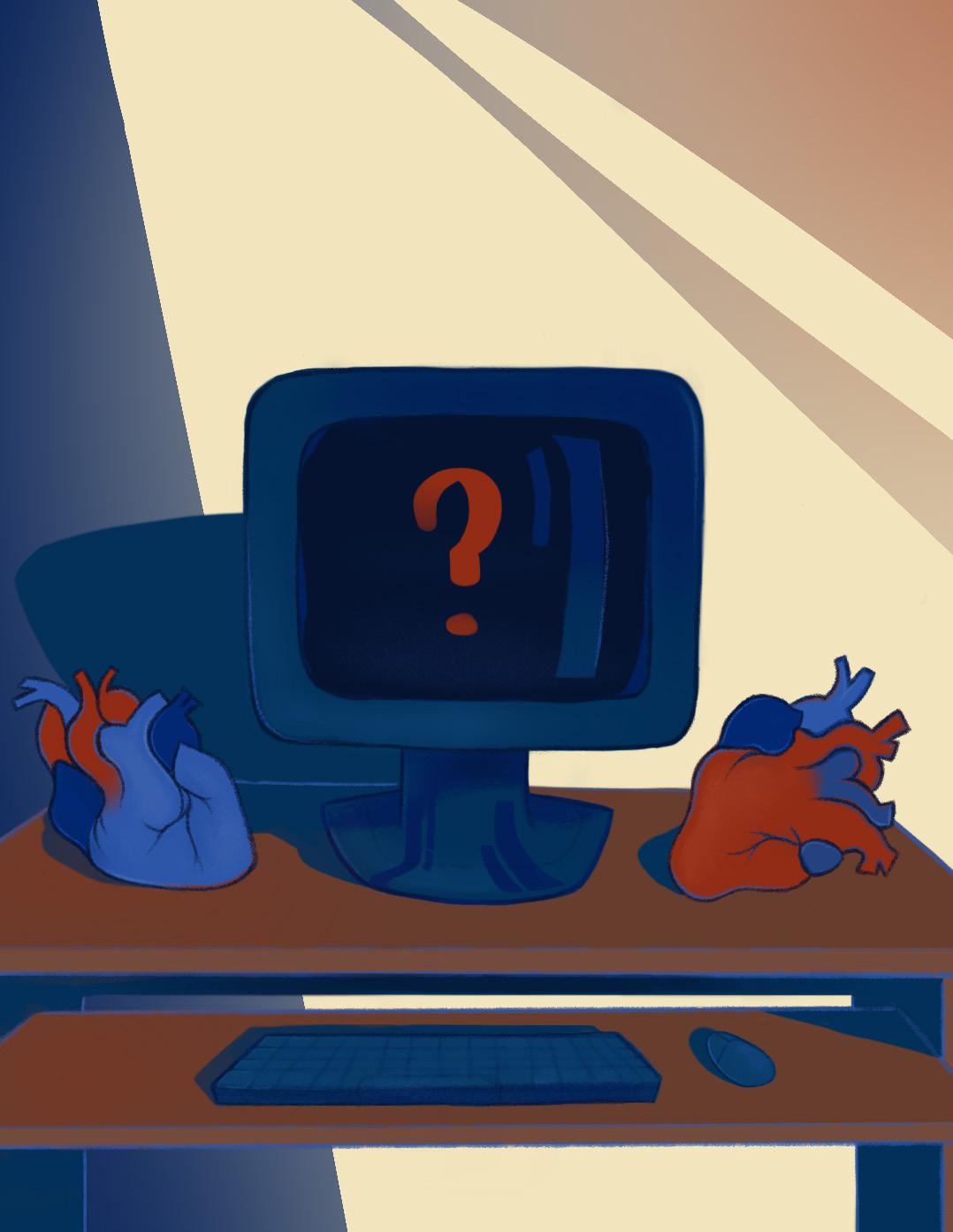
7 minute read
The New Era of Biology:
Differentiating Rare Inflammatory Diseases with the Boolean Method
Written by Anna Grinberg Illustrated by Oliver Kelley
Advertisement
Computational analysis of gene sequences has been a valuable tool for identifying molecular pathways which contribute to the understanding of medical conditions. The Boolean Lab at UC San Diego analyzes the activity of gene expression to compare host-immune responses for two different diseases that may aid in tracking prognosis and drug development.
Alarge amount of genetic information is accessible to biologists who don't know how to wrangle the data, and computer scientists who can’t interpret the biology. A bridge between applying computational formulas to data sets and identifying correlations between various molecular mechanisms is the new era of biology. For example, transcriptomes which contain the portion of RNA code for protein expression may be biomarkers which correlate to a certain disease.1 A biomarker may be a set of genes that express proteins, known as a gene signature, which contribute to a subsequent medical condition.1 Identifying and quantifying biomarkers corresponding to specific diseases can aid in revealing distinct molecular pathways and inform therapy development. However, this process is easier said than done. In the clinic, small patient sample numbers, especially with rare diseases, makes identifying biomarkers hard. In the laboratory, petri dish-based experimental models may not reflect human physiology. However, with computational assistance, these barriers can be overcome. One application could be a formula that simplifies clinical transcriptomic data into a correlational relationship with disease type and severity. This can be analyzed to differentiate between two symptomatically similar medical conditions and serve as a step towards accurate diagnosis and tracing disease severity.
In the clinic, separate patients may present with indistinguishable symptoms, with diagnoses requiring very different treatments. In recent years, a condition linked to post-COVID-19 infection, known as multisystem inflammatory disease in children (MIS-C) caused an inflammatory response in children, including skin rashes and a heart defect known as myocardi- al dysfunction. As MIS-C case counts accumulated, doctors found these symptoms hard to distinguish from another pediatric disease, Kawasaki disease (KD). This acute inflammatory disease was first described in Japan, 1967.2 Patients show symptoms of red eyes, hands, and feet. As an immune response to an unidentified antigen trigger, doctors observe high white blood cell count and long-lasting vascular damage which causes coronary artery aneurysms (inflamed arteries). Fortunately, MIS-C patients who present vascular damage do not experience permanent effects. In contrast, 25% of KD patients, if left with untreated coronary arterial walls, will develop life-threatening aneurysms.2 Although the early onset symptoms of these two diseases are similar, their heart conditions require distinct treatments to prevent exacerbation. An appealing way to distinguish KD from MIS-C and provide appropriate treatment is through computational differentiation of their mRNA expression signatures which actively transcribes protein products. Using mRNA sequencing and further analysis to find a disease’s unique transcriptome signature shows promise towards locating inflammatory pathways that correlate with disease and symptom severity and could possibly serve as targets for drug development.
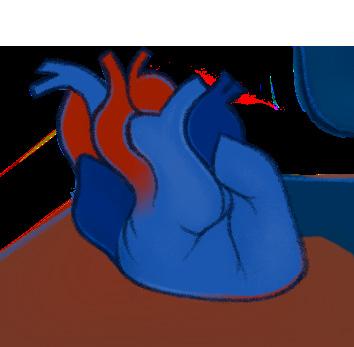
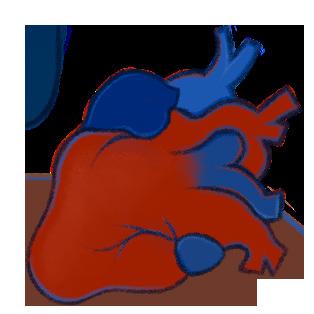
Dr. Debashis Sahoo, who leads the Boolean Lab at UC San Diego, took on the challenge to simplify these large transcriptomic datasets into a biomarker indicative of viral infection in collaboration with Dr. Jane C. Burns and Dr. Pradipta Ghosh. RNA sequencing data has allowed researchers to identify protein products that are correlated to host immune responses of certain viral infections. However, a biomarker that pertains to viral infections amongst these host immune responses has not been identified. To do this, researchers trained an artificial intelligence model on mRNA transcriptomes of patients infected by COVID-19 and influenza. To identify genes upregulated in COVID-19 infections, publicly accessible mRNA sequences of virally infected and healthy cells were analyzed against a specific gene’s expression levels as a reference point. Known as the ‘seed’ gene, this gene is chosen as the baseline from which correlational relationships can be derived.3 The re- ceptor angiotensin-converting enzyme 2 (ACE2), which enables COVID-19 entry, was chosen as the ‘seed’ gene since it is associated with COVID-19 infection, and high levels are indicative of a viral response.3 Boolean analysis, a way to mathematically detect the dependent relationship between two values, identified 166 genes which were upand downregulated in correlation to ACE2 mRNA expression levels.4 This group of genes were coined the viral pandemic (ViP) signature, which could be used as a fundamental viral marker. This 166-gene pool was further enriched for genes involved with unique pathways and trained, by the AI model, on influenza patients annotated with disease severity. This model obtained a 20-gene subset—coined the severe viral pandemic (sViP) sig nature. These ViP signatures served as mechanistic biomarkers that pro vided the framework for uncovering and differentiating diseases. of sViP correlated to patients with minor myocardial dysfunction.5 Blood transcriptomes of MIS-C patients were used to evaluate the protein products of marked genes that were tracked with clinical and laboratory outcomes such as the unique heart dysfunction of MIS-C patients.5 The results demonstrated that cytokine cellular communication and interferon upregulation was in higher quantities for MIS-C than KD. The greater magnitude of expression of these molecular factors involved in host-immune response in MIS-C determined that the severity and diagnosis of MIS-C may be quantitatively distinguished from KD. As computational methods help identify distinct gene expression biomarkers and highlight distinctive immune response features in rare diseases, it may aid in better understanding the nature of the disease. aid in setting guidelines for treatment. The Boolean lab has shown that an artificial intelligence approach to applying statistical concepts to biological data enables the identification of genetic biomarkers across a wide range of diseases. The strategy works in diverse contexts, such as mice, humans, or even pandemics, demonstrating how small sample sizes can be used to glean large insights through computer algorithms. This is especially important for rare diseases such as MIS-C and KD, where there isn't a large sample population. Biology contains complexities, uncertainties and exceptions, but Boolean logic can be used to control this noise.
In acute KD, ViP and sViP signatures could classify patients with large coronary arterial aneurysms from those with small ones. Additionally, the sViP signature could differentiate MIS-C patients with major or minor myocardial dysfunction.
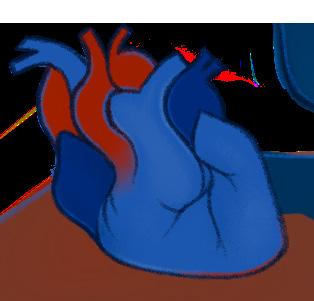
To test the accuracy of the ViP signature, the model was test ed against patient data. Dr. Jane C. Burns, a practicing pediatrician and Kawasaki disease expert at the UC San Diego School of Medicine, has experi ence with both KD and MIS-C patients. In 2020, she and her team collected whole blood samples from children in the emergency room presenting with fevers or any inflammatory symptoms. The blood samples were then isolat ed for RNA and the transcriptome was sequenced via next-generation sequencing, a concurrent examination of numerous gene fragments to detect sequence variations. dataset was then given to Dr. Sahoo, whose group analyzed the 166-gene ViP and 20-gene sViP signatures using data sets of MIS-C and KD patients. Surprisingly, both KD and MIS-C patients showed upregulation in the genes associated with the ViP signatures, sharing the same viral pandemic signature and indicating they were on the same continuum of host immune response. Curiously, no unique viral source has been recognized in KD, and compelling evidence exists pointing to KD as a disease not caused by a viral infection; however it shares an inflammation gene signature that is linked to serious viral infections.
Publically available transcriptome sequencing datasets enable scientists to computationally analyze gene expression in patients and gain understanding of a disease. This data is obtained from patients by collecting blood samples. These samples go into databases and are accessible to download for research purposes.
Beyond possible targets for drug treatments, the ViP signatures can po- tentially predict the severity of disease and diagnosis. In acute KD, ViP and sViP signatures could classify patients with large coronary arterial aneurysms from those with small ones. Additionally, the sViP signature could differentiate MIS-C patients with major or minor myocardial dysfunction. Samples with higher levels of sViP signature expression revealed to be patients with major myocardial dysfunction, and a low level
KD and MIS-C, both inflammatory diseases, result in host-immune responses that stimulate immune cells, causing over-secretion of signaling proteins called cytokines.6 The extent of cytokine expression can differentiate inflammatory diseases. Researchers in the Boolean lab used KD and MIS-C blood samples and serum cytokine arrays to identify the cytokine-receptor pair, IL15 and IL15RA, as a prominent component of a pathway which induces the ViP signature. IL15 codes for a cytokine which binds to IL15RA receptors on immune cells to trigger an inflammation cascade. Though both KD and MIS-C shared an IL15 cytokine response, other responses were more unique to certain diseases.5 Upregulation of two other cytokines, TNFα and IL1β, was unique to MIS-C samples.5 The identification of TNFα and IL1β as upregulated cytokines in MIS-C may make them a target for anti-inflammatory drugs. Another distinctive quality of MIS-C based on analysis of serum cytokines showed that it had higher cytokine levels than KD, indicating a higher intensity immune response. Cytokine levels in MIS-C and KD as mechanistic biomarkers contribute to the broader understanding of MIS-C as a disease. This may help discern disease severity and proposes a target for therapeutics.
Identifying unique markers of a disease helps improve our understanding of what mechanisms are involved with severity of the disease, which may
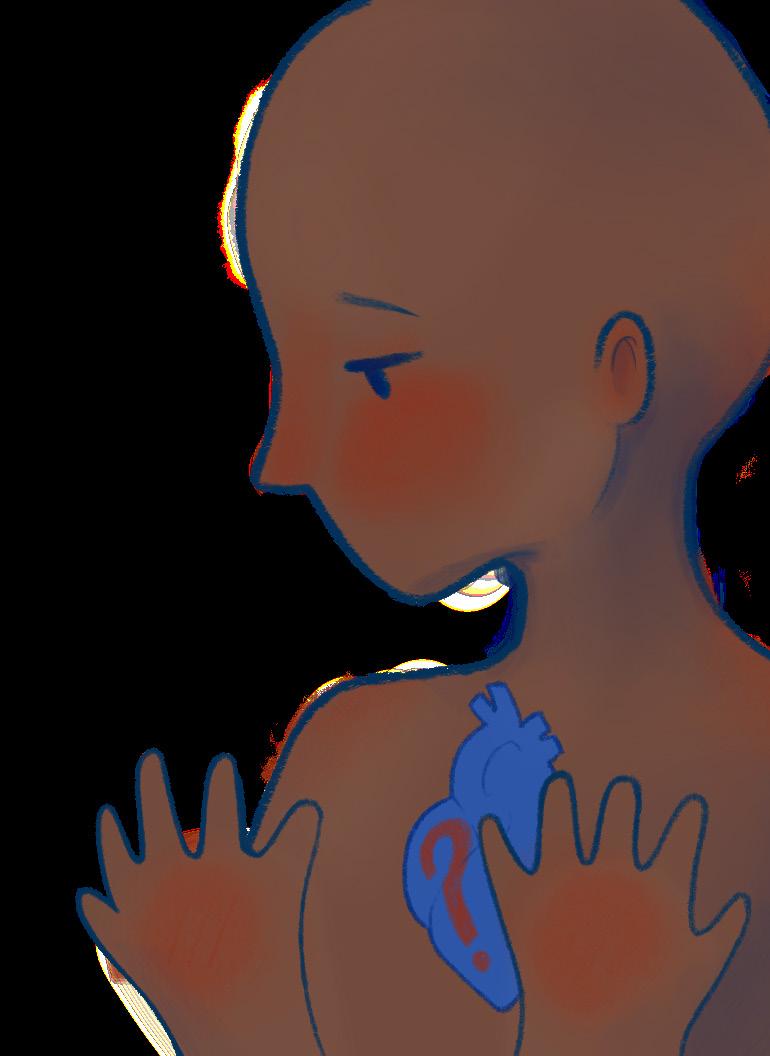
References
[1] Gönen M. 2009. Statistical aspects of gene signatures and molecular targets. Gastrointest Cancer Res.(2 Suppl):S19-21.
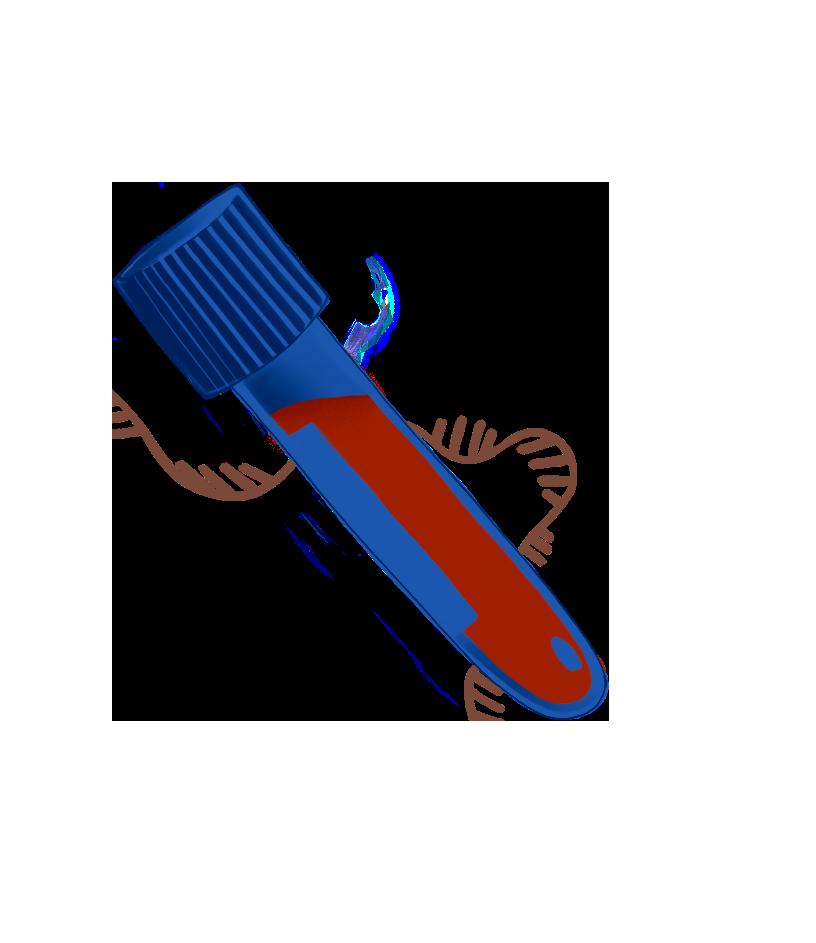
[2] Kushner, H. I.Turner, C. L., Bastian, J. F., Burns, J. C. 2004. The Narratives of Kawasaki Disease. Bulletin of the History of Medicine, vol. 78, no. 2, pp. 410–39.
[3] Sahoo D, Katkar G. D., Khandelwal S, Behroozikhah M, Claire A, Castillo V, et al. 2011. Al-guided discovery of the invariant host response to viral pandemics. eBiomMedicine, The LANCET vol. 68.
[4] Sahoo, D., Dill, D.L., Gentles, A.J., et al. 2008. Boolean implication networks derived from large scale, whole genome microarray datasets. Genome Biol 9, R157.
[5] Ghosh, P., Katkar, G.D, Shimizu, C. et al. 2022 An Artificial Intelligence-guided signature reveals the shared host immune response in MIS-C and Kawasaki disease. Nat Commun 13, 2687 (2022).
[6] Zhang JM, An J. 2007. Cytokines, inflammation, and pain. Int Anesthesiol Clin. Spring; 45(2):27-37.
Written
by Anna
Grinberg
Anna is a 3rd year Human Biology major from Eleanor Roosevelt College.