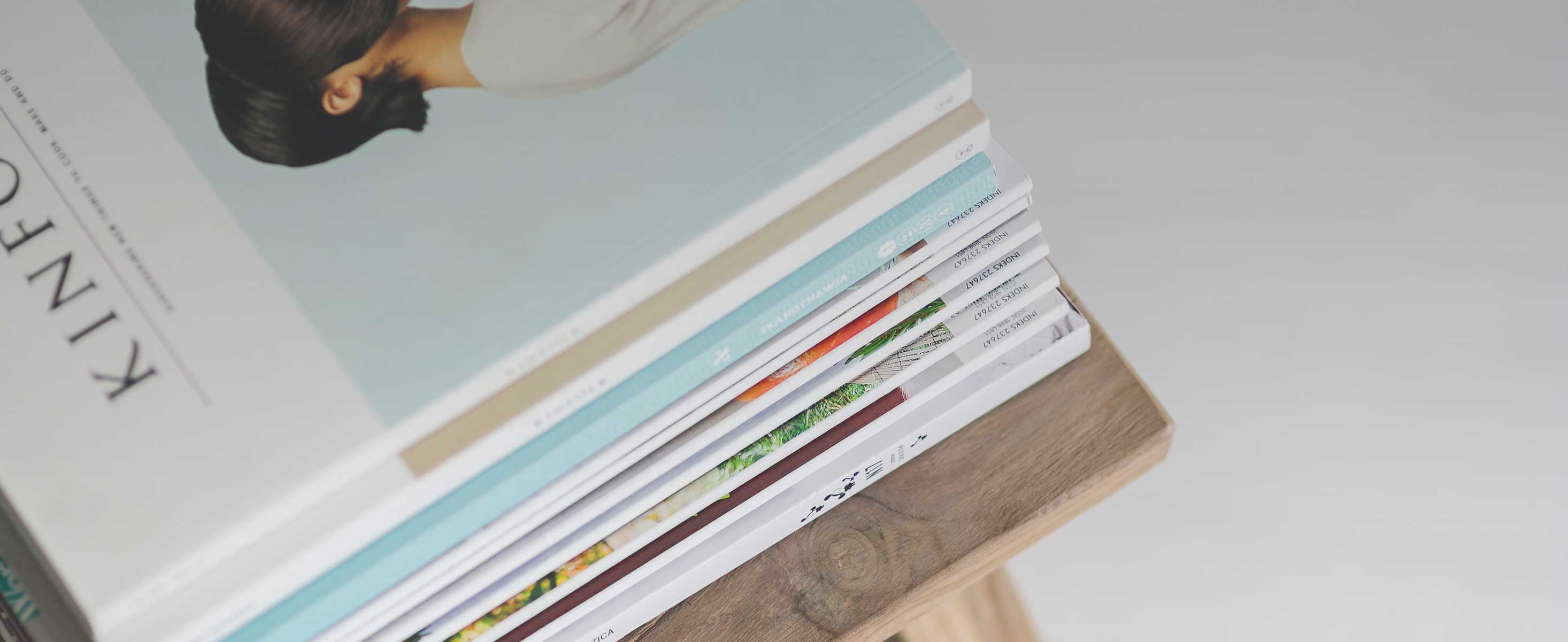
3 minute read
How to read articles that use machine learning – users’ guides to the medical literature
WHY THE STUDY WAS PERFORMED:
This paper is an introductory synopsis for readers of any machine learning article from medical imaging or computer vision journals. The user guide describes 3 goals to promote understanding of machine learning models with a critical eye: 1. To emphasise the importance of model validation. 2. Review basics of machine learning. 3. Review model implementation into clinical practice.
The building blocks of machine learning are explained, with differences defined between machine learning vs deep learning, and supervised vs unsupervised learning methods. Essential technical language is made accessible from quality glossary lists. These grounding terms have been cited in many subsequent articles, and form a useful repository for students of machine learning. While it may require more than one read to absorb the major and minor points, the emphasis throughout the article relates to how best apply machine learning research for the benefit of clinical practice. Clinical intuition and standard statistical principles still apply when it comes to evaluating machine learning/mathematical models.
HOW THE STUDY WAS PERFORMED:
The reader is stepped through a clinical research scenario for annual diabetic retinopathy screening. A hypothetical literature search produces articles which are difficult to compare, as each paper uses different data sets – test sensitivity vs independent samples vs clinical setting. This is familiar territory, and easily translates to appreciate the problems encountered for deriving, validating and establishing the clinical effectiveness of a machine learning tool.
WHAT DOES THE STUDY PROVIDE?
The following question list and dictionaries can be used to help understand and evaluate a machine learning study. For brevity, dictionary terms are simply listed.
1) Questions to assess the Validation of Machine Learning Models: • Is the reference standard high quality? i) Expert panel will reduce human judgement errors (interrater variability). ii) Experts blinded to machine learning predictions reduce bias. • Is the study design appropriate? i) Did the patient sample include an appropriate spectrum of patients to reflect a similar cohort in clinical practice? ii) A high quality design will ensure the validation data set is isolated from the training and tuning tasks. • Are the results unexpected? i) For machine learning studies, results can only be as good as the training data
REVIEWED BY Caterina Watson ASA SIG Research
REFERENCE Liu,Y., Cameron, P., Krause, J., Peng, L. The Journal of the American Medical Association
READ THE FULL ARTICLE HERE
supplied. Test results should not exceed expert annotation.
ii) Unexpected claims have arisen from retinal screening images and race/sex assignment. Independent researchers need to validate these findings with external cohorts. The results may be due to artifacts in the machine learning system, confounding factors, or flaws in the study design.
2) Glossary of general terminology associated with machine learning methods: Feature;
Hyperparameter; Label; Machine Learning; Artificial Intelligence; Deep Learning; Model;
Algorithm; Overfitting; Parameter; Reference standard; Training; Tuning.
3) Glossary of terms associated with machine learning methods: Types of machine learning schemes; Data set names [ Development set; K-fold cross validation; Training set; Tuning set; validation set ]; Regularisation [ Data augmentation; Early stopping; Ensemble; Fine tuning/Pre-initialisation/Warm start; Parameter regularisation].
RELEVANCE TO CLINICAL PRACTICE:
Machine learning is a tool to clarify the relationship between data and clinical features. Sonographers should identify overly optimistic model performance in research reports. A large gap in performance between tuning and validation may indicate overfitting to the tuning set. Clinical factors may also contribute to performance, such as population age or disease subtype. Assessment of overfitting involves machine learning expertise (qualitative assessment of tuning-validation performance gap) and clinical intuition (qualitative assessment of patient population differences between development and validation sets). It remains critical to know if performance remains high with external cohorts. n