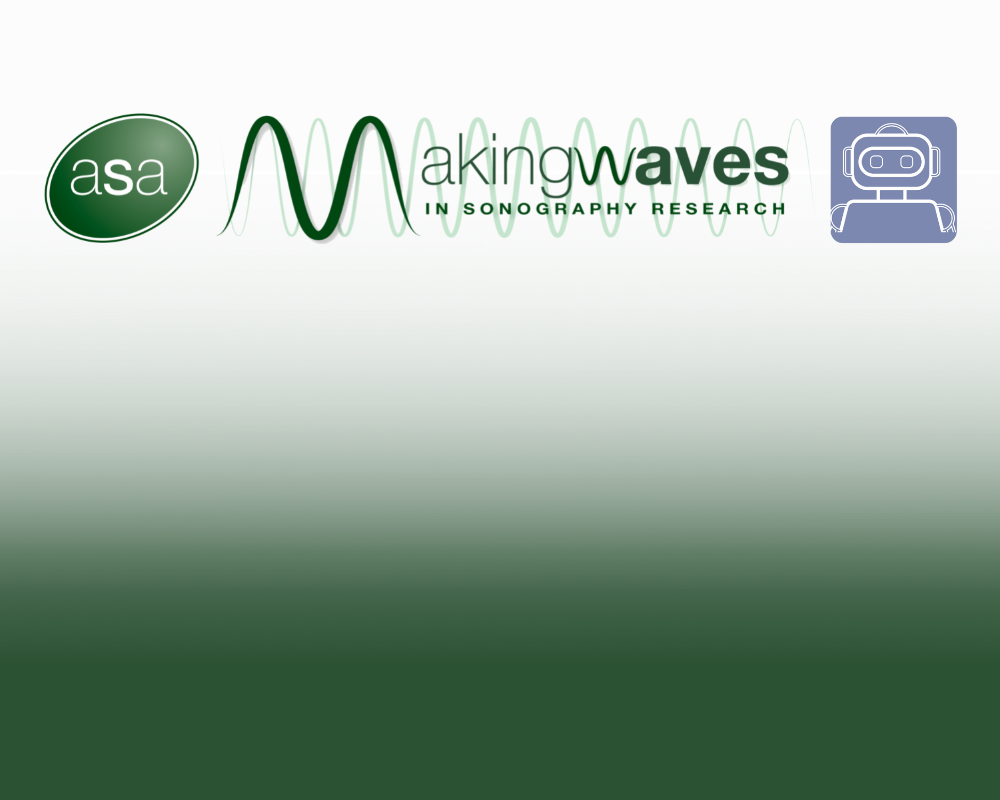
4 minute read
Deep learning radiomics based on contrast-enhanced ultrasound images for assisted diagnosis of pancreatic ductal adenocarcinoma and chronic pancreatitis
Deep learning radiomics based on contrast-enhanced ultrasound images for assisted diagnosis of pancreatic ductal adenocarcinoma and chronic pancreatitis
REVIEWED BY | Kosta Hellmanns ASA SIG: Emerging technologies
REFERENCE | Authors: Tong T, Gu J, Xu D, Song L, Zhao Q, Cheng F, Yuan Z, Tian S, Yang X, Tian J, Wang K & Jiang T. Journal: BioMed Central 2022; 20(74). Open Access: Yes
WHY THE STUDY WAS PERFORMED
The study aims to develop a deep learning model using advanced feature analysis (radiomics) on contrast-enhanced ultrasound (CEUS) images to assist radiologists in identifying pancreatic duct adenocarcinoma (PDAC) and chronic pancreatitis (CP), thus increasing diagnostic accuracy and avoiding unnecessary biopsy and surgery for patients.
HOW THE STUDY WAS PERFORMED
The study was undertaken with retrospective datasets from three hospitals. The hospital with the largest dataset was used as the primary cohort – patients admitted in 2020 used as the training cohort and those admitted in 2021 used as the internal validation cohort. The datasets from the remaining hospitals were smaller, and as such, used as two external validation cohorts. The inclusion criteria for the study were patients with pathologically confirmed CP without progression to pancreatic cancer in the six proceeding months or PDAC without distant metastasis, patients whose CEUS examinations were performed just prior to biopsy and surgery, and the availability of CEUS videos/images. The exclusion criteria were the presence of multiple lesions in the pancreas, a history of pancreatic surgery/chemotherapy, and inadequate image quality. This resulted in a sample of 351 for the training cohort, 109 for the internal validation cohort, 50 and 48 for the external validation cohorts.
For the analysis, one key CEUS image from each of the patient examinations was selected showing the maximum diameter of the lesion at approximately 35 seconds from injecting the contrast agent (early pancreatic phase of contrast perfusion). From this image, a region of interest (ROI) of the lesion area was determined by the radiologist on the raw greyscale image of CEUS acquisition, following which the ROI was marked at the same location on the CEUS images, before converting it to greyscale (for consistency across US machines) and resized to be appropriate for analysis. As shown below (extracted Figure 2), resized and grayscale ROI images were fed into the DLR model developed for the study, which then returned an AI score and heatmap for each lesion. In the first round read, radiologists made an initial decision on the lesions as per usual (i.e. blinded to each other, the original diagnostic reports and final pathology results, and without AI assistance). In the second round, radiologists could refine their first round of diagnoses with the additional AI score and heatmap information.
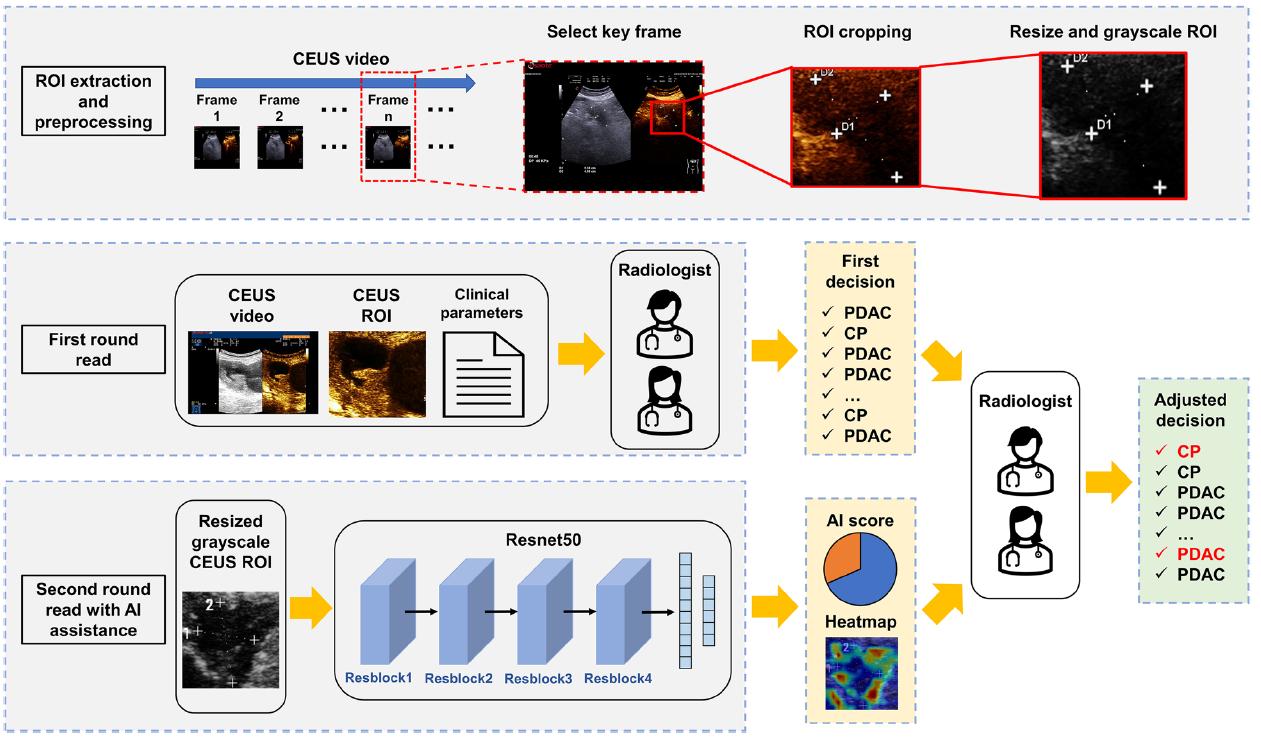
Figure 1. Workflow of ROI extraction, processing and the deep learning model
WHAT THE STUDY FOUND
Diagnoses were compared with confirmed pathological findings from biopsy or surgery in all patients. The statistical results of the cohorts are reported within the article. Results highlighted that the diagnoses of the five radiologists were either worse or comparable to those of the DLR model, with the model achieving better sensitivity and specificity than all radiologists in the first round read. In the second round read with AI assistance – all radiologists achieved higher sensitivity, with four out of the five radiologists achieving higher specificity in the internal validation cohort, two out of the five in the external cohort 1 and one out of the five in the external cohort 2.
RELEVANCE TO CLINICAL PRACTICE
The DLR model is an effective tool that can be utilised to assist radiologists in the diagnosis of PDAC and CP.