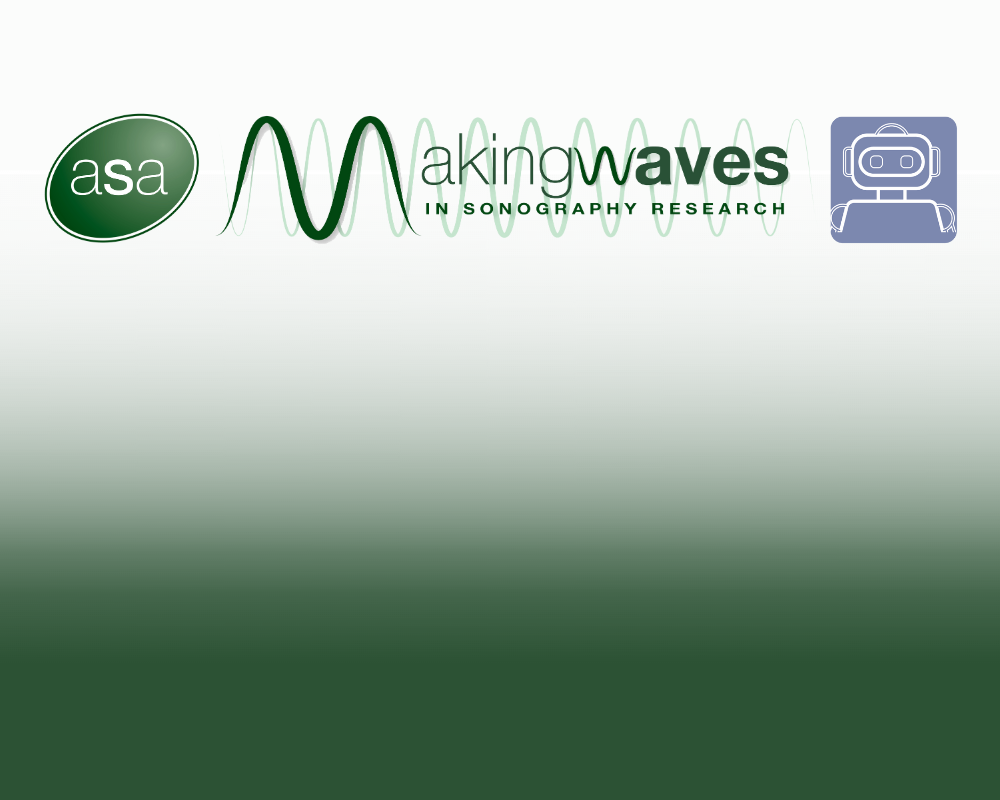
4 minute read
Artificial intelligence in breast ultrasound: application in clinical practice
Artificial intelligence in breast ultrasound: application in clinical practice
Reviewer: Lisa McGuire, AFASA | ASA SIG: Emerging Technologies
Authors: Brot H, Mango V
Why the study was performed
Ultrasound (US) has enormous clinical potential and economic benefits in diagnosing breast lesions when compared to other imaging modalities. This review explores the current applications of artificial intelligence (AI) in US and its potential impact on clinical practice. It discusses the impact of AI on clinical workflow and breast cancer detection, classification and diagnosis. This article also highlights the potential significance of AI in breast US for underserved areas.
How the study was performed
AI is a broad term encompassing various technologies, including machine learning (ML), deep learning (DL) and computeraided detection (CAD). Radiologists rely on qualitative visual assessment to characterise breast lesions. AI technologies analyse vast amounts of imaging data, hence assisting radiologists in identifying abnormalities and predicting outcomes, optimising the diagnostic process.
CAD is a commercially available AI software; however, there are a number of iterations. In general terms, once the lesion is identified as a region of interest, the CAD component generates a numerical assessment of the region. The software provides a BI-RADS category based on shape, orientation, margin, echo pattern and posterior features.
One DL AI model excelled in distinguishing between triple-negative breast cancer and other subtypes, significantly improving the accuracy, sensitivity, and specificity of four radiologists. The convolutional neural network multi-modal was developed by Zhou et al. and trained on combined grayscale US images together with colour Doppler and shear wave elastography features. This model surprisingly also outperformed core needle biopsy.
Another study investigated AI and deep learning radiomics (DLR) using US images in patients undergoing neoadjunctive chemotherapy (NAC). Jiang et al. developed a novel preoperative pathological complete response (pCR) prediction model, which is based on pre- and post-NAC US images, showing an AUC of 0.94 (95% CI, 0.91 to 0.77), outperforming two experienced radiologists. Additionally, Gu et al. prospective study used two post-treatment time points to assess response to NAC. This stepwise prediction DLR model successfully identified 90% of non-responders, triaging them to a different treatment strategy.
A predictive model of lymph node metastasis in patients with breast cancer using DL was developed by Zhou et al. The best-performing CNN model was superior to a consensus of five radiologists, achieving a higher sensitivity of 85%.
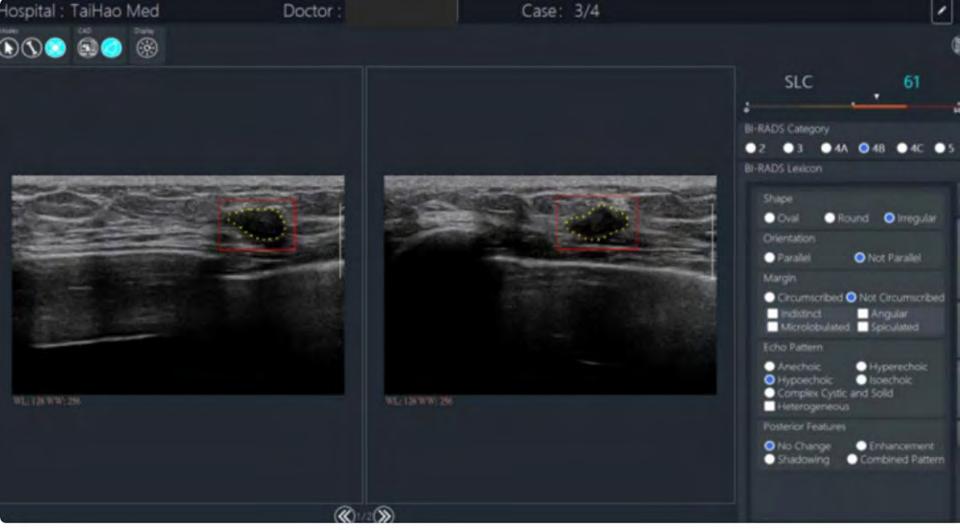
What the study found
Image analysis and interpretation algorithms have the potential to automate the detection of breast lesions in US images. This paper reports sensitivity and specificity comparable to that of experienced radiologists.
The review shows the adjunct of CAD enabled faster and more accurate interpretations.
The paper states AI can assist in differentiating between benign and malignant lesions, analysing features such as shape, margin and echogenicity. Large DL models have been trained on large data sets to recognise patterns and characterisation of lesions that may indicate malignancy, providing additional diagnostic support.
Relevance to clinical practice
The integration of AI into breast US has the potential to improve clinical outcomes through enhanced diagnostic accuracy. This review suggests AI can reduce unnecessary biopsies and, in turn, patient anxiety.
Despite the promise of AI in breast US shown in this review, several challenges remain: data quality and bias; AI models are heavily dependent on data quality and diversity of training. Inadequate datasets can lead to biased algorithms. It is also challenging to successfully integrate AI tools into existing workflows.
Most importantly, AI may improve access to healthcare and bring radiology services to underserved areas with few or no radiologists; however, further prospective studies are necessary to comprehensively assess the influence of AI in real-world clinical settings.
An excellent diagnostic performance was obtained by the AI tool detecting malignant breast lesions with a sensitivity of 100% and specificity of 86%