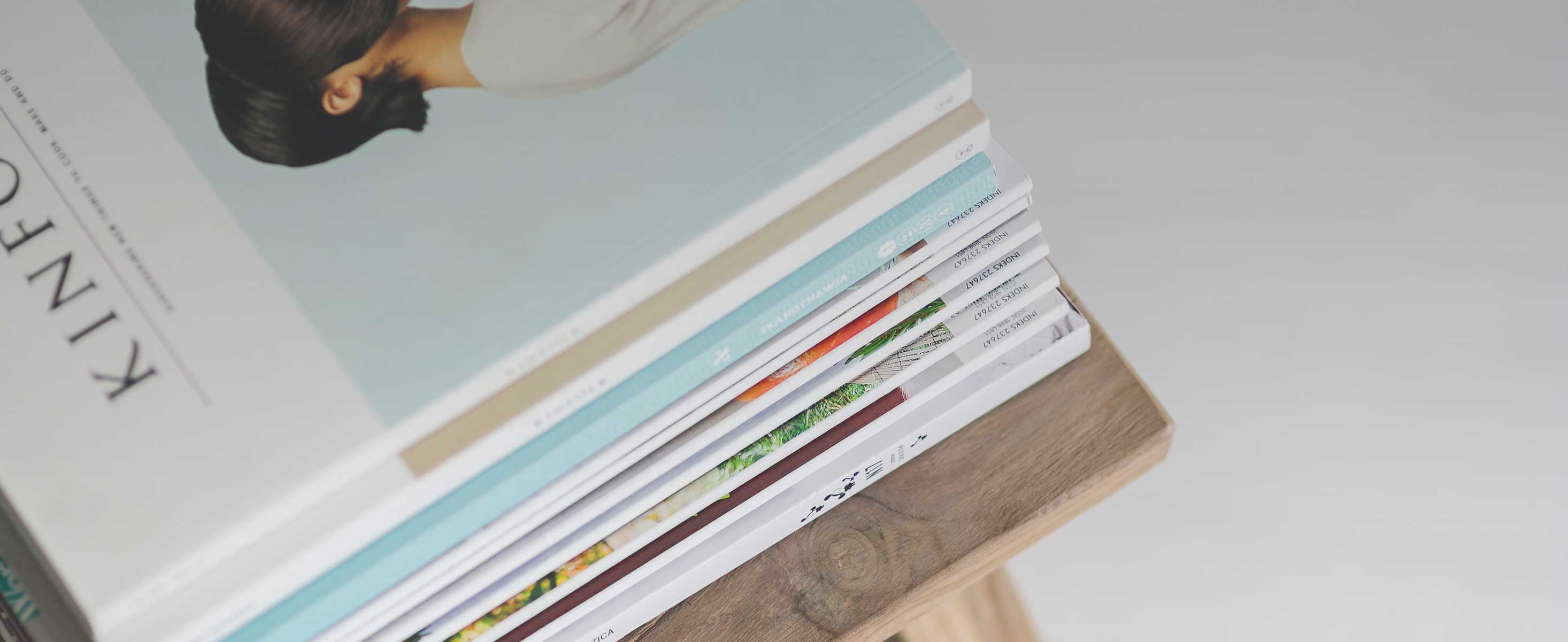
5 minute read
ChatGPT and Causal Reasoning in Epidemiology
Author: Louis Anthony Cox, Jr., PhD tcoxdenver@aol.com
A new generation of artificial intelligence (AI) systems has arrived. It’s already beginning to transform the highly skilled cognitive work of knowledge workers including researchers, data analysts, and programmers if not yet the deliberations of policymakers and the choices of decision-makers and risk managers. What are the implications for epidemiologists and casual reasoning?
The underlying technology consists of “large language models” (LLMs) that can engage in fluent and well-informed conversations with human users by continually calculating highly probable argumentation patterns and generating next words and phrases. This process is based on conditional probabilities estimated from massive training sets, such as the corpus of web-accessible documents – a technology sometimes caricatured as “autocomplete on steroids”. One of the currently best-known LLMs, ChatGPT, was launched in November of 2022. (“GPT” stands for “generative pre-trained transformer” reflecting the ability of such models to generate answers to questions, text summaries, stories, poems, and so forth using pre-trained neural nets that transform input sequences of words to output sequences, i.e., responses from the AI.)
How can large language models help epidemiologists?
How can epidemiologists take advantage of this emerging AI technology? Asking ChatGPT itself produced the following chat (italicized text is the author’s query; plain text is ChatGPT’s response; bold font highlights the parts of ChatGPT’s response that the author considers best summarize the main points):
Large language models like GPT-3 can help epidemiologists in several ways:
1. Data Analysis: Epidemiologists often have to analyze large amounts of data to identify patterns and trends. Large language models can help process and analyze this data more efficiently, which can help epidemiologists identify new insights and correlations.
2. Information Retrieval: Epidemiologists need access to vast amounts of scientific literature and research papers to stay updated on the latest findings. Large language models can help search and retrieve this information more efficiently, which can save time and improve the accuracy of their research.
3. Predictive Modeling: Epidemiologists often use predictive modeling to forecast the spread of diseases and plan for public health interventions. Large language models can help improve the accuracy of these models by incorporating more data and variables, which can lead to more accurate predictions.
4. Natural Language Processing: Epidemiologists often need to communicate their findings to the public and policymakers in clear and accessible language. Large language models can help generate summaries and explanations of complex scientific concepts, which can make their research more accessible to a wider audience.
Overall, large language models have the potential to revolutionize the field of epidemiology by enabling faster, more accurate data analysis and more effective communication of research findings to policymakers and the public.
At the moment, these and other use cases and potential benefits are perhaps more aspirational than real, as ChatGPT struggles to provide accurate references for its outputs (sometimes making up plausible-sounding ones, reflecting its underlying conditional probability models) and often answering technical questions with plausible-sounding answers that may not be correct, and hence that require human checking. However, larger and more powerful LLMs are on their way, and current ones are already useful if they are used with an understanding that their responses are not necessarily trustworthy. For example, the following question and response illustrate the current state of information retrieval and synthesis using ChatGPT to answer a question about fine particulate matter (PM2.5). Parts of the text in bold and italics deserve further scrutiny in the author’s opinion, as explained below.
Query: What are the few most important confounders of PM2.5-mortality associations?
PM2.5-mortality associations have been extensively studied in epidemiology, and several confounders have been identified that can affect the strength and interpretation of these associations. The following are a few of the most important confounders:
1. Temperature: Temperature is a strong confounder of the PM2.5-mortality association because both high temperatures and high levels of PM2.5 can increase mortality rates. Therefore, studies that do not adjust for temperature may overestimate the effect of PM2.5 on mortality.
2. Socioeconomic status: Individuals from lower socioeconomic backgrounds are more likely to be exposed to high levels of PM2.5, and they may also be more vulnerable to the health effects of air pollution. Therefore, studies that do not adjust for socioeconomic status may overestimate the effect of PM2.5 on mortality.
3. Smoking: Smoking is a major contributor to mortality, and smokers are more likely to be exposed to high levels of PM2.5. Therefore, studies that do not adjust for smoking may overestimate the effect of PM2.5 on mortality.
4. Health status: Individuals with pre-existing health conditions are more vulnerable to the health effects of air pollution. Therefore, studies that do not adjust for health status may overestimate the effect of PM2.5 on mortality.
5. Other air pollutants: Other air pollutants, such as ozone and nitrogen dioxide, can also affect mortality rates. Therefore, studies that do not adjust for other air pollutants may overestimate the effect of PM2.5 on mortality.
It is important for epidemiologists to consider and adjust for these confounders when studying PM2.5-mortality associations to accurately estimate the effect of PM2.5 on mortality.
The responses are certainly helpful in stimulating thought about possible confounders of fine particulate matter (PM2.5)-mortality associations, but their details require checking and thought. (For example, is it true or merely plausible that “smokers are more likely to be exposed to high levels of PM2.5”? Can’t individuals from lower socioeconomic backgrounds have higher mortality rates for many reasons other than possibly being “more vulnerable to the health effects of air pollution”? And is “Health status” an example of confounding or of effect modification?) Nonetheless, the potential for this technology to help epidemiologists is clear. It can remind them of relevant considerations, list the assumptions of technical methods and models, summarize what regulators and others have said, and in general act as a usually helpful, but not completely trustworthy, junior research assistant.
Of greater concern is that ChatGPT is not trained in ideal reasoning patterns but on the body of human reasoning found on the web. An extended (multipage) dialogue with ChatGPT recently published in Global Epidemiology (Cox, 2023) shows ChatGPT making and defending very strongly worded initial causal claims (“It is well-established that exposure to ambient levels of PM2.5 does increase mortality risk”) that it gradually modifies under sustained questioning to a much weaker conclusion that “It is not known with certainty that current ambient levels of PM2.5 increase mortality risk. While there is strong evidence of an association between PM2.5 and mortality risk, the causal nature of this association remains uncertain due to the possibility of omitted confounders, such as lagged daily temperature extremes, that could plausibly explain the observed associations.” Along the way, it displays classic fallacies of human reasoning and argumentation such as treating evidence of association as evidence of causation until challenged. Further, ChatGPT also introduced red herring and strawman arguments its response to a question about controlling for lagged daily temperatures was, “it is not always possible to control for every single potential confounder” even though it did not deny that the specific confounder in question could in fact be controlled for. However, the current version of ChatGPT is already open to sustained Socratic truth-seeking dialogue and will modify its initial position within a chat (although not yet its starting position for new chats) in light of a reasoned discussion.
The advent of LLMs and the emergence of genuinely useful conversational AI is seen by many as a likely turning point in the co-evolution of humanity and technology (Kissinger et al., 2021). For epidemiologists, it seems likely that as this technology improves – and major improvements are imminent – it will be applied to criticize and improve its own reasoning about causation and prevention of diseases and will become a valuable research assistant and then partner in discovering more effective ways to use data analysis and sound epidemiological reasoning to improve human health and longevity. This revolution has already begun. ■
References
Cox LA (2023). Causal reasoning about epidemiological associations in conversational AI. Global Epidemiology 5.
https://doi.org/10.1016/j.gloepi.2023.100102
Kissinger HA, Schmidt E, Huttenlocher D (2012). The Age of AI and our Human Future. Little Brown and Company. New York, NY.