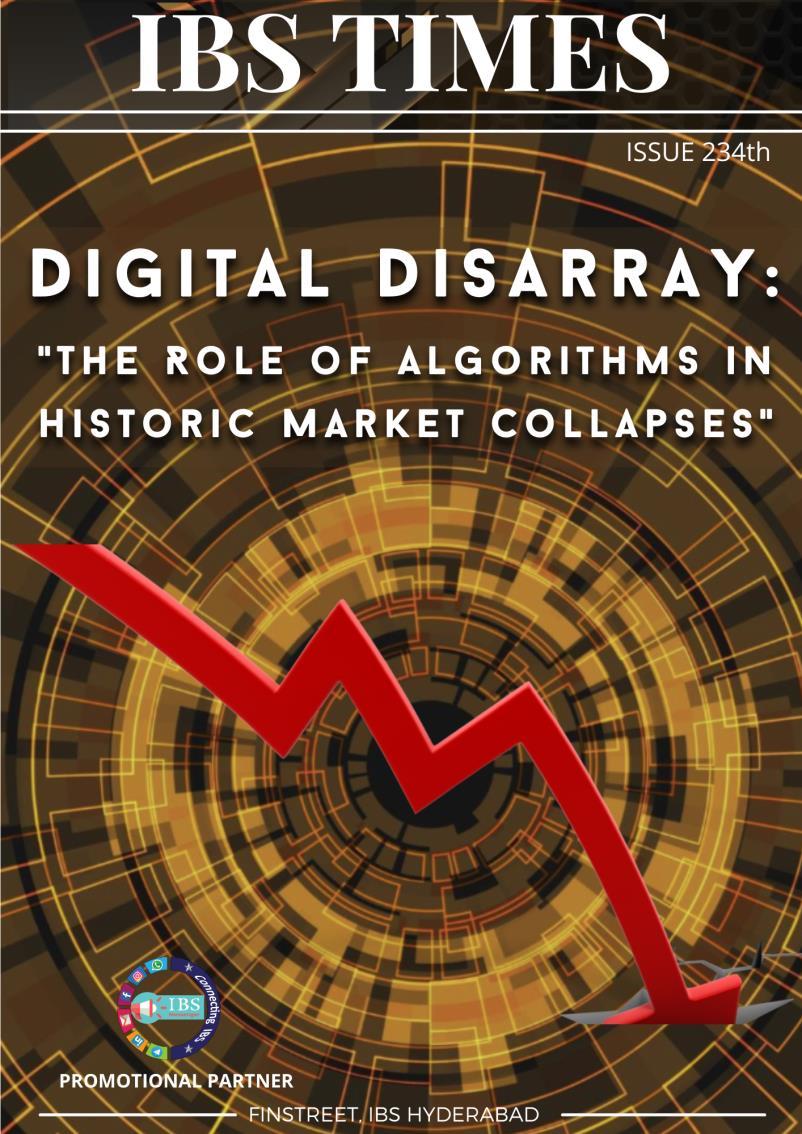
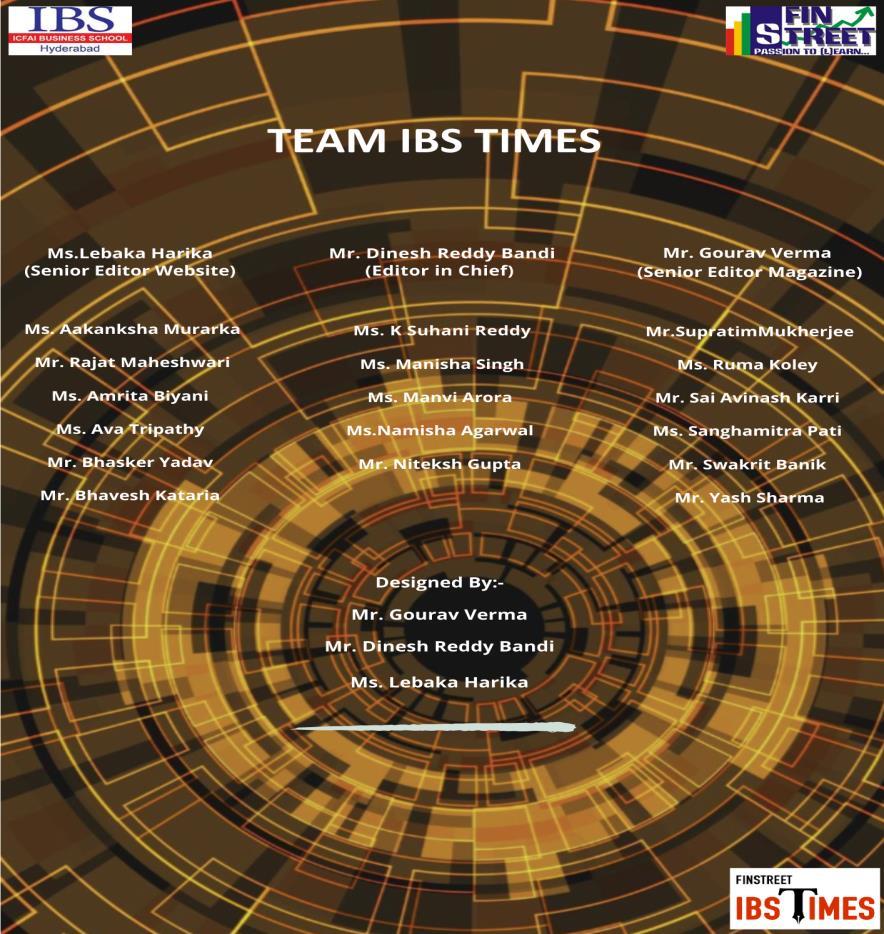
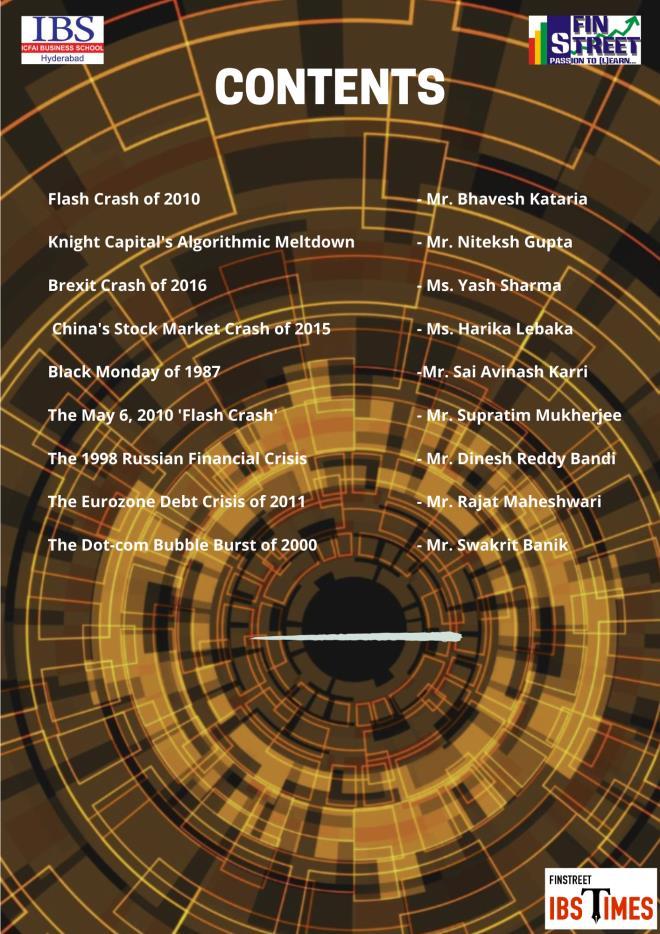
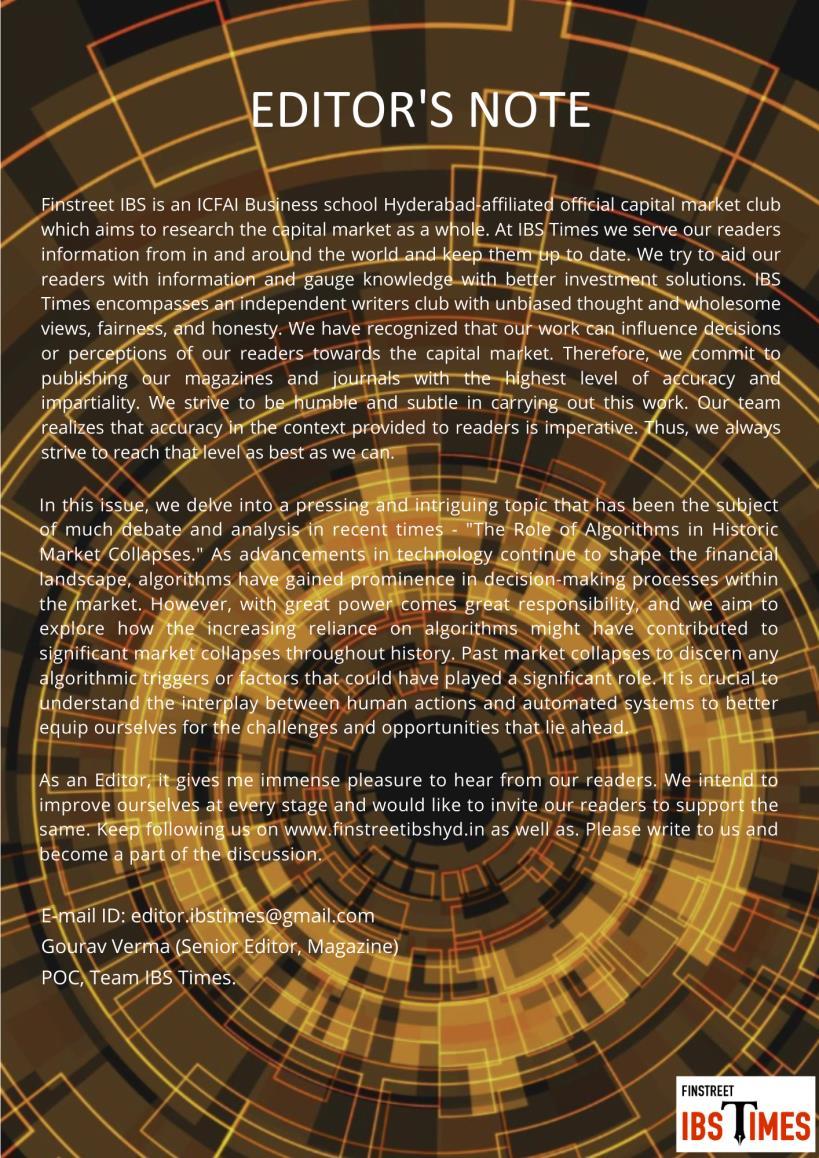
The Flash Crash of 2010 stands as one of the most significant events in the history of financial markets. On May 6, 2010, the global financial system experienced an unprecedented and rapid decline, as the Dow Jones Industrial Average (DJIA) plummeted nearly 1,000 points within minutes. This event, known as the Flash Crash, shocked traders and investors worldwide, raising concerns about the stability and integrity of modern financial markets. This essay delves into the causes, consequences, and regulatory responses to the Flash Crash, providing insights into the factors that contributed to this historic episode and the lessons learned in its aftermath.
dramatic decline and subsequent recovery of stock prices. Several factors contributed totheseverityandrapidityofthecrash:
High-Frequency Trading (HFT): The rise of high-frequency trading, driven by advanced algorithmsandsuper-fasttradingplatforms, playedapivotalroleintheFlashCrash.HFT algorithms, designed to capitalize on small price discrepancies, amplified the selling pressureandexacerbatedmarketvolatility.
MarketFragmentationandOrderRouting: The fragmented nature of the market, with multiple trading venues and decentralized order routing systems, intensified the transmissionandamplificationofthecrash. As panic selling increased, trades were routed to different exchanges, causing delays in executions and exacerbating price distortions.
Liquidity Imbalances: The Flash Crash exposed significant liquidity imbalances in the market. As liquidity dried up, the absence of buyers led to a cascade of sell orders, resulting in a further intensification ofthedownwardspiral.
The Flash Crash of 2010 began around 2:32 pm Eastern Time and lasted for approximately 36 minutes. During this brief period, market volatility reached unprecedented levels, resulting in a
To comprehend the factors that led to the Flash Crash, it is essential to examine key causesandcontributingelements:
High-Frequency Trading (HFT) Amplification: HFTstrategies,whichrelyon complex algorithms and lightning-fast execution, significantly contributed to the rapid decline. As market liquidity diminished, HFT algorithms withdrew from the market, exacerbating the selling pressure and accelerating the downward movement.
Execution Challenges: The fragmented structure of the market, characterized by multipleexchangesanddecentralizedorder routing systems, playeda crucial role in the Flash Crash. The swift transmission of trades across different venues, coupled with execution challenges during periods of extreme volatility, amplified the price decline.
Liquidity Evaporation: The sudden withdrawal of liquidity by market participants, both human and algorithmic, caused aliquidityvacuum. This evaporation of liquidity created an environment where even relatively small sell orders had a disproportionate impact on prices, triggeringachainreactionofpanicselling.
Role of Derivatives and ETFs: Certain derivativeproducts,suchase-MiniS&P500 futures contracts, were believed to have played a role in the Flash Crash. These instruments, along with the associated ETFs, experienced significant selling pressure,furthercontributingtothemarket turmoil.
TheFlashCrashof2010hadimmediateand long-term consequences, as well as leading toimportantlessonsformarketparticipants andregulators:
Investor Losses and Market Confidence: Many investors incurred substantial losses during the Flash Crash. Forced liquidations at unfavourable prices and erroneous trades resulted in financial damage and erodedmarketconfidence.Theeventraised questions about the reliability and stability offinancialmarkets.
: In response to the Flash Crash, regulators implemented various measures to address vulnerabilities exposed by the event.
Circuit breakers were introduced to temporarily halt trading during periods of extreme volatility, allowing markets to cool downandpreventingfurtherdisruptions.
Trading rules were revised to avoid erroneous trades and enhance price transparency.
Additionally, regulators focused on improving oversight of high-frequency trading activities, including the introduction ofregistrationrequirementsforHFTfirms.
The Flash Crash of 2010 revealed the vulnerabilities and risks associated with high-frequency trading, market fragmentation, and liquidity imbalances. Thishistoriceventpromptedregulatorsand marketparticipantstoimplementmeasures to enhance market resilience and integrity. While significant progress has been made, the ever-evolving landscape of financial marketsnecessitatesconstantvigilanceand proactivemeasures.
Continued Market Resilience and Monitoring: The Flash Crash emphasized the need for ongoing vigilance and monitoringofmarketstructuresandtrading activities. Market participants and regulators must adapt to rapid technological advancements and evolving marketdynamics,continuallyassessingrisks andimplementingappropriatesafeguards.
The lessons learned from the Flash Crash serve as a reminder of the importance of robust risk management, effective oversight, and continuous efforts to maintainthestabilityandintegrityofglobal financial systems. By remaining attentive and responsive to emerging challenges, market participants and regulators can worktogether to minimize the likelihood of futureepisodessimilartotheFlashCrashof 2010.
The American company Knight Capital Group offered institutional sales and trading, electronic execution, market creation,andotherglobalfinancialservices. With a market share of over 17% on the Nasdaq Stock Market and the New York Stock Exchange (NYSE), Knight was the largest trader of American stocks in 2012. Over 3.3 billion deals were executed on average every day by Knight's Electronic Trading Group (ETG), totalling more than $21billionindailytrading.
the first hour of trading, Knight purchased 150 different stocks for about $7 billion thankstothemalfunctioningsoftware.
Building Knight Capital Group into one of the top trading firms on Wall Street required 17 years of devoted effort. And in lessthananhour,itallalmostended. Every CEO's worst dread is what occurred to Knight Capital on the morning of August 1, 2012:The companywas in danger of failing due to a plain human error that was obvious in hindsight but practically challenging to detect beforehand. When the New York Stock Exchange (NYSE) opened that day, specific new trading software at Knight had a bug that wasn't discovered until after it was launched. In
The Dow Jones Industrial Average (DJIA) droppedapproximately1000pointsinjusta few minutes during May 6, 2010, Flash Crash, which prompted the SECto establish various new rules to control securities trading.First,circuitbreakerswererequired to halt trade if the market experienced "significantpricefluctuations"ofmorethan 10%throughout5minutes.Second,theSEC mandated more precise terms controlling transaction cancellation. Trades could be cancelledforeventsinvolvingfivetotwenty stocksiftheydeviatedmorethan30%from the "reference price," the last sale before pricing was disrupted. Trades could also be cancelled for events involving more than twenty stocks if they deviated more than 10% from the reference price. Third, the Securities Exchange Act Rule C.F.R. 240.15c3-5 ("Rule") became effective and mandated the implementation of risk management controls by exchanges and broker-dealers to maintain the integrity of their systems and executive evaluation and certification of the authorities. Few of the stocks Knight traded on that fateful day exceeded the 10% price change threshold; thus, the Flash Crash regulations, which were intended to stop trading based on price movements rather than trading volume,didnotfunctionasplanned.
Computer analysts at the NYSE saw that marketvolumesweretwiceashighasusual andlinkedtheincreaseinvolumetoKnight.
The NYSE then notified Knight's CIO, who convened the company's top IT personnel; in most trading companies, a kill switch in their algorithms would have been turned, or systems would have simply been shut down.It,therefore,struggledinthedarkfor another 20 minutes before concluding that the issue was with the new code. Knight had reverted back to the old code, which was still running on the eighth server and reinstalled it on the others because the "old" version purportedly worked. Even thoughthedamagehadalreadybeendone, night engineers discovered the cause after it had been deployed. They then shut off SMARS on all the servers. Knight had made over 4 million trades involving more than 397 million shares in 154 equities. It also assumed a net long position of about $3.5 billion in 80 stocks and a net short position of around $3.15 billion in 74 stocks. Most deals would stand and could not be cancelled since they were within the 10% price zone, as the NYSE's post-flash crash regulations required. That day, Knight's shares fell 33%, resulting in a mark-tomarket loss of more than $460 million for its trades. Other market participants may smell the blood in the water because news onWallStreetspreadsquickly.
Knight attempted to have the trades reversed. Mary Schapiro, the chairman of the Securities and Exchange Commission (SEC), made the proper choice by refusing to permit this for most of the stocks in question. After the "flash crash" of May 2010, guidelines were created to specify whentradesshouldbecancelled.Exceptfor six equities, Knight's buying spree did not cause the price of the purchased stocks to
increase by more than 30%, the cancellation threshold. All of those transactions were undone. The trades were upheld in the other cases. This was terrible newsforKnight,butitwasonlysuitablefor its trading partners, who had willingly sold their shares to Knight's computers. Knight's deals were not like those made during the flash collapse when shares of some of the biggest firms in the world suddenly started tradingforaslittleasapenny,andnobuyer could feign that the price they paid for the stock represented its actual market worth. Knight was forced to sell all the equities it had purchased once it became apparent that the trades would stand. A big sale into the market would have driven the price of those shares down to a point where Knight would not have been able to cover the losses, just as the morning's rampant buyinghaddone.GoldmanSachspurchased Knight'sunwantedpositionfor$440million, a considerable loss but one the company might be able to bear. If Knight had failed, Goldman would have been the only entity harmedexceptKnight'sshareholders.
Selling the unintentionally bought shares was just the beginning of Knight CEO Thomas Joyce's fight to keep his business alive. Without a cash influx, the deals had severely depleted the firm's capital, forcing it to drastically scale back its operations or even shut down completely. Customers were also at risk of leaving the company if they didn't believe in its ability to manage its finances and operations as word of the software disaster spread. A week later, a groupofinvestorsgaveKnight$400million, andbythefollowingsummer,acompetitor, Getco LLC, had bought the company. This case study will go into the circumstances that led to the disaster, what went wrong andwhere.
The Brexit referendum, which took place on June 23, 2016, had a significant impact on the value of the British pound sterling (GBP). Algorithmic trading, also known as automated or high-frequency trading, playedaroleinamplifyingthevolatilityand speed of market movements during this period.
computer programs to execute trades based on predefined rules and algorithms. DuringtheBrexitperiod,variousautomated trading strategies were employed by market participants to take advantage of short-term market movements. These strategies included trend following, momentum trading, and statistical arbitrage.
3.LiquidityandMarketDepth:
Algorithmic trading can enhance liquidity and market depth by providing continuous buying and selling pressure. However, duringtimesofextrememarketstress,such as the Brexit referendum, liquidity can dry up as algorithmic traders rapidly adjust their positions or exit the market. This can exacerbate price movements and lead to moresignificantpriceslippage.
4.FlashCrashofOctober7,2016:
1.VolatilityandMarketReaction:
The announcement of the Brexit referendum and subsequent campaigning created uncertainty in financial markets. As the referendumdate approached, polls and political developments began influencing market sentiment, leading to increased volatility in currency markets, including the GBP.
2.AutomatedTradingStrategies:Algorithmic tradingrelieson
One notable event that highlighted the impact of algorithmic trading on the GBP was the "flash crash" that occurred on October 7, 2016. During Asian trading hours, the pound experienced a rapid and significant decline against the US dollar within a short period of time. While the exact cause of the flash crash is still debated, algorithmic trading, particularly stop-loss orders triggering automated selloffs,isbelievedtohaveplayedarole.
5.RoleofNewsandSentimentAnalysis:
Algorithmic trading systems often incorporatenewsand sentiment analysisto make trading decisions. During the Brexit period, news releases, opinion polls, and political developments had a significant impact on market sentiment. Algorithmic trading systems could react to this news in
real-time, amplifying market movements andexacerbatingvolatility.
one hand, it made UK exports more competitive,benefitingsomeindustriesand boosting exports. On the other hand, it increased the cost of imported goods, which contributed to inflationary pressures andimpactedconsumerpurchasingpower.
The Brexit referendum and the subsequent market reactions had a significant impact on the value of the pound sterling (GBP). HerearesomeeffectsoftheBrexitcrashon thepoundsterling:
1.ImmediateDepreciation: The immediate aftermath of the Brexit referendum saw a sharpdepreciationofthepoundsterling.As the outcome of the referendum signalled the UK's decision to leave the European Union, market uncertainty and concerns about the economic consequences led to a sell-off of the pound. The GBP experienced a substantial decline against major currencies, including the US dollar and the euro.
2. Volatility: The Brexit crash introduced increased volatility into the currency markets, particularlyforthepoundsterling. Uncertainty regarding the future relationshipbetweentheUKandtheEU,as well as the potential impact on trade, investment,andtheoveralleconomy,ledto significant swings in the value of the GBP. Volatility persisted throughout the negotiation period and subsequent developmentsintheBrexitprocess
3. Economic Impact: The depreciation of the pound sterling had both positive and negative effects on the UK economy. On
4.InvestorConfidence: TheBrexitcrashand the uncertainty surrounding the negotiations and future trade agreements affected investor confidence in the UK. Foreign investors, concerned about the potential disruption to trade and access to the EU market, may have reduced their investments in the UK. This could have implications for foreign direct investment, business expansion, and overall economic growth.
5. Policy Responses: In response to the Brexitcrashandtheeconomicuncertainties it created, the Bank of England implemented monetary policy measures to stabilise the economy. These measures included interest rate adjustments and quantitativeeasingprograms.Theobjective was to provide support to the economy, manageinflation,andstimulategrowth.
6. Long-Term Outlook: The impact of the Brexit crash on the pound sterling is ongoing and will depend on the outcomes of the Brexit negotiations, trade agreements, and the overall economic performanceoftheUK.
The pound's value may continue to be influenced by market sentiment, economic indicators, and developments in the relationship between the UK and the EU. It isworthnotingthattheimpactoftheBrexit crash on the pound sterling is a complex and evolving situation, and the effects can vary across different sectors of the economy.
China's share market started to fluctuate in early July 2014. The Shanghai Composite Index surged by 152% in less than a year, from2050to5166pointsbyJune12,2015, as Chinese stock prices rose nearly unabatedly. Millions of middle-class and working-class Chinese families placed substantial bets on stocks, frequently using borrowed funds, which helped the market growevenmore.
ButonJune15,themarketabruptlystarted to decline, and in just 17 trading days, the indexfellby32%,from5166to3507points.
A second, more severe downturn began on August 18 after a brief period of volatility, during which the Shanghai market dropped byover27%injustseventradingdays.
Investors faced severe losses, losing on average more than $15,000 each. Two hundred thirty-eight thousand eight hundred people invested more than 5 million RMB (about 0.77 million US dollars) in the Chinese stock markets in May 2015; at the end of August, that number had fallento125,500.BetweenJulyandAugust, the number of accounts holding between 15,000 and 77,000 US dollars decreased by 869,600andover700,000.
Due to the stock market crash, a general market downturn swiftly expanded to the fund sector. First, the value of those funds' equities fell, lowering their net asset value. The CSMAR database shows that from May toAugust2015,theaveragenetassetvalue of equity and hybrid funds decreased by over 19%, and 25% of the funds saw value lossesofmorethan44%.
Second, investors largely withdrew their money because of poor fund performance. Due to these redemptions, stock prices continued to decline due to fund managers having to sell equities to manage their liquidityrisk.
During this catastrophe, the Shanghai and Shenzhen Stock Exchanges lost a combined market value of about 5 trillion US dollars.
Significant financial losses were incurred due to the stock market crash and the
accompanying fund redemptions, which promptedmanyfundinvestorstoaltertheir preferredinvestingmethods.
BlackMondayandTuesday
Shanghai's main share index lost 8.49% of its value on August 24. As a result, the global stock markets suffered enormous losses, and some foreign pundits dubbed that day "Black Monday." On August 25, therewerecomparablelossesofmorethan 7%, which led some observers to refer to thedayas"BlackTuesday."
The sudden depreciation of the yuan on August11startedaseriesofeventsthatare the immediate cause of all this. Since then, globalstockpriceshavefallenbymorethan $5 trillion. Disappointing statistics from Friday that revealed a dramatic slowdown in industrial activity in China and the Chinesegovernment'sinabilitytoannounce anysignificantnewmarket actionstodayto support equities prices appear to be the leading causes of today's Chinese market collapse.
Other emerging countries, particularly those whose growth models depend on Chinese demand for industrial and other commodities, have come under pressure due to a deteriorating forecast for Chinese growth and a decline in the value of the Chineseyuan.
The Fed has been prepping the global economy for the first interest rate increase in nearly a decade in September and has also put pressure on emerging markets. A stronger currency, fewer capital inflows to large emerging economies, and more challenging loan repayment terms for businesses and governments with dollardenominated debt all affect America's tightermonetarypolicy.
In other words, the global economy is undergoing a massive transformation as China strives to rebalance and prosperous economies try to normalize their policies. Markets are trembling due to the difficulty authoritieshavemanagingthatchange.
The China Purchasing Managers' Index (PMI), a closely followed indicator of national manufacturing activity, was reported to have fallen to 51.5 in August 2015byCaixinMedia.Thismarkedthestart of a slump that persisted until December 2015, with the PMI dropping below 50, which denotes a slowdown. The financial
information organization creates PMIs, which are economic indicators, from monthly polls of companies' purchasing managers.
Givenitsbreath-takingriseintheyear'sfirst half, a decline in the Chinese stock market seemed unlikely. To put its drop in perspective, remember that the Shanghai Composite is still up 43% from a year ago. At least in the medium term, the ripple effectsofmarketturbulenceshouldbekept toaminimum.
appears improbable in the interim. Asian governments are much more equipped to handle this economic climate change. Currency pegs that caused problems in the late 1990s have mostly been replaced with floating-rate systems, and financial institutions are now better run and more stableoverall.Neitherdoesafinancialcrisis comparabletothatof2008seemimminent. More robust than it was just before the financial crisis, the world's banking system is now. The mispricing of entire classes of risk assets and the interdependence of weak financial institutions, both of which contributed to the panic of 2008, are no longer present. The period of galloping emerging-market expansion that started around 2000 may finally end due to the currentglobalmarketmeltdown.
Shares represent a relatively small portion of Chinese wealth.Morereal estate is held, whose market has stabilized recently. The Chinese government still has room to make some of its most potent measures, such as lowering bank reserve requirements. A repeat of the Asian financial crisis 1997
The emerging-market growth that was fuelled by Chinese growth, low-interest rates, and surging commodity prices has been yanked away, leaving the developing world to deal with the aftereffects, as evidenced by the falling emerging-market indices and currencies, from Brazil to Turkey and Kazakhstan.Prosperous economiesaretheonlysource of economic growth now that emerging markets are struggling and China's rebalance still incomplete.Thatscenarioisunsettling
Introduction:
Black Monday refers to the infamous stock market crash that occurred on October19,1987.Itwasoneofthemost significant and severe financial crises in modern history. On that day, stock markets around the world experienced a suddenanddramaticdecline,resultingin massive losses for investors and financial institutions.
The crash began in the Asian markets, specifically in Hong Kong, and quickly spread to European and American markets. The Dow Jones Industrial Average(DJIA),akeyindicatoroftheU.S. stock market, plummeted by a record 22.6% in a single trading day, marking the largest one-day percentage drop in itshistory.
to higher borrowing costs. This raised fears that higher interest rates would slow down economic growth and corporateearnings.
Another factor was the proliferation of computerized trading and the use of program trading strategies. Automated tradingsystems,whichreliedoncomplex algorithms, exacerbated the market downturn by triggering a cascade of sell orders as stock prices began to decline. Thisamplifiedthedownwardpressureon the market. The crash had profound implications for global financial markets andeconomies.Itledtosignificantlosses for individual investors, financial institutions, and pension funds. Confidence in the financial system was shaken,andtherewereconcernsabouta potential economic recession. However, central banks and governments took swift action to stabilize the markets and prevent a deeper crisis. In the aftermath of Black Monday, regulators implemented various measures to address the vulnerabilities that contributed to the crash. These included the introduction of circuit breakers, which temporarily halt trading during periods of extreme market volatility, and enhancedriskmanagementpractices.
Several factors contributed to the Black Monday crash. One primary cause was the increasing concern over rising interest rates in the United States. The Federal Reserve had been tightening monetarypolicytocurbinflation,leading
BlackMondayremainsaseminaleventin financial history, serving as a stark reminder of the inherent volatility and
risks associated with the stock market. It serves as a valuable lesson about the importance of robust risk management, regulatory oversight, and the interconnectedness of global financial markets.
The Black Monday stock market crash of 1987 was influenced by several factors, which combined to create a perfect stormoffinancialturmoil.Herearesome ofthekeyfactorsthataffectedthecrash:
OvervaluationandAssetBubbles: Before the crash, stock markets, particularly in the United States, had experienced a prolonged period of significant growth. This led to a situation of overvaluation, with stock prices surpassing the underlying fundamentals of the companies. Asset bubbles had formed in varioussectors,includingtechnologyand real estate, creating an unsustainable situation.
Rising Interest Rates: The U.S. Federal Reserve, under the leadership of Chairman Alan Greenspan, had been gradually increasing interest rates to combat inflationary pressures. Higher interestratesmeantincreasedborrowing costs for businesses and individuals. This raised concerns that economic growth would slow down, affecting corporate earnings and triggering a market correction.
These automated trading programs relied on complex algorithms to execute large volumes of trades. As stock prices began to decline, these programs automatically triggered a cascade of sell orders, intensifying the market downturn.
GlobalizationandInterconnectednessof Markets: The globalization of financial marketsmeantthatdevelopmentsinone part of the world could have an immediate and profound impact on markets globally. The crash originated in the Asian markets, particularly Hong Kong, and quickly spread to European and American markets due to the interconnectivityoffinancialsystems.
At the time of the crash, there were no mechanisms in place to halt trading temporarily during periods of extreme market volatility.Thisabsenceofcircuitbreakers meantthatthemarket'sdownwardspiral continued unabated, leading to panic selling and further exacerbating the decline.
Investor
Factors: Market psychology played a significant role in the crash. As prices began to fall rapidly, panic and fear gripped investors, leading to widespread selling. The fear of missing out on potential gains turned into a fear of significant losses, creating a selfreinforcingcycleofsellingpressure.
Program Trading and
Systems: The increasing use of computerized trading systems and program trading strategies played a significant role in exacerbating the crash.
Regulatory and
Responses: The crash highlighted the need for improved regulatory oversight and risk management in financial markets. In response, regulatory bodies
implemented measures such as circuit breakers and enhanced monitoring of program trading. Central banks also stepped in to provide liquidity and stabilizemarkets.
These factors combined to create a severe market downturn on Black Monday, leading to substantial losses for investors and financial institutions. The crashservedasawake-upcallformarket participants and regulators, resulting in changes to market structure and risk managementpractices to prevent similar eventsinthefuture.
held by an investor declines, the margin requirement may increase, leading to margin calls. During the crash, as stock prices plummeted, investors faced substantial losses, which eroded the value of their holdings. This triggered margin calls from brokers, demanding additional funds to meet the increased margin requirements. Investors who were unable to fulfil these margin calls were forced to sell their securities to repay the borrowed money, resulting in further selling pressure and contributing tothedownwardspiralofstockprices.
During the Black Monday stock market crash of 1987, margin calls and liquidity issues played a significant role in exacerbating the downturn and intensifying the financial turmoil. Here's anexplanationofthesefactors:
Margin Calls: Margin trading allows investors to borrow money from their brokers to purchase securities. Investors are required to deposit a certain percentage of the total value of the investment as collateral, known as margin. When the value of the securities
Liquidity Issues: Liquidity refers to the ability to buy or sell assets quickly without causing significant price fluctuations. During the Black Monday crash,liquiditybecameacriticalconcern. As stock prices fell rapidly, investors rushed to sell their holdings, overwhelming the market with sell orders.Thehighsellingpressureandlack of buyers led to a liquidity crunch. The market depth, or the number of willing buyersandsellers,becameinsufficientto absorb the flood of selling orders. This led to sharp price declines and increased volatility. Additionally, market makers and specialists, who provide liquidity by buyingandsellingsecurities,struggledto keep up with the overwhelming selling pressure, exacerbating the liquidity issues.
The combination of margin calls and liquidity issues created a vicious cycle. Margin calls forced investors to sell their securities,whichfurtherincreasedselling pressureanderodedliquidity.Thelackof liquidity, in turn, made it difficult for investors to exit their positions, leading tomorepanicselling.
These factors contributed to the severity and speed of the stock market crash on Black Monday. The forced selling due to margin calls and the strain on liquidity intensified the downward spiral of stock prices, resulting in significant losses for investors and financial institutions. The crash highlighted the importance of managing margin risk and maintaining adequate liquidity in financial markets to preventsuchcascadingeffects.
The Black Monday stock market crash of 1987 remains one of themost significant events in financial history. It serves as a stark reminder of the inherent risks and vulnerabilities within the global financial system. Here are some key points to conclude the impact and lessons from BlackMonday:
Factors at Play: Several factors contributed to the crash. These included concerns over rising interest rates, overvaluation, asset bubbles, the proliferation of computerized and program trading, the interconnectedness of global markets, and psychological factorssuchaspanicandfear.
MarginCallsandLiquidityIssues: Margin calls and liquidity problems exacerbated the crash. Margin calls forced investors to selltheirsecurities,intensifying selling pressure and contributing to the downward spiral. Liquidity issues arose as market depth proved insufficient to absorb the flood of selling orders, resultinginaliquiditycrunch.
Regulatory and Policy Responses: In response to the crash, regulators and
policymakers implemented measures to address the vulnerabilities that contributed to the turmoil. Circuit breakers were introduced to temporarily halt trading during periods of extreme volatility. Risk management practices were enhanced, andregulatoryoversight was strengthened. Central banks provided liquidity to stabilize the markets.
Lessons Learned: The Black Monday crash underscored the importance of robust risk management, adequate liquidity, and regulatory oversight in financial markets. It highlighted the risks associated with overvaluation and asset bubbles, as well as the potential impact of computerized trading systems. The crash also emphasized the interconnectedness of global markets andtheneedforcoordinatedresponses.
Lasting Impact: The crash had profound implications for investor confidence, the financial industry, and the broader economy. It led to reforms and improvements in market structures and risk management practices. Lessons learned from Black Monday influenced subsequent responses to financial crises, shaping regulatory frameworks and risk mitigationstrategies.
Overall, the Black Monday stock market crash was a significant event that demonstrated the fragility of financial markets and the potential for rapid and severe downturns. It serves as a historical reminder of the importance of prudent risk management, sound regulatory oversight, maintaining market liquidity to prevent and mitigate future financialcrises
On May 6, 2010, the global financial markets experienced one of the most dramatic and bewildering events in recent history the 'Flash Crash.' Within a matter ofminutes,majorstockindicesplummeted, only to recover just as quickly, leaving investors and regulators scrambling for answers.Attheheartofthisunprecedented event was the role of algorithmic trading, which had gone awry, exposing the vulnerabilities of an increasingly complex andinterconnectedfinancialsystem.
Algorithmic trading, also known as highfrequency trading (HFT), had been gaining prominence in the years leading up to the Flash Crash. This practise involves using sophisticated computer algorithms to execute trades at lightning-fast speeds, often measured in milliseconds. The algorithms analyse vast amounts of data, seeking patterns, and making split-second tradingdecisionsbasedonpredefinedrules. The goal is to take advantage of small price discrepancies and capitalise on market movementsbeforehumantraderscaneven react.
TheFlashCrashbeganlikeanyothertrading day but quickly spiralled into chaos. At approximately 2:32 p.m. Eastern Time, the Dow Jones Industrial Average (DJIA) suddenly plummeted nearly 1,000 points, representing a loss of around 9% in just a matterofminutes.Panicspreadthroughout
the market, triggering a wave of selling as investorsrushedtocuttheirlosses.
One of the key factors contributing to the rapid decline was the cascading effect of stop-loss orders being triggered. Stop-loss orders are automated instructions that prompt a trade to be executed once a specificpricelevelisreached.Asthemarket started to decline, a large number of these orders were triggered, flooding the market with sell orders and exacerbating the downwardpressure.
Further compounding the issue were the interactions between various algorithmic trading strategies. The intense competition between different algorithms led to a feedback loop, where selling begot more selling. As prices dropped, algorithms reacted by intensifying their sell orders, further driving down prices in a selfreinforcing cycle. Amidst this chaos, liquidity evaporated. The sudden surge in sell orders overwhelmed the market, causing bid-ask spreads to widen significantly.Thismadeitdifficultforbuyers to find sellers at reasonable prices, exacerbating the downward spiral. The lack of liquidity also resulted in a temporary breakdown of normal price discovery mechanisms, with some stocks trading at absurdly low prices or even at one cent. However, as quickly as it had begun, the
Flash Crash came to an end. Within minutes, the market began to recover, erasingmostofthelosses.Bytheendofthe trading day, the DJIA had only declined about 3%, but the shockwaves of the event reverberatedformuchlonger.
In the aftermath of the Flash Crash, regulators launched extensive investigations to determine the cause and prevent similar events in the future. The U.S. Securities and Exchange Commission (SEC) and the Commodity Futures Trading Commission (CFTC) conducted a joint investigation, analysing trading data and interviewingmarketparticipants.
Their findings highlighted several contributingfactorstothe FlashCrash. One crucial element was the market's lack of proper risk controls and circuit breakers. Circuit breakers are mechanisms that halt tradingtemporarilywhencertainthresholds are breached, allowing time for market participants to reassess their strategies. At the time of the Flash Crash, the existing circuit breakers were ill-equipped to handle the rapid market decline and failed to act promptly.
Another critical factor was the design of certain algorithmic trading strategies. Some algorithms were programmed to aggressively pursue market share and execute trades regardless of the prevailing market conditions. These strategies lacked adequate checks and balances, leading to excessive volatility, and exacerbating the downward pressure. Furthermore, the interconnectedness of global financial markets played a role in amplifying the effects of the Flash Crash. In a highly interconnected system, where trades are executed across multiple venues and countries, the rapid transmission of
information and trading activities can exacerbate market movements. The Flash Crash highlighted the need for enhanced coordination and risk management among globalmarketregulators.Inresponsetothe Flash Crash, regulators implemented several reforms to mitigate the risks associatedwithalgorithmictrading.TheSEC introduced new rules that required market participants to implement risk controls, such as maximum order sizes and trading velocity limits. Circuit breakers were also revised to provide more effective safeguards against extreme market volatility. Market participants themselves took steps to improve their risk management practises. Trading firms and exchanges enhanced their pre-trade risk controls, refined their algorithms, and developed better monitoring systems to detectunusualtradingactivity.
Despite these efforts, the Flash Crash remains a stark reminder of the vulnerabilities inherent in an increasingly complex and technology-driven financial system. The rise of algorithmic trading has undoubtedly brought efficiency and liquidity to the markets ,but has also introduced new risks. As technology continues to evolve, regulators and market participants must remain vigilant, constantly adapting their practises to ensure the stability and integrity of the globalfinancialsystem.
In the annals of economic history, few events have resonated with such profound reverberations as the Eurozone Debt Crisis of 2011. Its seismic impact rippled through the intricate tapestry of global financial markets,disruptedpoliticallandscapes,and tested the very foundation of economic stability in Europe. Yet amidst the multifaceted causes and consequences of this crisis, one intricate thread weaves through the narrative: the intriguing interplay between the crisis and the realm of algorithmic trading. As we delve into the depths of this captivating connection, we embarkon a journey tounravel the enigma surrounding the Eurozone Debt Crisis, unearthing the intricate web of its aftereffects on financial markets, political conditions, and the delicate balance of economicstabilityintheheartofEurope.
To understand the algorithmic trading connection,itiscrucialtograsptheessence of the Eurozone Debt Crisis. The crisis emerged from the confluence of factors such as excessive public debt, insufficient fiscal discipline, and structural weaknesses within the Eurozone. It manifested as a sovereign debt crisis, with countries like Greece, Spain, Portugal, and Ireland facing severe difficulties in servicing their debt obligations. These nations faced difficulties
in servicing their debt obligations, leading to concerns about their solvency. The crisis exposed deep-seated issues within the Eurozone's economic and monetary framework, ultimately impacting various aspectsofEuropeansociety.
The debt crisis originated in 2008 following thecollapseofIceland'sbankingsystemand subsequently spread to Portugal, Italy, Ireland, Greece, and Spain in 2009. This led to the creation of the term "PIIGS" to refer to these countries. The crisis resulted in a significant loss of confidence in European businessesandeconomies.
To address the crisis and prevent the collapseoftheeuroandfinancialcontagion, European countries provided financial guarantees,andtheInternationalMonetary Fund (IMF) also played a role. Credit rating agencies downgraded the debts of several Eurozone countries, with Greece's debt even being downgraded to junk status. Countries that received bailout funds were required to implement austerity measures aimed at reducing public-sector debt growthaspartoftheloanagreements.
The Eurozone debt crisis was influenced by various factors, including the 2007-2008 financialcrisis,theGreatRecessionof2008-
2012, the real estate market crisis, and property bubbles in different countries. Additionally,thefiscalpolicies ofperipheral Eurozone states regarding government expenses and revenues contributed to the crisis.
By the end of 2009, Greece, Spain, Ireland, Portugal, and Cyprus, as peripheral Eurozone member states, faced challenges inrepayingorrefinancingtheirgovernment debt and supporting their troubled banks without external financial assistance. Institutions such as the European Central Bank (ECB), the International Monetary Fund (IMF), and eventually the European Financial Stability Facility (EFSF) stepped in toprovideassistance.
In 2009, it was revealed that Greece had significantly underestimated its budget deficit, violating EU policy and raising concerns of political and financial contagion, which could potentially lead to thecollapseoftheeuro.
To address the crisis, the creation of the EFSF was approved in 2010 by the seventeen Eurozone countries. Its purpose was to tackle and provide support for the on-going crisis. The peak of the European sovereign debt crisis occurred between 2010and2012.
As fears of excessive sovereign debt grew, lenders demanded higher interest rates from Eurozone countries in 2010. The high levels of debt and deficits made it more difficult for these countries to finance their budget deficits, especially with low economic growth. To combat the crisis, some affected countries implemented measures such as tax increases and spending cuts, which further contributed to
social unrest and a crisis of confidence in leadership,particularlyinGreece.
During the crisis, international credit rating agenciesdowngradedthesovereigndebtof several countries, including Greece, Portugal, and Ireland, to junk status. This exacerbated investor fears and intensified theseverityofthecrisis.
In early 2010, the impact of the crisis became evident through the increasing spreads on sovereign bond yields, particularly between affected peripheral member states such as Greece, Ireland, Portugal,Spain,andGermany.
By May 2010, Greece faced the need for assistance from the Eurozone. Subsequently, Greece received multiple bailouts from the EU and IMF, contingent upon the implementation of mandated austerity measures aimed at reducing public spending and increasing taxes. Despitethesemeasures,Greece'seconomic recessionpersisted,leadingtosocialunrest. The country faced the risk of sovereign default in June 2015 due to the divided politicalandfiscalleadership.
In the following month, the Greek citizens votedagainstabailoutandfurtherausterity measures imposed by the EU. This raised the possibility of Greece exiting the European Monetary Union (EMU). The potentialconsequencesofGreecereturning to using the Drachma ranged from a complete economic collapse to a surprising recovery.
Ultimately, Greece remained part of the EMU and gradually showed signs of
economic recovery in subsequent years. Over a five-year period, unemployment dropped from its peak of over 27% to 16%, while annual GDP transitioned from negative numbers to a projected growth rate of over two percent within the same timeframe.
event, such as a downgrade of a country's credit rating or a spike in borrowing costs, triggers a sell-off, algorithmic trading algorithms can intensify the downward spiral by automatically executing a large volume of sell orders. This can lead to a rapid decline in prices, further eroding investor confidence and exacerbating the crisis.
Algorithmic trading, with its lightning-fast execution and intricate mathematical algorithms, played a pivotal role in exacerbating the volatility and speed of the Eurozone Debt Crisis of 2011. These sophisticated trading systems, employed by financialinstitutions,hadaprofoundimpact on market dynamics, intensifying the contagioneffectandamplifyingtheseverity of the crisis. One of the key characteristics of algorithmic trading is its ability to execute trades at extraordinary speeds, far surpassing the capabilities of human traders. This high-speed trading created an environment where market movements occurred with unprecedented swiftness, leaving little time for investors to react and adjusttheirpositionsaccordingly.
While these algorithms aim to maximize profits, they can also contribute to market fragility. For instance, when a significant
Another aspect of algorithmic trading is its role in amplifying the contagion effect within the Eurozone. The interconnectedness of financial markets enabled the swift transmission of financial distress from one country to another. As news of fiscal troubles in one country broke, algorithmic trading algorithms reacted by initiating sell orders not only for the affected country's assets but also for assets of other vulnerable economies. This createdadominoeffect,asthealgorithmicdriven sell-offs in one market spilled over into others, deepening the crisis and spreadingpanicamonginvestors.
Furthermore,algorithmictradingalgorithms are often programmed to engage in highfrequency trading, where trades are executed in rapid succession within short timeframes. This approach to trading can amplify market volatility, as high-frequency traders exploit small price differentials and capitalize on short-term market fluctuations.Intimesofcrisis,therapid-fire trading actions of algorithmic traders can exacerbate price swings, making it increasingly challenging for investors to accurately assess market sentiment and make informed decisions. The reliance on algorithmic trading during the Eurozone Debt Crisis also revealed potential vulnerabilities in the financial system. The
interconnectedness of financial institutions, many of which employed algorithmic trading strategies, intensified the impact of market downturns. As losses mounted, banks faced liquidity challenges, leading to atighteningofcreditavailabilityandfurther exacerbatingtheeconomicdownturn.
BankFragility:
European banks were heavily exposed to the sovereign debt of troubled countries. The crisis eroded confidence in the banking sector, leading to liquidity problems and even bank failures. This instability put significant strain on the broader financial system. The reliance of banks on algorithmictradingexacerbatedthefragility of the European banking system during the crisis. Many financial institutions were heavily exposed to sovereign debt and engaged in high-frequency trading, leading to severe losses. As banks faced liquidity challenges, credit tightened, worsening the overalleconomicsituation.
marketreforms,leadingtosocialunrestand politicalturmoil.
b. Rise of Populism: The Eurozone Debt Crisis contributed to the rise of populist movements across Europe. Citizens, frustrated with the handling of the crisis, directed their anger towards traditional political establishments, resulting in the growthoffar-rightandfar-leftparties.
c.ChallengestoEuropeanIntegration:
The crisis exposed the weaknesses of the Eurozone's institutional framework. It heightened tensions between stronger and weaker economies and raised questions about the future of European integration. The crisis led to debates on the need for deeper fiscal and political integration to preventsimilareventsinthefuture.
a. Recession and SlowRecovery: The crisis plunged several Eurozone countries into recession, with declining GDP, rising unemployment, and reduced consumer spending. The road to recovery was long and arduous, with some countries still grappling with the consequences of the crisis.
a.AusterityMeasures:
In response to the crisis, many heavily indebted countries implemented austerity measures to reduce their budget deficits. These measures often involved unpopular spending cuts, tax increases, and labour
b.StructuralReforms: To restore economic stability, many European countries implemented structural reforms aimed at enhancing competitiveness, increasing productivity,and improving publicfinances.
These reforms often faced resistance from vestedinterestsandsocietaldivisions.
c. Long-term Implications: The Eurozone Debt Crisis of 2011 had long-term implicationsforEurope'seconomicstability. It exposed the need for stronger fiscal discipline, improved financial regulation, and better crisis management mechanisms. It also highlighted the importance of addressing economic disparities within the Eurozone.
Bottom-line:
The Eurozone Debt Crisis of 2011 had farreaching consequences on financial markets, political conditions, and economic stability in Europe. In the aftermath of the crisis,significantpoliticalandsocialchanges unfolded acrossEurope.Thepublic, already grappling with the economic fallout, directed their frustration towards traditional political establishments. The perception that the crisis was mishandled andinfluencedbyfinancialelitesfuelledthe rise of populist movements. Antiestablishment parties gained traction, challenging the existing political order and fuelling Euro scepticism. This political upheaval created additional complexities in navigating the path towards recovery and implementingnecessaryreforms.
economic and monetary framework. The crisis highlighted the need for stronger fiscal discipline, improved financial regulation, and enhanced crisis management mechanisms. Efforts were made to strengthen economic governance within the Eurozone, such as the establishment of the European Stability Mechanism (ESM) to provide financial assistance to member states in distress.
Achieving lasting economic stability required comprehensive reforms and a critical reassessment of the Eurozone's
Additionally, reforms focused on structural adjustments, including labour market reforms and fiscal consolidation measures, to enhance competitiveness and improve public finances. The crisis also underscored the importance of addressing economic disparities within the Eurozone. Structural reforms aimed at promoting growth and reducing economic imbalances became essentialtoachieveamoreharmonizedand resilient Eurozone. Greater coordination and cooperation among member states were necessary to mitigate the risk of future crises and foster economic stability. While algorithmic trading did not directly cause the crisis, it played a role in exacerbating market volatility and contagion effects. The crisis led to significant political and social changes, with the rise of populism and challenges to European integration. Achieving lasting economic stability required extensive reformsandarevaluationoftheEurozone's economic and monetary framework. By learning from the lessons of the past, policymakers can strive to build a more resilient and integrated Europe, better equipped to navigate future challenges and mitigate the potential risks associated with algorithmictrading.
"In the world of trading, algorithms are the silent heroes, executing strategies with speed, precision, and tireless efficiency, transforming markets into a symphony of numbers and opportunities."
This article analyses the role of algorithmic trading during the COVID-19 market crash based on available information up until 2021. The nature of financial markets and algorithmic trading continues to evolve, and further research may be necessary to gain a comprehensive understanding of their interplay. The COVID-19 pandemichad a profound impact on global financial markets, leading to a significantmarketcrashinearly2020.
2019. As the virus spread rapidlyacross the globe, governments implemented strict lockdown measures, travel restrictions, and social distancing guidelines to curb its transmission. These measures resulted in severe disruptions to economic activity and supply chains, leading to widespread concerns among investors and a sharp declineinmarketindices.
The COVID-19 pandemic had a significant impact on global financial markets in 2020. Intheearlymonthsoftheyear,asthevirus spread rapidly worldwide, countries implemented lockdown measures and travel restrictions to contain its transmission. These measures had severe consequences for businesses and economies, leading to a major market downturn often referred to as the "COVID19marketcrash."
Introduction: The Covid-19 Market Crashof2020
The outbreak of COVID-19, caused by the novel coronavirus SARS-CoV-2, was first identified in Wuhan, China, in December
Initial Sell-Off: In late February and early March 2020, global stock markets experienced a swift and severe sell-off as investors grew increasingly worried about theeconomicimpactofthepandemic.
Stock indices, such as the S&P 500, Dow Jones Industrial Average, and major European and Asian indices, witnessed steep declines, with many entering bear marketterritory(adeclineof20%ormore).
GlobalStockMarketDecline: Stockmarkets around the world experienced sharp declines, with major indices plummeting in ashortperiod.IntheUnitedStates,theS&P 500 and Dow Jones Industrial Average both fell into bear market territory, experiencing their fastest-ever descent from record highs.
Volatility and Liquidity Issues: The market crash was accompanied by extreme volatility, with daily price swings reaching recordlevels.
increased demand for remote work solutions,telemedicine,ande-commerce.
Government Intervention and Stimulus: Central banks and governments worldwide responded with unprecedented measures to stabilize financial markets and support economies. Major central banks, including the Federal Reserve, European Central Bank, and Bank of England, implemented interest rate cuts and launched large-scale asset purchase programs (quantitative easing). Governments introduced fiscal stimulus packages, including direct financial aid to individuals and businesses, loans, tax cuts, and infrastructure spending, to mitigate the economic impact of the pandemic.
Investors faced liquidity issues, as market participants rushed to sell their assets, leading to strained liquidity conditions and difficultyinexecutingtrades.
Sectoral Impact: The pandemic's impact varied across sectors. Travel and tourism, hospitality, retail, and energy were among the hardest hit, as lockdowns and travel restrictions severely curtailed demand. Conversely, sectors such as technology, healthcare, and online retail experienced relativeresilienceorevengrowth,drivenby
Understanding Algorithmic Trading: Algorithmic trading refers to the use of computer algorithms to execute highspeed, automated trading decisions. These algorithms analyse large volumes of data, including market trends, news, and other relevant information, to generate trade orders. The aim is to capitalize on small price discrepancies and execute trades swiftly, often in fractions of a second. The COVID-19 pandemic unleashed a wave of marketturmoilin2020,causingasignificant crash in global financial markets. Amidst this unprecedented volatility, algorithmic
trading emerged as a key player in exacerbating the turbulence. Algorithmic trading, powered by computer programs executing trades based on predefined instructions, contributed to increased volatility and liquidity challenges during the crash. As investors rushed to sell their holdings, algorithmic trading algorithms amplified price swings, leading to heightenedmarketstress.
played a notable role in exacerbating market turbulence. In this article, we will delve into the COVID-19 market crash of 2020 and analyse the impact of algorithmic tradingduringthisperiodofunprecedented uncertainty.
Flash crashes, sudden and severe price declines within a short timeframe, became more frequent due to the interconnectedness of algorithmic trading systems. These crashes were triggered by unexpected interactions between algorithmsorreactionstoperceivedmarket conditions. Furthermore, algorithmic trading facilitated herd behaviour and feedback loops, perpetuating market volatility. Regulatory concerns arose, prompting the implementation of circuit breakers to halt trading during extreme volatility. As financial markets continue to evolve, it is crucial to address the risks associated with algorithmic trading and implement measures to ensure market stabilityandresilienceduringtimesofcrisis. The COVID-19 pandemic brought about an unprecedented market crash in 2020, with global financial markets experiencing extreme volatility and significant declines. Amidst this turmoil, algorithmic trading
1.Algorithmic Trading and Market Efficiency:
Algorithmic trading, also known as algo trading or automated trading, involves the use of computer programs to execute trades based on predefined instructions, such as price, timing, or quantity. Algorithmictradinghasbecomeincreasingly prevalent in financial markets due to its potential for efficiency, speed, and the abilitytoprocessvastamountsofdata.
2.IncreasedVolatilityandLiquidityIssues:
During the COVID-19 market crash, algorithmictradingcontributedtoincreased volatilityandliquiditychallenges.Asmarket participants rushedto sell offtheirholdings amidst mounting uncertainty, algorithmic trading algorithms executed a cascade of trades, amplifying price swings and exacerbating market volatility. This rapid tradingactivity,combinedwithreduced
The impact of algorithmic trading during this period of unprecedented uncertainty
liquidity and market depth, created an environmentofheightenedmarketstress.
3.FlashCrashPhenomenon:
Algorithmic trading played a role in the occurrence of flash crashes during the market crash of 2020. Flash crashes are suddenandseverepricedeclinesthatoccur withinaveryshortperiod.
prompted regulatory scrutiny and the implementationofcircuitbreakers
These crashes can be triggered by algorithmic trading programs interacting in unexpected ways or reacting to perceived market conditions. The interconnectedness of algorithmic trading systems can magnify theimpactoftheseflashcrashes,leadingto substantialmarketdisruptions.
4.HerdBehaviourandFeedbackLoops:
Algorithmic trading can contribute to herd behaviour and feedback loops, further exacerbating market volatility. As certain price levels or market signals are breached, algorithms may respond by triggering additional trades in a self-reinforcing manner. This feedback loop can amplify market movements, causing prices to deviatesignificantlyfromtheirfundamental values.
5.Regulatory Concerns and Circuit Breakers:
The increased role of algorithmic trading during the COVID-19 market crash
Circuit breakers are mechanisms that temporarily halt trading to provide a cooling-off period during periods of extreme market volatility. These circuit breakers aim to prevent disorderly market conditions and allow market participants to reassesstheirstrategies.
Conclusion
While algorithmic trading has revolutionized financial markets, the COVID-19 market crash highlighted its potential contribution to market turbulence.High-frequencytrading,herding behaviour, and liquidity challenges amplified market volatility, while the speed and automation of algorithmic trading contributed to flash crashes. Balancing market efficiency and stability remains a significant challenge for regulators and marketparticipants.
Introduction:
The2000dot-combubblecrashremainsa notable event in financial market history, marked by the fast ascent and eventual downfall of several internet-based enterprises.Whilemanycausescontributed to the crash, this paper will focus on the role of algorithmic trading and its influence on the dot-com bubble's demise. Algorithmic trading, often known as automated or high-frequency trading, is a type of trading that uses computer algorithms to make deals at fast rates. We can learn a lot about the link between technology and market volatility by looking at how algorithmic trading affected the eventsthatleduptothecrisis.
TheDot-ComBubble:
To grasp the relevance of algorithmic trading, it is necessary to first understand the beginnings of the dot-com boom. The fast rise of the internet in the late 1990s inspired enormous excitement about the possibilities of online companies. Investors flocked to technological firms, frequently bypassing traditional indicators such as profitability and earnings. As stock prices rose,themarketsawasurgeininitialpublic offerings (IPOs) for internet-based businesseswithlittleornoactualassets.
To comprehend the significance of algorithmic trading, one must first understand the origins of the dot-com boom. The rapid emergence of the internet in the late 1990s sparked considerable optimism about the potential of online businesses. Investors rushed to technical companies, frequently ignoring traditional indicationslikeprofitabilityandearnings.As stock prices increased, the market saw an increase in initial public offerings (IPOs) for internet-based enterprises with little or no assets.
Algorithmictradingcontributedsignificantly to the exacerbation of the dot-com boom and its eventual fall. One important reason was the increasing speed and volume of transactions made possible by algorithmic techniques. Algorithms enabled traders to executeordersquickly,leadingtothefrenzy ofbuyingandsellingdot-comstocks.Asthe bubble swelled, momentum-based algorithms drove upward price movements even further, creating an atmosphere in which stockvalues were disconnected from underlyingfundamentals.
Irrational excitement and herd mentality among investors created the dot-com boom. With its capacity to scan massive
quantities of data and respond to market signals in real time, algorithmic trading magnified these behavioural biases. Algorithmsbasedonmomentumandtrendfollowing tactics fuelled market frenzy, resulting in exorbitant valuations and an unsustainableupwardcycle.
As the dot-com bubble burst, the market's weakness became clear. Many online firms failed to generate revenues or had poor business ideas, making their prices unsustainable. When investor mood finally shifted, the bubble crashed quickly and severely. The market's collapse was worsened by algorithmic trading, since the same algorithms that had drove prices upward now hastened their descent. The automatic execution of sell orders caused a chain reaction of selling, increasing market volatilityandcontributingtothecrash. One of the key factors that contributed to the financial crisis was the widespread use of complex financial instruments, particularly mortgage-backed securities (MBS) and collateralized debt obligations (CDOs). These instruments were often based on subprime mortgages, which were loans given to borrowers with lower creditworthiness. When the U.S. housing market experienced a downturn, many of these mortgage-backed securities and related derivatives became highly risky and difficult to value accurately. Algorithmic trading played a role in exacerbating the crisisthroughseveralchannels:
Amplificationofmarketmovements
Herdmentality
LiquidityIssues
Riskmanagementfailures
It is important to note that algorithmic trading itself was not the root cause of the crisis. The crisis had its origins in a range of factors, including loose lending practices, inadequate regulation, and a lack of transparency in the financial system. However,theriseofalgorithmictradingand its specific characteristics amplified the effects of the crisis and highlighted the need for better risk management practices and regulatory oversight in financial markets.
The dot-com bubble burst led to introspection and regulatory responses aimed at preventing similar crises. Regulators implemented measures to enhance market stability, such as circuit breakers and stricter oversight of trading practices. Market participants also recognizedtheimportanceofmaintaininga balance between technological advancements and prudent risk management. The development of risk controls and improved oversight of algorithmic trading sought to mitigate the potentialforfuturemarketdisruptions. The Securities and Exchange Board of India, or SEBI, is the regulatory organisation in charge of monitoring the Indian securities industry. While the collapse of the dot-com bubble predominantly impacted the global market, including India, SEBI introduced a number of policies and initiatives to
improve the Indian securities market and safeguard investors. Following the dot-com bubble crash and related developments, SEBI enacted the following major regulations:
Increased Disclosure and Reporting Requirements: SEBI tightened disclosure and reporting requirements for firms listed on Indian stock markets. This featured tougher financial reporting standards, risk factor disclosure, and prompt information distributiontoinvestors.
Corporate Governance Reforms: The SEBI implemented initiatives to improve India's corporate governance practises. This included stronger standards for independent directors, audit committees, and related party transactions disclosure. These measures seektopromote corporate transparencyandaccountability.
IEPF (Investor Education Protection Fund): SEBI prioritised investor education programmes in order to enhance knowledge about investing risks, market volatility, and the significance of due diligence. They concentrated their efforts on teaching individual investors about investment products, market movements, andfinancialliteracy.
Enhancing Market Infrastructure: SEBI has taken initiatives to improve the efficiency and transparency of the Indian securities market. This entailed putting complex trading platforms, risk management systems, and monitoring procedures in place to detect market manipulation and insidertrading.
Monitoring and Surveillance: SEBI enhanced its monitoring and surveillance capacity to detect abnormalities, market
misuse, and fraudulent operations. This includes employing technologically advanced techniques for market surveillance, data analysis, and proactive riskmanagement.
Regulatory Framework for Collective Investment Schemes: To protect investors' interests, SEBI implemented stronger restrictions for collective investment schemes (CIS). This was done to avoid fraudulent schemes and to ensure that CIS operators adhere to disclosure standards andinvestmentlimitations.
Framework
Collective Investment Schemes: To protect investors' interests, SEBI implemented stronger restrictions for collective investment schemes (CIS). This was done to avoid fraudulent schemes and to ensure that CIS operators adhere to disclosure standards andinvestmentlimitations.
It's worth noting that, while these laws were enacted in the aftermath of the dotcom bubble's demise, they were also impacted by other worldwide events, such as the 2008 global financial crisis. SEBI's regulatory structure is constantly evolving in order to respond to changing market dynamicsandprotectinvestorinterests.For the most up-to-date information on SEBI rules, visit their official website or speak withacompetentfinancialadvisor.