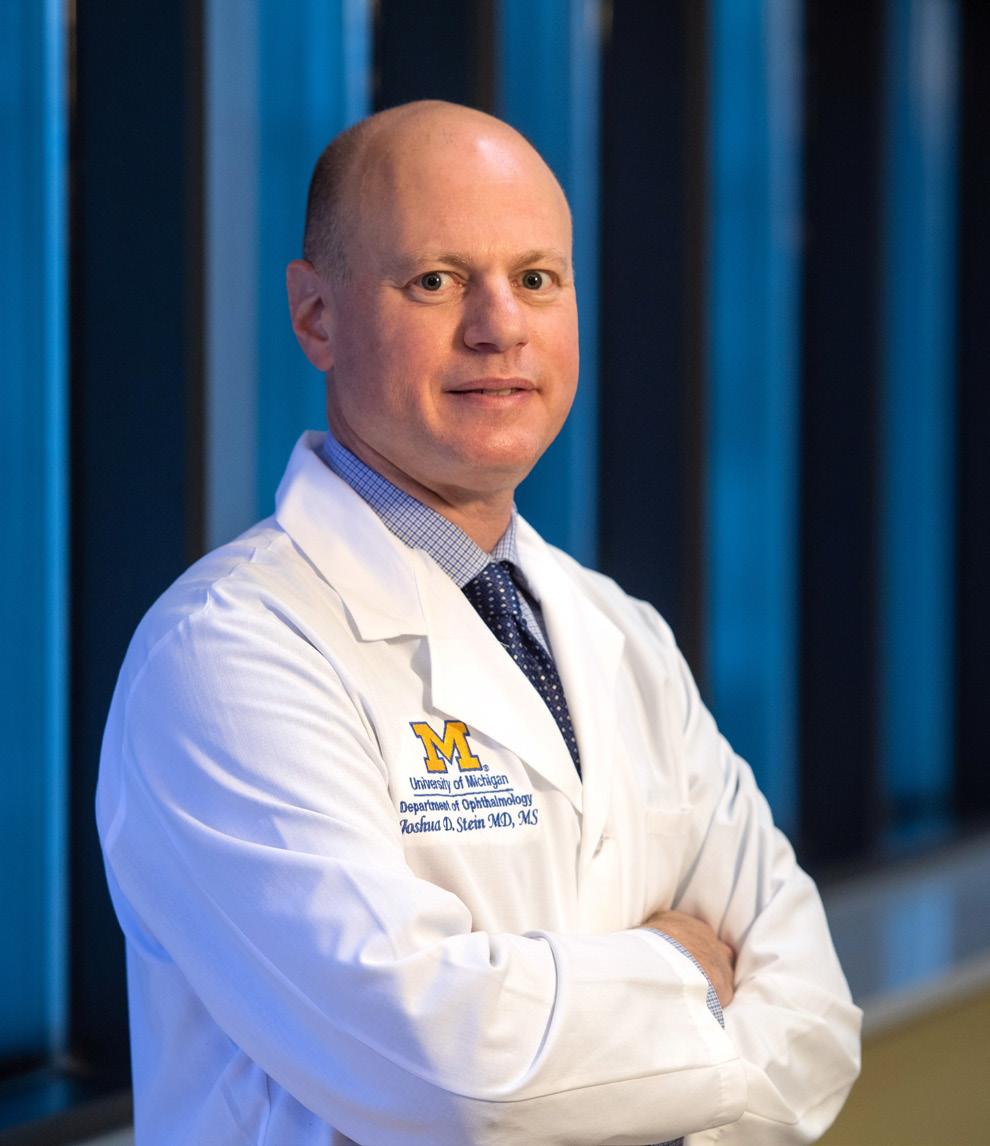
5 minute read
Beyond the Electronic Health Record
Combining the powerful number crunching capabilities of Big Data analytics with the information held in patient electronic health records (EHRs) has revolutionized health research. Investigators can now combine vast amounts of patient information and, using tools like machine learning, analyze the data to answer important questions about diseases, risk factors and treatment outcomes.
At the forefront of applying this approach to the study of ophthalmic diseases is Joshua Stein, M.D., M.S. A glaucoma specialist and health services researcher, Dr. Stein is Principal Investigator and Chief Data Officer of the Sight Outcomes Research Collaborative (SOURCE), a consortium of 14 leading academic ophthalmology departments that pool EHR and other ocular diagnostic data (removing protected health information) into a common research database housed at U-M.
The SOURCE platform is utilized in two of Dr. Stein’s latest initiatives. Both aim to improve the quality of research into the most common vision-threatening diseases, and both are supported by NIH R01 grants.
Improving Disease Identification
To leverage the power of exciting tools such as machine learning and artificial intelligence (AI) to come up with new ways to improve the quality of eye care, enhance patient outcomes, and reduce disparities in care, it is essential to train these models using high quality data. When researchers build these sophisticated models, if enough patients are misclassified as affected by a given ocular disease when indeed they do not have that condition, or vice versa, this can negatively affect the performance and usefulness of these models.
One way that a patient may be misclassified as having or not having an ocular disease is when researchers rely on administrative billing codes entered by clinicians during office visits to determine which patients are and are not affected by diseases of interest. Relying solely on billing codes to tell which patients have and do not have particular eye diseases poses a number of problems: certain eye diseases lack unique billing codes; some billing codes are not specific enough to distinguish between different conditions; and occasionally clinicians pick incorrect billing codes.
To address these problems, Dr. Stein and his team have developed a sophisticated approach that goes well beyond sole reliance on administrative billing codes. They incorporate information from multiple areas of the electronic health record to more accurately identify which patients have common sight-threatening diseases such as glaucoma, diabetic retinopathy, and age-related macular degeneration. For patients who have these conditions, Dr. Stein’s team can accurately determine the type and severity of the condition, which eye or eyes are affected, and whether the condition is stable, improving, or getting worse.
Just because an eye disease is common does not mean every patient experiences it in the same way. Similarly, different eye care professionals in different settings may document the same signs and symptoms of a condition differently in the electronic health record. These factors make it very difficult to use electronic health records to build models that can accurately predict the likelihood of a patient having a particular condition. To overcome some of these challenges, Dr. Stein’s team uses tools such as natural language processing to dive deeper into the electronic health record to locate additional relevant details from the clinic visit.
As Dr. Stein explains, “for each of the four million patients in our SOURCE Ophthalmology Big Data repository, we are able to assign a probability from 0 to 100 percent as to whether they have glaucoma, diabetic retinopathy, or macular degeneration. With accurate classifications for these patients, researchers can integrate this information into the AI models they are building to predict, among other things, which patients are most likely to experience favorable or unfavorable outcomes.” Other applications of this enhanced disease identification approach include incorporating the predictions into studies of the epidemiology of these different eye diseases, using them to determine if disparities in care exist for patients of different sociodemographic characteristics, and helping identify patients who may be eligible for randomized clinical trials of new therapeutic interventions for these conditions.
Health Disparities in Utilization, Quality and Outcomes (HealthDOC)
Dr. Stein and his team, in collaboration with researchers at Northwestern University, have also been leveraging the Sight Outcomes Research Collaborative (SOURCE) Ophthalmology Big Data Repository to study disparities and inequities in the receipt of eye care services and outcomes of eye care among racial and ethnicity minorities and other vulnerable groups. The main objective of this NIH-funded research is to determine the extent to which social determinants of health may affect quality of eye care and outcomes for patients with common sight-threatening eye diseases such as cataract, glaucoma, and diabetic retinopathy.
An innovative aspect of this project is the linking of clinical data from the electronic records of eye care recipients in SOURCE to other large pots of data. This linking provides Dr. Stein and his team a more holistic view of patients and the array of factors that may affect their care quality and outcomes. For example, by incorporating information on race, ethnicity, level of education, marital status, income, household net worth, type of health insurance, primary language and even the number of family members living in the household, the team can see how these factors may be impacting the eye care patients are receiving.
“The first step in reducing or eliminating long standing inequities in eyecare that contribute to poorer outcomes among vulnerable populations is to identify the underlying factors that are most responsible,” explains Dr. Stein. “Once we can identify such factors, especially those which are modifiable, we can develop strategies to improve care quality and outcomes for these patients.”
Header image caption: Joshua Stein, MD, MS