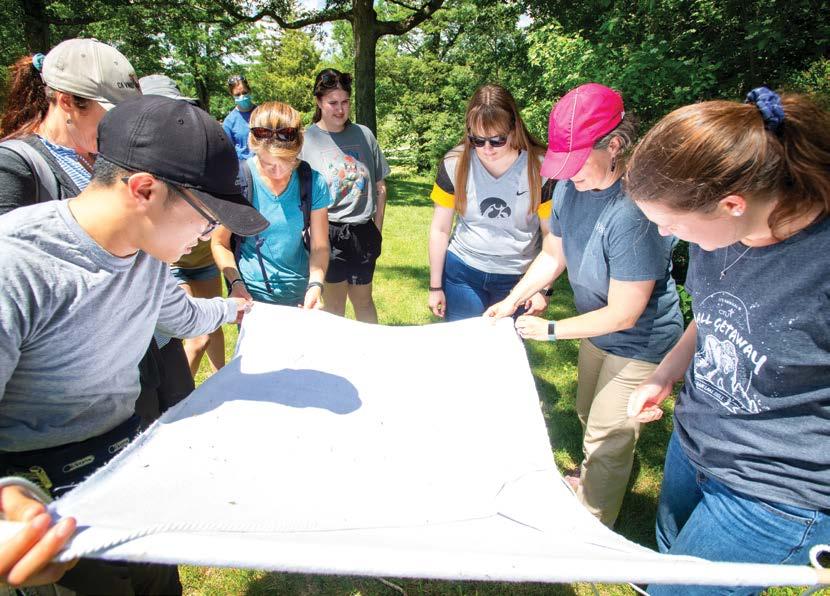
3 minute read
Predicting Tick Trends
WARM WEATHER means more outdoor activities—and a disease-carrying pest: the Ixodes tick. Commonly known as the black-legged tick or deer tick, Ixodes species begin searching for blood meals during spring and early summer, and humans are on the menu. A bite by one of these infected arachnids can transmit a variety of pathogens including Lyme disease, the most widely reported vector-borne disease in the United States.
To help protect against these nearly invisible ectoparasites, a team of University of Iowa epidemiologists has developed new disease surveillance strategies that couple historical data with information drawn from internet search terms to predict current trends in Lyme disease. The researchers say their innovative models are tools that local health agencies can use to tap free, up-to-date information—in this case, search history data from Google—to better understand Lyme disease patterns and make more timely, targeted interventions to battle the steadily rising incidence of the disease.
The team, led by Professor of Epidemiology Christine Petersen, set out to develop these “nowcasting” models—so-called because they’re designed to “predict the present”—to address reporting lags of up to two years that hamper current public health surveillance and mitigation efforts.
The research team built statistical models capable of predicting Lyme disease incidence in five regions of the United States: Northeast, Midwest, Southeast, Southwest, and West. Two different models were developed for each geographic region—one model using only search terms related to the name, symptoms, and ticks that carry Lyme disease, and another model using those same disease-specific terms plus a broader list of terms, identified by Google Correlate, that an average person would be searching for during internet browsing. The researchers reviewed internet search data between 2004 and 2019, collected at monthly intervals to match against Lyme disease incidence data from the CDC.
When fit to the CDC data, the researchers found that both models provided accurate estimates of Lyme disease incidence in four of the five geographic regions; however, the model that included colloquial search terms resulted in more accurate predictions.
“Using web-based data from Google or social media sites to predict health outcomes is gaining popularity and credibility from a variety of public health audiences,” observes first author Eric Kontowicz (20PhD). The combination of “strong surveillance efforts and data coupled with computational modeling techniques can generate models that produce accurate predictions of disease trends,” he says.
The study was published in the open-access journal PLOS ONE and also included UI faculty researchers Kelly Baker, Grant Brown, Margaret Carrel, and Jim Torner.