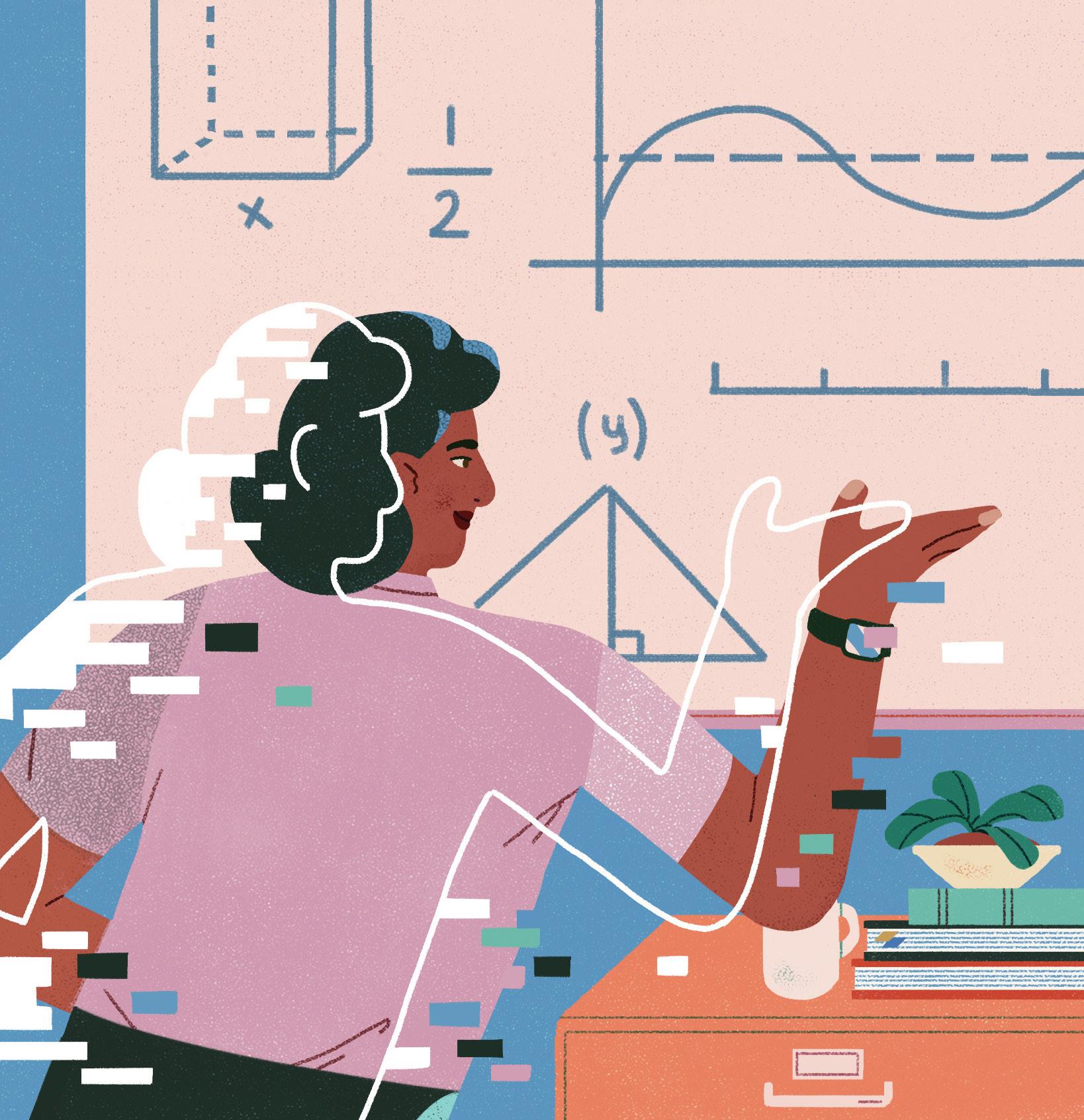
2 minute read
How AI Support Could Add Up to Better Math Teaching
PROJECT CREATING AUTOMATED SYSTEM TO ANALYZE TEACHING QUALITY WINS FUNDING IN GLOBAL COMPETITION
BY CHRIS CARROLL
Think of a teacher who inspired you, made you think in a new way or even got you revved up to do algebra homework. There was likely more to their influence than just being a great person—like the way they listened carefully to what students said in class and rolled that into their lesson to boost engagement and understanding.
The technical term for that skill mastered by the best instructors is “uptake,” and it’s not easy to teach to teachers, or to assess how well they employ it. The traditional way to evaluate it and other teaching practices combines a yearly classroom observation and rating by experts in a process that’s infrequent, staffing-intensive and highly subjective. But a project led by Jing Liu, assistant professor of education policy, could one day provide frequent, automated measures of teacher performance.
Known as M-Powering Teaching, or MPT, the system last month was selected as one of 30 educational technology projects to win $250,000 in funding from the Learning Agency, a nonprofit education-focused agency supported by Schmidt Futures, the Bill and Melinda Gates Foundation, and other high-profile philanthropies.
MPT uses natural language processing, a branch of machine learning and artificial intelligence, to analyze how math teachers instruct and interact with students, with the goal of providing a stream of near-instant feedback. It wouldn’t replace human-based ratings or feedback to teachers, and the system has plenty of human checks and balances, said Liu, whose research focuses on how education policy intersects with data science.
But when fully operational, it has the potential to be faster and more precise than humans can be when measuring performance.
“What we do is combine theory and knowledge from teaching, learning and linguistics, and use an automated process that generates useful insights from a transcription of the class,” Liu said. “We can measure, for example, how many times in the class a teacher is uptaking student ideas, or when the teacher asks questions, are they close-ended yes-no questions, or open-ended ones that can generate useful discussion?”
The technology was developed based on thousands of hours of archived recordings of math instruction housed at Harvard University—where Liu’s MPT partner Professor Heather Hill researches teaching quality and training programs—and at the University of Michigan. The third core team member, Dora Demszky, is an incoming assistant professor in educational data science at Stanford University who studies natural language processing.
It will soon be tested at a school district in Utah, and MPT should eventually be folded into a teaching improvement app fielded by another partner, the startup company TeachFX, which currently offers insights based on metrics on talk patterns in classrooms, like how long teachers speak before a student can get a word in edgewise.
Math instruction is just the beginning, Liu said. “Improving math skills is a national priority, and basis for STEM education, so it’s a natural thing to focus on,” he said. “But we are starting to expand it to apply to language arts, and it can be useful for many subjects and disciplines in the future.”