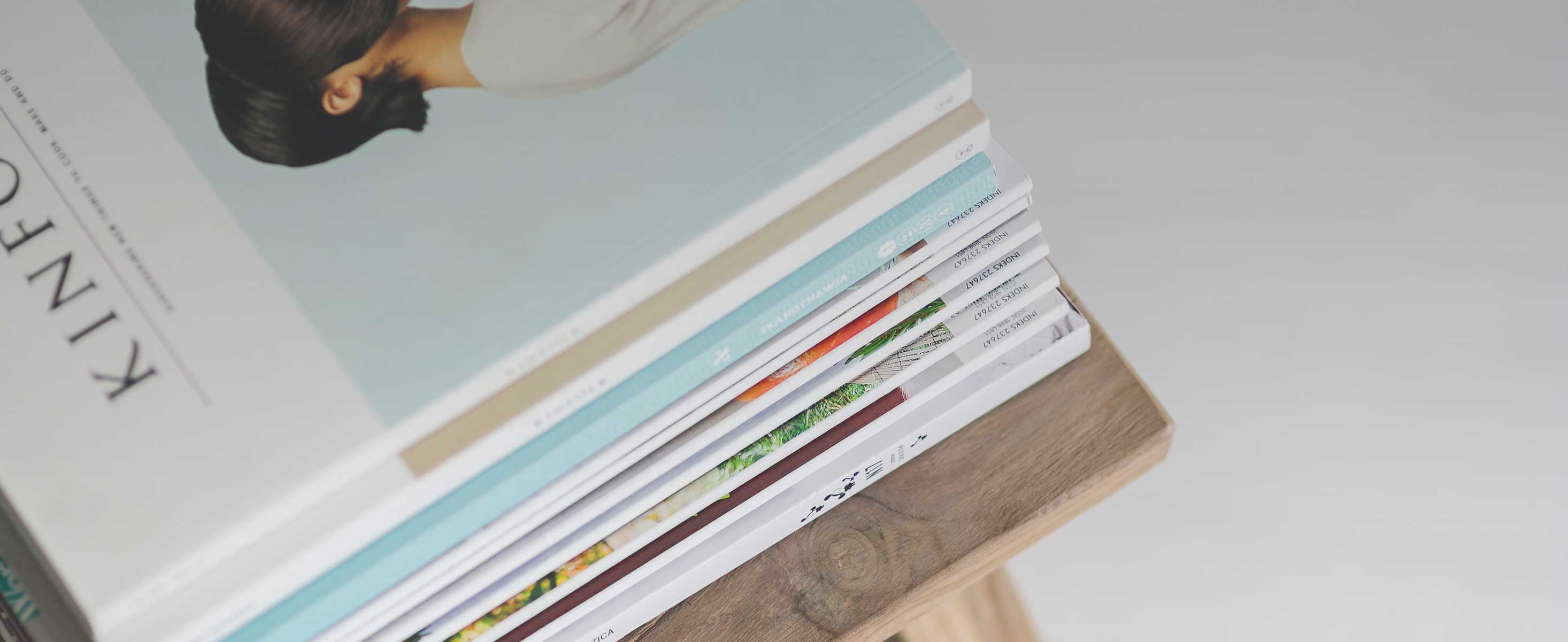
2 minute read
3.3 Recommender System - Germany
The Central Office for Information Technology in the Security Sector (ZITiS), within the German Federal Ministry of the Interior, has been working on a recommender system, similar to those used by platforms such as Amazon, Netflix and Spotify. Recommender systems work on the assumption that in large, diverse datasets, similar users select similar items. Based on this knowledge, and after an initial period of learning, items of potential interest are automatically recommended to the user.
For large law enforcement agency databases containing vast quantities of data from criminal investigations, finding relevant information is often like searching for a proverbial ‘needle in the haystack’. ZITiS was specifically motivated to develop the recommender system to meet the exigencies of modern financial crime investigations that typically require the scrutinizing of vast quantities of financial data. Large cases of this kind could take years of work to search through and to find and cross-check relevant information for the case. With the increase of data and ever-growing databases, the frequency with which such large cases arise is likely to increase. The system itself is an active learning content-based tool, which means that the algorithm tries to interactively query valuable information for officers based on prior searches or similar user preferences.
While a promising application, there are some challenges concerning creating effective recommender systems for law enforcement. Primarily, it is essential that a recommender system with decision-making capacities is not biased. Bias will affect the accuracy and truthfulness of the information and could compromize an investigation. This will be a significant challenge for law enforcement to carefully navigate as recommender systems are in fact designed to encourage users in a specific direction, so as to avoid searching in multiple directions. For instance, recommender systems in the private sector, such as those used by Amazon, Netflix and Spotify, are designed to encourage users to ‘buy more products’, ‘watch more films’ or ‘listen to more music’. Law enforcement will need to ensure that its recommender systems truly seek to provide valuable information for law enforcement and not merely encourage ‘more arrests’. Another challenge is that ordinary recommender systems use limited data types, such as either audio or video exclusively, whereas with law enforcement these systems would have to work with a very broad range of types of data, such as emails, images, audio, video. Other potential challenges include ensuring accurate meta-data creation, the variety of content, the addition and contextualization of new content, explanation of recommendations, and the ‘cold start’ – starting from nowhere and having to link cases, individuals and evidence that may be relevant.