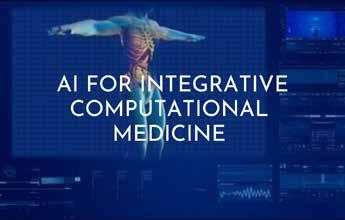
6 minute read
AI for integrative computational medicine
Research activity of Axis 2 during the period 2019-2021
Research topics
This axis aims to generate new fundamental knowledge in medicine to create computational models of the human body and to analyze digital health data in order to improve medical practice in disease prevention, diagnosis, therapy monitoring, and patient rehabilitation. To achieve this purpose, this axis uses data-driven methodological approaches based on machine learning, but also on the integration of mathematical models of human anatomy and physiology. A typical example is the generation of a digital patient which integrates patient data of various natures and at various temporal and spatial scales and analyzes these data to solve a wide range of clinical tasks. A major issue for this axis is how to manage personal and health data while enforcing data privacy and security requirements. This can be handled both by the development of new technologies (such as federated learning) and by the action of dedicated organizations (hospitals, health data hubs, etc.). The main clinical applications of this axis are in neurology, neuroimaging, oncology (prostate, lung, thyroid, abdomen, etc.), cardiology, vascular diseases, nuclear medicine, digital pathology, and functional rehabilitation.
3IA Chairs
A total of 11 researchers have contributed to Axis 2: • 7 Chairs since Oct. 2019 (N. Ayache, H. Delingette, M. Sermesant, R. Deriche, J-P. Merlet, O. Humbert, and
F. Bremond), • 3 Chairs since Sept. 1, 2021 (V. Zarzoso, J. Raffort, M. Zuluaga), • 1 International chair (S. Ourselin) since 2019. Two Chairs are Medical Doctors (O. Humbert, J. Raffort).
International chair - since 2019
Sébastien Ourselin - King’s College London AI for Healthcare & Image-guided Interventions. He is Head of School of Biomedical Engineering & Imaging Sciences, Professor at King’s College London and Director of the EPSRC Image-Guided Therapies UK Network+. He published more than 400 articles (h-index: 64). He is associate editor for IEEE Tr. on Med Imaging,J of Med Imaging, Nature Scient. Reports, and Medical Image Analysis.
Chairs 2019
Nicholas Ayache - Inria
AI for e-patients and e-medicine
We are designing and exploiting modern AI methods: (i) to personalize the parameters of advanced models of the e-patient, (ii) to drive e-medicine algorithms on personalized e-patients (i.e. digital twins) for automated diagnosis, prognosis and therapy, in an efficient, robust, safe and explainable manner. We also seek to augment databases with biophysical simulation.
François Brémond - Inria
Video analytics for human behavior understanding
Video analytics enables us to measure objectively the behavior of humans by recognizing their everyday activities, their emotion, eating habits and lifestyle. Human behavior can be modeled by learning from a large quantity of data from a variety of sensors to improve and optimize, for instance, the quality of life of people suffering from behavior disorders.
Hervé Delingette - Inria
Joint biological and imaging biomarkers in oncology
We exploit joint information from imaging and biological data to improve the diagnosis and treatment planning, focusing on lung cancer. This approach relies on methods involving unsupervised deep learning, uncertainty quantification, sparse Bayesian feature selection and the handling of confounding factors.
Olivier Humbert - Université Côte d'Azur
Comprehensive omics profiling for precision medicine in Oncology
I am combining various patient extracted “omics” data, including multimodal imaging features, for integrative and data-driven computational medicine. I focus on challenging fields in oncology such as (i) radiogenomics and outcome-focused research in metastatic breast cancer and (ii) the accurate prediction of response to immunotherapy.
Jean-Pierre Merlet - Inria
Non-invasive assessment of disabilities
We use mathematical/AI methods for (i) designing non-intrusive and affordable monitoring/assistance devices that are adaptable to the user’s/doctor’s needs, (ii) deducing medically pertinent health-indicators from the data, taking into account measurement errors, and (iii) detecting rare events that may be the sign of emerging pathology.
Maxime Sermesant- Inria
AI and biophysical models for computational cardiology
The application of AI in healthcare is challenging due to its lack of robustness and explainability. This project aims to introduce physiological priors in AI through biophysical models. This can be done by reformulating problems through such models, by learning spatiotemporal dynamics from biophysics or by augmenting features and data with such simulations.
Rachid Deriche - Inria
Computational brain connectomics
This project will reconstruct and analyze the brain’s neural connections network, the connectome, via a computational brain connectomics framework based on ground-breaking AI algorithms and ML tools to gain insight into brain architecture, functioning and neurodegenerative diseases.
Chairs 2021
Juliette Raffort-Lareyre - CHU Nice / Université Côte d'Azur
Applications of AI for patients with vascular diseases
Our team develops AI-derived applications for patients with vascular diseases including aortic aneurysm. We aim to create aid-decision support systems to enhance evidence-based decision and precision medicine through a translational approach including: identification of biomarkers, automatization of vascular imaging analysis, development of predictive scores using machine learning.
Vicente Zarzoso - Université Côte d'Azur
IAblation: Artificial Intelligence for patient-centered atrial fibrillation ablation
Atrial fibrillation is the most common sustained arrhythmia in clinical practice and remains the last great frontier of cardiac electrophysiology. The project aims to put forward new AI techniques to help cardiologists perform more effective patient-tailored catheter ablation procedures to treat this challenging cardiac condition.
Maria A.Zuluaga - Eurecom
Learning-based Models in Medical Imaging: Closing the Gap towards Clinical Translation
We aim to close the gap hindering the translation of AI systems into clinical practice. To this end, we develop novel AI tools for healthcare that 1) learn incrementally as data become available, 2) generalize across multi-modal data and 3) perform quality control of a model and can generate alerts when a decreased in performance is detected.
Actions and highlights
• Federated learning: The Fed-BioMed project led by M. Lorenzi (Axis 1) is a nationwide initiative that is currently being tested and that concerns the analysis of PET images (Chair: O. Humbert) in real-world conditions using the infrastructure of a local hospital. The extension of this framework to large-scale international clinical projects is actively studied by the international chairholder, S. Ourselin. • Medical imaging analysis and multiomics: Medical images are processed by deep learning algorithms to detect abdominal organs in ultrasound images, to create biomarkers for immunotherapy patient selection from whole slide imaging, and to detect cancer lesions in MR prostate imaging (Chairs: N. Ayache and H. Delingette). The analysis of medical images is extended to include biological (omics) data to create more powerful biomarkers and to develop precision medicine. This meta-analysis is performed in the context of lung cancer by combining radiomics and metabolomics (Chair: O. Humbert) but also by combining radiomics and transcriptomics to improve the treatment of patients with aortic aneurysm (Chair: J. Raffort). For these tasks, a major concern is to create high-quality datasets and to monitor the performance of algorithms across heterogeneous and unseen datasets (Chair: M. Zuluaga). • Cardiac imaging and signals: AI algorithms are developed to create patient-specific electromechanical models of the heart but also to create physics-informed networks capable of simulating cardiac electrophysiology (Chair: M. Sermesant). Furthermore, data-driven approaches are investigated to perform patient selection and therapy guidance in the context of catheter ablation for the treatment of atrial fibrillation (Chair: V. Zarzoso). • Brain connectomics: The relation between brain connectivity and neurological diseases is investigated through the generation of novel biomarkers partially based on diffusion MRI (Chair: R. Deriche). • Patient monitoring: The video-based monitoring of the everyday activities of elderly subjects in real-world settings was developed to produce new reference datasets for the field and to include the detection of emotions (Chair: F. Bremond). Furthermore, ML was used to improve the control of a cable-driven parallel robot that can assist frail patients to stand and walk (Chair: J-P. Merlet).