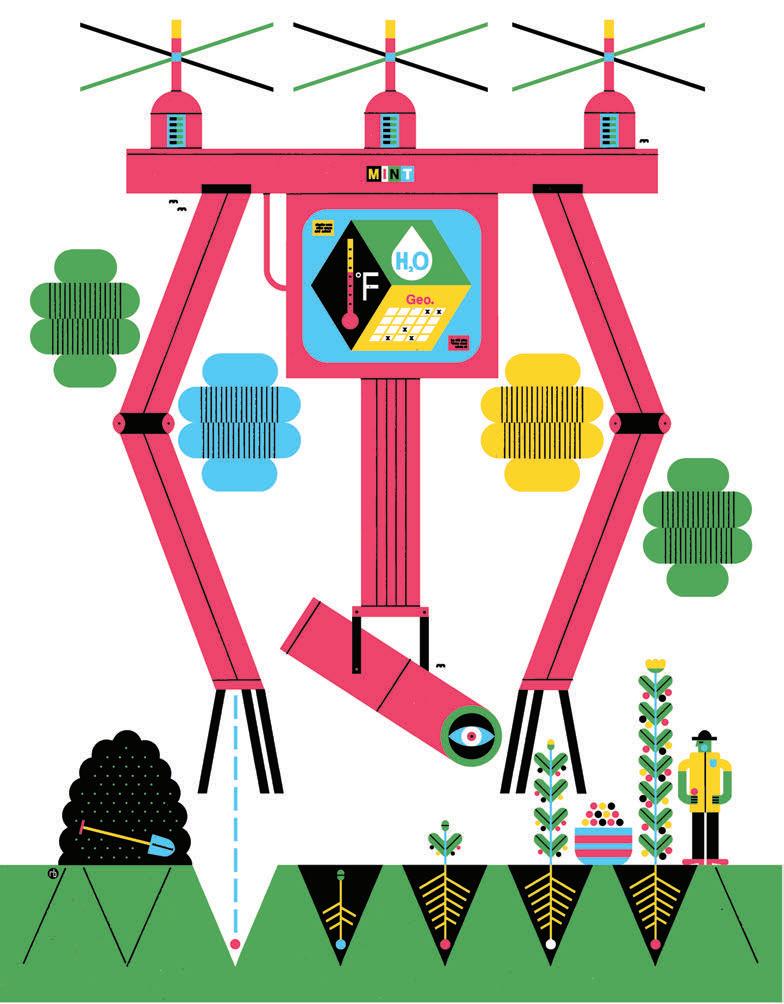
4 minute read
MINT “ could help in boosting crop production because it provides the right information at the right time.
on livestock and deepening food insecurity and malnutrition,” according to the United Nations Office for the Coordination of Humanitarian Affairs.
Ethiopia also suffers from occasional flooding that can ruin crops, giving farmers more to worry about.
Advertisement
Still, many believe the country’s farming sector might have a bright future, the challenges notwithstanding.
Ethiopia’s different climate and soil types make it possible to grow diverse crops, says Gebissa Yigezu of Haramaya University in Ethiopia. Additional government incentives, improved infrastructure and increased credit and donations from international organizations might spur higher crop yields and exports, he explains in the academic paper “The Challenges and Prospects of Ethiopian Agriculture.”
MINT’s integrated modeling aims to make those efforts much more effective.
MINT in Action
With a grant from the Defense Advanced Research Projects Agency, Gil began working on MINT in 2019. She finished a prototype one year later. Gil says MINT represents an advance in artificial intelligence. “Goal-oriented problem solving is not new in AI. But goal-oriented problem solving where you are integrating models from different scientific disciplines is a completely new approach.”
In creating MINT, which spans AI research in knowledge representation, reasoning and machine learning, Gil first converted diverse data for weather, soil, topography and many others into data that computer modeling could understand and use.
Then she provided the advanced AI system with data and models from different disciplines and goals inspired by questions from scientists such as: What crops would give a higher yield next year? When should the planting of maize start so it can be harvested before flooding starts? Should the government subsidize fertilizers?
After receiving the knowledge from scientists about how their models work, Gil says, MINT aggregates the conference. Gil’s team has been generating thousands of model runs under different initial conditions to explore different assumptions and scenarios.
When operational, MINT “could help in boosting crop production because it provides the right information at the right time” for different types of farming, says Yihenew Zewdie, an Ethiopia-based expert in food systems and natural resource governance.
Solving Other Intractable Problems
With different data and simulation models, MINT could play an important role in addressing other significant problems ranging from fires to floods and other natural disasters.
The MINT modeling framework is being used in a research project headed by the University of California, San Diego’s San Diego Supercomputer Center to bring the power of AI to help firefighters strategize how best to plan controlled burns and manage unexpected blazes.
Gil also works with the Technical University of Munich to model the impact on streamflow and farming from changes in the timing and size of snowmelt in central Kyrgyzstan.
In a collaboration with the Texas Advanced Computing Center at the University of Texas at Austin, Gil is working with models of groundwater that help predict water availability in Texas aquifers under different conditions. Gil works with Suzanne Pierce and others in the Texas Disaster Information System (TDIS), which serves as an information clearinghouse to support improved natural disaster planning, response, recovery and mitigation efforts in Texas. Pierce leverages MINT and its AI technology for floods to predict and identify the most at-risk areas.
“MINT is a paradigm-shifting approach that can help you accelerate, improve and expand what you can analyze,” Pierce says. “I think it can make a world of difference across the many wicked problems that society is currently facing.”
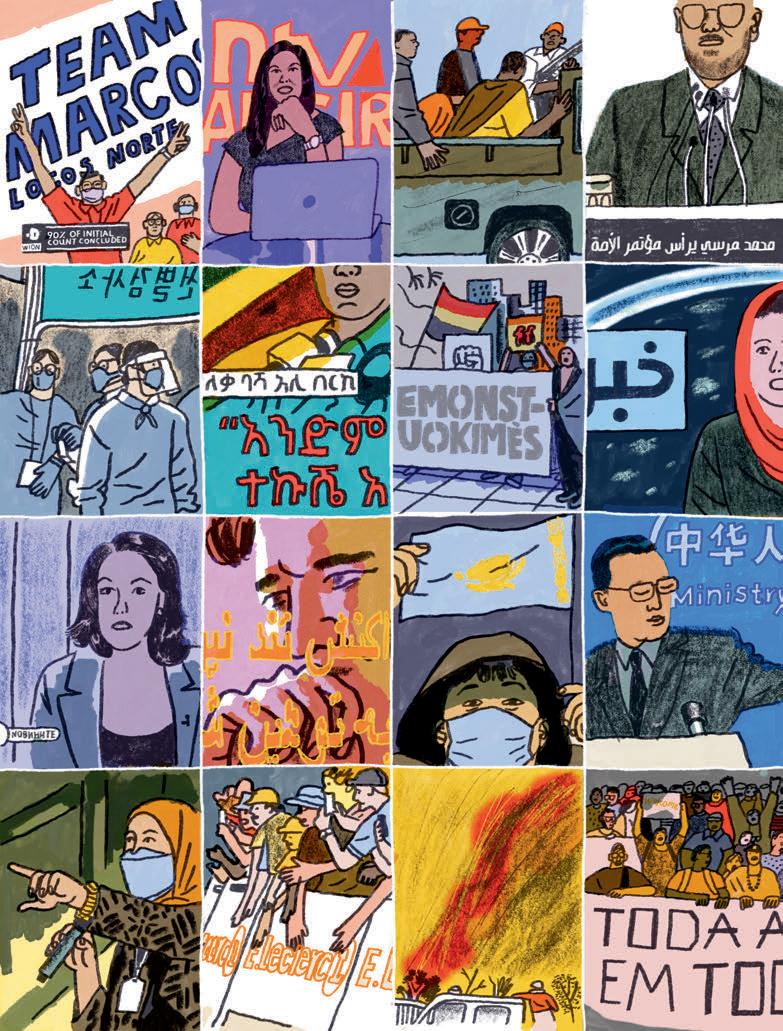
Say you’re curious about political unrest in Thailand. A Google search brings up links to thousands of news articles. You begin the time-consuming task of poring over the top stories, tedious screen crawl by screen crawl.
Now imagine your computer could do all that reading for you and provide short summaries of each article in seconds. What’s more, imagine that you also have access to articles written in Thai or other languages, not just English.
Researchers at USC’s Information Sciences Institute (ISI) have been working on two separate artificial intelligence tools that do just that — an effort that not only has big implications for the U.S. intelligence community and journalists, but for anyone who wants to know what’s going on in the world.
“Fundamentally, what our algorithms do is make information from foreign language sources available to Englishspeaking users, without requiring any foreign language expertise or any foreign language examples to teach the systems,” explains Liz Boschee. As director of ISI’s Boston office and a research team leader, she specializes in natural language processing, the field of AI, computer science and linguistics that involves which involves training computers to process and analyze large amounts of natural language data.
Two Government-Funded Projects
Boschee is a key researcher in two AI projects funded by the Intelligence Advanced Research Projects Activity, part of the federal government’s Office of the Director of National Intelligence.
The first, launched in October 2017 and concluded in September 2021, is MATERIAL, which stands for Machine Translation for English Retrieval of Information in Any Language. It allows a user to search in English for speech and text content in Tagalog, Swahili, Somali, Lithuanian, Bulgarian, Pashto, Farsi, Kazakh and Georgian. Relevant results are then summarized for the user in English.
These languages are particularly challenging and are dubbed “lower-resource” because they don’t have large data sets available for building machine translation or language models, unlike “high-resource” languages such as Spanish and French.
introduced to the public. Boschee says both have the potential to provide English-language speakers access to news in languages they don’t know.
Through deep learning, computers can find words in a foreign language that are similar in meaning to their counterparts in English. Think of the word “protests.” By reading millions (or sometimes billions) of words scraped from the web, AI models can learn that “protests” and “demonstrations” are closely related.
By doing the same thing in many languages simultaneously, those models can learn that “protest” and “maandamano” (a Swahili word for “protest”) are also closely
Using AI tools developed at USC, users can search foreign language sources and read them in English. B y Greg Hardesty