Statistical issues in survival analysis (Part XVVVVVV)
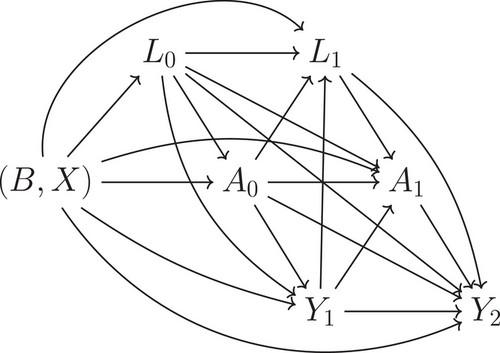
December 18, 2024
Marginal structural models (MSMs) are often used in settings for longitudinal studies with timevarying treatment or covariates observed for each individual at several time points when trying to estimate the causal effects in a way that accounts for time-dependent confounding. Meanwhile, simulations studies are often used in order to evaluate how well a new or current statistical methods performs under a set of pre-defined scenarios. However, this has not been a simple construct for an MSM. Some methods have been proposed for simulating from Cox MSMs but have had various restrictions.
Also, they defined a risk score function based on a hazard assumption at time zero or at any other time point. They also allowed a bivariate Gaussian copula to describe the association between this risk score and a latent continuous variable which determines the outcome variable. They next discussed their extended algorithm for estimating the cumulative distribution function of the risk score and also for simulating data. Also, extending their algorithm to simulate data for a continuous time MSM is straightforward. For their illustrative simulation study, their aim was to compare the simple sandwich variance estimator and nonparametric bootstrap as two methods for calculating confidence intervals (CIs) as well as testing for treatment effect modification when fitting an MSM using IPTW. They found improved performance of bootstrap CIs relative to sandwich Cis which they said agreed with previous research of which they cited several examples. The sandwich estimator also tended to underperform in smaller sample sizes by underestimating the variance.
In their discussion, while they assumed that visit times are the same for all individuals and equally spaced, they said they could also generalize to unequally spaced visits by rescaling the time axis between subsequent visits. They also assumed the risk score is continuous, but with some simple modifications could be made discrete. Also, they assumed static treatment regimes since dynamic treatment regimes are more complex. In conclusion, their algorithm did use Gaussian copulas, though one could extend to non-Gaussian ones but it might not allow for the same properties.
Written by,
Usha Govindarajulu, PhD
Keywords: survival, MSM, causal effect, simulation, Gaussian copula
References
Seaman SR and Keogh RH (2024) “Simulating Data from Marginal Structural Models for a Survival Time Outcome”. Biometrical Journal. https://doi.org/10.1002/bimj.70010
https://onlinelibrary.wiley.com/cms/asset/f9ea88b0-72ed-4b45-b356-eff5cfdc7e48/bimj70010fig-0001-m.jpg