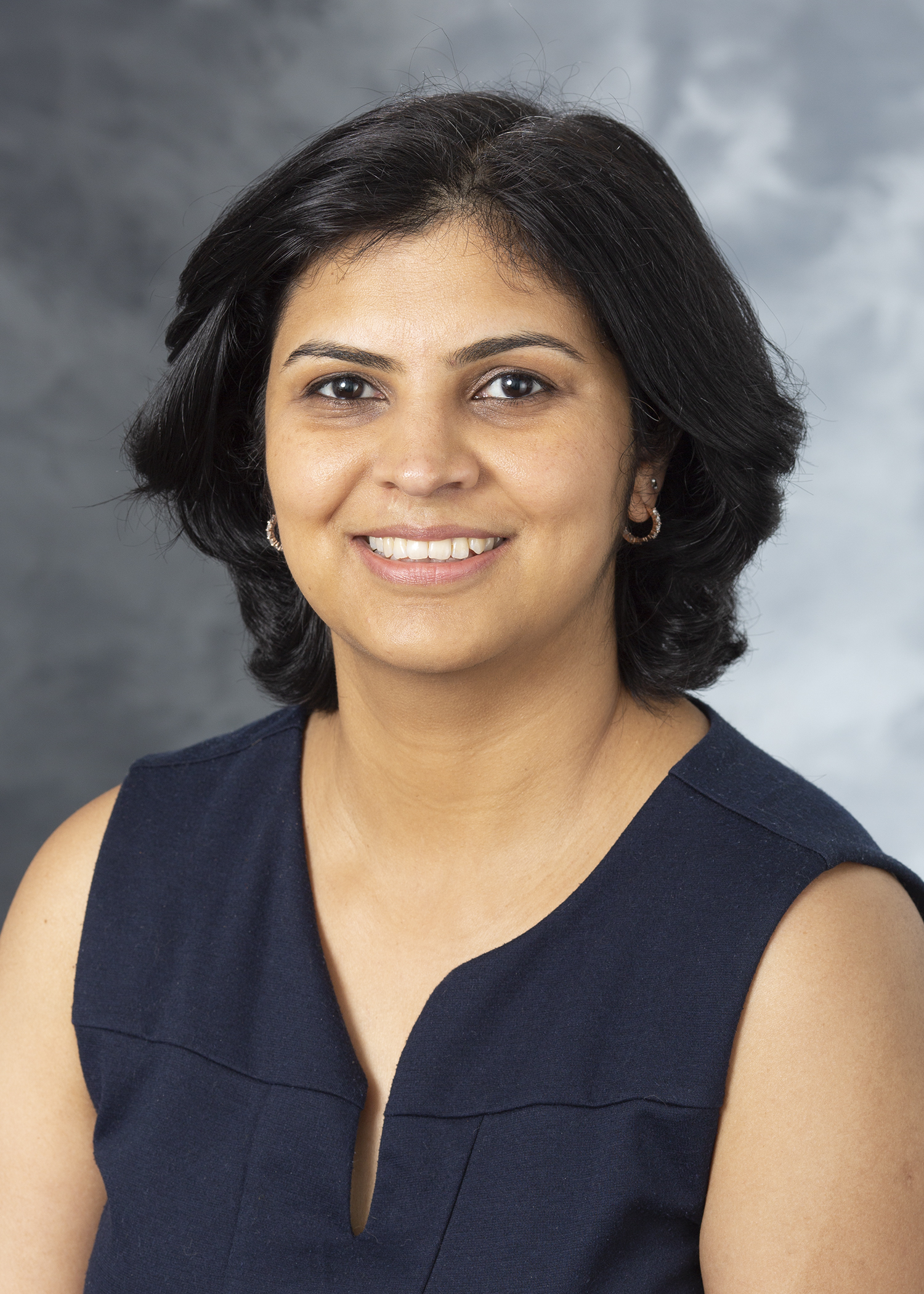
1 minute read
ML4MI Initiative Fosters Interdisciplinary Collaboration at UW–Madison
Machine learning (ML), a branch of artificial intelligence (AI) and computer science, is an emerging field which uses algorithms to analyze and draw inferences from patterns in data. ML and AI approaches are the basis of sensational innovations – like self-driving cars or ChatGPT – as well as something as familiar as Netflix’s recommendation engine.
The possibilities of ML applications in medicine continue to grow, including predicting a patient’s prognosis or monitoring symptoms to allow more proactive management and care. Another exciting potential use of ML is as a tool to support radiologists and pathologists in interpreting medical images. This application might accelerate productivity and improve diagnostic accuracy.
While interest in ML for medical imaging is explosive, taking new methods and technologies from “code to clinic” remains a major challenge. Pallavi Tiwari, PhD, who leads UW–Madison’s Machine Learning for Medical Imaging (ML4MI) Initiative believes interdisciplinary collaboration will be the key to achieving this goal. The ML4MI Initiative – sponsored by the Departments of Radiology, Medical Physics, and Engineering – fosters exactly that.
“We are hoping to bring together the researchers who are developing these sophisticated algorithms and methods and the clinicians/radiologists who make clinical decisions,” she explains.
With a world-class engineering program and an outstanding team of machine learning researchers in the School of Medicine and Public Health, UW-Madison is uniquely suited to this collaborative endeavor. By bridging the gap between these groups, the ML4MI Initiative will catalyze the translation of these emerging technologies into clinical practice.
To kick-start the initiative, an ML4MI retreat was held on March 15 at Memorial Union Terrace. Over 100 members of the UW–Madison community attended the event, where leading researchers at UW presented their work.
While these talks highlighted the immense potential of machine learning to solve challenging problems in image reconstruction, image processing, and computer-aided diagnosis, they also emphasized the need for further breakthroughs before these methods can be integrated into clinical practice.
“This retreat is just the first step,” said Dr. Tiwari. “In the future, we hope to have events that are open to an even wider audience.”