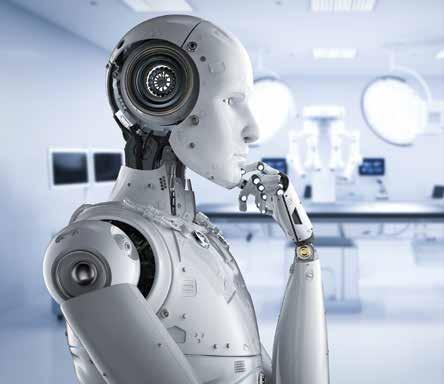
9 minute read
Artificial Intelligence May Help Radiologists Detect Fractures in Daily Practice
In a recent paper published in Radiology, Guermazi et. al. used artificial intelligence (AI) algorithm for automatically detecting X-rays that are positive for fractures. They showed AI assistance helped reduce missed fractures by 29 per cent and increased readers’ sensitivity by 16 per cent, and by 30 per cent for exams with more than one fracture, while improving specificity by 5 per cent. They concluded that AI can be a powerful tool to help radiologists and other physicians to improve diagnostic performance and increase efficiency, while potentially improving patient experience at the time of hospital or clinic visit.
Ali Guermazi, Professor of Radiology and Medicine, Boston University Nor-Eddine Regnard, Radiologist
Advertisement
Missed fractures on standard radiographs are one of the most common causes of medical errors in the emergency department and can lead to potentially serious complications, delays in diagnostic and therapeutic management, and the risk of legal claims by patients.
In a study recently published in Radiology, Prof. Ali Guermazi et. al. Showed an improvement in clinicians' diagnostic performance in detecting fractures in standard radiography using artificial intelligence software, BoneView by Gleamer, compared to reading radiographs without assistance.
This was a retrospective study including 480 radiographic examinations of adults over 21 years of age, with indications of trauma and fracture prevalence of 50 per cent. Radiographs included were of the limbs, pelvis, dorsal spine, lumbar spine and rib cages.
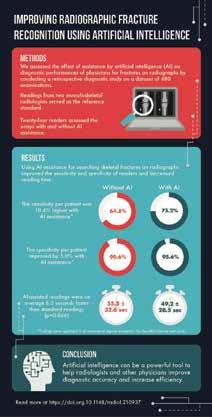
Figure 1: This infographic summarizes the study design and the main results of the primary analysis
The radiographs were obtained from various US hospitals and clinics.
There were 350 fractures in 240 patients and 240 examinations without fractures.
The radiographs were analyzed twice (with and without assistance of Gleamer's Boneview automatic fracture detection software). Readers had 1-month washout period between the two analyses.
There were 24 US board certified readers from six different specialties (four radiologists, four orthopaedic surgeons, four rheumatologists, four emergency physicians, four family medicine physicians, and four emergency physician assistants) with different levels of seniority for radiologists and orthopaedic surgeons.
The gold standard was established based on the independent reading of radiographs by two musculoskeletal radiologists. Discrepancies were adjudicated by an expert musculoskeletal radiologist, Prof. Guermazi. It was also determined whether the fractures were obvious or not.
The standalone performance of the software on this dataset was evaluated globally, as well as the improvements in the diagnostic performance of the readers in terms of sensitivity, specificity and time saving. An analysis was also made by type of reader and by anatomical location of the fractures.
The results of the study showed an absolute gain in sensitivity in the detection of fractures of 10.4 per cent with the assistance of the Boneview software of 75.2 per cent against 64.8 per cent without the assistance of the software (p<0.05).
There was also an absolute gain in specificity of 5 per cent in fracture detection with software assistance, with specificity increasing from 90.6 per cent without assistance to 95.6 per cent with software assistance (p<0.05).
A significant absolute gain in reading time of 6.3 seconds per radiograph (p=0.046) was found, with reading time decreasing from 55.5 seconds without assistance to 49.2 seconds with software assistance. These results from the primary analysis are summarised in Figure 1.
The absolute gains in sensitivity per patient were 7.6 per cent for radiologists, 9.1 per cent for orthopaedic surgeons, 9.9 per cent for emergency physicians, 9.4 per cent for emergency physician assistants, 17.2 per cent for rheumatologists and 9.3 per cent for family physicians.
The absolute gains in specificity were 2.8 per cent for radiologists, 2 per cent for orthopaedic surgeons, 3.4 per cent for emergency physicians, 2.5 per cent for emergency physician assistants, 14 per cent for rheumatologists and 5.2 per cent for family physicians.
Sensitivity gains were better for non-obvious fractures (+12.4 per cent) than for obvious fractures (+7.5 per cent) (p= 0.05).
Sensitivity gains were large (more than 10 per cent) and significant for most locations (foot-ankle, knee-leg, hip-pelvis, elbow-arm, ribs). Sensitivity
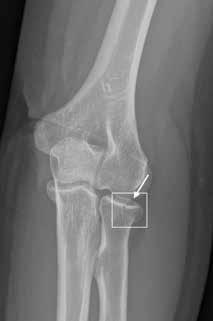
Figure 2: Anteroposterior elbow radiograph shows a radial head fracture which was missed by 4 readers without software assistance, and by no readers with software assistance Figure 3: Oblique radiograph of the ribs shows 2 fractures of the 5th and 6th left ribs which were detected by the software. At least one of the fractures was missed by 9 readers without software assistance.
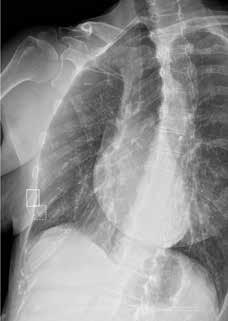
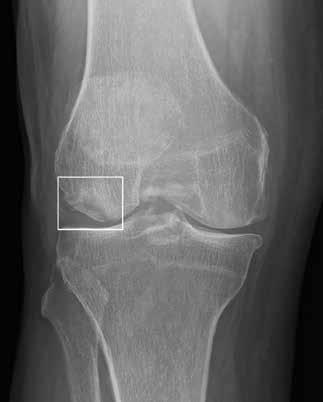
Figure 4: Anteroposterior knee radiograph shows subchondral fracture of the lateral femoral condyle which was missed by 3 readers without software assistance, but picked by all readers with software assistance. Figure 5: Anteroposterior shoulder radiograph in internal rotation shows a calcification around the glenoid which was mistaken for a fracture by 6 readers without software assistance. The software did not label this as a fracture. Only 2 readers still considered it to be a fracture with software assistance.
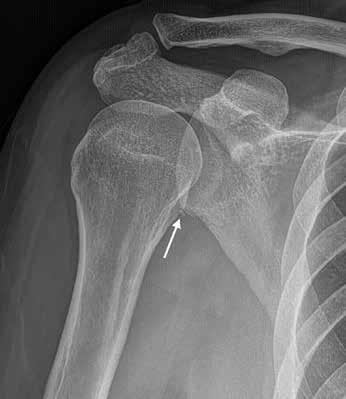
gains were not significant for the dorsolumbar spine and shoulder-clavicle.
Absolute gains in sensitivity per fracture were similar for single (+10.1 per cent) and multiple (+11.5 per cent) fractures (p=0.54).
The standalone performance of the software was good with an Area Under the ROC Curve of 0.97.
The sensitivities per patient averaged 88 per cent. The sensitivities and specificities were 93 per cent and 93 per cent for feet-ankle, 90 per cent and 93 per cent for knees-legs, 90 per cent and 87 per cent for hips-pelvis, 93 per cent and 100 per cent for handswrists, 100 per cent and 97 per cent for elbows-arms, 84 per cent and 83 per cent for shoulder-clavicle, 77 per cent and 69 per cent for rib cage, 77 per cent and 80 per cent for dorsolumbar spine respectively.
Figures 2, 3 and 4 illustrate fractures missed by some readers, which were recovered by using the software. Figure 5 shows an example of a false positive by some readers but not with the software.
The absolute gains in sensitivity per patient were 7.6 per cent for radiologists, 9.1 per cent for orthopaedic surgeons, 9.9 per cent for emergency physicians, 9.4 per cent for emergency physicians, and 8.5 per cent for physicians in the general population.
In conclusion, the results of this study recently published in Radiology showed an improvement in the diagnostic performance of readers from different medical specialties using Gleamer's BoneView automated fracture detection software in the detection of limb, pelvis, rib cage and dorsolumbar spine fractures in sensitivity and specificity as well as a time saving in the reading of standard radiographs.
These results can have major impact on the management of patients by emergency physicians, radiologists, orthopedists, rheumatologists and family doctors. Indeed, the gain in diagnostic performance can have an impact on the immediate management of patients in the emergency room or in the general practice, by increasing the relevance of the diagnostic and therapeutic management. In particular, type of software can probably help to select the necessary complementary examinations (CT and/or MRI) for the right patients to confirm fracture diagnoses and assess their severity at an early stage and thus avoid complications such as fracture displacement, non-union, pseudoarthrosis, persistent pain and
AUTHOR BIO
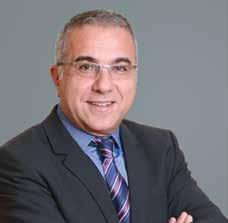
Guermazi is a Professor of Radiology and Medicine and Assistant of Diversity at Boston University School of Medicine. He is the founder and Director of the Quantitative Imaging Center (QIC) within the Department of Radiology at Boston University School of Medicine, Boston, MA, a research group focusing on Musculoskeletal Radiology and especially MRI that provides radiological analysis services for industry, foundation or NIH-sponsored trials. Professor Guermazi was the Deputy Editor of the musculoskeletal imaging section for the RADIOLOGY journal from 20132019. He has authored or co-authored more than 600 peer-reviewed papers and made numerous scientific presentations at national and international osteoarthritis, rheumatology and radiology meetings.
Regnard is French board-certified musculoskeletal Radiologist and co-founder of GLEAMER. He has 12 years of clinical experience. His interest in artificial intelligence led to the conception of several algorithms that help radiologists world-wide in their routinely daily practice. even algodystrophy. This may allow patients to be treated early by immobilisation or surgery and for fractured patients to be referred to specific trauma departments.
This can probably increase patients’ recruitment to consultations and orthopaedic surgery.
The gain in specificity provided by the software may also result in a reduction in the overtreatment of patients who do not have fractures. The time saved in the analysis of radiographic readings could be used to treat more complex cases.
From an organisational point-of-view, having such a tool would allow radiologists to prioritise the reading of standard radiographs by probability of anomalies. This would help them in choosing which radiographs to analyse first. This is relevant in the emergency context where the flow of additional radiographs can be significant and added to the usual workflow.
In addition, the use of such a tool can decrease the rate of discordance between the opinion of emergency physicians and radiologists, thus decreasing the rate of second visits to the emergency room for the patient and increasing patient satisfaction. Finally, missed fractures are one of the most frequent causes of complaints from patients visiting the emergency room. The use of this type of tool should help to reduce this legal risk.
All these aspects could be investigated in further studies of the medical, economic and legal impacts of the software.
The study had several limitations: the prevalence of fractures was significantly higher than in real life. The readers had only the clinical indication of the traumatic context without the precise location of the painful symptoms. This is a usual situation for radiologists but is more unfavourable for clinicians who are used to analysing images after examining the patient. Another limitation is the choice of the gold standard on the basis of specialised musculoskeletal radiologists' advice only on standard radiographs. This type of gold standard is less precise than the result of a CT or MRI scan but remains relevant to the software's objective, which is to show fractures that are visible on standard radiographs and not occult. Finally, the readers were not analysing the images in their usual workflow and were therefore not limited in their analysis time as may be the case in real life.
All in all, this original study by Prof. Ali Guermazi is the first of such magnitude with so many US physician specialists, on so many anatomical locations to scientifically demonstrate the interest of using an artificial intelligence software for automatic fracture detection on standard radiographs.
