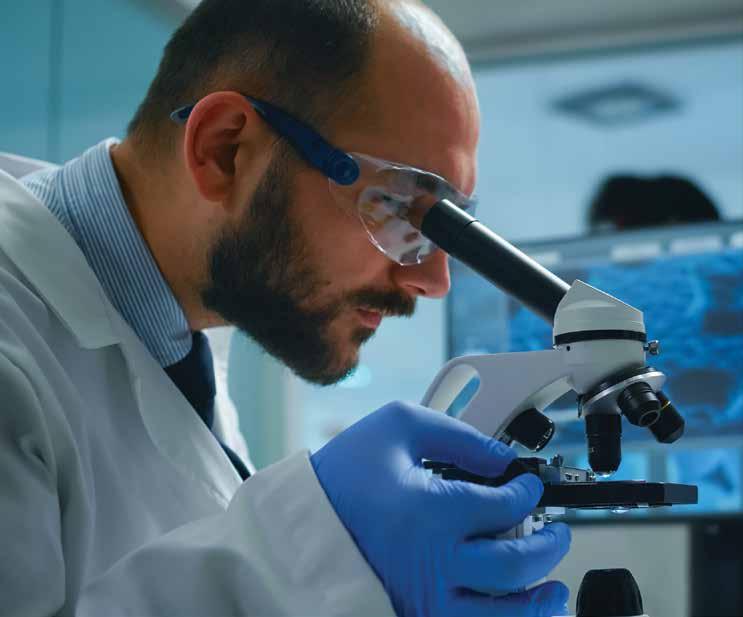
6 minute read
AI to Identify Cancer Cells by their Acidity
The ability to identify different cell types quickly, effectively, and in a minimally invasive fashion is an important goal in medicine. Immunofluorescence remains the most used method to image biological phenomena happening at the cellular and sub-cellular levels, despite drawbacks such as phototoxicity. This is due to the reactive oxygen species generated that affects cell physiology and health. Also, there is a need for cell fixation to allow antibodies to cross the cell membrane. The latter aspect hinders some downstream analyses that require live cells. In addition, to account for the heterogeneity of biological samples, single cell measurements are needed. Therefore, novel techniques that would allow scientists to investigate living cells in a non-invasive way at the single-cell level are required.
Intracellular acidity as a biomarker
Advertisement
Intracellular acidity is linked with numerous biological processes such as migration, division and apoptosis. Deviations from the physiological intracellular pH are associated with cancer growth, invasion, and metastasis. Specifically, cancer cells have a more alkaline intracellular environment. Several methods have been developed to probe cellular acidity, which predominantly focus on fluorescence markers or functionalised nanoparticles. The former method is limited by photobleaching, while the latter one requires complex multi-step protocols.
An interesting approach was introduced in 2017 by Hou and co-workers which described a novel simple and inexpensive single-cell pH-based imaging method that allowed for the identification of cancer cells via common pH indicators and UV-vis micro-spectroscopy.
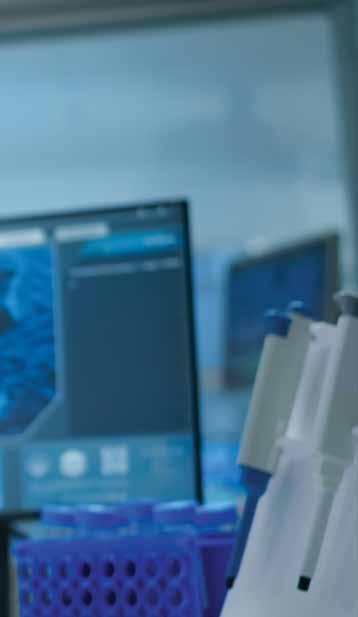
Combining pH-based colorimetric imaging and AI for single-cell classification
Recent advancements in Artificial Intelligence (AI) have enabled novel approaches aimed at extracting information from biomedical images to identify specific phenotypes and aid disease diagnosis. We recently published a work that describes a novel approach where quantitative pH-based colorimetric imaging is combined with AI-based single cell segmentation and classification. This methodology focuses on the use of a pH-sensitive dye, Bromothymol Blue (BTB), as shown in Fig. 1. First, the cell membrane is permeabilised by incubating the cells for 15 minutes with ethanol at 5 per cent concentration. When imaged using a standard optical microscope, upon internalisation of 0.5 mg/mL of BTB, the intracellular environment acquires a characteristic colour based on the unique physiological condition of each cell. Specifically, each type of cell was shown to exhibit a specific colour ‘fingerprint’ consisting of a unique combination of Red, Green, and Blue (RGB) components.
Novel methods capable of quickly identifying single cancer cells while keeping them alive for downstream analyses would enable improved diagnostics and allow clinicians to culture them for personalised drug tests. This article highlights how combining AI and optical microscopy led to an inexpensive and accurate way to classify cancer cells based on their intracellular acidity.
Chwee Teck Lim, Professor and Director, Institute for Health Innovation and Technology, Department of Biomedical Engineering, and Mechanobiology Institute, National University of Singapore
Yuri Belotti, Research Fellow, Institute for Health Innovation and Technology, National University of Singapore
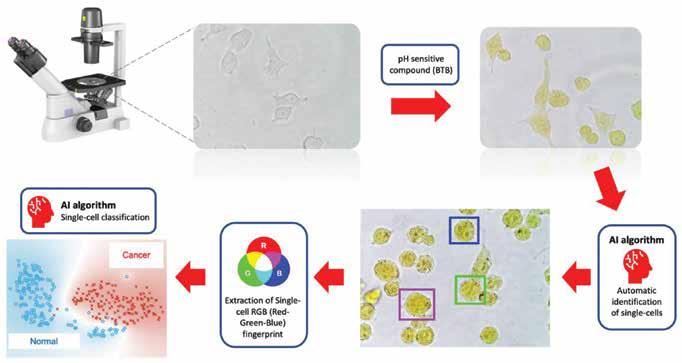
Fig. 1. Workflow of the single-cell AI-aided colorimetric pH imaging. Cells are treated with Bromothymol Blue (BTB), a pH-sensitive dye. An optical microscope fitted with a colour digital camera was used to acquire RGB images. An automated single-cell segmentation algorithm identifies single cells and extracts RGB features. Finally, another AI algorithm classifies the cells based on the RGB features.
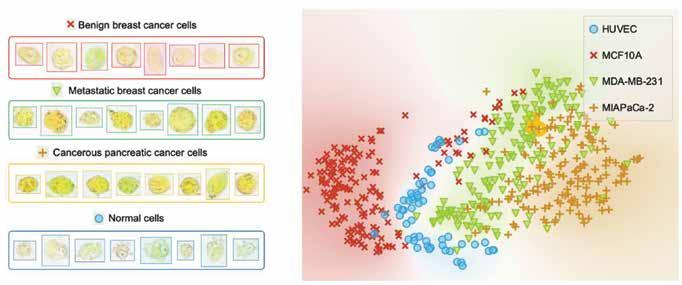
Fig. 2. Single cell computational classification. Cell lines investigated were MCF-10A, MDA-MB-231, and MiaPaCa-2 and HUVEC. On the left, examples of colour images of individual cells are shown, for each cell line. On the right, a t-distributed Stochastic Neighbour Embedding (t-SNE) plot is employed to reduce high-dimensional data in a two-dimensional map, for each cell line.
Normal cells (HUVECs) were found to have the lowest levels of pH versus all the cancer cell lines. For example, higher pH was found in the metastatic breast cancer cell line, MDA-MB-231, as compared with the non-cancerous cell line, MCF-10A. These findings are in accordance with past studies.
Moreover, each cell type exhibits a characteristic spatial distribution of the RGB values. This information was also taken into account and used as a feature to automatically identify the different cell types using AI algorithms. The computational classification was performed by comparing an increasing number of different cell types. Singlecell classification accuracy of ~97 per cent was achieved when comparing MCF-10A with MDA-MB-231.
The classification accuracy reached ~92 per cent when performing the analysis on three cell lines, including the human pancreatic cancer cell line Mia-PaCa-2 to the breast cell lines previously analysed. Finally, adding a fourth cell line to the analysis – the umbilical vein endothelial cells (HUVECs) - a classification accuracy of 92.7 per cent was achieved. The demarcation between the different groups is observed in the t-SNE plot (Fig. 2).
The computational classification was validated using 10-fold crossvalidation for model testing. Further validation was done using both ‘in silico’ co-cultures (where datasets are generated by randomly combining values of the colorimetric features obtained for each cell line) and actual co-cultures.
After going through the analysis pipeline, cells remain viable and can be cultured for several days. Although the use of BTB led to better computational classification accuracy, this method was also successfully proven without the use of BTB, by simply capitalising on the inherent colorimetric differences between cell lines. This is promising and will enable further developments towards a label-free version of this approach. The presented technique could also be
used for sub-cellular analyses. In fact, various cellular components have been characterised by different pH levels as reported previously.
Concluding remarks and future perspectives
This method made it possible to discriminate among normal, non-tumorigenic and cancerous cells purely by focusing on their intracellular acidity. The non-invasive nature of this single cell classification methodology makes it extremely easy and useful for identifying various cell types and can be integrated with current screening and diagnostic methodologies. The protocol takes ~35 minutes, including BTB internalisation, image acquisition, image segmentation and classification. Moving forward, a real-time version where single cells in either adherent or suspended state can be quickly detected and individually manipulated. This will offer a less expensive alternative to the current standard flow cytometry-based cell sorting, while maintaining cell viability, which can then be integrated with downstream analysis. To sum up, our method can be used as a fast, inexpensive and accurate tool to diagnose cancer, monitor cancer progression and/or effectiveness of a treatment, and even alerting the risk of a relapse.
AUTHOR BIO References are available at www.asianhhm.com
Chwee Teck Lim is the NUSS Professor at the Department of Biomedical Engineering, Mechanobiology Institute and Director of the Institute for Health Innovation and Technology (iHealthtech) at the National University of Singapore. His research interests include mechanobiology, microfluidics for disease diagnosis and precision therapy, and wearable technologies for healthcare applications. He has authored more than 400 peer-reviewed journal papers and cofounded six start-ups.
Yuri Belotti is a Research Fellow at the Institute for Health Innovation and Technology (iHealthtech) at the National University of Singapore. His research interests include the development of novel microfluidic devices for liquid biopsy and bioinformatics pipelines for early-stage cancer prognostics.