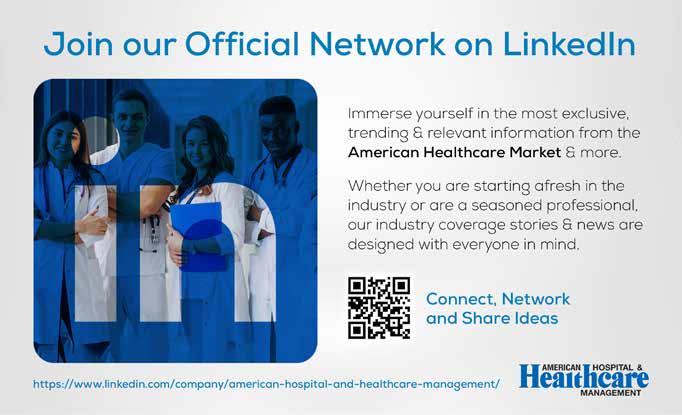
7 minute read
Integration
Deploying Artificial Intelligence (AI) technology to health records is the next step in the evolution of healthcare. More must be done with less, and this technology saves time in analysis of volumes of data, helping clinicians move from assessment to intervention faster, speeding up recovery time. Clinicians are utilizing this technology in multiple ways. Diagnosis is at the forefront. Quicker diagnosis leads to faster response times. The patient is the winner with the use of this technology.
Lorie Morris Senior Vice President Clinical, Prestige Healthcare
Artificial Intelligence (AI) is defined as the intelligence of machines, as opposed to the intelligence of humans or other living species. It usually involves a combination of software and hardware. It also refers to situations wherein machines can simulate human minds in learning and in analysis, and it can work at problem solving. This is also known as machine learning (ML).
Technology has evolved quickly over the past decade, making AI feasible in healthcare. It is becoming an integral part of the healthcare continuum. Utilization of AI technology first became apparent in the diagnostic-imaging. Since that time, its uses have spread across multiple areas, likely impacting all healthcare providers and patients. It is being utilized from assessment to evaluation. This technology has sped up the time from onset of first symptoms to resolution.
AI is transformational technology that includes clinical analytics, machine learning, digitized data acquisition, computer analysis, natural language processing (NLP), Clinical Decision Support System (CDSS), machine vision, data mining, motion analysis, and machine perception. This perspective will look at clinical analytics, machine learning, digitized data, computer analysis, NLP, data mining and machine perception.
Case Study
Let’s examine how this technology is impacting one patient’s journey to wellness. When a patient first experiences some type of unusual symptom that is concerning to them, they reach out to their medical professional or seek direct medical care through another entry point to the healthcare system. This may include Urgent Care Clinics, Virtual Healthcare, or Emergency care. This is the point when AI technology may first impact this patient’s outcomes. As medical records are becoming more interoperable, medical professionals have access to patient’s health information, and AI technology can quickly scan these records, or other uploaded documents to assist medical provider to determine “how” current symptoms may be related to past known medical information. The provider can also quickly determine what medications, and possible side affects may be related to current symptoms. The fast speed of reading healthcare data helps providers understand “If” current reported symptoms are related, or “If” a new condition or problem has arisen.
AI software can scan for “key words”, speeding up a search if a specific diagnosis, or symptom has been documented before. This type of technology can scan electronic records for pre-selected words. NLP software can also be utilized to read narrative notations in the medical record. Pre-programmed words or phrases assist this technology to scan documents to convert specific information to a summary version quickly.
For this example, let’s use a case study example of a patient with new onset of symptoms of chest pain. The patient is presented at the hospital’s emergency room. At intake, the provider accesses the patient’s electronic health record (EHR) and obtains medical history. EHR is quickly scanned using AI technology. Within minutes, medical history reveals that the patient has a history of asthma and anxiety. There is no recent history of an accident, or injury. No history of other medical diagnosis is available and all the problems are noted. Patient reports pain in “mid-chest and mid-back”. “It comes and goes and is sharp and burning, but sometimes feels like someone is pushing against me with pressure”.
The Second use of AI technology will be in the diagnostic phase of care. Radiographic testing will likely be ordered for this patient. Radiography is reliant on imaging technology. This technology helps capture high quality images, and helps interpret what the pictures reveal. Without use of AI technology, the diagnostic phase would be slowed considerably. This technology also indicates possible diagnosis, as well as interventions. Increase in usage of radiography for diagnosis is directly related to advances in imaging technology and computerization. These advancements have benefitted patients with diagnosis and treatment.
Radiology technicians have benefitted from advancements in technology to assist them with machine vision and machine perception. It is easier for the technician to determine if a patient is in the best position to obtain accurate results. This technology also speeds up time by reducing the number of images that must be taken. Ultimately, it reduces the stress and pain that patient experiences by reducing the number of times they must be moved, or repositioned. Positive consequences of AI technology in radiographic examinations are: increased efficiency and throughput with imaging departments. It has increased accuracy of results, while speeding up the time needed from examination to results.
Machine learning in radiography has had positive effects on outcomes for patients. Algorithms are out- performing radiologists in spotting malignant tumors and have assisted with biomarker testing. AI technology has help improve diagnosis to cure rates for multiple conditions. Design, Implementation, and Evaluation of AI enabled solutions have revealed the importance of marrying data science with disciplines, such as incorporation of evidenced based interventions alongside of automated digital identification.
For our patient, the incorporation of radiologic AI technology helps speed up the time to diagnosis, and improves the accuracy of that diagnosis. Let’s assume that the x-ray’s reveal that the lungs do not show any abnormalities. The EKG shows no abnormalities in the heart. More time is needed for laboratory tests, but preliminary results are back. Lab results show White Blood Cell counts within normal limits. Red Blood Cells are low, as well as the Hemoglobin and Hematocrit. The computer recommends more diagnostic testing. Machine Learning Software indicates that more testing is needed. Since the levels are significantly low, the decision is made to admit the patient to the hospital. While diagnosis is not completed at this point, the patient’s initial symptoms are being managed, and is comfortable. Total time for this case thus far, less than 4 hours. Prior to AI technology, this time to treatment, thus far, could have resulted in many more hours, and more hours of pain for the patient.
Analysis
Clinical Decision Support System (CDSS)’ main objective is to diagnose the disease efficiently by using previous patient data. Features presenting for a patient can be matched to a computerized pre-existed medical dataset. AI technology recommends the diagnosis. Clinicians combine their knowledge with results from the AI software, and are able to make quicker informed decisions.
According to the National Academies of Science, Engineering, “Diagnostic mistakes lead to roughly 10% of patient fatalities, and 6 to 17% of hospital problems. Diagnostic mistakes, according to experts, are caused by: collaboration and integration of health information technology is inefficient (Health IT), com munication breakdown between physicians, patients, and their families, and healthcare work system that is designed to be insufficiently supportive of diagnostic procedures.
AI driven systems have been shown to outperform humans in critical analysis. Improvements in technology have aided in more accurate diagnosis of Cancer, Cardiac Conditions, and in renal conditions. Precision Medicine is the most common use of AI in healthcare. It predicts what treatment protocols are likely to succeed on a patient based on various patient attributes and in the treatment. Machine learning using a “neural network” has been in development since the 1960’s. It is well established in research, and is commonly used to assist in diagnosing and treating many health conditions.
Rule based systems have been in use since the 1980’s. In healthcare, they have been used in clinical decision support purposes over the last couple of decades. Many EHR providers furnish a set of rules with their systems. It allows EHR records to provide analytic information for reporting.
At the time our patient transfers to the inpatient general medical/surgical unit at the hospital, her care is transferred to a new physician, and a new care team. The nurse completes an additional assessment for admission to the hospital. The Machine Learning Technology that uses Natural Language Processing (NLP) tools will transform health information from previous health information records, medical process notes, and laboratory/diagnostic results assists the nurse to complete the assessment faster, incorporating relevant data into assessment. This information reduces redundancies in repeatedly asking questions. The technology also assists in recommending interventions and developing a care plan.
AI technology assists to transform aggregates of health information into a usable format. Nearly 80% of information kept or “locked” in electronic health record systems is unstructured data. Machine learning in healthcare frequently uses natural language processing (NLP) tools to transform data in to analyzable data.
Conclusions:
AI in healthcare has a significant place in disease diagnosis and prediction, information processing, saving clinician’s time, speeding time to treatment, and in cost savings to patients and providers. The future of AI in Healthcare seems assured. Research and development have moved healthcare further down the road to acceptance of this technology.
The most challenging problems are in:
• Securing acceptance of this technology
• Cost of securing the technology
Outcomes have proven that this technology is playing a critical role in medical management, and that its usage will continue. The benefits far outweigh the barriers.
AI is most often utilized in healthcare for:
• Help with diagnosis
• Administration of healthcare
• Interpretation of health information
Summary
AI technology has had positive impacts on outcomes for patients. It is likely that the progression of this type of technology will continue to expand. Outcomes across multiple disease conditions have shown reduced total dollars spent with reduction in healthcare tests, number of visits, length of stay in the hospital, and targeting specific treatment with higher cure rates. This technology has reduced the number of hours needed for staff, and the results have been mostly for the good. On the diagnostic side, this technology has shown a smaller error rate than historic results, indicating that this technology, when used alongside human knowledge, can improve overall outcomes. References are available at www.americanhhm.com