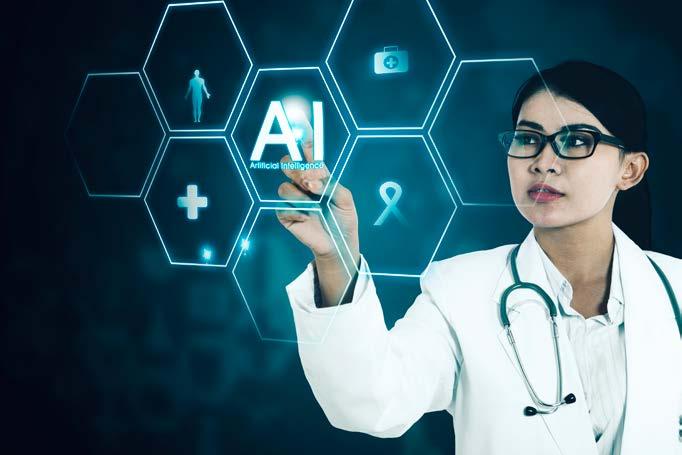
14 minute read
Artificial Intelligence and Digital Health
Artificial Intelligence is rapidly transforming healthcare. From tentative footholds in radiology and clinical laboratories, it is blossoming from a diagnostic tool to a generative prodigy that will soon surpass human expertise leading to better control and management of chronic disease, the root cause of all acute diseases, to their cure.
James Doulgeris Chairman, Population Health Advisory Board, RSDSA
Advertisement
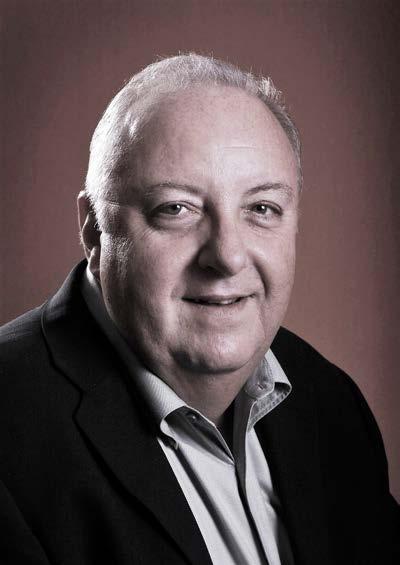
1. How would you describe the role of artificial intelligence (AI) in the field of digital health, and what potential benefits does it offer to patients and healthcare providers?
Artificial Intelligence (AI) is rapidly transforming the practice of healthcare. From tentative footholds in radiology and clinical laboratories, it is blossoming into an essential diagnostic tool that is tireless, flawless in its detailed examinations and performs it all with impressive speed and fidelity.
For now, it is also only as good as its programmers, relying on humans for insight, performing the routine work and leaving the thinking to people. As AI evolves and learns, it will soon surpass human expertise leading to better control of chronic disease, the number one cost and killer to earlier diagnoses and cures for everything from viruses like COVID to cancers.
Here are the key areas where AI has made significant contributions in today’s healthcare landscape:
1. Disease Detection and Diagnosis: AI algorithms can process and interpret medical imaging at a speed and accuracy level that exceeds human capability leading to earlier and more accurate diagnosis of conditions ranging from cancers to cardiovascular diseases. With virtually every disease state, early diagnosis is the key to a successful cure.
2. Predictive Analytics: AI can analyze numerous data points from a patient's medical history, genetic information, and lifestyle habits, called social determinants of health, to predict the risk of chronic disease leading to acute events and allow for early intervention. This capability impacts a range of areas to improve health status from long term chronic conditions to avoiding hospital readmissions.
3. Treatment Personalization: AI has become an essential tool to develop personalized treatment plans in an area called pharmacogenomics, which is the study of how genetic makeup can affect one’s response to drugs by considering a patient’s medical history, genetics, and lifestyle. Also called precision medicine, pharmacogenomics can dramatically improve the efficacy of treatments and reduce sideeffects tailored to each individual.
4. Drug Discovery: AI can expedite the process of drug discovery and clinical testing by predicting how different chemical compounds interact. As AI moves into generative stages (self-learning), the development of new treatments for a multitude of conditions can be accelerated by years.
5. Population Health: AI has made managing health status and trends from an individual to a population basis possible. By gathering patient data in electronic medical records to create single page patient overviews for primary care physicians, AI has moved the physician relationship from the exam room laptop to the patient by summarizing everything from diagnostic testing to vital sign trends. On a population health basis, by managing big data, AI provides tools to manage chronic disease on large scales to identify comorbidities associated with primary disease states from rare diseases to diabetes, kidney disease and cancer to name a few.
6. Risk Identification and Management: As a general rule, fifty percent of the cost of healthcare is created by five percent of the patients. All of these patients are in an acute stage of illness. It is also true that under the age of 65, over 75% of the cost is caused by chronic disease and over 65 the cost raises to over 95%. There are three stages of health status – episodic, chronic, and acute. Episodic conditions are events like injuries that will heal with little or no lasting effect. Chronic diseases need to be managed to be slowed, stopped, or reversed so that they do not progress to an acute stage. Using AI to assess risk to identify patients in the 75th to 95th percentile, or those who will move to the 95th to 100th percentile that consumes 50% of our costs is the key to population health management. While we are putting things in terms of cost, we are really talking in terms of managing the health status of people – meaning their quality of life, their life span, their time with their families. Integrating new information datasets such as social determinants of health (like socioeconomic status, education level, physical environment) in their risk predictions and treatment recommendations can enhance the effectiveness of chronic disease management while leading to more holistic and equitable healthcare.
7. Administrative Tasks and Data Accuracy: AI can do more than assist with administrative and clinical record-keeping tasks such as scheduling appointments, billing, and patient records management, it can perform them virtually autonomously allowing healthcare staff to focus on patient care while minimizing human error. Taking human error out of practice and hospital operations is not just an administrative matter of efficiency, it is a critical component to ensuring the quality of data entering population health systems is accurate. Poor data leads to poor information. Poor information leads to improper actionable information, which can and does have a negative impact on patient care and outcomes.
8. Telemedicine: AI literally makes telemedicine possible because of the shortage of clinical personnel and the preference of the younger generation to interact virtually whenever possible. Chatbots and virtual health assistants can provide 24/7 support, answering patient inquiries, and accurate and essential triage ensuring available clinical personnel are treating cases best managed by human intervention. Again, with generative AI, as these systems learn, they will be able to manage more and more satisfying the needs and wants of the emerging patient base as it ages into the system.
9. Mental Health: AI tools like chatbots already offer psychological support and interventions, supplementing traditional mental health care services and serving to triage acute cases to human intervention.
10. Remote Monitoring and Wearables: AI is used in devices that monitor vital signs, physical activity, fall monitoring and emergency alerts, sleep patterns, glucose levels, oxygen levels and much more are commonplace. Many of us use them without even thinking about their function in the form of smart watches. Wearables and remote monitoring devices already play an important role in alerting emergency services of acute events from heart attacks to falls. If a patient does not cancel the alarm, help will be on the way, an essential when time is literally a matter of life and death.
2. What are some key applications of AI in digital health that have improved healthcare outcomes?
Here are some specific examples where AI has been employed to make a significant improvement in healthcare:
1. Medical Imaging and Diagnosis: Google's DeepMind is an AI system that can diagnose eye diseases as precisely as the best ophthalmologists. Zebra Medical Vision reads medical imaging such as X-rays, CT scans and MRI scans to detect a range of conditions autonomously efficiently, accurately and rapidly.
2. Predictive Analytics: AI systems can examine patient data from everything from wearables to hospital monitors in real-time to predict potential health crises before they happen. For instance, Google's DeepMind can predict an acute kidney event up to 48 hours before it happens, providing doctors with a valuable window to intervene and save lives.
3. Precision Medicine: Tempus uses AI to treat cancer using pharmacogenetics and by analyzing clinical and molecular data. This allows physicians to make real-time, datadriven decisions, improving outcomes.
4. Drug Discovery: Atomwise uses AI to predict molecular combinations that are most likely to have a desirable therapeutic effect for specific disease states, significantly speeding up the process of drug discovery, speeding the research and development stage for new drugs substantially.
5. Mental Health Support: AI chatbots like Woebot provide psychological support helping patients to manage their mental health. While not a replacement for professional help, these tools support traditional therapy by providing immediate help whenever it is needed.
6.Remote Patient Monitoring: The IWatch continues to make impressive strides by measuring oxygen levels, detecting atrial fibrillation, sleep disorders, falls with automatic calls for emergency help and soon, glucose levels for diabetics in real time. It also provides a wide range of other health and fitness metrics when paired with the iPhone health app.
New applications are coming online, particularly those using generative AI in a disease, diagnostic and individualized class basis quickly becoming more effective the more they are used, promise to revolutionize healthcare in ways that we have yet to appreciate.
3. How do you ensure the ethical use of AI in digital health, particularly in terms of patient privacy, data security, and algorithm transparency?
Ensuring the ethical use of AI in digital health is crucial to maintain trust and protect the interests of patients. This has always been a dilemma with healthcare analytics and using healthcare data, particularly big data. The more data there is available, the better the systems work. Deidentification is not hard to accomplish. On the other hand, deidentifying patients denies them the benefits of early diagnosis of diseases that could be life altering or threating. Finding a balance is challenging. Nonetheless, here are some measures that can help:
1. Patient Privacy and Consent: Patient privacy laws like the Health Insurance Portability and Accountability Act (HIPAA) in the U.S. or the General Data Protection Regulation (GDPR) in the EU provide protection at the expense of a massive, and expensive bureaucracy. Deidentification and informed consent combined with what the data will be used for provides the belt and suspenders underpinning patient privacy.
2. Data Security: High level cybersecurity is essential. Regular security audits, vulnerability assessments, and the use of encryption can help ensure data is kept safe.
3. Algorithmic Transparency: To mitigate issues related to equity, bias and fairness, there should be reasonable transparency about how algorithms work and make decisions.
4. Validation and Testing: AI systems should undergo rigorous testing and validation before they are deployed and be continuously monitored post-deployment as well.
5. Accountability: It is essential that a chain of accountability within each organization be in place so that when things go wrong, they can be addressed promptly and by qualified personnel.
6. Inclusivity and Fairness: The data used to
Intelligence
Digital Health the future of healthcare, unleashing a new era of precision medicine and patient-centric care. train AI systems should be obtained from a standardized data set to ensure they are representative of diverse populations to avoid bias in predictions and recommendations.
4. Can you share specific examples of AI-driven technologies or solutions that have had a significant impact on patient care and health management?
AI has had its greatest impact in diagnostics, particularly for radiology and ophthalmology:
1. Aidoc analyzes CT scans to identify acute abnormalities such as strokes, pulmonary embolisms, and cervical spine fractures.
2. Zebra Medical Vision reads and analyzes X-rays, CT scans, and MRI scans serving to detect a wide range of cancers, cardiovascular conditions, liver diseases, and related conditions earlier than traditional radiology substantially improving outcomes.
3. Google's DeepMind worked with Moorfield’s Eye Hospital to develop an AI system that can diagnose eye diseases with a rate of accuracy equal to or exceeding the best ophthalmologists leading to earlier diagnosis and improved outcomes.
4.AliveCor's KardiaMobile, pairs with a smartphone to detect atrial fibrillation, a common heart rhythm disorder that if left untreated, can lead to serious health issues like stroke. iPhone pairs with iWatch to perform the same function.
5. Butterfly Network's Butterfly iQ is a handheld ultrasound device connected to a smartphone to capture and analyze ultrasound images enabling point-of-care ultrasound imaging, making medical imaging more accessible and convenient.
6. Tempus analyzes clinical and molecular data to help physicians make real-time, data-driven cancer care decisions. Their work in genomics and precision medicine has been transformative in providing personalized treatment plans for cancer patients.
7. IBM Watson Health for Oncology uses AI to analyze a patient’s medical information and offers evidence-based treatment options. It helps doctors make more informed decisions about patient care.
5. What challenges or barriers exist in the integration of AI into digital health systems, and how do you address them to ensure successful implementation?
AI in healthcare has a set of challenges that must be overcome. Here are my top six areas that give me the most concern:
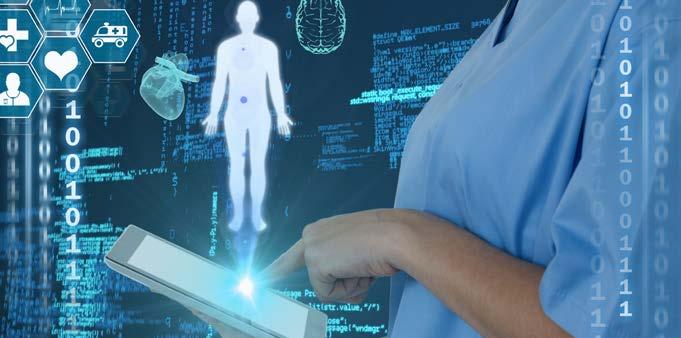
1.Data Privacy and Security: Stringent privacy laws hurt as much as they help. A lot of work must be done for laws to catch up with technology to make the two work effectively together. This is a big ask because the knowledge gap between legislators and technology companies is broad and widening by the day.
2. Data Quality and Interoperability: Inconsistent or incomplete data can reduce the accuracy of AI systems. The human factor is a systemic and continuing concern, and it is not going away anytime soon. The newer generations moving into the workforce do not have the same work ethic and training to maintain consistency and accuracy as the generation leaving the workforce. This is a societal issue that, ironically, AI must be used to overcome. Moreover, different healthcare systems and vendors often use different formats or standards for their data, making interoperability a significant challenge. This is where legislation lags behind reality. Requirements for standardization have been put in place but are not enforced. It is time for the public and private sectors to get on the same page for the public good.
3. Lack of Understanding and Trust: Many healthcare providers may not understand AI well enough to trust its use in patient care, and their concerns are not unfounded. If AI makes a mistake, it could potentially lead to patient harm. Healthcare providers are data driven and cost benefit studies are required to illustrate the overall benefits of AI usage versus delaying usage. Delaying progress to achieve perfection is a very expensive undertaking.
4. Regulatory Challenges: Particularly in the United States, federal regulation and state regulation are at odds with voids and considerable inconsistencies. Again, everyone must get on the same page for the public good.
5. Ethical and Bias Issues: Using broad databases representing a true public model without political or assumed bias in a standardized model for all AI training will ensure that everyone is working from reality instead of a politicized construct. This is easier said than done. It will take considerable leadership and political will to get this right.
6. Technical Infrastructure: Healthcare providers need a strong technical infrastructure to implement AI. Not all healthcare systems may have the necessary hardware, software, and technical expertise. Using the cloud as a common system is an obvious solution, however, it also has a glaring vulnerability because it represents a singular, very large target.
6. How do you strike a balance between the use of AI and the human touch in healthcare delivery, and how does this impact patient experiences and outcomes?
There is no reason to strike a balance between using AI and the human touch in healthcare delivery because AI is a tool to enhance the quality, efficiency, and effectiveness of patient care, not a replacement for it.
When I was at Osler Health, we did a study comparing over 10,000 patients who received only physical therapy for chronic back pain and an equal number of patients who received only chiropractic care. Surprisingly for us, the patients receiving chiropractic care cost over $2,000 per year each less than those receiving traditional physical therapy at a savings of over $20 million dollars per year.
Upon further investigation, the patients were happier, healthier and reported lower pain levels. What made the difference according to patients? Physical therapy, while it delivered results, was work, it was painful, it was demanding. Chiropractic on the other hand did not necessarily deliver the same clinical results, but it was nurturing, caring and made them feel better overall.
Similarly, AI while can provide extraordinary efficiency, precision, and access to new forms of treatment it cannot replace the empathy, understanding, and human touch and connection that a human healthcare provider can offer.
Simply, good news or bad news, it is also all about faith in the caregiver, not the machine assisting the caregiver regardless of how smart, advanced or competent it is. AI cannot hold a patient’s hand, reassure them or give them a hug.
7. What are the most promising areas or domains within digital health where AI can make a transformative impact in the near future?
Artificial intelligence has a singular advantage over human intelligence – the ability to process massive amounts of data and transform it into understandable and often actionable information.
The advent of generative AI, or artificial intelligence that improves itself autonomously, is going to be the game changer going forward.
This is a generation beyond machine learning and a step into cognitive intelligence where decisions cease to be made by algorithms and begin to be made using applied experience, intuitive pattern recognition and sentient intuition. The most important aspect of this newfound capability would be curiosity and independently taking the initiative to satisfy it.
A good example would be taking a large dataset of emergency room visits and wondering why some areas are higher than others. Then taking the initiative to regionalize the higher areas, match them to physician practices and having the intuition to visit practice websites to determine if the practices closed for lunch, were available for off hours call and comparing them statistically to practices where emergency room visits were lower.
This would be a daunting task for an analytic staff, if the staff were available for a weekslong study despite the millions of dollars that could be saved and pressure removed from hospital emergency rooms, a reward of tens of thousands of dollars for every dollar spent on the study.
For an advanced AI system, the study would happen automatically with a full report and detailed actionable plan distributed to management in the time it would take for management to pose the question.
And, this one simple intuitive spark, one of so many they would have to be prioritized by the level of impact to avoid overwhelming the human side of the system, would materially improve patient safety, services, efficiency, effectiveness, and cost in both hospitals and practices alike.
The same impact on the clinical side of healthcare benefiting hand in hand with infrastructure improvements would not just be transformative, it would be revolutionary.
The inherent bias of politics, special interests, unions, medical specialties, local, state and federal governments and bureaucracies and all other stakeholders must agree to stand aside and allow a complete dataset representing the entire population as it is be the baseline dataset.
These facts will be our friends and a robust AI system will be the tool to provide actionable information and a plan to improve upon them.
9. How does AI contribute to personalized medicine and treatment plans in the context of digital health, and what challenges do you face in implementing this approach?
Presently, AI is a great tool for physicians and other healthcare professionals. In the near future, it will be a brilliant collaborator that provides unparalleled insight while physicians interpret and communicate on a human to human basis.
10. How do you involve healthcare professionals and patients in the development and evaluation of AI-based digital health solutions to ensure user-centered design and usability?
I have a hard and fast rule before implementing new systemic programs: collaborate twice with three separate groups then program once. Field test, adjust. Repeat. Repeat then repeat again until every group in every region is reasonably satisfied.
I also have one other rule – never let perfection stand in the way of progress. Set a reasonable timeline and stick to it.
11. Can you discuss the role of AI in predictive analytics and early detection of diseases in digital health, and how it can contribute to preventive healthcare?
Predictive analytics are all about preventative care, whether they are employed for risk management or used in a diagnostic setting to detect disease in a lab or imaging study. The smarter AI becomes, the more accurate and complete the instructions, information and directions will be to the healthcare provider.
12. Looking ahead, what future trends or advancements do you anticipate in the intersection of AI and digital health, and how do you plan to stay at the forefront of these developments?
Staying at the forefront of any technology is a matter of planning, prioritizing and deployable resources.
The field is racing forward so quickly in so many ways and areas, it is not so much what to keep up with but what is most needed and what providers can most afford.
Other considerations are hardware, how to pay for it and where to put it if systems are not cloud based and, if they are, how to securely move all those masses of data securely in limited bandwidth. Then there are added operating costs from energy to debt service.
Analytic systems can and should make up their cost with savings, but in a hospital system, for example, cannibalizing revenues to share savings can and usually is a losing proposition, and a big one if it is done in a big way. The hospital world is still a fee for service world.
Since medically retiring after 35 years in CEO roles in hospitals and value-based care providers, James Doulgeris stays active by advising healthcare companies and systems in the AI and analytics spaces, and as a healthcare journalist and novelist. He also volunteers to develop analytic tools to create actionable information to improve care and treatments for the rare disease community.