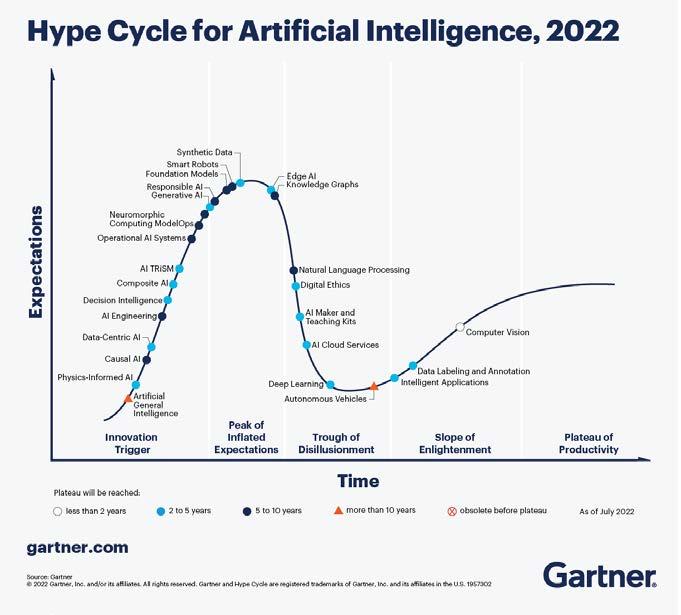
4 minute read
Artificial Intelligence for Brain MRI
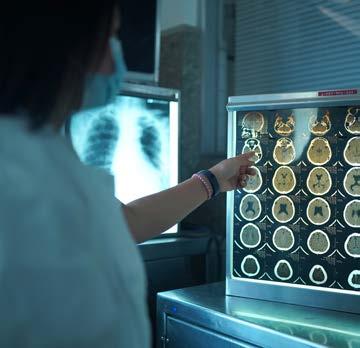
AI is transforming health care, particularly in radiology given the large digital datasets. Neuroradiology applications such as brain MRI dominate the research arena, though clinical translation and validation have been limited. This article reviews the scope of neuroimaging use cases including normal variation, disease, safety, intervention, and workflow optimization.
Advertisement
Artificial intelligence (AI) is a broad and evolving field that develops computer systems to simulate human intelligence in areas such as visual perception, speech recognition, and decision-making. AI is currently transforming society and health care on multiple fronts including robotics, telemedicine, electronic health records, clinical decision support, and outcomes prediction.
A vast array of approaches can be utilized, including statistical machine learning and deep learning with neural networks. The Gartner hype cycle for AI illustrates the timing and trends for different subtypes of AI, from oldest (computer vision) to more recent technologies such as generative AI and neuromorphic computing. (Figure 1. )
For each field of medicine, there are many opportunities as well as challenges. Radiology—which uses imaging technology to diagnose and treat disease—is a logical use case with large and diverse digital datasets.
Neuroradiology is one of the most challenging and interesting applications, with the complexities of brain structure and function serving as inspiration for the architecture of modern neural networks.
The workhorse of neuroradiology is magnetic resonance imaging (MRI), which utilizes magnetic fields to measure tissue properties in response to different magnetic pulse sequences. For a neuroradiologist like me, learning to interpret a brain MRI requires years of training and practice to appreciate the complex variations related to technique, age, and disease. After reviewing each examination, I create a radiology report that contains the details of diagnosis and recommendations for management. In this fashion, radiologists play pivotal roles in the imaging care cycle of every patient. Upstream steps include examination ordering by the referring clinician, patient scheduling, study protocoling, performance of the scan by a technologist, image generation, and addition to the radiologist worklist. Downstream steps can include image postprocessing to improve detection or diagnosis, secure data transfer to an approved site, and communication with the referring physician or patient.
Neuroimaging is a hot topic in AI radiology research, accounting for nearly 25% of all publications. However, few ideas actually make it to market, of which the majority are vendor-funded with a paucity of peerreviewed evidence, demonstrable efficacy, and generalizability. Moreover, there is vast heterogeneity in deployment methods, pricing models, and regulatory classes. In other words, the very complexities that make neuroradiology an attractive area for AI research have profoundly limited its clinical validation and translation. In this article, we will review the literature on potential AI use cases in neuroimaging, from normal age-related variation and disease processes through interventions and life cycle optimization.
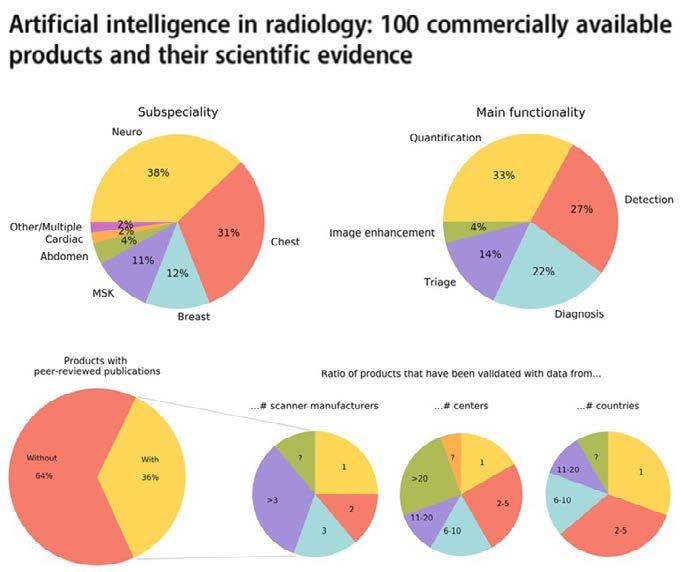
Lifespan: AI algorithms trained on global brain MRI repositories can provide standardized normative templates across the human lifespan with respect to age, sex, and race, corrected for site-specific technical parameters. These results can help quantify normal population variation and developmental stages, as well as improve identification of disease phenotypes with different treatment responses and outcomes.
Perinatal: AI can enhance fetal and neonatal image quality (accelerated scanning, motion correction, denoising); automate postprocessing and segmentation (biometry, volume reconstructions); perform image analysis (disease detection, classification); and provide decision support (outcomes prediction, need for intervention).
Congenital: AI can improve characterization and quantification of complex brain malformations such as callosal hypogenesis, cerebellar hypoplasia, malformations of cortical development, and holoprosencephaly.
Tumor: AI can perform standardized feature extraction (tumor mapping, atlas deformation, metastatic disease burden); enable precision diagnosis (integrated with histology, grade, molecular information) and therapy (targeted biopsy, radiation, medical treatment); and facilitate decision support (tumor aggressiveness, response to therapy, quality of life, survival). (Figure 2)
White matter: AI can help classify and quantify lesion burden, assess temporal evolution, provide differential diagnosis, and predict functional outcome in white matter disorders.
Epilepsy: AI can provide multimodal correlation and quantitative feature extraction to improve lesion detection and classification; predict patient seizure burden and outcomes; and optimize medical and surgical treatment plans.
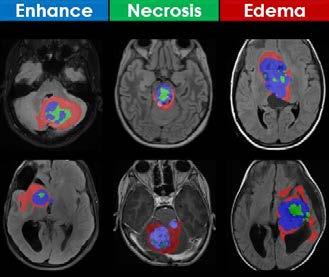
Stroke: AI can assess risk factors and classify stroke subtypes (large vessel, small vessel, venous, perinatal, global hypoxiaischemia); map involved territories and predict progression; evaluate need for/benefits of intervention; and quantify complications (hemorrhage, herniation, hydrocephalus).
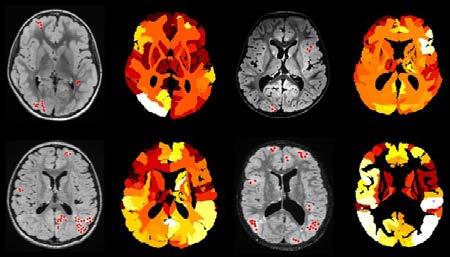
Hydrocephalus: AI can help in segmenting enlarged brain ventricles; determining pattern and etiology (obstructive versus communicating); quantifying shape and volume; and detecting temporal changes that require intervention (shunt failure or overshunting).
Safety: AI can aid with “better, faster, safer” imaging via safety modeling, scan acceleration and denoising, contrast and radiation dose reduction/elimination, and image enhancement or synthesis.
Trauma: AI can evaluate clinical mechanism, grading, and risk scores; determine most appropriate imaging approach; identify acute hemorrhage and fractures; triage types of traumatic brain injury; and predict prognosis and utility of rehabilitation.
Intervention: AI can assist with pre-procedural (patient selection, planning); peri-procedural (efficiency, quantification, navigation, equipment selection); and postprocedural (patient response, management) steps.
Workflow: AI can be applied to optimize the entire imaging life cycle including ordering, scheduling, protocoling, worklist triage, lesion detection, disease classification, image postprocessing, structured reporting, management recommendations, and communication with the referring clinician.
In conclusion, there is a broad spectrum of potential AI use cases in neuroradiology, each at a different stage of development with unique opportunities and challenges. Widespread adoption and implementation at AI will require global consortia and public-private partnerships to facilitate big data sharing for training, testing, and validation. Because radiologic data is uniquely noninvasive, longitudinal, and quantifiable, the use of AI could revolutionize precision medicine approaches when integrated with pathologic, genomic, and clinical information. We are on the cusp of a revolution in digital medicine, where radiologists can serve as technology/innovation drivers and central consultants for an integrated and interconnected model of global health.
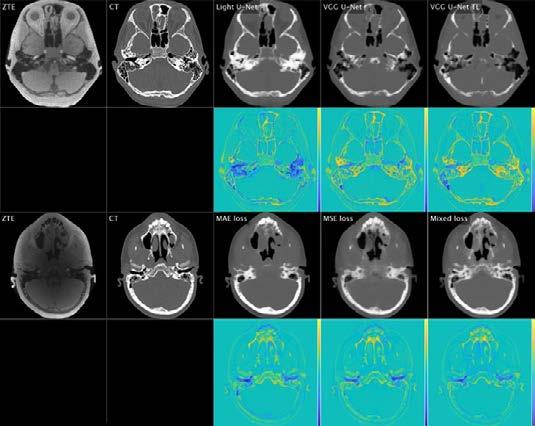
References are available at www.europeanhhm.com
Mai-Lan Ho is Professor of Radiology and an international physician leader, scientist, and educator specializing in advanced imaging and precision health. She studied engineering at Stanford/MIT, medicine at Washington University, radiology at BIDMC/Harvard, and neuroradiology at UCSF. Her books include Neuroradiology Signs, The AAWR Pocket Mentor, and Pediatric Neuroimaging: State-of-the-Art.