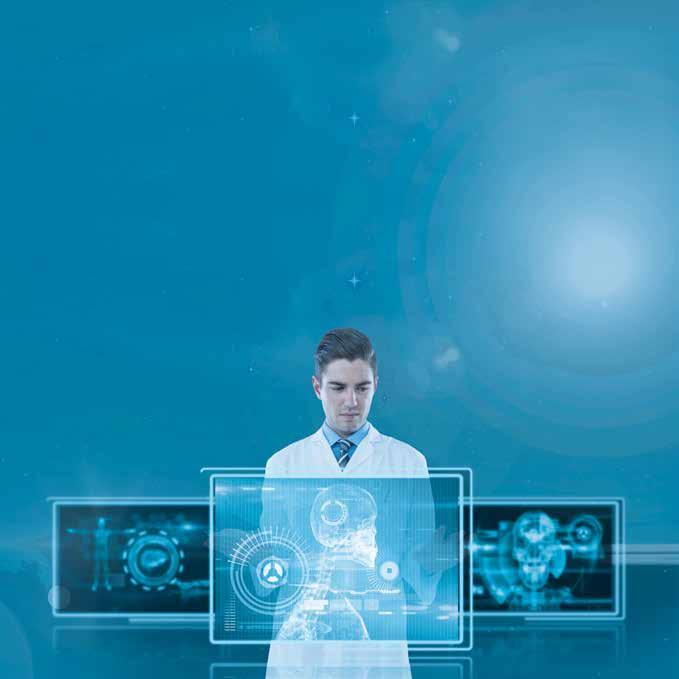
9 minute read
How AI is Transforming the Pharma Industry
In many ways, every aspect of the pharma industry is ripe for AI transformation from drug discovery and the performance of trials, to remote patient monitoring, medication adherence tools and beyond. This article considers some of the potential legal considerations when entering into licensing collaborations for the use of AI, in particular for drug discovery.
Lydia Torne Partner, Simmons & Simmons LLP
The global pharmaceutical industry is currently facing many, wide ranging, challenges, including an aging population, increased life expectancy, a rise in chronic conditions, reduced funding for treatments, reduced numbers of clinical staff, the ever increasing cost of drug development and raw materials, and supply chain issues. Consequently, the pharmaceutical industry is increasingly looking at how a wealth of data, including compound libraries, trial data, and patient data, can be used and reused by artificial intelligence (“AI”) to alleviate these challenges and improve patient care.
The opportunities for AI in the pharmaceutical industry are vast: in many ways, there is no area which is not ripe for AI transformation. For the purposes of this article, we will focus on drug development using AI, though many of the issues discussed may apply to other AI applications in the pharma industry.
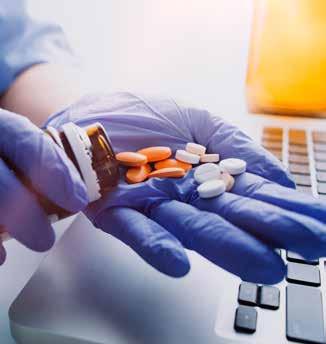
Drug development
Developing a new pharmaceutical product is currently estimated to cost in the region of US$944m to US$2,826bn taking approximately ten years from discovery to approval. Soaring costs reduce the number of drug products being developed (with particular hesitancy in developing drug products for rare diseases where, due to the limited patient population, recovery of development costs may be challenging). The use of “computer aided drug design” (“CADD”) to reduce these metrics by accelerating the identification and early stage discovery and assisting with the development of a new drug product is therefore of great interest.
CADD can be broadly described as the use of AI to identify and/or develop a potential lead drug candidate. For example, to review and assess a small molecule library against a defined target to identify potential “hits”, or to assist with “molecular docking” (e.g. software which predicts both the binding affinity between ligand and protein and the structure of protein–ligand complex) to develop product candidates. Another AI predicts characteristics of the product, e.g. predicting the absorption, distribution, metabolism, excretion, and toxicity properties of the product candidate thereby allowing scientists to better understand the product’s safety and efficacy profile before it ever reaches a trial subject. Likewise, the use of AI can be hugely helpful in repurposing an existing or an abandoned drug product. For example, using an AI model to map an existing drug product onto huge patient data sets to identify whether it might be viable as a drug product for another indication; or to look into why a particularly promising drug product when tested in animals, fails once used in-human.
Once identified, AI can then be deployed to accelerate and improve the outcomes of clinical trials. Increasingly pharmaceutical companies are applying AI to patient data to identify the optimal trial subject, most likely to have the best response to the relevant product.
The use of in silico trials continues to attract attention, “testing” a drug product against data sets for theoretical clinical trial subjects. While, as yet, it is no replacement for in-human studies, it may offer an effective “screening” tool for a product to ensure that a Phase I trial is as positive as possible; it may also be used to complement a clinical trial (reducing the number of enrolled patients and improving statistical significance), and/or support clinical decisions. For example, the PreDICT project used patient data models to assess the cardio toxicity of new drugs.
Legal considerations
While many pharmaceutical companies are trying to hire talented software developers to create and/or oversee the use of complex AI, this is often not an easy task due in part to a global lack of resources, industry culture considerations, and traditionally significant remuneration in the tech industry. Other pharmaceutical companies are entering into collaboration agreements with software companies either for the development of bespoke models or to use “off the shelf” technology.
When entering into these types of collaborations there are specific legal issues which should be considered carefully and addressed. These include the software developer’s rights in and to any product its software identifies or refines, how the software developer should be remunerated and how liability for the product should be apportioned.
Co-developer and co-owner?
If AI is crucial to the discovery of a new drug product, should the software developer (or more controversially, the AI itself) constitute a co-inventor of the resulting drug product pursuant to patent laws? Even if listed as a co-inventor, should it co-own the relevant product patent?
The courts have recently begun considering the issue of AI as an inventor of a patented technology. In Thaler v. Comptroller General of Patents Trade Marks and Designs, Dr Thaler invented DABUS - an AI "creativity machine" which Dr Thaler included as the inventor on a number of patent applications. In the UK, the High Court and the Court of Appeal considered whether AI software could constitute an inventor recognising that the most advanced AI systems operate in a “black box” where their decisions and actions are outside the comprehension and control of the human software developer. The UK courts held that an "inventor" under English law must be a natural person, such that DABUS could not be an inventor. The European Patent Office ("EPO") and the US Patent and Trademark Office ("USPTO") have ruled similarly. While, the Australian Federal Court initially considered that AI, such as DABUS, was capable of being an inventor under Australian patent laws, this has since been overturned on appeal. At the time of writing the only known jurisdiction to have granted patent rights to an AI inventor is South Africa.
Notably, the courts are not empowered to determine whether AI should be an inventor; only whether AI can be an inventor as a matter of statutory interpretation in the relevant jurisdiction. As a result, governments have begun to intervene to question the policy considerations of whether AI should be an inventor. The UK government’s recent consultation on the impact of AI on intellectual property law suggests that respondents are split with many believing AI should not own intellectual property rights in their own name, but the AI developer should logically be a co-owner of any patent for an AI-assisted invention. In view of diverging opinion, the UK government is considering whether AI-devised inventions should be protected through a new form of IP protection.
To best protect their interests, pharmaceutical companies using AI via a licensing collaboration should ensure that the contractual terms of their licensing agreement provide for any inventions and intellectual property rights resulting from the use of the AI to be solely owned by the pharmaceutical company, including appropriate assignment provisions and obligations requiring the software developer to assist in procuring IP protection for the relevant invention and perfecting title to that IP right.
Remuneration structures
Software licences often rely on a one-off or periodic licence fee structure for a certain number of users to use the software. However, if licensed AI is integral to the development of a drug product, which may generate billions of dollars of revenue for the pharmaceutical company, software developers may explore alternative remuneration structures.
While no-one disputes that a pharmaceutical company will need to expend extensive further resource developing and commercialising any drug product identified or refined by AI, there is an argument that the value of the AI software used to make that discovery is far greater than a limited licence fee. The interest in seeking an uplift in remuneration will be intensified if the AI system (or an element of it) has been developed or adapted specifically for that pharmaceutical company, recognising the additional expenditure the developer has incurred to develop a specific program, the potentially limited ability to re-use such specific model, and the market advantage afforded to the pharmaceutical company as a result of having exclusive access to such a bespoke software model. However, applying a usual royalty structure is likely to be considered excessive for the contribution.
Alternative remuneration might be structured by way of milestones payable if/ when the AI identifies a potential drug product, and if such drug product achieves certain stages of development and is ultimately authorised. If a royalty is considered appropriate (perhaps, for example, if the developer is providing a broad package of assistance incorporating discovery, CADD modelling, and even in silico testing) this might be structured as a small capped royalty if sales exceed a certain threshold or addressed via commercial milestone payments.
Liability
In direct correlation with claims for additional remuneration based on the material contribution of the AI system in drug development, is the extent to which the software developer should be liable for the resulting product. If the software is truly crucial to creation, should the developer not also bear some liability?
A usual contractual tool to apportion liability is indemnification. Careful thought will need to be given to the triggers for indemnification. In particular, requiring indemnification from a software developer for issues with the drug product (e.g. defective product) is challenging given the extensive further development performed by the pharmaceutical company and is likely to be resisted. Instead, you might apportion liability slightly differently. For example, providing for indemnification from the AI software developer in connection with issues specific to the software model (e.g. third party IP infringement or misuse of data claims or bias in the algorithm), coupled with a claw back right in respect of milestone payments made (so, if a milestone payment is made for commencing a Phase I trial using the AI generated drug product, which then subsequently materially fails, a portion of the milestone payment would be repayable). If the AI software developer is unwilling to bear any risk and liability for the resulting product this is a strong counterargument for the provision of increased remuneration.
In addition to contractual liability, thought should be given to the AI developers possible statutory liability for any resulting product and/or the software it provides, pursuant to a country’s product liability regime (with those regimes in the EU and the UK currently changing to provide for product liability for software). The EU’s proposed AI Liability Directive will also make it easier for claims to be brought arising out of harm caused by defective AI (including class actions, which are expressly referred to in the Directive).
Pharmaceutical companies should also ensure that their contractual indemnification provisions include recovery of any losses suffered as a result of the AI model being in breach of applicable AI regulations. In April 2021, the EU published its draft Regulation on AI. In its present form, the regulation applies to (among others) providers and users of AI, prohibits certain AI uses and imposes additional obligations in respect of "high-risk AI systems" including increased record keeping, oversight, governance and reporting measures. Currently, AI aimed at drug development is neither prohibited, nor constitutes a “high-risk AI system” (unlike AI driven medical devices). However, as the use of AI in drug development increases, this may change. Likewise other uses of AI by the pharmaceutical industry (e.g. trial subject/patient monitoring) may become considered “high risk” for much the same reasons as AI driven medical devices are. The draft AI Regulation proposes that non-compliance should result in significant financial penalties of up to 2%, 4% or 6% of worldwide annual turnover depending on the nature of the infraction. The UK (and other jurisdictions) is also considering introducing its own legislation in respect of AI. The pharmaceutical industry should, therefore, continue to monitor whether the development and use of AI software in its business is likely to trigger additional regulatory obligations, not least because the use of AI in the context of healthcare and life sciences is likely to attract the attention of regulators due to the material physical harm which could potentially result from an issue with such AI. If so, obligations for ensuring compliance with (and corresponding liability for) these regulatory obligations should be contractually passed to the AI developer.
Conclusion
The above reflects a fraction of the legal considerations associated with the use of AI in the pharmaceutical industry. Further detailed thought should be given to data protection compliance, cybersecurity and regulatory and ethical compliance issues, among many more. While there are many legal challenges to consider, with more to surely reveal themselves in the coming months and years, it should not be forgotten that AI presents a tremendous opportunity for the pharmaceutical industry to improve and accelerate its development pathway and, most importantly, to deliver a material difference to patients.
References are available at www.pharmafocusamerica.com
Lydia Torne is a Partner at international law firm Simmons & Simmons LLP. Her specialisation is in life sciences licensing transactions including, research, and development and commercialisation agreements for early stage, pre-clinical and clinical assets, and related collaboration and consortia arrangements. Lydia has a particular focus on biotechnology assets and digital health.
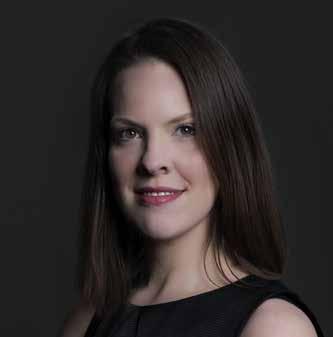