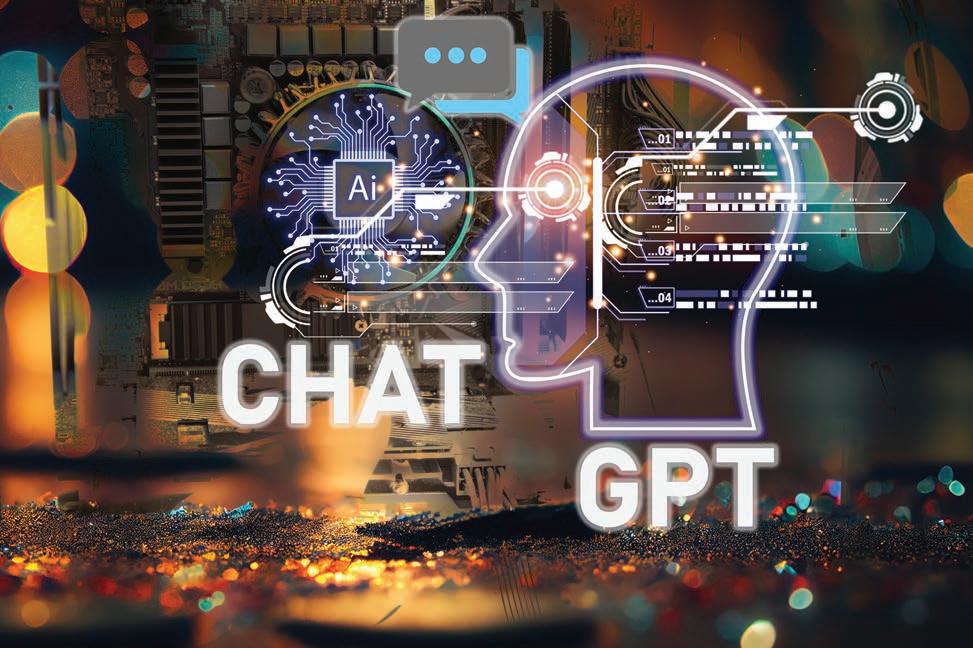
2 minute read
AI hits the big time
by Martin
UNTIL RECENTLY, CYNICS BELIEVED THAT ARTIFICIAL INTELLIGENCE WAS THE TECHNOLOGY OF THE FUTURE AND ALWAYS WOULD BE. BUT THEN C hat GPT ARRIVED AND WE’RE IN A VERY DIFFERENT WORLD
Words: CHRIS PARTRIDGE
SUDDENLY, WE have a system that can search for information on any subject and write on it in correct, vernacular English. Its capabilities range from the trivial to the quite profound.
What is Rihanna’s favourite colour? ChatGPT says “Rihanna has stated in interviews that her favourite colour is green. In particular, she has mentioned that she likes ‘kelly green,’ which is a bright, vivid shade of green.”
What is the historical significance of the voyages of Henry the Navigator? ChatGPT lists his achievements in expanding Portuguese maritime power, not glossing over the slave trade and concludes: “Overall, Henry’s voyages were instrumental in advancing European exploration, colonisation and trade in the Age of Exploration, and helped establish the foundation for the globalised world we know today.”
It can even parody writing styles. I asked it to rewrite the opening to Dickens’ Tale of Two Cities in the style of Ernest Hemingway (because why not?) and it came up with: “In Paris, they did things differently than in London. It was the best of times, it was the worst of times, all at once. That’s how the story goes, anyway.” Which is not bad really.
Understandably, there has been a lot of doom mongering about the devastation of whole strata of middle class jobs, a tidal wave of cheating at school and university and the undermining of democratic debate. And the end of the human race, more than likely.
I will discuss the probable impact of AI on society later, but first, how does it actually work?
A bit of explanation
The central structure of any AI is a neural network, an array of interconnected computer nodes called neurons that work together to process input data and produce output predictions.
Each neuron takes input values and applies weights and biases to produce an output signal that is applied to the next layer of neurons and the process continues until the final output is reached.
The network is trained by presenting it with a set of input-output pairs, the weights and biases being adjusted to minimise the difference between the network’s predictions and the actual output. This is done manually. Once trained, the network is locked and can begin its work.
So far, neural networks have mainly been used for tasks such as image and speech recognition where traditional rule-based approaches are difficult or impossible to implement.
Far from perfect
AI systems have significant weaknesses, however. One is the need to train the system for each application, which is a lengthy and laborious process, but inadequate training can render the system as useless as trying to get a five-year-old to deliver legal judgements.
A more subtle weakness is unpredictable ignorance caused by skewed training data. Facial recognition systems, for example, work badly when presented with black faces simply because (in the US and Europe at least) they amount to less than 20 per cent of the population, so the number of black faces they get to practise on is much smaller than the number of white faces.
Previous attempts to create AI chat systems have been naive,