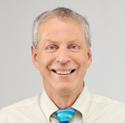
6 minute read
The Doctor Will See You Now
Artificial intelligence (AI) is enabling many advancements in healthcare, ranging from improved quality of care and patient safety, to improved access to care. AI can act like a supervisor looking over our shoulder as we practice making sure that we don’t make mistakes. It can also make us work more efficiently by taking care of menial tasks like typing notes or summarizing a patient record for an insurance company. It is improving our experience as clinicians as well as improving the experience of our patients. But now AI is also making it practical to go one step further: ensuring that our patients are receiving good care every day, all day long, regardless of where they are.
As practicing physicians, we know that even with a much larger support staff we still can’t keep track of all our patients every day. So how do we feel confident that our diabetic patients are keeping their sugars under control, our congestive heart failure patients are watching out for their salt and fluid intake, and our frail elderly patients aren’t teetering on the edge of needing to go to the ED? The answer is through remote patient monitoring and digital therapeutics, both of which are now made practical because of AI.
Remote patient monitoring (RPM) has been around for over a decade. It allows wireless devices such as weight scales, blood pressure monitors, and wearable fitness trackers to record measurements and automatically send them to our electronic health records (EHRs). If there are abnormal readings, we get alerts. There are two problems with this. The first is that historically there have been too many alerts, and that’s where AI comes to the rescue. AI systems are able to not only look at specific values or trends, but also look at patterns to see if a collection of data points is truly pointing to the need for an intervention, or simply an acceptable fluctuation. And the beauty of AI is its use of machine learning that allows it to improve its algorithms over time. Better algorithms mean fewer alerts and a higher positive predictive value that the alerts will be actionable.
The second problem with RPM is that historically they’ve required patient engagement. The patient would have to wear something or remember to step on a scale or take a blood pressure measurement. They’d also have to remember to charge the batteries. But if you’re trying to continually monitor, for instance, a frail elderly patient’s health, the wearable device won’t be monitoring their health while it’s charging. In fact, when that frail elderly patient wakes up, feels crummy and decides to stay in bed, they’re not going to put on their activity monitor, check their weight, or check their blood pressure on the day those readings are most important! Did they not measure their weight because they forgot, or is it because they
are unconscious on the floor? That’s where AI has made ambient patient monitoring possible. Ambient monitoring is like your smoke detector: it continuously and reliably monitors your safety without you having to engage with it or even think about it. There are now wireless radar devices that can privately monitor the activity of patients in their homes, their sleep patterns, and even their heart rate and respiratory rate without requiring the patients to do anything! Slower walking can indicate an impending decline in health [1] and increased risk of falls [2]. Restless sleep can also indicate impending clinical decline [3]. And, with the use of AI, emergency events can be predicted on average 10-14 days in advance using ambient monitors, allowing for plenty of time for interventions to be effective in preventing the need for an ED visit [4].
AI is also helping make digital therapeutics (DTX) more effective. DTX are apps for your smartphone, tablet, or laptop that help patients with chronic conditions take care of themselves. For example, a diabetes DTX app can see that the patient’s sugar is high and, using AI, give them advice regarding their diet or insulin dosing. Behavioral health DTX apps are helping patients control their anxiety and depression without having to contact their healthcare provider. With the shortage of healthcare providers, DTX is giving patients access to care that was otherwise impossible [5]. And generative AI is making it possible to have verbal conversations with voice assistants like Siri or Alexa that can have therapeutic benefits as well [6].
We all want our patients to maximize their health, make good healthcare decisions, and receive good care regardless of whether they are in our office or the majority of time when they are not. While we don’t need to continually be reminded that each of our patients is doing well each day, AI-enabled tools like remote patient monitoring and digital therapeutics will give us the peace of mind to know that they are receiving good care all the time. +
Dr. Garber is an internist, the Medical Director for Informatics, and the Associate Medical Director of Research at Reliant Medical Group.
References
1. Onder G, Penninx BW, Ferrucci L, Fried LP, Guralnik JM, Pahor M. Measures of physical performance and risk for progressive and catastrophic disability: results from the Women’s Health and Aging Study. J Gerontol A Biol Sci Med Sci. 2005 Jan;60(1):74-9. doi: 10.1093/gerona/60.1.74. PMID: 15741286.
2. Adam CE, Fitzpatrick AL, Leary CS, Hajat A, Ilango SD, Park C, Phelan EA, Semmens EO. Change in gait speed and fall risk among community-dwelling older adults with and without mild cognitive impairment: a retrospective cohort analysis. BMC Geriatr. 2023 May 25;23(1):328. doi: 10.1186/s12877-023-03890-6. PMID: 37231344; PMCID: PMC10214622.
3. Schütz N, Saner H, Botros A, Pais B, Santschi V, Buluschek P, Gatica-Perez D, Urwyler P, Müri RM, Nef T. Contactless Sleep Monitoring for Early Detection of Health Deteriorations in Community-Dwelling Older Adults: Exploratory Study. JMIR Mhealth Uhealth. 2021 Jun 11;9(6):e24666. doi: 10.2196/24666. PMID: 34114966; PMCID: PMC8235297.
4. Rantz M, Phillips LJ, Galambos C, Lane K, Alexander GL, Despins L, Koopman RJ, Skubic M, Hicks L, Miller S, Craver A, Harris BH, Deroche CB. Randomized Trial of Intelligent Sensor System for Early Illness Alerts in Senior Housing. J Am Med Dir Assoc. 2017 Oct 1;18(10):860-870. doi: 10.1016/j.jamda.2017.05.012. Epub 2017 Jul
12. PMID: 28711423; PMCID: PMC5679074.
5. Youn SJ, Jaso B, Eyllon M, Sah P, Hoyler G, Barnes JB, Jarama K, Murillo L, O’Dea H, Orth L, Pennine M, Rogers E, Welch G, Nordberg SS. Leveraging Implementation Science to Integrate Digital Mental Health Interventions as part of Routine Care in a Practice Research Network. Adm Policy Ment Health. 2023 Aug 24. doi: 10.1007/s10488-02301292-9. Epub ahead of print. PMID: 37615809.
Click here for additional reference(s).