
7 minute read
Artificial Intelligence in Mental Healthcare: A Story of Hope and Hazard
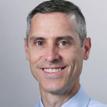
The term artificial intelligence (AI) was coined at a computer science conference at Dartmouth College in 1956. Its use in healthcare began soon after, starting with rule-based systems that followed hard-coded instructions and then evolving to more advanced models that learn independently using data. Though the rate of adoption of AI in mental healthcare has been slower than in other medical specialties, its presence is growing.
AI has the potential to address several challenges faced by mental healthcare. Providers today have at their fingertips an overwhelming amount of data. Electronic health records contain tens of thousands of data points and providers must consider an increasing number of them when making clinical decisions. However, there are limits to how many variables humans can take into consideration at once, and when that capacity is exceeded, we experience information overload. When compounded by stress, fatigue, and competing demands on attention, information overload can lead to errors [1]. One of the most critical decisions providers make is determining suicide risk. Suicide was among the top 9 leading causes of death for people ages 10-64 and remains the second leading cause of death for people ages 10-14 and 25-34 [2]. There are dozens of factors that must be considered when assessing suicide risk, and making accurate assessments is challenging [3]. Healthcare providers struggle to identify patients at risk: 54% of people who die by suicide were seen by a healthcare provider in the month before their death, but clinicians are typically no better than chance at estimating suicide risk [4]. Several AI models have been developed that can sort through large amounts of data to identify patients at risk for suicide. One model, which calculated a risk score using a combination of electronic health record data and patient report, performed better than clinician assessment alone [5]. With rigorous testing via randomized controlled trials, such models might eventually prove to be a cost-effective way to bring at-risk patients to the attention of busy providers [6].
In addition to augmenting clinical decision making, AI can also help advance our understanding of mental disorders. The mental health diagnostic categories used currently are heterogeneous; two patients with different sets of symptoms can receive the same diagnosis. There is also considerable overlap between mental disorders such that the same set of symptoms can result in different
diagnoses [7]. Diagnostic heterogeneity and overlap complicate using diagnostic categories for clinical care and research. Artificial intelligence, particularly a form called unsupervised learning that can discern new patterns in data, can be used to identify subpopulations within diagnostic categories. This approach has been used to identify subgroups of mental health disorders such as depression and PTSD [8,9].
Another challenge in mental healthcare is determining which treatment will work for a particular patient. Patients frequently endure several trials of medications before finding one that is effective. If there was a way to personalize treatment recommendations, then patients could be spared a lengthy trial-and-error process. AI models have been developed that predict responses to a range of mental health treatments, including antidepressants and transcranial magnetic stimulation [8,10].
As with any technology, there are risks associated with using AI in healthcare. AI algorithms can be biased and perpetuate healthcare disparities. In one striking example, a large health insurance company used an AI model to identify patients with greater illness severity to provide them with more support. However, the model incorrectly underestimated the illness severity of Black patients and therefore misjudged the care management support they deserved [11]. Another limitation of AI is that some AI models make decisions in a “black box” fashion, where the model cannot provide the reasoning used to make the decision. This lack of transparency can lead to distrust among providers and patients. A further weakness of AI models is that their accuracy can degrade over time, known as model drift. For example, the dramatic impact that COVID had on healthcare caused some models that were created before COVID to perform poorly [12]. To detect and correct drift, models require monitoring and sometimes need retraining. These maintenance needs may increase the cost of AI compared to traditional methods. Also, AI models can struggle when faced with data they have not seen before, so a model created in one healthcare setting may not perform as well in another. This weakness, known as a lack of generalizability, can limit the widespread use of an AI model.
Healthcare systems can take several steps to mitigate AI risks. Healthcare systems that use AI should have governance in place to ensure that AI is safe, effective, and beneficial [13]. Governance should be performed by multidisciplinary teams composed of providers, patient representatives, bioethicists, and AI experts. These teams can assess AI models for bias, explainability, and generalizability. Healthcare systems should also plan for how to transparently communicate with providers and patients about how AI is being used to deliver care. One can imagine a situation where an AI tool delivers a result (e.g. a risk score for suicidal behavior) that differs from the provider’s judgment. This mismatch might confuse patients and providers and hinder treatment decisions.
The presence of AI in mental health is likely to continue to grow. Providers will encounter AI more frequently and it will become increasingly necessary to understand its risks and benefits. Healthcare training programs should incorporate AI competency into curriculums, similar to how students learn how to critically evaluate biomedical literature [14]. With this knowledge, providers can critically assess AI models they encounter and have informed conversations with their patients about how AI is impacting the care they receive.
AI in mental healthcare today is at a tipping point, where tremendous potential is tempered by significant risk. Research has led to the development of accurate tools, but more work is needed to better understand their real-world impact on providers, healthcare systems, and patients. Such work is underway, and there is reason to be optimistic about the benefits AI may bring to mental healthcare.
Rajendra Aldis, MD, MSCS; Associate Medical Director of Research Informatics, Cambridge Health Alliance Physician Informaticist, Health Equity Research Lab; Instructor in Psychiatry, Harvard Medical School. Email: raaldis@challiance.org
Nicholas Carson, MD, FRCPC; Division Chief, Child and Adolescent Psychiatry, Cambridge Health Alliance Research Scientist, Health Equity Research Lab; Assistant Professor in Psychiatry, Harvard Medical School. Email: ncarson@challiance.org
Disclosures: Dr. Carson receives research funding from Harvard University, NIMH, SAMHSA, and NCCIH.
References
1. Nijor, S., Rallis, G., Lad, N., & Gokcen, E. (2022). Patient Safety Issues From Information Overload in Electronic Medical Records. Journal of patient safety, 18(6), e999–e1003. https://doi.org/10.1097/PTS.0000000000001002
2. CDC, (2023). Facts About Suicide. CDC. https://www.cdc.gov/suicide/facts/index.html
3. Franklin, J. C., Ribeiro, J. D., Fox, K. R., Bentley, K. H., Kleiman, E. M., Huang, X., Musacchio, K. M., Jaroszewski, A. C., Chang, B. P., & Nock, M. K. (2017). Risk factors for suicidal thoughts and behaviors: A meta-analysis of 50 years of research. Psychological bulletin, 143(2), 187–232. https://doi.org/10.1037/bul0000084
4. Ahmedani, B. K., Simon, G. E., Stewart, C., Beck, A., Waitzfelder, B. E., Rossom, R., Lynch, F., Owen-Smith, A., Hunkeler, E. M., Whiteside, U., Operskalski, B. H., Coffey, M. J., & Solberg, L. I. (2014). Health care contacts in the year before suicide death. Journal of general internal medicine, 29(6), 870–877. https://doi. org/10.1007/s11606-014-2767-3
5. Nock, M. K., Millner, A. J., Ross, E. L., Kennedy, C. J., Al-Suwaidi, M., BarakCorren, Y., Castro, V. M., Castro-Ramirez, F., Lauricella, T., Murman, N., Petukhova, M., Bird, S. A., Reis, B., Smoller, J. W., & Kessler, R. C. (2022). Prediction of Suicide Attempts Using Clinician Assessment, Patient Self-report, and Electronic Health Records. JAMA network open, 5(1), e2144373. https://doi.org/10.1001/ jamanetworkopen.2021.44373
Click here for additional reference(s).