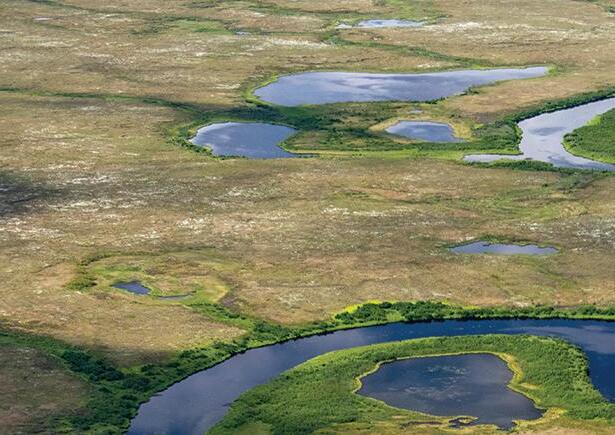
7 minute read
Google grant to advance artificial intelligence methods in Center science
Machine learning and neural networks will help track Arctic change
Maddie Rocklin, Associate VP, 90 West
Sarah Ruiz, Science Writer/Editor
With advances in artificial intelligence technologies, computers can be trained to imitate human language, play chess, categorize images, solve puzzles, write college essays, and even restore damaged ancient texts. With the help of a new $5 million grant from Google.org, the tech company’s philanthropic arm, Woodwell Climate will be putting AI to yet another use—fighting climate change.
The grant will be used to expand the development of the Permafrost Discovery Gateway (PDG), an open-access resource that allows users to visualize and explore data on changing Arctic landscapes. Under the leadership of Woodwell Climate Associate Scientist, Dr. Anna Liljedahl, AI methods will help speed up data processing, allowing researchers to rapidly identify patterns and trends in permafrost thaw. The results will be essential to informing climate mitigation and adaptation strategies.
“Timely tracking of permafrost thaw is critical to assessing impacts and informing action, but current limitations in technology, combined with the rapid pace of change in Arctic landscapes, have held us all back,” says Dr. Liljedahl. “This project will be groundbreaking in speeding up data analysis and unlocking completely new technological capabilities in how we do science in swiftly evolving landscapes, and, ultimately, what science itself can do.”
AI is nothing new
Although AI seems to be a new tool, methods like machine learning and neural networks have been used for decades for their unique talent at recognizing patterns in large datasets. Several projects in Woodwell’s Arctic program already use AI to churn through thousands of satellite images to detect specific features in the landscape.
Data science specialist at the Center, Dr. Yili Yang, uses AI models trained to spot retrogressive thaw slumps (RTS) in permafrost rich regions of the Arctic. Thaw slumps are features that form in response to subsiding permafrost and can be indicators of greater thawing on the landscape, but they are hard to identify in images.
“Finding one RTS is like finding a single building in a city,” Dr. Yang says. “It’s time consuming, and it really helps if you already know what you’re looking for. Their trained neural network can pick the features out of high-resolution satellite imagery of the region with fairly high accuracy.”
Research Assistant Andrew Mullen uses a similar tool to find and map millions of small water bodies across the Arctic. A neural network was used to generate a dataset of these lakes and ponds that allows Mullen and other researchers to track seasonal changes in their area.
With the new Google grant, Dr. Liljedahl says there are opportunities to use AI not just for the data creation side of research, but trend analysis as well.
“Our goals for the Gateway would utilize new AI models to identify trends or patterns or relationships between features like ice wedge polygons and elevation, soil or climate data,” says Dr. Liljedahl.
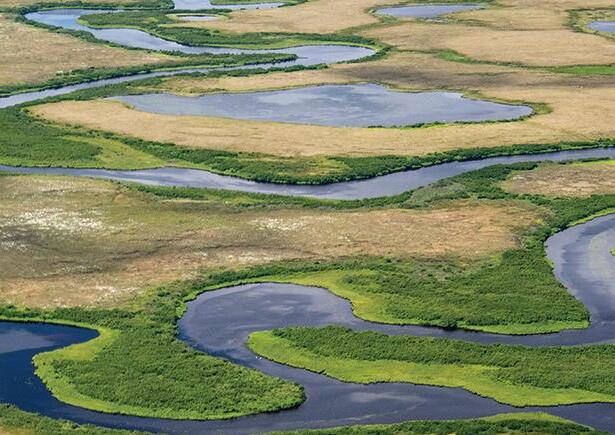
We need speed
Uncovering trends in Arctic change is critical. The Arctic is warming at up to four times the rate of the rest of the planet. Permafrost—or ground that has remained frozen for at least two consecutive years—is thawing rapidly, causing widespread ground collapse and infrastructure damage, threatening Arctic communities, and releasing carbon into the atmosphere.
The technology funded through Google will enable scientists, decision makers, community members, and other interested groups and individuals to explore permafrost thaw features that formed during the previous month, check out seasonal forecasts of permafrost thaw, predict disturbance events based on weather forecasts, estimate carbon and infrastructure loss from abrupt permafrost thaw, and analyze the shape and size of permafrost thaw features and their patterns across the landscape over time. This resource will also be applicable beyond the scope of permafrost thaw, enabling experts to adapt this technology for other research areas and expand access to key climate data and insights across a range of issues and regions.
“Permafrost, often perceived as a distant phenomenon in the remote North, has broad impacts on the world that are yet not fully understood. The Arctic Data Center looks forward to preserving and sharing pan-Arctic permafrost datasets with anyone looking to find and utilize them for scientific research,” says Matthew Jones, Director of Informatics R&D at National Center for Ecological Analysis and Synthesis, University of California Santa Barbara—a partner organization on the project.
The resource will also support Arctic communities in their adaptation measures. Many communities in Alaska and Canada have already begun to feel the impacts of thawing permafrost—enduring collapsed homes and roads and in some cases being forced to relocate.
“Once frozen and resilient, the Arctic is transforming into a landscape that is thawing and fragile. The Permafrost Discovery Gateway is a resource that communities can use to access data, explore climate change impacts, and find resources to adapt in healthy ways,” said Michael Brubaker, Director of Community Environment and Health at Alaska Native Tribal Health Consortium.
“Permafrost covers a huge region of the northern hemisphere land mass, and its disappearance will fundamentally contribute to global climate change,” said Guido Grosse, Head of Permafrost research at Alfred Wegener Institute, Helmholtz Centre for Polar and Marine Research, and a project partner. “New geospatial methods and data processing techniques employed in this project… will provide a first highly detailed look at permafrost thaw and its consequences at the pan-Arctic scale.”
The effort will also collaborate with Permafrost Pathways, another major initiative led by Woodwell Climate, providing data to support their carbon monitoring work.
“This funding through Google.org will allow us to take permafrost carbon modeling to the next level by including abrupt thaw and integrating AI solutions into the estimations of permafrost carbon losses, enabling us to monitor threats to infrastructure in near-real time, estimate future risk, and inform planning to help us better respond to and prepare for permafrost hazards in Alaska communities and beyond,” Dr. Liljedahl says.
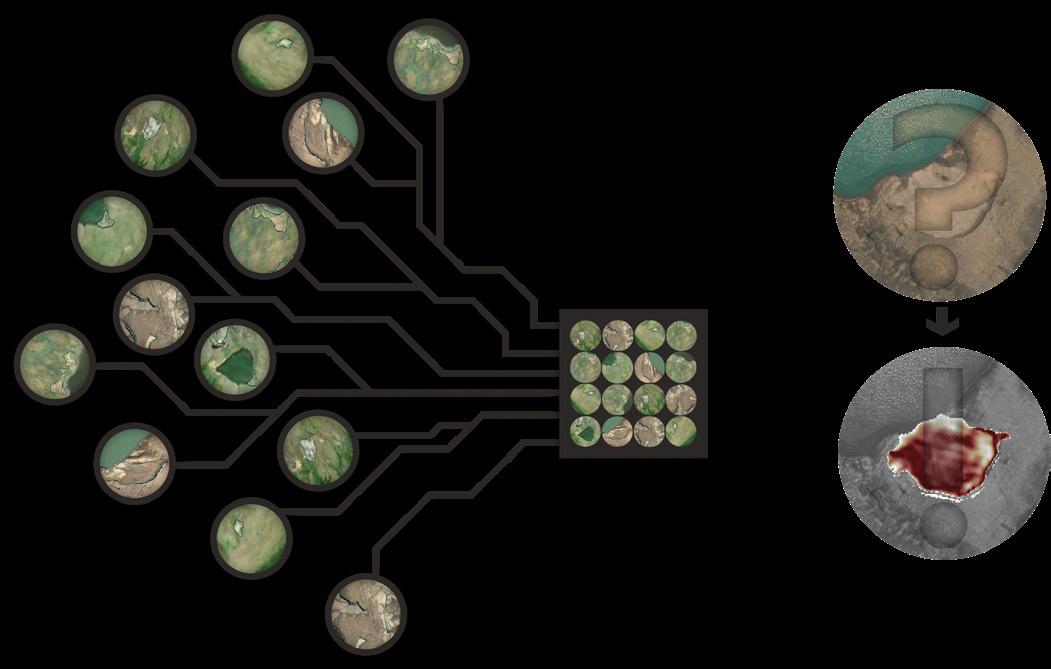
How do neural networks work?
These trained models are all examples of neural network-based AI and the comparison to human brains is apt.
Neural networks are composed of interconnected, mathematical components called “neurons.” Also like a brain, the system is a web of billions upon billions of these neurons. Each neuron carries a fragment of information into the next, and the way those neurons are organized determines the kind of tasks the model can be trained to do.
“How AI models are built is based on a really simple structure—but a ton of these really simple structures stacked on top of each other. This makes them complex and highly capable of accomplishing different tasks,” says Research Assistant Andrew Mullen.
In order to accomplish these highly specific tasks, the model has to be trained. Training involves feeding the AI input data, and then telling it what the correct output should look like. The process is called supervised learning, and it’s functionally similar to teaching a student by showing it the correct answers to the quiz ahead of time, then testing them, and repeating this cycle over and over until they can reliably ace each test.
In the case of Dr. Yili Yang’s work, the model was trained using input satellite images of the Arctic tundra with known retrogressive thaw slump features. The model outputs possible thaw slumps which are then compared to the RTS labels hand-drawn by Research Assistant Tiffany Windholz. It then assesses the similarity between the prediction and ground truth, and automatically adjusts its neurons to improve the similarity. Do this a thousand times and the internal structure of the AI starts to learn what to look for in an image. The organization of those billions of neurons re-configures to produce a reliable output. Sharp change in elevation? Destroyed vegetation and no pond? Right geometry? If so, that’s a potential thaw slump.
Just as it would be impossible to pull out any single neuron from a human brain and determine its function, the complexity of a neural network makes the internal workings of AI difficult to detail—Mullen calls it a “black box”—but with a large enough training set, you can refine the output without ever having to worry about the internal workings of the machine.