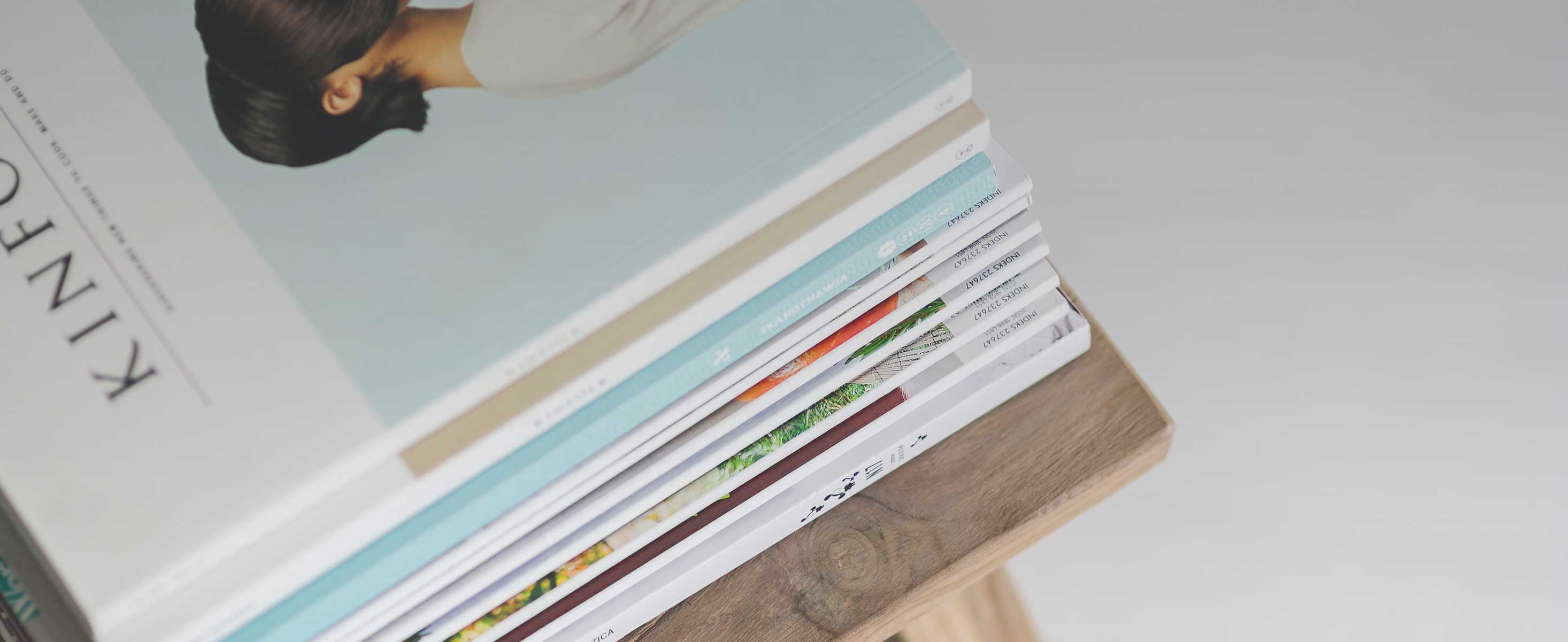
6 minute read
Annex 2A. Methodology for Determining Bank Distress
st A te - owned b A nks versus P riv A te b A nks in sout H A si A 81
fintech/bigtech platforms,20 and bringing financial education to rural areas. The shortfalls involve costly recapitalizations because of weak governance, operational inefficiencies, credit misallocation, and lack of risk management that can trigger large contingent liabilities.
The macrofinancial benefits can involve some stabilization of the credit cycle through countercyclical lending. But this benefit comes at a large cost of greater capital misallocation to less productive firms and large fiscal costs of recapitalization that is needed to backstop lending in downturns and that can crowd out other important public expenditures, such as on health care and education. The proposed reforms are urgently needed for SOCBs in South Asia and beyond to clearly generate net socioeconomic benefits.
identifying Distress Using Financial Soundness indicators
We define a distress event as the breach of a quantitative threshold. In principle, the threshold could be determined by an economic relationship (identity), predicted/expected value, or even a practical rule of thumb. The threshold value I , together with an actual value of an indicator I, then help determine distance to distress and generate a dummy variable, Di,n,t, identifying observed distress.
We identify distress at Indian public sector banks (PSBs) using selected indicators of financial soundness. The main indicator of distress is if the interest coverage ratio (ICR) drops below 1. As robustness checks, we use the return on assets (ROA) dropping below zero; the bank capital adequacy ratio (CAR) against a threshold related to the minimum prudential requirement banks want to keep; and non-zero emergency liquidity assistance (ELA) provided by the central bank to a commercial bank. For ELA, we are missing data because it is difficult to distinguish between regular and emergency liquidity transactions as reported in the banks’ public financial accounts.
The average annual probability of distress (PD) can be estimated as the average probability of distress using historical data on identified distress events:
1 * 1| ;0 ,
PD T N t 1 1 , , , ,i = ∑ D I I n N T in t i nt i { }∑ = ≥ = =
(2A.1)
where Di,n,t is the distress 0/1 dummy variable and i = [private sector banks; public sector banks].
Examining the Distress Factors
To assess whether certain bank characteristics could drive bank vulnerability to distress, we run a logit regression for Dn,t on bank characteristics (size; age; bank type, whether public sector or private); funding model of the bank (the loan-to-deposit ratio, net foreign exchange exposure); and macrofinancial shocks (commodity price shocks, portfolio capital flows). All are included in the vector of control variables, Xi,n,t , together with year fixed effects:
pDin t ( ) , , − pDin1 ( , ,t ) α ε X = + in t i, , nt, , . (2A.2)
We avoid including the indicators, In,t or their transformations that are used to identify distress: that is, Di,n,t. Including those would result in estimating a tautological relationship. By adding year fixed effects, we capture common time factors and any other relevant macroeconomic shocks. This approach also reduces the need to cluster errors.
By running the logit regression, we are most interested in uncovering whether stateowned commercial banks (SOCBs) are on average more prone to distress than privately owned banks—conditional on other factors, such as size, funding models, and governance indicators. Here, domestically owned private banks (PVTBs) serve as the control group.
82 H idden debt
Adjustments in Distress and Loss Given Distress
Let us define the loss given distress (LGD) as the monetary loss due to all forced adjustments that the PSB in distress must perform to survive, restructure, or close. Therefore, compared with the traditional expected loss formula, our LGD is equal to the loss given distress multiplied by the exposure in distress. The LGD is estimated relative to the control group of similar private banks.
The LGD can be estimated based on the monetary value of all the adjustments that happen when a distress event occurs (Dn,t = 1). For PSBs, we focus on the following adjustment, ADJ|Dn,t = 1, through five categories of adjustment j:
1. Percent change in capital 2. Percent change in provisioning 3. Percent change in debt 4. Percent change in lending 5. Percent change in fixed assets (including sale of fixed assets).
The LGD for an individual PSB can be estimated as follows:
| 1,
=(, ,) ( 1)LGD w in t j J=∑ ,, , , ADJ Dj i jn t i , nt = (2A.3) where ADJi,j,n,t is the monetary loss due to adjustment j of bank n of type i in distress at time t. Setting wj = 1 assumes that all adjustments in distress are equally important.
To estimate the adjustment size for each category j for distressed SOCBs relative to private banks, we run the following regression:
, , , , θ θ = + + + + ,
ADJ PSBjn t − −D D FE CE
PSB
n nt l n t l n t nt , (2A.4)
where θPSB is the parameter of interest. FE n are bank fixed effects. CEt are common time effects. We interact the distress dummy with the PSB dummy to estimate the difference in adjustment between distressed PSBs and PVTBs. The lag l takes values 0 and 1.
Estimating the impact of SOCB Distress on investments by Private Firms
Our outcome variables of interest are the economic impacts of SOCB distress on firm financing and private investment. The impact of SOCB distress can vary by the type of dominant adjustment that SOCBs undertake in distress—and the size of the adjustment.
We utilize a reduced-form framework for SOCB distress relative to private bank distress. We run the following regression:
FIN β γ ∈= + +D Xin,t i,n,t n,t n,t , (2A.5)
where FINn,t is firm lending (log-log growth in debt) and investment (log-log growth in fixed assets), respectively; and X are controls, including sector, year, and firm fixed effects. βi is our coefficient of interest, which is expected to be negative. That is, a firm linked to a bank that experiences distress will have greater problems in undertaking investment, other things remaining equal. If |βSOCB| > |βprivate|, distresses of SOCBs are more harmful than distresses of private banks. For instance, compared with private banks, SOCBs could be adjusting in times of distress mostly by reducing lending, while serving firms that do not have other banking options (links to private banks). If |βSOB| ≅ |βPrivate| and β is significantly negative overall, distresses of private and public banks are equally harmful. Note that here SOCBs could be still more problematic if they experience distress more frequently than private banks. If |βSOB| < |βPrivate|, distress of SOCBs could be less harmful: for example, because SOCBs can sustain lending and avoid closing branches even if in distress—including due to the soft budget constraints they could enjoy. Then, especially during systematic stresses— such as during economic recessions, nearfinancial crisis, or financial crisis episodes—SOCB presence in the banking system could support the resilience of lending through the cycle and private investments. But the cost of this resilient lending could be borne by higher taxes or more government debt.
The results of the regressions are presented in annexes 2B and 2C.