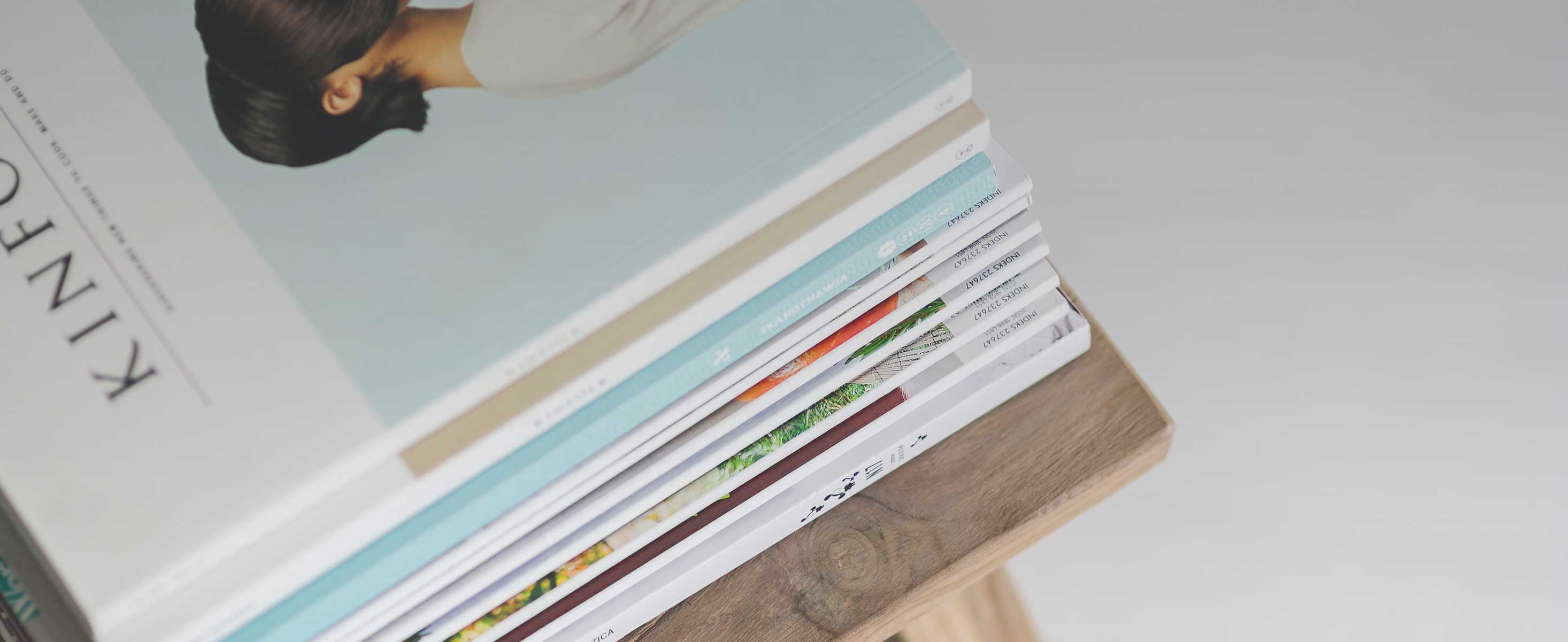
12 minute read
Pakistan, and Sri Lanka, 2005–17
112 H idden debt
FiGURE 3.12 Total Liabilities of Loss-Making State-Owned Enterprises in india, Pakistan, and Sri Lanka, 2005–17
20
18
Percent of GDP 16
12
8
4
0 12 12 14 15
1 2 2 2 2 5 4 4 4
3 3 5 4 5 5 3
2005 2006 2007 2008 2009 2010 2011 2012 2013 2014 2015 2016 2017
India CPSEs Pakistan Sri Lanka
Source: Melecky, Sharma, and Yang 2020 (see details in annex 3A). Note: CPSEs = central public sector enterprises.
Indian CPSEs are not engaged in inherently more risky activities than private firms.
Overall, the results in table 3B.3 show that CPSEs do not have significantly more volatile sales or profits than comparable non-SOEs.15 Adjusting for size is critical: even though the raw volatility of CPSE sales is significantly lower (column 1), this difference disappears when we include size as a control.
Although individual SOEs do not face more volatile conditions than individual nonSOEs, the SOE sector as a whole could be more volatile because the shocks hitting SOEs are more correlated. But this hypothesis also is not confirmed: The volatility of aggregate CPSE sales has been similar to the aggregate volatility of total sales of private firms in the Prowess database in recent years.
Why Do SOEs Underperform Comparable Private Firms?
Using the same basic regression specification, we next show that SOEs commercially underperform otherwise comparable private sector firms. We regress indicators of performance on the CPSE dummy and controls, such as size, age, and sector-year fixed effects. The results are shown in table 3B.4.
Indian CPSEs overemploy labor and capital. Controlling for size, age, and sector, the revenue-to-wage bill ratio for CPSEs is 85.8 log points lower and their revenue-to-fixedassets ratio is 21.5 log points lower (columns 2 and 4, respectively).16 Thus, CPSEs earn less per unit labor cost and per unit capital than comparable private firms. CPSEs also have a higher debt-to-asset ratio than comparable non-SOEs (column 6).
We further compare CPSEs with other firms in terms of revenue-based productivity measures: revenue total factor productivity (TFPR); and the marginal revenue products of capital (MRPK), labor (MRPL), and material inputs (MRPM). The estimates of TFPR, MRPK, MRPL, and MRPM are based on the procedure outlined in Asker et al. (2014), which is based on a model in which firms produce differentiated products using a simple (industry-specific), constant-return CobbDouglas production function and face a demand curve that is constantly elastic. The details of the estimation are presented in annex 3C. TFPR measures sales per unit inputs and should not be equated with physical total factor productivity (TFP). Differences in TFPR across firms could reflect distortions such as input adjustment costs, markups, and
sout H A si A’ s st A te - owned enter P rises 113
policy distortions. In a sense, TFPR is similar to the revenue-to-input measures examined in table 3B.4. The difference is that it adjusts for the usage of capital, labor, and raw material by employing industry-specific production function coefficients.17
Indian CPSEs are not concentrated in sectors in which profit margins are lower.
Table 3B.5 compares the revenue productivity across CPSEs and other firms.18 The regressions follow the same specifications as those employed in tables 3B.3 and 3B.4. CPSEs have a significantly higher TFPR than nonSOEs in the absence of other firm-level controls (column 1). However, this gap becomes statistically insignificant upon introducing controls for size (column 2). Controlling for firm size makes a difference because larger firms have significantly higher TFPR on average, perhaps reflecting higher price markups due to their greater market power. This suggests that CPSEs do not have lower TFPR than comparably large private firms.
Next, we consider the marginal revenue products of labor and capital. CPSEs have significantly lower MRPK and MRPL than nonSOEs (columns 4–8). The gap in MRPL is particularly high (about 83 log points). These results suggest that CPSEs use too much capital and labor—especially the latter—that could be released and efficiently reallocated to private firms with higher marginal returns and capital and labor.
Finally, we observe that CPSEs have a significantly higher marginal revenue product of intermediate inputs (columns 7 and 8). In a sense, CPSEs compensate for the overuse of labor by “underusing” other inputs. For example, SOEs could be using more manual processes, which consume less power.
It has long been argued that SOEs underperform due to internal management problems. It is harder to align the incentives of management and owners in the public sector (see, for example, Ehrlich et al. 1994). The compensation of managers tends to be less strongly linked to the firm’s market performance in SOEs (Borisova, Salas, and Zagorchev 2019). A competing hypothesis is that SOEs managers are prevented from making optimal choices because too many external constraints and conflicting objectives are imposed on them, such as a government mandate leading to excessive hiring (Shleifer and Vishny 1994). The results in tables 3B.4 and 3B.5 are more consistent with the latter theory. In particular, they would seem to favor the hypothesis that SOEs are constrained from adjusting labor use. While internal managerial problems can also lead to inefficient input choices, they are unlikely to cause a systematic and persistent overemployment of an input.
This interpretation is also consistent with recent evidence from a natural experiment involving SOE privatization in India (Baird et al. 2019). This study finds that SOE privatization improved the allocative efficiency of labor across firms by reducing the overemployment of labor in the public sector and reallocating it to private firms with higher marginal returns to labor. Finally, given that we are measuring revenue productivity and not physical productivity, the results could also reflect mandates that prevent SOEs from adjusting prices in response to changes in input costs (Khanna 2012). Some (implicit) mandates could require the SOE to act as an insurance mechanism for volatile prices.
Based on the idea that corporate governance reforms could improve SOE performance by enabling better monitoring, greater operational autonomy, and reduced political interference, “corporatization” and corporate governance reforms have taken root in South Asian countries. For example, the Indian government has updated corporate governance guidelines for CPSEs and rates CPSEs on compliance with the guidelines (Government of India 2010). The guidelines relate to the quality and independence of the board of directors, audits, accounting standards, disclosures, and risk management.
To examine whether CPSEs with better corporate governance perform better, we merged data on annual corporate
114 H idden debt
governance (CG) ratings of CPSEs into the Prowess panel. The data are limited because not all CPSEs are rated, but there is some variation in the ratings across CPSEs and over time.19 This variation allows us to examine the association between the ratings and performance within the subset of CPSEs that have been rated.
Improvements in corporate governance must be complemented by broader reforms in the governing environment around SOEs.
The results indicate that a higher corporate governance rating is associated with better performance in the cross-section (table 3B.6). Controlling for sector-year effects, a onepoint improvement in the rating (which ranges between 1 and 5) is associated with a 31.4-log point higher revenue-to-wage-bill ratio (column 1). It is also associated with a 5.6-percentage point reduction in the probability of distress (ICR less than 1) (column 7) and a 0.07-point lower debt-to-asset ratio (column 5). However, these correlations are not significant when controlling for firm fixed effects. Because it is possible that the corporate governance rating of CPSEs is correlated with unobserved factors affecting firm performance, the sensitivity of the results to firm fixed effects makes it hard to claim that this regression measures the impact of improved corporate governance. The weak correlation suggests that corporatization alone would not solve the problems of SOE underperformance and financial distress.
The results call for more serious consideration of such reforms as part of package of SOE reforms. The evidence base on corporatization is limited and suggests that corporatization needs to be complemented by even broader reforms of SOE governance and related political economy issues to be effective. Berkowitz, Ma, and Nishioka (2017) argue that part of the positive impact of SOE corporatization in China was due to external changes, such as reduced hiring pressures on SOEs. Corporatization does not necessarily solve the problem of SOEs being captured by politicians or other insiders (Shleifer and Vishny 1994; Qian 1996). For example, there is evidence that the Chinese Communist Party still maintains a high degree of control over corporate SOE boards (Fan, Morck, and Yeung 2011).
Are Soft Loans and Soft Budgets the Root of Contingent Liabilities?
Loans can be used to acquire productive assets, pay for working capital, or cover the occasional loss. The latter course of action is not sustainable if financial markets are competitive and a firm continues to stack up losses. To explore this mechanism, we examine the correlation between the debt-to-asset ratio and accumulated losses in the Prowess panel.
Higher cumulative losses are associated with more indebtedness. Controlling for firm fixed effects and sector-year fixed effects, the estimated β in the specification in table 3B.7 implies that a Rs 1 billion higher accumulated loss is associated with a 0.006 points higher debt-to-asset ratio (column 2). To put these numbers in perspective, the standard deviation of cumulative loss in 2016 is approximately Rs 40 billion. The estimate thus implies that increasing cumulative loss by 1 standard deviation increases the debt-to-asset ratio by 0.24 points. The median debt-toasset ratio was 0.36. Importantly, this association is significantly stronger among CPSEs. The estimated value of δ (column 4) implies that the additional effect among CPSEs is 0.004 points. This suggests that CPSEs are more likely than other firms to use debt to cover losses.
We explore the soft loan hypothesis—and more broadly, the soft budget constraint hypotheses—further by examining how the financial adjustment to distress and shocks differ among CPSEs and non-SOEs.
Soft loans and implicit guarantees distort the incentives of SOEs to monitor debt levels
and act early to improve performance. The results are presented in table 3B.8. Consider first what happens to the average firm on becoming financially distressed (denoted “Enter distress” in the table) (columns 1, 3, and 5). On average, firms that enter distress have a significantly lower growth rate of
sout H A si A’ s st A te - owned enter P rises 115
paid-in capital (equity) and assets (columns 1 and 5). This makes intuitive sense: firms in distress are essentially drawing down their assets. The relationship between distress and the growth rate of debt is positive, but the level of statistical significance is marginal (column 3). Further, this association is not robust to additional firm controls.
The coefficient on the interaction of distress with the CPSE dummy is statistically significant and positive in the case of fixed assets, implying that distress does not hamper CPSEs from acquiring fixed assets to the same extent as it does to non-SOEs. This result is consistent with the soft budget constraint hypothesis. SOEs could also be required to expand they investment to stimulate the economy as the condition of recapitalization or other bailouts—as is the case for the stateowned banks (see chapter 2).
The interaction of distress with the CPSE dummy is statistically insignificant in the case of paid-in capital or debt. This could be because CPSEs adjust through other unobserved channels, such as grants. Another possibility is that our distress measure is too permissive to be able to detect adjustment: as shown earlier, even 20 percent to 30 percent of non-SOEs have an ICR of less than 1 in any given year.
To address these issues, we explore more stringent measures of distress by using large shocks to earnings. We first identify the 10th and 90th percentile of revenue growth rates for every industry in the Prowess panel data. A firm that experiences a revenue growth rate above the 90th percentile of its historical industry norm is classified as experiencing a positive “shock,” while a firm that experiences a revenue growth rate below the 10th percentile of its industry norm is classified as experiencing a negative “shock.” Our assumption is that these unusual shocks to revenue reflect external market demand factors.20 Results using similarly defined profit shocks are similar.
Table 3B.9 shows that the raw incidence of negative shocks—that is, not controlling for factors such as firm size—does not vary significantly across CPSEs and non-SOEs (columns 1 and 4). This result is reassuring because in order to identify the differential adjustment of CPSEs to shocks, we ideally need shocks that are symmetric across CPSEs and other firms. However, controlling for firm-level size, CPSEs have a higher probability of being in a negative shock than other firms (columns 2 and 5). More broadly, the statistically significant coefficients on firmlevel variables like CPSE status, size, and age indicate that the shocks as measured are not entirely external to the firm (columns 3 and 6).
The results on the differential adjustment to shocks are presented in table 3B.10. First, considering the regressions without CPSE and shock term interactions (columns 1, 3, and 5), the baseline pattern of adjustment to a shock makes intuitive sense. On average, firms facing a negative (positive) shock have significantly lower (higher) growth rates of debt, paid-in capital, and fixed assets. The most likely explanation is that the availability of funds to finance asset growth is sensitive to revenue shocks because banks and investors see revenue shocks as indicative of repayment capacity.
Next, we observe that the coefficients on the interaction of the CPSE dummy with shocks have consistently a reverse sign to the corresponding baseline shock coefficients (columns 2, 4, and 6) and, in most cases, are statistically significant. This suggests that access to financing—whether through equity or debt—is significantly less sensitive to revenue shocks for CPSEs than for the average firm and that CPSEs do not need to adjust to shocks through assets to the same extent as other firms. Another interpretation is that private firms infer a negative shock as a signal of lower future returns and slow down their borrowing and investment, but SOEs do not behave similarly—suggesting that the latter’s decision making is less sensitive to market signals. Overall, the results are consistent with the soft budget constraint hypothesis, which states that the incentives of SOEs to monitor debt levels regularly and act early to improve performance are distorted.