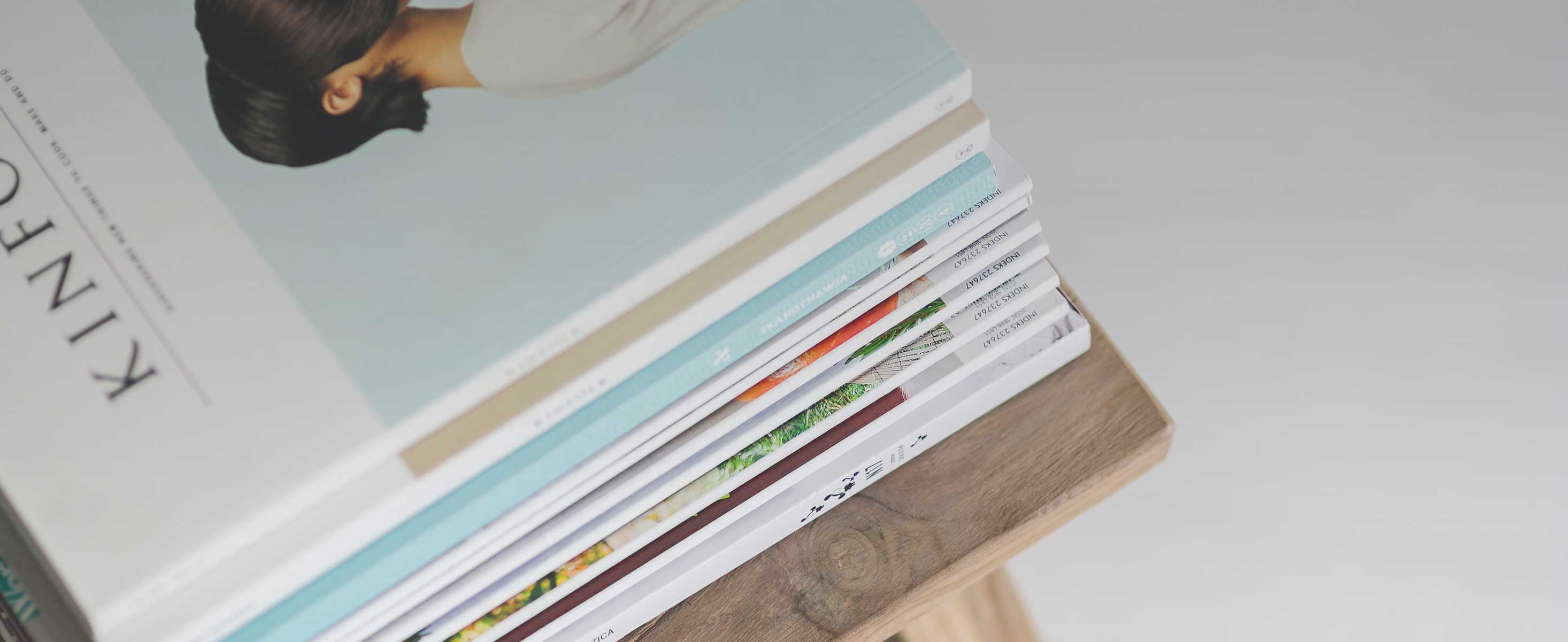
5 minute read
Notes
Since the output and input variables used in the production function are based on monetary values (deflated at the industry-year level), the resulting estimated measures of efficiency are revenue based (Klette and Griliches 1996).
Observing output prices allows us to compute physical quantity produced by the firm and hence isolate demand factors from revenue-based productivity (Foster, Haltiwanger, and Syverson 2008). TFPQit is the Hicks-neutral measure of physical total factor productivity we are interested in estimating. Using the method in Foster, Haltiwanger, and Syverson (2008), a simple measure of technical efficiency is constructed: tfpqit = tfprit /lnpit. Although firm-level prices are observed and output is in physical quantity, capital and material inputs are still based on expenditure deflated with an industry-specific price index. This implies that for any deviation from a perfectly competitive input market, capital and material inputs would also include unobserved idiosyncratic input price variation. To correct for the “input price” bias arising from the correlation between input prices and quantities, Grover and Maloney (2021) rely on Blum et al. (2018), which extends the Gandhi, Navarro, and Rivers (2020) method to recover estimates of markups using output price data. Following De Loecker et al. (2016), this methodology is extended to address the input prices bias stemming from unobserved firm-level input prices, henceforth TFPQit, along the lines of De Loecker et al. (2016).
After estimating the production function parameters, firm-level markups (μit) can be recovered from marginal costs (mcit) and output prices. Specifically, markups are calculated based on the expression derived from the first order condition of the firm’s cost minimization of the flexible material inputs:
µ = α − mln it it ln −εSit it (2A.2)
where αit m is the output elasticity of materials estimated from the production function; Sit is the share of material inputs expenditure (Mit) over total sales (Rit); and εit is the ex post shocks to the estimated production function. As markups are the wedge between prices and marginal costs, mcit = lnPit − μit.
Notes
1. See https://www.worldbank.org/en/topic/urbandevelopment/overview#1. 2. See Chauvin et al. (2017) for the United States, China, and India; Combes, Duranton, and
Gobillon (2008) for France; De la Roca and Puga (2017) for Spain; and Henderson, Nigmatulina, and Kriticos (2019) for Africa. 3. They use building heights to develop a measure of density adjusted for floor area and show that by not taking into account the quality of built structures, naive measures of population density end up conflating crowding and livable densities.
4. In contrast, in Brazil, India, and Malaysia, shares of urban farmers are all less than 7.5 percent (Henderson and Kriticos 2017; Henderson and Turner 2020). In many African countries, this share has been rising rather than falling (Hommann and Lall 2019). 5. Some studies, however, find little correlation with resource sectors and argue that it is the unusually low agricultural productivity and poor service provision that propels migrants to the cities (see Henderson and Turner [2020] and chapter 3 on migration and labor mobility in this volume). 6. For a survey of the overall channels of sharing, matching, and learning effects, see Combes and
Gobillon (2015). 7. These worker and firm quality effects can be identified econometrically by controlling for human capital explicitly and controlling for firm-specific fixed effects. 8. See Audretsch and Feldman (1996), Buzard and Carlino (2013), and Buzard et al. (2016) for evidence on the United States, and Carrincazeaux, Lung, and Rallet (2001) for evidence on France. 9. See Rosenthal and Strange (2008); Carlino and Kerr (2015); Ellison, Glaeser, and Kerr (2010); and
Kerr and Kominers (2015) for evidence on the United States, and Capello and Lenzi (2014) for the European Union. 10. For reviews, see Rosenthal and Strange (2004) and Melo, Graham, and Noland (2009). 11. For a complete discussion, see an earlier volume in the Productivity Project series, Productivity
Revisited (Cusolito and Maloney 2018). 12. TFPR is computed using a Hicks-neutral total factor revenue productivity after estimating a gross-output production function, following the nonparametric estimation strategy of Gandhi,
Navarro, and Rivers (2020). While both wages and TFPR estimates are interpreted as a productivity premium of density, their magnitudes are not directly comparable. In wage equations, all the effects should be rescaled by the share of labor in the production function (Combes et al. 2010).
One key reason for this difference is the presence of urban costs, which are expected to increase nominal wages through local input markets, housing costs, and urban disamenities, and thus yield larger estimated wage elasticities. 13. This result is in line with that of Handbury and Weinstein (2015), who use detailed barcode data and find a positive elasticity of price with respect to population. 14. The elasticity estimates of urban disamenities in this chapter is somewhat higher than those of
Ahlfeldt and Pietrostefani (2019), who find a pollution elasticity of +22 percent, using ordinary least squares estimation with OECD data. They also find a congestion elasticity of +8 percent using a different measure of congestion and average travel speeds. Their meta-analysis of 13 studies estimating elasticities of crime (not focusing on homicides, as in Grover, Lall, and Timmis [2021]) in high-income countries has a mean elasticity of −24 percent. 15. Prices in Grover and Maloney (2021) are computed following standard literature: De Loecker,
Fuss, and Van Biesebroeck (2014); Dhyne et al. (2017); Amiti, Itskhoki, and Konings (2019); and Lenzu, Rivers, and Tielens (2019). Nonetheless, quality effects may be underestimated. As a result, the prices may overestimate the quality-adjusted prices in dense locations. For Colombia, the authors use alternative indexes to control for quality, following the approach in Eslava and
Haltiwanger (2021). The quality-adjusted prices are positively correlated with density, implying that TFPQ is highly negative and significant in cities. 16. For more details on this issue, see Mendershausen (1938); Marschak and Andrews (1944); Bond and Söderbom (2005); Ackerberg, Caves, and Frazer (2015), and a formal discussion by Gandhi,
Navarro, and Rivers (2020). This approach has two key advantages compared with standard proxy variable methods. First, it solves for identification issues when the production function contains at least one flexible input, such as materials. Second, it imposes no restrictions on the elasticity of substitution between inputs by modelling the production function nonparametrically.