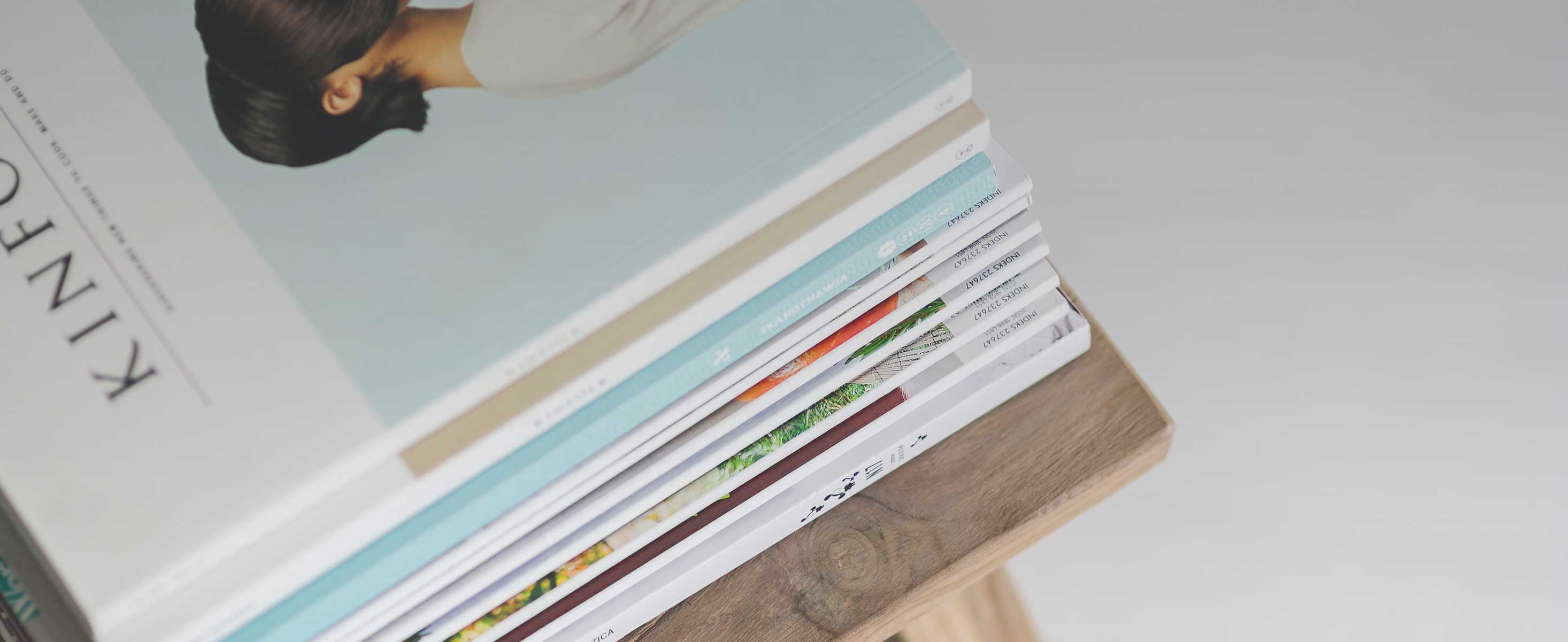
10 minute read
S.1 Examples of More Careful Price Measurement for Services
TABLE S.1 Examples of More Careful Price Measurement for Services Subsector Least preferred price deflator Example of more firmspecific price deflator Example of adjustment for quality
Restaurant PPI or CPI Price of a meal
Incorporating the quality of the meal Hotel PPI or CPI Price of a room Incorporating the comfort and amenities of the room Management consultancy PPI or CPI Hourly fee of consultant Incorporating contractspecific terms
Advertising PPI or CPI Price of a printed ad or of 1 minute of television advertising Accounting for the number of viewers seeing the advertisement
Source: Elaboration from Eurostat 2016. Note: CPI = consumer price index; PPI = producer price index.
health services). These services, by definition, do not carry a market price and are usually excluded from firm-level analyses.
Financial services. A second group comprises financial services, whose sales or output can be difficult to define. Customer fees alone do not capture a bank’s full income from borrowers or account holders, especially given that many financial service providers charge low fees or none at all. To get around this issue, national accounts statistics attempt to measure output by looking at spreads between interest rates that banks face (measured by reference rates) and that banks provide to deposit account holders or charge to their borrowers.4 Constructing such measures gets especially complicated with more-complex financial products involving different currencies or risk profiles. Because of the complications around measurement, financial sector firms are usually excluded from firm-level data analysis or treated separately.
Services delivered for free. Radio, television, and digital services that are delivered for free pose additional challenges for measuring output. Advertising revenue could provide some indication of value, but this value does not always reflect the full value that consumers obtain from these services. This underestimation of the value of free services is particularly relevant for many digital services, as discussed more extensively later in this Spotlight.
Measuring Inputs
Quantifying inputs such as labor, capital, and other intermediate goods and services can similarly be more challenging for services than for manufacturing.
Labor. First, the quality of labor is hard to measure. As chapter 1 highlighted, services subsectors vary greatly in their use of skilled labor. Even though productivity measures rarely adjust for the skill content of labor, a differentiation between unskilled and skilled labor can be useful to better understand variations in productivity across sectors.
The question is how to measure the skill content of labor. One approach is to use wages as a measure of quality-adjusted labor input, but wages often reflect not only the contribution of labor to production but also the competitiveness of the labor market. Alternative measures of human capital—such as educational attainment or work experience—are often not available and require datasets covering both employees and employers. One such dataset is the matched employer-employee dataset (the Annual Social Information Report, RAIS) in Brazil.
Capital. Second, many services tend to rely more on intangible forms of capital than on physical capital, and these are harder to measure. Intangible capital covers a wide range of assets, such as software, research and development (R&D), organizational culture, brand value, and relationships with clients and suppliers.
The value of these assets is often difficult to measure, especially when they are developed in house rather than purchased externally. Brynjolfsson, Rock, and Syverson (2021) argue that unmeasured intangible capital can lead to underestimations of productivity at early stages of a firm’s life cycle (because physical capital and labor are used to produce unmeasured intangible capital that provides few returns to begin with) but can lead to overestimations of productivity later (when the intangible capital has generated benefits as a productive, albeit unmeasured, input).
Nevertheless, some progress has been made to improve the measurement of some forms of intangible capital, especially of information and communication technology (ICT) capital. Unfortunately, such measurements often tend to be available only in high-income countries. In some cases, indirect measures of intangible capital could be used, such as the market valuation of a firm (as discussed, for example, in Hall 2001).
Intermediate inputs. Third, services often rely on other services as intermediate inputs, meaning that all the issues related to measuring the output of services— discussed in the previous section—will also affect the measurement of inputs. Griliches (1994) argued that productivity measurements of the IT sector in the United States should be interpreted with care because, among other reasons, many of the intermediate inputs were not appropriately deflated. A better measurement of services outputs will therefore also improve the measurement of the inputs in this sector.
Transaction-level data—for example, from tax records or digitally collected through electronic fiscal devices (EFDs) or electronic invoices (EIs)—can especially help to disentangle links between firms. Such datasets are now increasingly available and are used by researchers to better understand how linkages matter for firm performance, including those between manufacturing and services firms (Avdiu et al. 2021).5
In addition, many of the challenges present in manufacturing—such as difficulties in constructing measures of capital stock (even though services tend to use less physical capital) or determining the factor elasticities of inputs—are likely also present for services. Factor markets are also rarely fully competitive, meaning that value-based
approaches to measuring inputs (as used for measuring capital, for example) are prone to capture not only quality but also market power.
Subsectors that are particularly challenging for measuring production inputs are those involved in the leasing or outsourcing of these inputs—for example, employment outsourcing firms, real estate rental agencies, and machine leasing firms. In most datasets, the inputs would appear on the balance sheet of the outsourcing or leasing firm, but the production benefits are realized in the host firm where production takes place. This could lead to an underestimation of these inputs for the host firm (since they are not counted as being part of the host firm) and therefore an overestimation of productivity. For the outsourcing firm, productivity is likely underestimated, since output is determined by the fees paid by the host firm and not the actual production that the workers or capital are contributing to.
Estimating Productivity
These challenges of measuring outputs and inputs also affect the estimation of productivity since productivity, in its essence, is a ratio between outputs and inputs. The potential for mismeasurement has long worried economists regarding whether productivity in the services sector, particularly in digitally enabled services, had been underestimated.
One of the big productivity puzzles of recent years is understanding the marked slowdown in measured productivity since 2000, all at a time when digital technologies have made tremendous gains. The advances in the data economy appear everywhere but in productivity data (see, for example, Brynjolfsson and MacAfee 2014; Feldstein 2017). Even though the extent to which mismeasurement fully explains the observed lower productivity growth rates has been questioned (for example, in Syverson 2017), mismeasurement remains a concern and highlights the need for better data collection.
Metrics of Productivity
In its simplest form, productivity can be measured as labor productivity by calculating the ratio of outputs or value added to employment (for example, value added per worker). This approach has the advantage that it can often be easily calculated using readily available data and can also be easily compared between sectors and countries (even as this comparison can be misleading for all the reasons discussed here).
Value added is often preferable to revenue as a measure of output, especially for sectors relying significantly on physical inputs, such as the retail and food sectors. The disadvantage of this measure is that it does not correct for the use of capital: capital-intensive firms will appear to be productive even if they do not use their capital productively. Even though services firms tend to be less intensive in physical capital than manufacturing, there are some exceptions (such as air transportation, water
transportation, and telecommunications), meaning that labor productivity comparisons with these sectors can potentially be misleading. Labor productivity also does not correct for the use of intangible capital, which can be highly important as well.
Total factor productivity (TFP) gives a more complete account of the technical efficiency of how capital, labor, and intermediate inputs are jointly used in the production process and therefore is often a preferred metric. The challenge is that TFP is not directly observed but instead is estimated using either a production function or a cost function approach. This introduces a further need for assumptions, including on how inputs are combined in the production process. In addition, since TFP corrects for capital use, any mismeasurement of capital will affect the estimated productivity level. The likely underreporting of intangible capital in services provision will therefore also affect TFP estimations.
Separating Prices and Quantities
The importance of separating prices and quantities was already highlighted in the earlier section on measuring outputs, but for productivity analyses that compare firms, this is especially important. Prices can reflect both market power and quality, meaning that value-based productivity measures become intertwined with both (see, for example, Cusolito and Maloney 2018; De Loecker, Eeckhout, and Unger 2020; Hsieh and Klenow 2009). Firms that appear to be productive may in fact simply face limited competition. Higher prices can reflect higher quality, but they do not necessarily. With technological innovations, it may be possible to lower prices while producing outputs at the same or even higher quality. The larger heterogeneity in quality as well as potential competition concerns in certain sectors mean that the scope is larger for market power and quality differences to play a role.
For most analyses, the preference is therefore to use quantity-based measures of productivity (such as quantity TFP, abbreviated TFPQ) instead of value-based measures of productivity (such as revenue TFP, abbreviated TFPR) and ideally to also control for quality differences. A variety of methods have been adopted to correct the influence of prices in productivity measurements by applying some correction derived from economic theory. This usually requires making assumptions, such as what demand looks like (for example, Hsieh and Klenow 2009) or that firms are choosing inputs such that costs are minimized (for example, De Loecker and Warzynski 2012). The appropriateness of such corrections is still a subject of debate, but no standard approach has yet emerged (Syverson 2020).
Estimation Challenges: Simultaneity, Selection, and Heterogeneity
In addition to the issues involved in measuring outputs and inputs and the difficulties in incorporating market power and quality, there are other challenges in estimating TFP that may be more problematic for services than for manufacturing. There
are the usual concerns about the simultaneity bias between productivity and input choices, as well as selection biases that can make it difficult to interpret econometric estimates. The heterogeneity of the services sector might also be greater.
Simultaneity bias. The simultaneity bias relates to how to disentangle whether it is that the increased use of inputs raises outputs or that managers have firmspecific knowledge about their potential to raise output or prices that leads them to increase their inputs. With services relying less on fixed inputs that cannot be increased or decreased easily (for example, capital) and more on variable inputs that can be adjusted in a shorter amount of time (for example, labor), the simultaneity bias is likely different for services firms than for manufacturing firms.
Selection bias. The selection bias relates to firm exit often being correlated with productivity, with less-productive firms being more likely to exit than more-productive firms, such that productivity measures appear to be higher. Given that rates of firm churn are much higher in services, this is a relatively bigger concern for estimating TFP in services than in manufacturing.
Observed heterogeneity. Another concern is the assumption of a common production function across firms in a sector. While not unique to services, the greater observed heterogeneity in value added per worker in services is consistent with quality differences being more varied. If inputs and outputs cannot be adjusted well for quality, assuming a common production function may lead to misleading results.
Corrections can be applied to reduce the influence of some of these biases. For example, common methods to correct for simultaneity bias and selection bias include those by Ackerberg, Caves, and Frazer (2015), Levinsohn and Petrin (2003), and Olley and Pakes (1996). Nevertheless, some of the wider challenges around market power and quality will be harder to address, unless better and more detailed data are available.
Measuring Trade
Many of these challenges related to measuring outputs and inputs also apply to measuring trade in services. Although goods trade can be measured by tracking customs records, many services—unless they are either embodied in goods (such as film on a Blu-ray disc or software on a DVD)6 or result in the movement of goods (such as transportation services)—will not be passing through customs. In some cases, it is not the service itself that crosses a border but rather either the provider (such as through temporary migration or setting up a subsidiary firm) or the consumer (as through tourism). Capturing each form of services trade might require different measurement approaches.