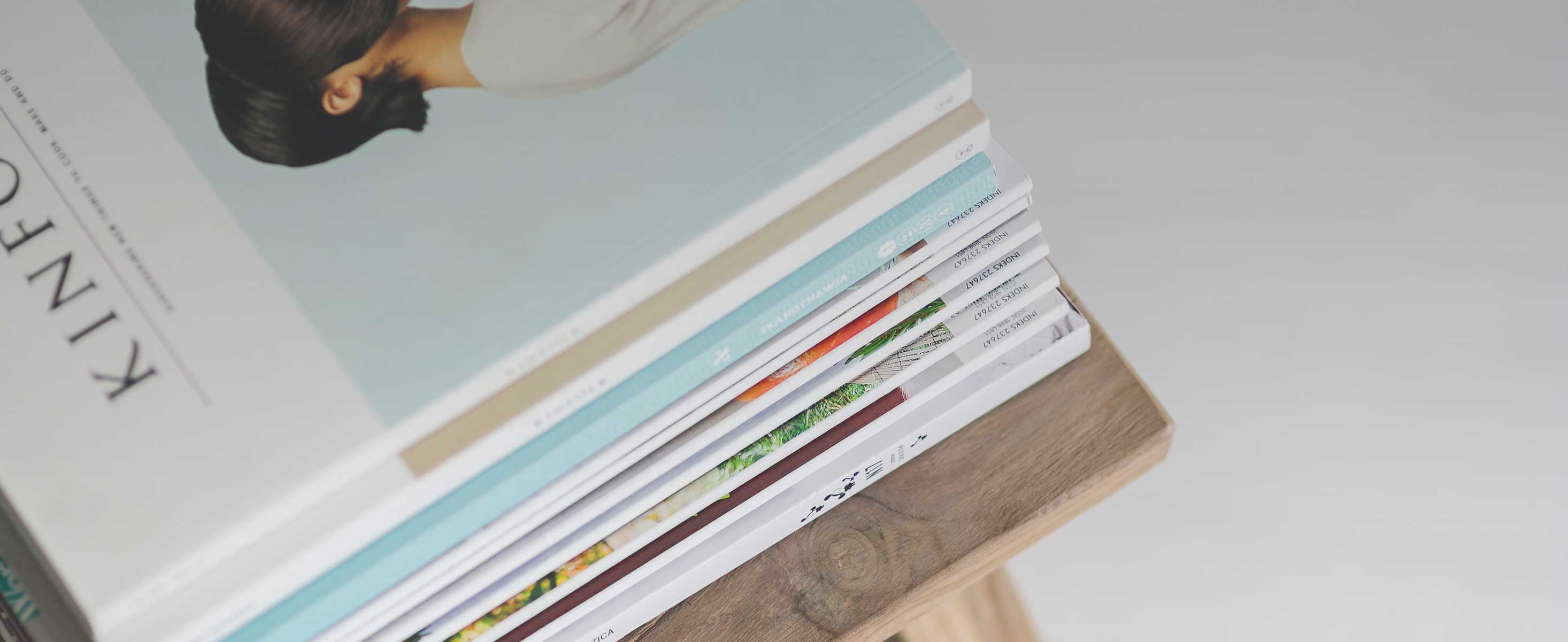
4 minute read
to Paint a COVID-19 Picture
BOX 1.1
Tunisia: Using Phone Surveys and Microsimulations to Paint a COVID-19 Picture
Phone surveys present real-time evidence from the ground (such as income and living standards) while microsimulations try to quantify the overall expected effects for the economy (such as poverty and welfare). How the two approaches corroborate each other can be illustrated with the case of Tunisia.
As the pandemic unfolded, five waves of phone surveys were conducted. The selfreported results indicate that about half of the households saw living standards deteriorate compared with the pre-COVID-19 period, particularly among the poor and the bottom 40 percent. Those hardest hit include informal workers—especially in the private sector or self-employment—in construction, manufacturing, accommodation and food services activities, and transport. The surveys also show that the deterioration in welfare was caused by job and income loss along with higher food prices.
These findings are corroborated by the microsimulations. Using pre-COVID-19 administrative data, the first exercise simulates the impact on consumption, poverty, and inequality using labor income and consumption. The second exercise simulates price effects to determine the change in disposable income. The third exercise identifies high-risk sectors (tourism, textiles, mechanical and electrical industry, transport, commerce, and construction), which are also the industries where a large number of poor and vulnerable are likely to be employed.
The microsimulations project an increase in poverty ranging from 7.3 percentage points (a more than 50 percent rise) in the optimistic scenario to 11.9 percentage points (an almost doubling) in the pessimistic scenario. They also add value by estimating the degree to which government compensatory measures can mitigate some of the losses: poverty would increase an estimated 6.5 percentage points in the optimistic scenario with mitigation measures as opposed to 7.3 percentage points without it.
Put together, the two methodological approaches not only support each other’s findings but also indicate trends or furnish estimates such that they build on each other to provide a more robust picture. In Tunisia’s case, these combined results would give policy makers a better idea of which segments of the population need to be targeted (and in which sectors), along with the potential effects from mitigation measures and policies.
Lessons from this exercise also highlight the crucial role that administrative data can play for such analysis and estimates. Administrative records are less likely to be susceptible to biases relative to specially administered surveys. Moreover, the former may be collected as part of an actual state support program or exercise and be more accurate,
continuous, low-cost, and granular in nature. On the other hand, one-off surveys are very helpful tracking tools in such crisis situations and provide glimpses of the socioeconomic situations of households, although they may lack the consistency of administrative data. The upshot of this data exercise is that policy makers need a combination of different tools and data, administrative records, more frequent national surveys (like labor force surveys), and even infrequent phone surveys to track the wellbeing of households.
This report is divided into two parts, which differ by analytical approach: Part I is based on direct observation of the impact on welfare through evidence from phone surveys. Chapter 2 assesses the unequal impact that COVID-19 has had on households across multiple countries and economies in MENA, with a focus on jobs, earnings, health care, social protection, and welfare distribution. Chapter 3 examines the effect that the pandemic has had on the welfare of the population of the West Bank and Gaza, especially jobs, income, and food insecurity. Chapter 4 examines the decline in living standards of Tunisians during the pandemic, which continued even after the lockdown measures had been lifted. And chapter 5 explores the trends of recovery in Djibouti households since the onset of the pandemic—including a subsample of refugee households, which includes refugees and asylum seekers from other countries.
Part II builds on various welfare simulations. Chapter 6 continues the analysis of Tunisia, using phone surveys and microsimulations, to probe the anticipated impact on poverty and value of government mitigation measures. Chapter 7, which focuses on the West Bank and Gaza, explores the impact on poverty and inequality, with microsimulations that rely on microdata (including phone surveys). Chapter 8, which focuses on the Islamic Republic of Iran, assesses the distributional impact of COVID-19-induced inflation and loss of income on welfare and poverty, along with the value of government compensatory measures; but in the absence of phone surveys, it relies on household survey data from before the pandemic. And chapter 9 looks at the impact of the pandemic on poverty for Syrian refugees and the host community in Lebanon, with simulation tools that use pre-COVID-19 survey data and household income and consumption data.
The report’s assessments on changes in employment, income, consumption, living standards, and inequality should help guide policy makers and other stakeholders to design policies to minimize escalations in poverty and to provide income and social support to those worst hit, while exercising fiscal prudence.