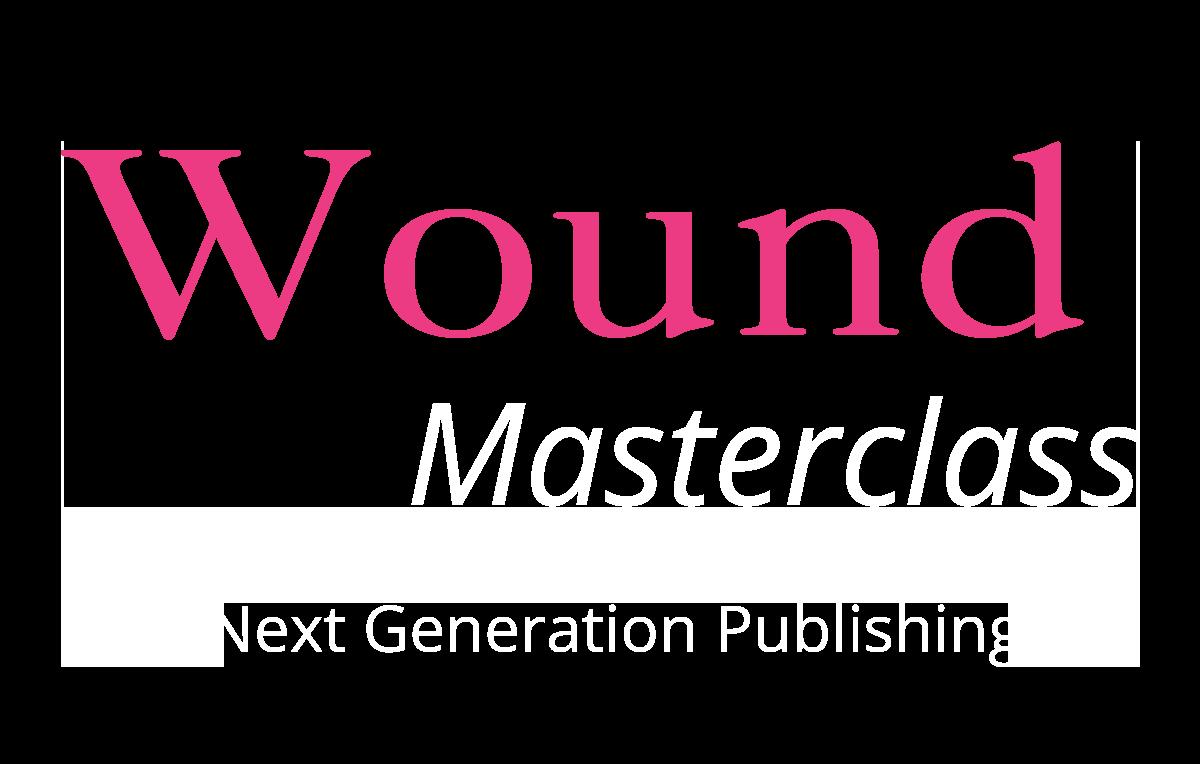
1 minute read
Limitations of Applying Summary Results of Clinical Trials to Individual Patients: The Need for Risk Stratification
and 10 new nosocomial pressure ulcers were counted. In effect, there are two processes; the case where there is no new pressure ulcer, where the value is always 0, and the case where there are new nosocomial pressure ulcers, and the count is greater than 0.
In the second case, let’s say that we have a retrospective observational study of 1,000 pressure ulcers from 700 patients where we are looking at TTH in days. As we examine the data, we notice that 20% of the data is zero; a skeptic might ask how it is possible for a wound to occur and heal all on the same day? That would seem highly unlikely, and would make one wonder about the data generating process; there might be systemic left-censoring occurring as a result of the TTH being computed from the start of the study window, rather than the date of injury; not only would this result in zeroinflation, but systemically would pull the data leftwards, resulting in an undercounting of the actual TTH of all wounds.
Zero-inflated data is best modelled using a class of tools called mixture models. These models are able to model the parameters of both processes and result in good approximations of the data. In the case of systemic left-censoring, as described in the second case of zero-inflation above, Bayesia mixture models can estimate the true length of TTH of the wounds by systematically adjusting TTH upwards based on the model and observed data, and pooling of information across wounds.
Conclusion
Acknowledgement of the pathologies of wound care data allows us to identify ways that we can deal with them in a principled and ethical manner. One of the limiting factors in contemporary wound care research is the elidation and ignorance of the pathologies with wound care data; this leads to the use of statistical tools that cannot deal with the nature of the data. This is unfortunate, as we are in a golden age of statistical flexibility, statistical software, and computational power. The combination of these 3 factors allows us to deal with many of these pathologies, and arrive at better insights that allow clinicians to deliver better care, decrease the cost of care to payers, drive innovation in wound care modalities, and drive forward the field of wound care.
References
1. Colchero F, Clark JS. Bayesian inference on age-specific survival for censored and truncated data. J Anim Ecol. 2012 Jan;81(1):139-49. doi: 10.1111/j.1365-2656.2011.01898.x. Epub 2011 Aug 26. PMID: 21883202
2. Chapter 4. Measurement error and bias. Epidemiology for the uninitiated. [Internet]. Available from: https://www.bmj.com/about-bmj/resources-readers/publications/epidemiologyuninitiated/4-measurement-error-and-bias. Accessed 11/04/2023
3. Haas, M., Pigorsch, C. (2009). Financial Economics, Fat-Tailed Distributions. In: Meyers, R. (eds) Encyclopedia of Complexity and Systems Science. Springer, New York, NY. https://doi. org/10.1007/978-0-387-30440-3_204
March - April 2023