Co-DesignwithMyself:ABrain-ComputerInterfaceDesignToolthatPredicts LiveEmotiontoEnhanceMetacognitiveMonitoringofDesigners
ANONYMOUSAUTHOR(S)
Fig.1.ConceptDiagramofCo-designwithMyselfUsingThe“Multi-Self”DesignTool.
Modulatingthefeelingsofconfidencetowarddesignoptionsandmetacognitivemonitoringareimportantcomponentsofdesign intuition.Wefindnotmanycreativitysupporttoolsexploredthepotentialofbiofeedbacktoaiddesignprocessesinthisregard.In thecurrentstudy,wepresent“Multi-Self,”aBCI-VRdesigntoolthataimstoenhancemetacognitivemonitoringduringarchitectural design.Itevaluatesdesigners’ownvalenceandarousaltotheirdesignworkandpresentsdesignerswiththeirbiofeedbackvisuallyin realtime.Apilotproof-of-conceptstudywith24participantswasconductedtoevaluatethefeasibilityofthetool.Interviewresponses regardingtheaccuracyofthefeedbackweremixed.Themajorityoftheparticipantsfoundthefeedbackmechanismusefulandthetool elicitedmetacognitivefeelingsandstimulatedexplorationsofthedesignspacewhilemodulatingsubjectivefeelingsofuncertainty.
CCSConcepts: • Human-centeredcomputing → Systemsandtoolsforinteractiondesign
AdditionalKeyWordsandPhrases:CreativitySupportTool,Brain-ComputerInterface,AffectiveComputing,Biofeedback,Metacognition
ACMReferenceFormat:
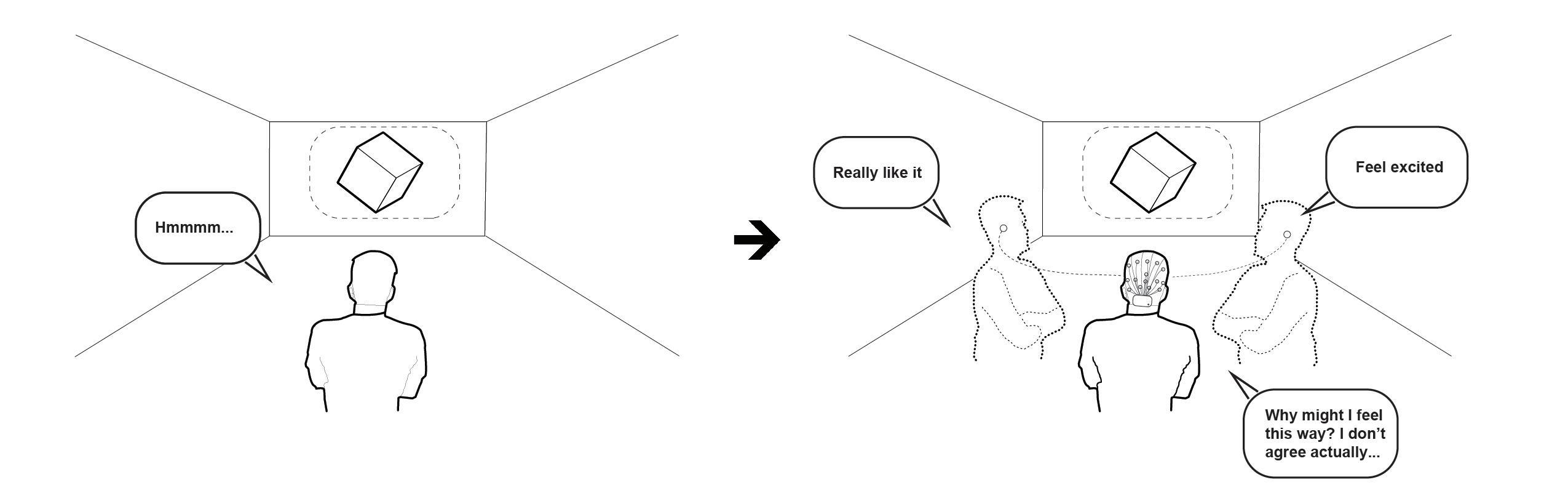
AnonymousAuthor(s).2018.Co-DesignwithMyself:ABrain-ComputerInterfaceDesignToolthatPredictsLiveEmotiontoEnhance MetacognitiveMonitoringofDesigners.In ACM,NewYork,NY,USA,21pages.https://doi.org/XXXXXXX.XXXXXXX
1INTRODUCTION
Designisregardedasanon-linearanditerativeprocessofdivergentandconvergentthinking.Duringthedivergent thinkingprocesses,designersframethedesignspaceandexploreasmanydesignoptionsaspossible,followedby designevaluationandsynthesisintheconvergentthinkingprocess.Theiterativedecision-makingprocessesarefull
Permissiontomakedigitalorhardcopiesofallorpartofthisworkforpersonalorclassroomuseisgrantedwithoutfeeprovidedthatcopiesarenot madeordistributedforprofitorcommercialadvantageandthatcopiesbearthisnoticeandthefullcitationonthefirstpage.Copyrightsforcomponents ofthisworkownedbyothersthanACMmustbehonored.Abstractingwithcreditispermitted.Tocopyotherwise,orrepublish,topostonserversorto redistributetolists,requirespriorspecificpermissionand/orafee.Requestpermissionsfrompermissions@acm.org.
© 2018AssociationforComputingMachinery. ManuscriptsubmittedtoACM
CHI’23,Hamburg,Germany, Anon.
ofuncertainty,whichmakesitchallengingfordesignerstoevaluatetheirdesignsintheearlystages.Itisalmost impossibletoforeseeifanearlydecisionwillbesuccessfulduetothecomplexityitcanunfold,especiallyinwicked designproblems.Expertdesignersrelyonintuitiontomakeconfidentjudgments,butthelackofself-reflectionand awarenessintheirresponsestodifferentdesignoptionsmakemanydesignerssecondguessthemselves[19, 38, 52]. Whendesignersaskthemselves“AmIontherighttrack”or“IfIhavefoundtherightdesign”,theanswerscouldemerge fromtheirintuitiveconfidenceratherthanrationalevaluations.Sometimes,designersalreadyhavetheanswerbut theyhesitatetomoveforward.Iflackingconfidence,excessivehesitationcoulddiscouragedesignersfromthoroughly exploringthedesignspaceandlimittheircreativity.Strongintuitionandbeingabletomaintainawarenessoftheir self-confidenceinearlydesignstagesareimportantforcreativedesignpractices[43].
Intuition,self-awareness,andfeelingsofconfidenceareentangledconstructsinthedesigncontext.Despitethe complexnatureofintuition,itisdescribedasacombinationofunconsciousevaluativeprocessesandconsciousreflective actsinthedesignpractice[19].Theconsciousreflectivepractice,alsoknownasself-awarenessormetacognitive monitoring,describestheprocessof“thinkingaboutthinking”andconsciouslyadoptingeffectivementalstrategies[1]. Indeed,beingabletoidentifythestrengthandweaknessesofone’sownideasandbeawareoftherationalesbehindtheir decision-makingwillimprovebetterideaevaluationandselection[48].Feelingsofconfidenceaboutdesigndecisions, namedepistemicuncertaintybyBallandChristensen,isalsocloselyrelatedtodesignevaluation[8].Accordingtothis outlook,fluctuationsinepistemicuncertaintymaybeanimportanttriggerthatpromptsdesignerstoalterorreinforce theirmentalapproachtoadesignchallenge.Toomuchuncertaintymaylimitthecreativeprocessbycreatinginsecurity, apprehension,andcreativeparalysis[3].Toolittleuncertaintyduringtheearlystagesofdesignmaycontributeto designfixation.Someepistemicuncertaintycanleadtodeliberatecounterarguments,initiatecreativereconsideration, andpotentiallypushadesignforward[8, 45].Intheory,metacognitivemonitoringplaysacrucialroleinmodulating andkeepingepistemicuncertaintywithineffectiveparameters,therebyavoidingtheextremesofdesignfixationor creativeparalysis[6, 10, 38].Asdemonstrated,self-awarenessandtheabilitytoperceiveaccurateuncertaintyand modulateafeelingofconfidenceindesignarecriticalpartsofstrongdesignintuition.Buildingupstrongintuition requiresyearsofstrategicpractice.Evenso,maintainingawarenessofconfidenceisstillchallengingbecauseinternal mentalstatesaredifficulttoperceive.
Recognizingtheimportanceofintuitivefeelingstowardsdesign,ourmotivationistoexplorethepossibilityof adesigntoolthatcouldmodulatedesigners’feelingsofconfidenceandaiddesigners’intuitivejudgmentthrough affectivecomputingandbiofeedback,specificallyinanarchitecturaldesigncontext.Affectiscloselyrelatedtointuition, metacognition,anduncertainty.Onecommonmodelofaffecttheorydescribesemotionsthatemergeinresponseto environmentalstimuliasablendofthreeindependentfactors:(1)pleasure,alsoknownasvalence,whichisanoverall feelingsof“positivity”or“negativity”towardssomething;(2)arousal,whichdescribesthedegreeof“excitement”;and (3)dominance,whichreferstofeeling“unrestricted”or“restricted”inanenvironment[39].Affectssuchas“gutfeelings” areconsideredtobefoundationsofintuition[17].Previousresearchhasshownthatmetacognitiveexperiencesare generallyassociatedwithimprovedaffect,especiallywithfeelingsofpleasure[14, 15].Uncertaintyisusuallyassociated withnegativeaffect,butitcanbepositivewhenpresentinlimitedamountsincreativecontexts[2].Knowingtheclose relationshipbetweenaffectandintuition,feelingsofconfidence,andmetacognition,weenvisionadesigntoolthat predictsiftheuserfeelsexcitedorpositivetowardsthecurrentdesign.Moreimportantly,thetoolwillvisualizethose predictionstousersinrealtimetohelpthemperceivetheirinternalstatesinathird-personperspective,inhopesof increasingself-awareness,modulatingfeelingsofconfidence,andimprovingintuition(Figure1).
Co-DesignwithMyself:ABrain-ComputerInterfaceDesignToolthatPredictsLiveEmotiontoEnhanceMetacognitiveMonitoringofDesigners CHI’23,Hamburg,Germany,
Priorworkhasusedavarietyofmeanstoidentifyaffect.Oneapproachisgroundedinevaluatingfacialexpressions andgestures,whichcanbedonecomputationallyviaemotion-recognitionalgorithms.Suchevaluations,however,are basedonsecondaryexpressionsofaffect,whichcanvaryquiteabitindifferentcultural,social,andindividualcontexts. Mostimportantlyforourpurposes,designisoftenasolitaryprocessandwhenworkingalonemanydesignersmaynot registertheirfeelingsviafacialexpressions.Anothermethodthathasbeenusedtoidentifysomeformsofaffectis electrodermalactivity(EDA),butthisphysiologicalreactionislackinginnuanceanddoesnotchangefastenoughto capturetherapidlychangingresponsesthatmayoccurduringthedesignprocess.UsingEEGmeasurementsprovidesa muchgreaterlevelofdetailanddirectlyevaluatesbrainresponses.Previousstudieshaveshownthataffectivestatescan bemeasuredviaEEG,includingaffectiveresponsestoarchitecturalenvironments[4, 9, 56, 62].Additionalstudieshave classifiedpleasure,arousal,anddominanceinnumerouscontextsandactivities,providingbenchmarksofaccuracythat canbeusedtoevaluateourBCIperformance[21,26,29,37,59,63].
Insum,thecurrentprojectprovidesseveralimportantcontributionstohuman-computerinteractionresearchandto thedevelopmentofcreativity-supporttools:
• Wepresentaclosed-loopBCIdesigntoolthatidentifiesandvisualizesusers’real-timeaffectlevelsbasedon theirEEGdata.
• WehaveexaminedtheBCItool’sfeasibilityandusabilitybyconductingusertesting.
• Theresearchevaluatesthepotentialforreal-timeaffectawarenesstomodulatefeelingsofconfidence,stimulate metacognitivereactions,andsupportcreativityfordesigners.
• Wediscussthepotentialapplicationsofthisworkandfutureavenuesforaffectivecomputingandbiofeedback insupportingcreativedesignprocesses.
2RELATEDWORKS
HerewereviewedrelatedworksonEEG-basedemotiondetection,devicesortoolsthatprovidebiofeedbacktousers, andhuman-AIco-creativetools.
2.1EmotionDetectionBasedonBCI
Givenitswiderangeofapplications,automaticemotionclassificationisgainingtheattentionofscholarsinHCIfields. Recentimprovementinconsumer-gradewearableEEGdevicescapturesinterestamongtheHCIcommunityinusing EEGforemotionclassification[58].Particularlyfocusedonarousalandvalenceaffectiveresponse,previousstudieshave usedtheEEGclassificationapproachinthecontextofusingmusicstimuli[42],musicvideos[5, 11, 55, 61, 64, 65],and videoclips[31, 36, 44, 57, 60].Theimmersivevirtualreality(VR)’sabilitytoevokeemotiongivesVRmoreinteresttobe usedasatoolinemotiondetectioningeneral[18, 22, 24, 25, 30, 67].Infact,theself-reportedintensityofemotionwas foundtobesignificantlygreaterinimmersiveVRcomparedtosimilarcontentinnon-immersivevirtualenvironments [7].InarelevantVRstudy,Marín-Moralesetal.[37]usedanEEGclassificationapproachandreachedanaccuracyof 75.00%alongthearousaldimensionand71.21%alongthevalencedimension(chancelevel:58%)intheclassificationof fouralternativevirtualrooms.Thesamestudywasoneofthefirststudiesthatdevelopedanemotionrecognitionsystem usingasetofimmersiveVRasastimuluselicitationanddiscussedtheapplicationoftheapproachinarchitecture.The currentstudytakesafurtherstepandaimsatdevelopingadesigntoolbasedonemotiondetection.
CHI’23,Hamburg,Germany,
Fig.2.ANew“Reflectors”TypeofAICo-creativeTool.
2.2BiofeedbacktoMyself
Severalstudieshavebeendonetohelpusersbeawareoftheirinnerstatesthroughdifferentmodalitiesoffeedback. OnestudydevelopedSelf-Interface,anon-bodydeviceattachingtothespinethathelpedusersperceivephysiological signalsbytranslatingthemtohapticfeedback[23].Similarly,anotherstudyhelpeduserstoperceivetheirpupildilation thatareassociatedwithdifferentcognitivetasksbymakingpupildilationaudible.Theirfindingssuggestedmostusers wereabletoassociatecognitiveactivitieswiththesounds,whichelicitedmetacognitiveawareness[12].Besideshaptic andaudialfeedback,athirdstudytranslatedheartrateintoshape-changingdisplays,whichbroughtawarenessof users’physiologicalstatesthroughvisualfeedback[66].TheInnerGardenprojectcreatedagardenwherementalstates identifiedbyEEGandbreathsensorsweretranslatedintoweatheranddaydurationoftheworld.Thedevicewas meanttoencourageself-reflectionandfostermindfulness[50].Themajorityofthestudiesfocusedmoreondeveloping thefeedbacksystemandlessonuserexperiencesinthecontextofanapplication.Nonetheless,thosestudiesoffer creativeideasabouthowbiofeedbackcouldbeperceivableandsuggesthowperceivingbiofeedbackcouldbenefit metacognitiveawareness.Otherapplicationsofbiofeedbackhavebeenexploredsuchasmodulatingbreathing[47], enhancingstorytelling,promotingcommunication[53],[20]andgaming[27, 41].Oneinterestingstudyinthesleep facilitationcontextdiscusseddesignstrategiesthatwefoundadaptabletoourstudyinthedesigncontext[54].Thestudy
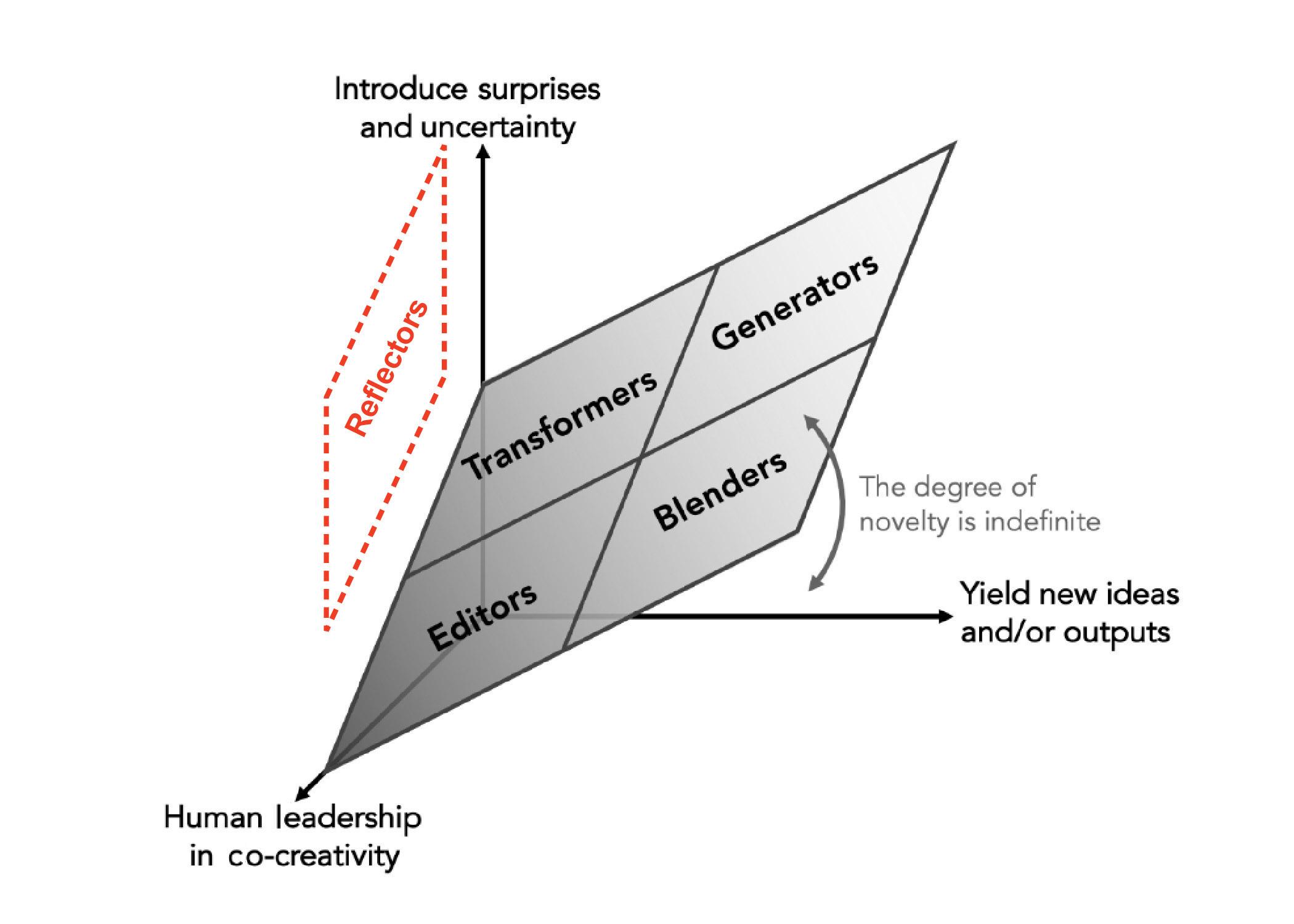
Co-DesignwithMyself:ABrain-ComputerInterfaceDesignToolthatPredictsLiveEmotiontoEnhanceMetacognitiveMonitoringofDesigners CHI’23,Hamburg,Germany,
pointedouttheimportanceofmotivatingexploration,promotingresponsivenessofBCI,andfacilitatingself-expression. Ourstudysharedthesamegoaltoincreaseuserawarenessinadifferentcontext.Thoughwefoundfewprojectshave specificallyexploredbiofeedback’spotentialindecision-aidingandsupportintuitionduringthedesignprocess.
2.3Co-EvaluatewithanAgent
Inarecentreviewarticle,Hsing-ChiHwang[28]arguedthatAI-basedco-creativetoolscanbedividedintofourtypes: “Editors”thatenablespecificdesignexecution,“Transformers”thatchangedesigncontenttodifferentforms,“Blenders” thatmixcreativeelementstogether,and“Generators”thatproduceentirelynovelcreativeproducts.Wefinditnotable thatthiscategorizationschemadoesnotincluderoomforthetypeofstabilizingandbiofeedbackmechanismsthatwe envisionedforthecurrentproject.Thus,weproposeafifthtypeofco-creativetool,“Reflectors.”Theydon’tcreate,but instead,focusonempoweringdesigners’self-awarenessandenhancingbyenhancingtheirself-awarenessandintuition duringtheprocessofconfrontingadesignproblem(Figure2).Indeed,human-AIco-creativeprocesswillenrichthe designspaceanddynamicsofdesigniterationandwethinkitisequallyimportanttodevelopaco-evaluatingagent thathelpsusersselecttheircreations.
3METHOD
WepresenttheBCIdesigntool“Multi-Self”whichaidsarchitecturaldesignprocesses.Itwasdevelopedwithtwo primaryparts.Thefirstwasanapplicationthatalloweddesignerstocreateaninteriorlobbyspaceinvirtualreality. Wechosethetaskofdesigningalobbybecauseitwasaverycommondesignproblemandbecausealobby’sfunction andrangeofpotentialformswerequiteflexiblecomparedtootherarchitecturalfeatures.Wedecomposedtheprocess oflobbydesignintofourcomponents:the Envelope thatdeterminedtheoverallgeometryandwindowsoftheroom, the Layout offurniturearrangements,the Fixtures thatinthiscasewerelimitedtolighting,andthe Color palettefor thevariousroomcomponents.Ineachofthesecategories,wepre-generated14to31models,whichcouldbefreely combinedyielding35,726,880potentialsolutionsinthedesignspace(Figure3).
TominimizemotoractionsandacquirecleanEEGdataofaffectduringthedesignselection,wedevelopedamixedmodalitycontrolfunctionforthislobbydesignprocess,whichcombinedheadpointingandkeypresses.Userswere abletofocusonadesignoptionthrougheyemovementsandthenpressbuttonstoadjustandconfirmtheselection.In additiontoprovidinggreaterease/fluidityinthedesignprocess,thisapproachhelpedtoreducelargemotionartifacts thatcanlimittheeffectivenessoftheEEGclassification.
ThesecondprimarycomponentofMulti-SelfwasaBCIalgorithmthatcategorizedusers’affectiveresponsesbased ontheirEEGdata(describedinmoredetailbelow),andprovidedreal-timebiofeedbackabouttheseresponseswithin theVRdisplay.TheformofthisfeedbackwasbasedontheemotioncoordinatesystemdevelopedbyLang[33].Thefirst quadrantdisplayedhigh-arousalandhigh-valencestates(emotionsgenerallydescribedas“joyful”or“excited”).The secondquadrantdisplayedhigh-arousalandlow-valencestates(“fearful”or“enraged”).Thethirdquadrantdisplayed low-arousalandlow-valencestates(“boring”or“sad”),andthefourthquadrantdisplayedlow-arousalandhigh-valence states(“relaxed”or“calm”).Duringthedesignprocess,users’liveemotionalfeedbackwasvisualizedinthecornerof theVRdisplayasamovingcoloredpointonthiscoordinategraph(Figure4).
3.1HardwareandSoftwareSetup
TheBCIdesigntoolincorporatedanEEGheadset,aVRheadset,anXboxadaptivecontroller,andacombinationof commercialandopen-sourcesoftware.EEGdatawereacquiredfromanon-invasive,32-channel,gel-basedmBrainTrain
261 262 263 264 265 266 267 268 269 270 271 272 273 274 275 276 277 278 279 280 281 282 283 284 285 286 287 288 289 290 291 292 293 294 295 296 297 298 299 300 301 302 303 304 305 306 307 308 309 310 311 312
CHI’23,Hamburg,Germany,
Fig.3.DesigningaLobbySpacebyCombiningEnvelope,Layout,Fixtures,andColor.
SmartingPro(mBrainTrainLLC.,Belgrade,Serbia).ChannelslocatedatFp1,Fp2,F7,F3,Fz,F4,F8,FT9,FC5,FC1,FC2, FC6,FT10,T7,C3,Cz,C4,T8,TP9,CP5,CP1,CP2,CP6,TP10,P7,P3,Pz,P4,P8,POz,O1,O2basedontheInternational 10-20system.AnHTCVivehead-mounteddisplaywaswornontopoftheEEGheadset(Figure5).EEGdatacollected duringthe“offline”trainingsessionforeachparticipantwerepreprocessedusingtheEEGLabtoolboxinMATLAB R2020b[13].MATLABwasalsousedtotrainthemodelsforinterpretingandcategorizingparticipants’neurological data.Duringtheonlinetestingsessions,thereal-timeEEGdatawasacquiredbyOpenVibe[49]andsenttoMATLAB throughtheLabStreamingLayer[13].TheEEGclassificationresultswerethendeliveredfromMATLABtotheUnity3D virtual-realitydisplayengineviatheUserDatagramProtocol(UDP).
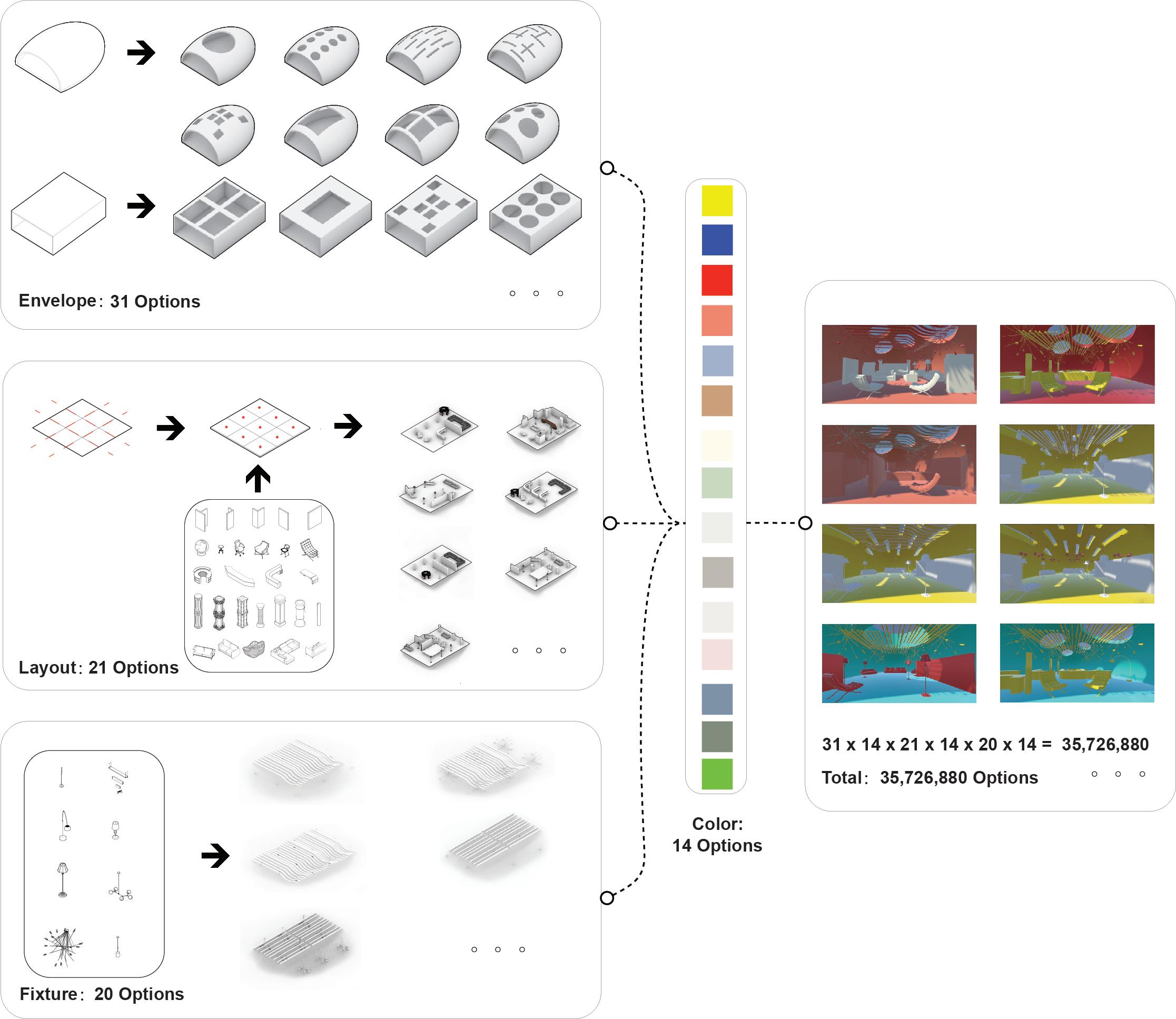
Co-DesignwithMyself:ABrain-ComputerInterfaceDesignToolthatPredictsLiveEmotiontoEnhanceMetacognitiveMonitoringofDesigners CHI’23,Hamburg,Germany,
Fig.4.(A)UsingtheEmotionCoordinateSystemtoDisplaytheResultsofEEGClassification;(B)Real-TimeVisualFeedbackinVR fromMulti-Self;(C)NeurologicalFeedbackandPop-upPanelsfrom"Multi-Self"inVRduringtheValidationSession.
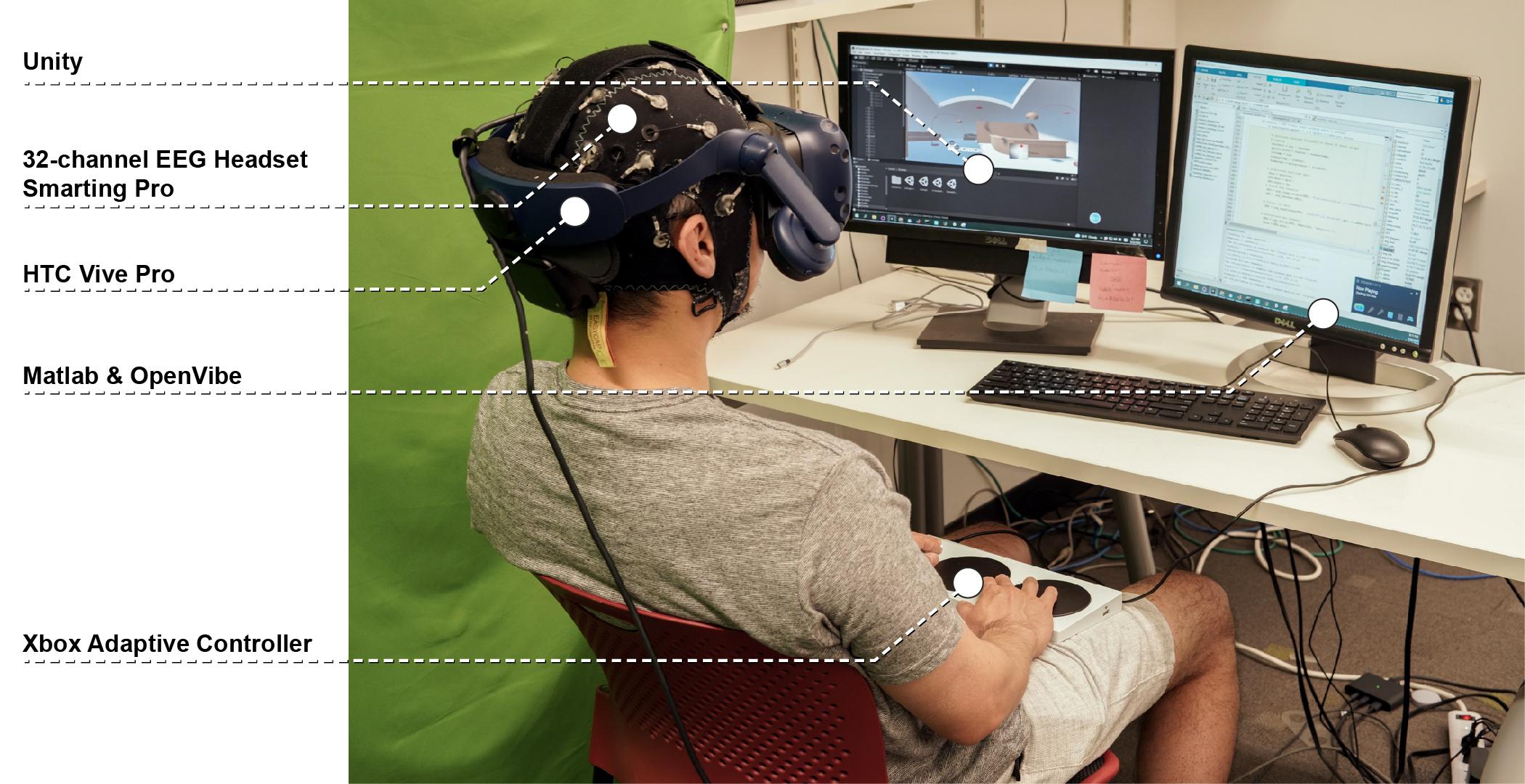
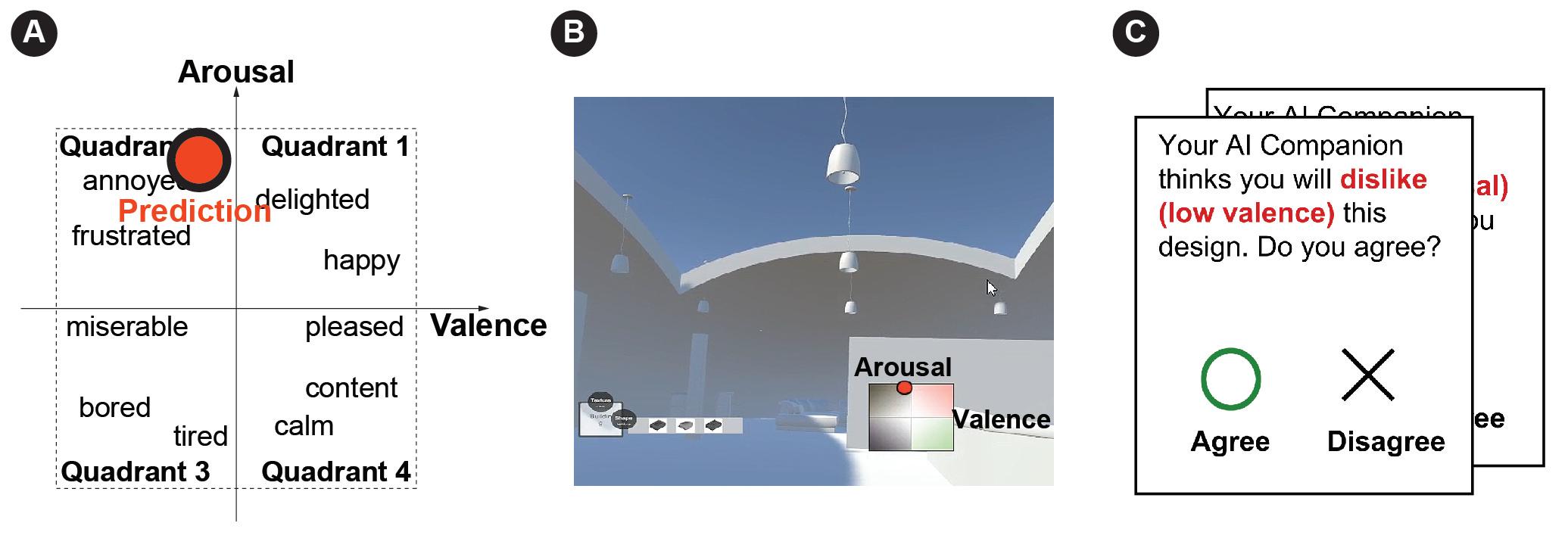
Fig.5.ExperimentSettingoftheAugmentedCo-DesignProcess.
3.2EEGPre-processingPipelineandMachineLearning
EEGdatafromthetrainingsessionandtheonlinesessionfollowedthesamepre-processingpipeline.Thedatawent throughaninitialArtifactSubspaceReconstruction(ASR)procedureviathecommercialmBrainTrainStreamersoftware (mBrainTrainLLC.,Belgrade,Serbia).Afterthisprocedure,theEEGdatastreamwasbandpassedtoisolatetherelevant frequencyband(1–40Hz).Then,badchannelsthatwereflatformorethan5secondsorexceedahigh-frequencynoise standarddeviationof4wereremovedandinterpolatedfromsurroundingchannels.TheEEGdatawerere-referenced basedontheaveragebandpowerfromallchannels.Foreachofthe32channels,theta(4–7Hz),alpha(7–15Hz),and 7
365 366 367 368 369 370 371 372 373 374 375 376 377 378 379 380 381 382 383 384 385 386 387 388 389 390 391 392 393 394 395 396 397 398 399 400 401 402 403 404 405 406 407 408 409 410 411 412 413 414 415 416
CHI’23,Hamburg,Germany,
Anon.
beta(15–30Hz)bandpowerswereextractedin2-secondtimewindows(with0.5-secondoverlaps).Wethenusedthe MinimumRedundancyMaximumRelevancy(mRMR)algorithm[46]toselectthefeaturesoftheEEGdatathatbest predicteddifferencesinemotionalresponses.Thisalgorithminvolvedaniterativeloopinwhich4datasegmentsare usedasatrainingsetand1wasusedforvalidatingtheprediction,eventuallyleadingtotheidentificationofthedata featuresthatgavethebestpredictiveaccuracy.Thevalidationsetwaschronologicallypartitionedtoavoidtemporal correlationthatwouldmistakenlyimprovetheaccuracy[35].Theclassificationanalysiswasconductedusingthe fitcecocfunctioninMATLAB(Figure6).
3.3Participants
Thepilottestwasintendedtoprovidepreliminaryfeasibilityanduser-feedbackdata,ratherthanrobustconfirmation ofscientifichypotheses.24participantswithnormalorcorrected-to-normalvisionwererecruitedthroughconvenience sampling(word-of-mouthandannouncementondepartmentale-maillists).Tenparticipantshadanarchitecturaldesign background.Theiragesrangedfrom19to36(M=22.9,SD=4.86).14participantsreportedasfemaleand10participants reportedasmale.ThreeparticipantsindicatedthattheyhadpreviouslyusedBCIsonrareoccasions,and21participants indicatedthattheyhadneverusedBCIs.Fourparticipantsreportedhavingmorethan8yearsofdesignexperience;Six participantsreportedhaving3–8yearsofexperience;Sixparticipantsreportedhaving1–3yearsofexperience;Eight participantsreportedhavingnodesignexperience.Wecategorizeddesignerswithover3yearsofdesignexperience asexpertdesignersanddesignerswithlessthan3yearsofexperienceasnovicedesigners,addingupto10expert designersand14novicedesigners.Sixoftheparticipantsreportedhavinglessthansixhoursofsleeptheprevious night—anotablefactorthatcouldpotentiallyaffectneurologicalresponsesandmoodstates.Theoverallstudyprotocol wasapprovedbytheInstitutionalReviewBoardpriortothestartofresearchactivities.
3.4ExperimentProcedure
Sessionswereconductedforoneparticipantatatime.Eachparticipantwasfirstaskedtocompleteapre-experiment survey.TheresearchersthenassistedtheparticipantwithfittingtheEEGandVRequipment.Theparticipantwasasked toengageinatrainingsessionsothattheBCIcouldbecomefamiliarwiththeirindividualemotionalresponses.This involvedobservingapanoramicVRimageofanarchitecturalenvironmentfor10secondswhilewerecordedEEG dataandthencompletingthebriefSAMscale[33]toreportarousalandvalence.Theprocesswasrepeateduntil49 pre-selectedenvironmentswereexperienced,whichtookaround15minutesintotal.
Next,participantswereplacedintoapracticesessionwithlimiteddesignoptionstofamiliarizethemselveswiththe head-pointingandXboxadaptivecontrollercontrols.Aresearchassistantgaveinstructionssuchas“pleasechangethe lobbyenvelopetoadifferentmodel,”andthepracticecontinueduntiltheparticipantwasabletofluidlyusethecontrols. Duringthistime,theresearcherspre-processedthedatacollectedduringthetrainingsessionandusedittocomplete themachine-learningclassification.Wetrainedtwo3-class,user-specific,linearSupportVectorMachine(SVM)models topredicthigh,neutral,andlowarousal,andhigh,neutral,orlowvalence.Thisprocesstookaround5to10minutes, andweexplainedtotheparticipantsthatwewereanalyzingthedatawhiletheylearnedthedesigncontrols.
Eachparticipantthencontinuedtothetesting/validationsession.Inthispartoftheexperiment,allofthelobby designoptionswereavailabletotheparticipant,andtheywereaskedtoexperimentwithdifferentoptionstocreatean effectivedesign.Weusedtwotechniquestovalidatetheaccuracyofthefeedbacktool.First,whentheparticipantmade anychangetothedesign,theywerepromptedtowaitfor3seconds,duringwhichtheirbrainactivitieswereanalyzed andclassifiedbytheBCItool.Theparticipantswerethenshowntheneurologicalfeedbackandaskedthroughapop-up
417 418 419 420 421 422 423 424 425 426 427 428 429 430 431 432 433 434 435 436 437 438 439 440 441 442 443 444 445 446 447 448 449 450 451 452 453 454 455 456 457 458 459 460 461 462 463 464 465 466 467 468
Co-DesignwithMyself:ABrain-ComputerInterfaceDesignToolthatPredictsLiveEmotiontoEnhanceMetacognitiveMonitoringofDesigners CHI’23,Hamburg,Germany,
paneliftheybelievedittobeaccurate(Figure4).Forexample,ifthemodelpredictedtheuserwasfeelinghigharousal, thepopupwindowwouldsay“YourAIcompanionthinksyouwouldfeelexcitedaboutthisdesignchange,doyou agree?(Yes/No).”Aftermakinganotherdesignchange,theparticipantwouldexperiencethesecondvalidationmethod, whichaskedthemtoreportarousalandvalenceviatheSAMscalewithoutseeingtheneurologicalfeedback.Thesetwo validationmethodsalternateduntiltheyhadeachoccurred6times.
Finally,theparticipantswereallowedtocontinuefreelyworkingonlobbydesignsuntiltheyweresatisfiedwith theoutcome,duringwhichtimetheycouldviewtheongoingfeedbackfromtheBCIresponse-trackingtool.Each participantwasaskedtocompletethreedifferentlobbydesignsinthisfashion,whichgenerallytookabout15minutes. Whensatisfiedwiththeirwork,theparticipantsremovedtheVRandEEGequipmentandcompletedashortdebriefing interviewabouttheirexperiences(Figure6).
3.5EnvironmentalStimuliUsedforTrainingtheBCI
Themostcommonenvironmentaldatasetsusedforemotionalresponseanalysisinpriorresearch,suchastheDEAP dataset[32],wereintheformatoftwo-dimensionalvideos.Wewereabletofindonlytwoexistingdatasetsoffering immersive3Dvideosforthispurpose.Oneofthemincludedoutdoorhumanandanimalactivitiesandwasthusunsuited forarchitecturaldesignanalysis[40].Anothersmallexisting3Ddatasetdidincludeindoorenvironmentalstimuli,but thisamountedtoonlyfoursamples,whichwasinsufficientforourmachinelearningneeds[37].Therefore,wecurated ourownimmersive3Denvironmentalstimulitostimulateaffectiveresponsesfromtheparticipants.Sinceweassumed participants’emotionalresponsestoarchitecturalenvironmentswouldbehighlypersonalized,wedidnotprescribe arousalandvalencelabelstotheseimagesbyaskingexpertstorateeachstimulus.Instead,wetriedtocreateaselection thatwouldbelikelytoelicitabroadrangeofemotionalresponses.Inthefirststepofthisprocess,wedownloadone hundred360-degreepanoramicimagesfromtheinternet.Someofthemwereimagesofreal-worldenvironmentsand otherswererenderingsofimaginedspaces.Toremovestimulithatwouldbeunlikelytoprovokeanaffectiveresponse, twojudgeswithover5yearsofarchitecturaldesignexperiencewereaskedtoviewallofthedownloadedimagesvia thesameHTC-Viveheadsetusedintheexperimentandtoreporttheirarousalandvalencelevelsrelatedtoeachimage usingtheSAMscale.Weremovedimagesthatreceived“moderate”scoresfrombothjudgesoneachaxis(scoresof2or 4,outofa1–5range).Thefinalselectionof50imageswasmadewiththegoalofobtainingabalancebetween“high” (5),“neutral”(3),and“low”(1)scoresoneachaxis,toensurethateachquadrantontheEmotionCoordinateSystem wasreasonablyrepresented.Duetooneoperationerrorfromtheexperimenter,thefirststimuluswassystematically notpresentedtoalltheparticipants.Eventually,participantsexperienced49stimuli.Thedistributionofjudges’ratings andtheaverageratingsof25participantswereshowninFigure7.
3.6OutcomeMeasures
Wemeasuredmachinelearningperformancebythreemetrics:(1)offlinevalidationaccuracy,(2)onlineuseragreement withthedisplayedneurologicalfeedback,and(3)consistencybetweenonlineself-reportingandunseenneurological feedback.Itshouldbeemphasizedagainherethataseven-participantconveniencesampleisnotsufficienttovalidate theseoutcomesscientifically;thepurposeofthispilotstudywasinsteadtoconductproof-of-concepttestingandobtain initialuserfeedback.Becauseourtrainingdatawasimbalanced,wemeasuredtheimbalanceratiocalculatedbythe divisionbetweenthenumberoftheclasswiththemostsamplesandthenumberoftheclasswiththeleastsamples. Validationaccuracywascalculatedastheaverageof5-foldvalidation,whichmeantthatwepartitionedthedata intofivechronologicallyseparatepartstoavoidtemporalcorrelations[35].Werepeatedlyused80%ofthedataasthe 9
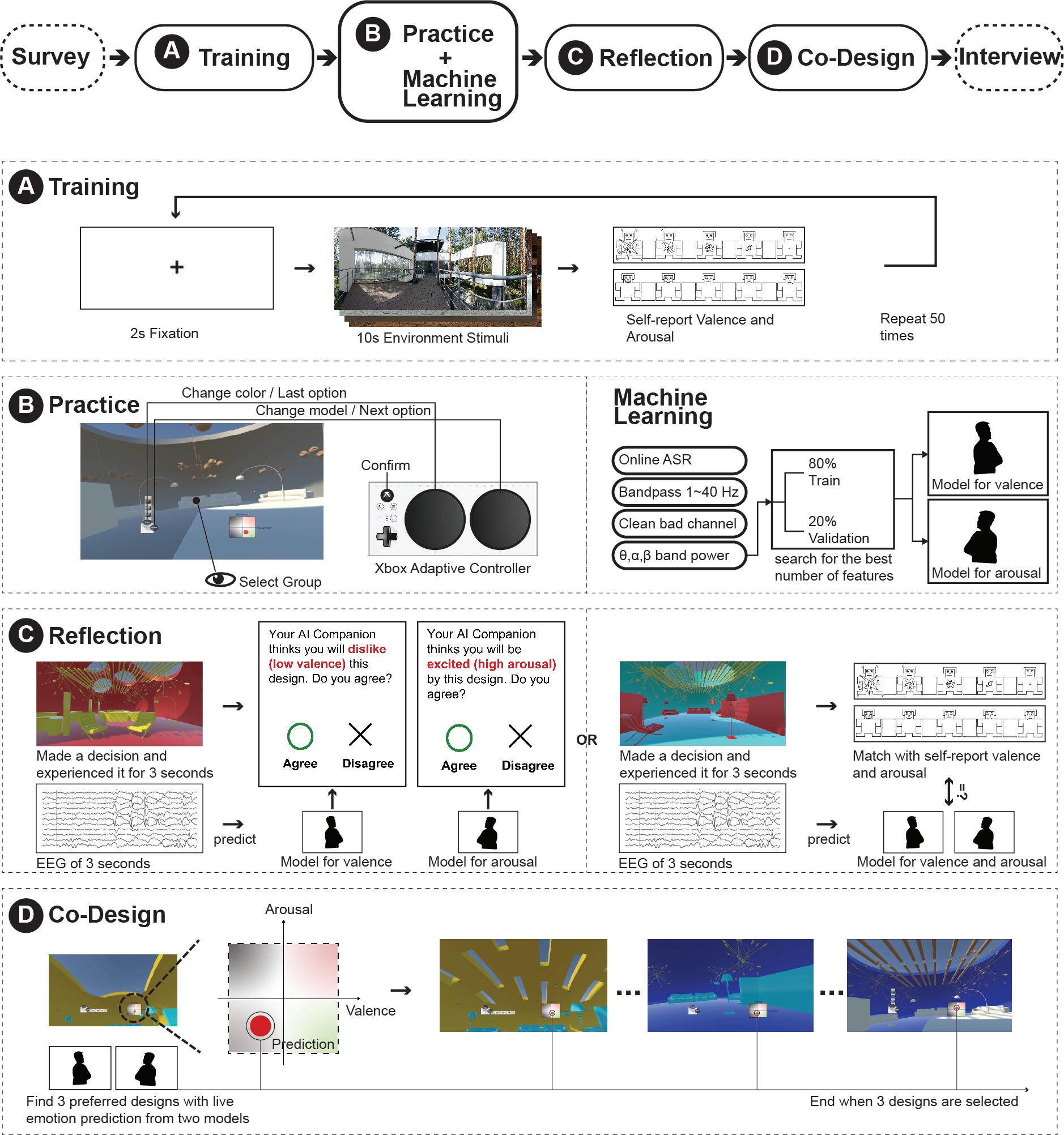
521 522 523 524 525 526 527 528 529 530 531 532 533 534 535 536 537 538 539 540 541 542 543 544 545 546 547 548 549 550 551 552 553 554 555 556 557 558 559 560 561 562 563 564 565 566 567 568 569 570 571 572
Co-DesignwithMyself:ABrain-ComputerInterfaceDesignToolthatPredictsLiveEmotiontoEnhanceMetacognitiveMonitoringofDesigners CHI’23,Hamburg,Germany,
Fig.7.TheEmotionRatingsofStimulibytheJudgesandtheParticipants:(A)AverageEmotionRatingsofTwoJudges; Dotlocations wereslightlydodgedtomakeoverlappingdotsvisible. (B)AverageEmotionRatingsof25Participants. Dottedcircleshighlightsome stimuliforcomparison.
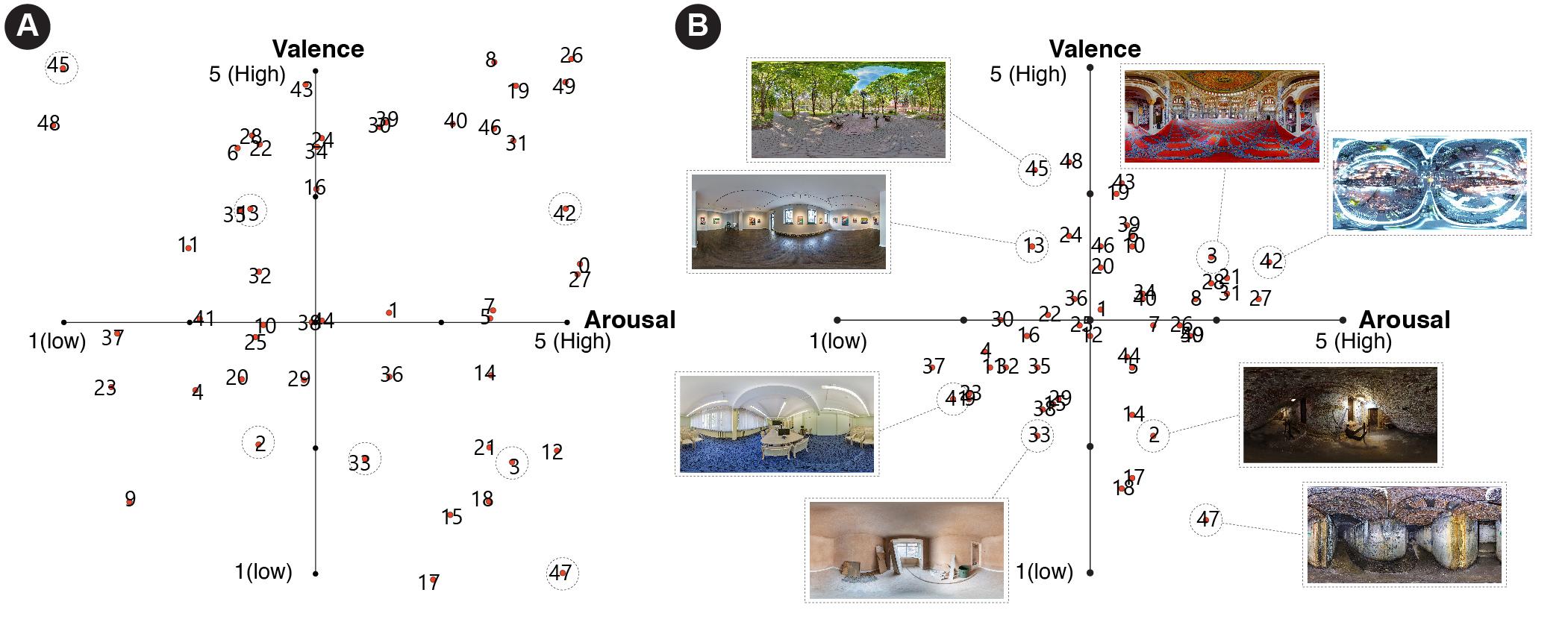
Theuseragreementvalidationwasasimplecalculationofthenumberofagreementsdividedbythetotalnumberof trials.Thoughthismeasurewassubjectiveandsusceptibletobias,itdescribedperceivedaccuracyandthushelpedus tounderstandtheperceivedtrustbetweentheuserandthetool.
Theconsistencybetweenonlineself-reportsofarousalandvalencevs.theunseenmachine-learningpredictions wasalsomeasuredasthenumberofagreementsdividedbythenumberoftotaltrials;theseoutcomeswereabetter indicationoftheobjectiveaccuracyrate.
Qualitativedataweregatheredduringsemi-structuredexitinterviewstounderstandthepotentialimpactsof emotionalfeedbackonmetacognitivefeelings.Westartedwithsevenquestions:[Q1]Couldyouuseafewwordsto describeyourexperiencesinthedesignscene?[Q2]DoyouthinkyoubuiltsometrustwiththeAIsystemandcould yougiveasubjectiveratingfrom1to10?[Q3]Doyouthinkthereal-timevisualizationofvalenceandarousalchanged yourdecision-making?[Q4]Doyouthinkthevisualizationofvalenceandarousalencouragedyoutoexploremore designoptions?[Q5]WhatwasthegreatestchallengeduringyourdesignprocesswiththeAIsystem?[Q6]Ifyouhad magic,whatchangesyouwishtomakeforthisdesigntooltoaidyourdesignprocessbetter?[Q7]Whatotherdesign scenariosdoyouthinkthisdesigntoolcanbeappliedto?
Fromthetranscription,weratedusers’subjectivetrustlevelonanordinalscale(“low”,“medium”,and“high”). Becauseparticipantsinteractedwiththereal-timeemotionpredictionsmoreactivelyinthedesignscenario,which wouldsignificantlyaffecttheirtrustinthesystemandwasn’tmeasuredbytheuseragreementinthevalidationscenario. Wethinksubjectivetrustlevelwouldprovideanotherperspectivetotheuserperceptionofoursystem.Weratedthe subjectivetrustlevelbasedonthefollowingcriterion:Ifparticipantsgaveasubjectivetrustratingabove7in[Q2] duringtheinterview,ortheyexpressedopinionssuchas“Thesystemmetmyexpectation”or“thesystemwasreally responsive”,weratedthemas“high”.Ifparticipants’subjectivetrustratingwasbetween3and7,ortheyexpressed opinionssuchas“Ionlytrustthesystemundercertaincircumstances”,weratedthemas“medium”.Ifparticipants’ subjectivetrustratingwasbelow3ortheyexpressedopinionssuchas“Thepredictionsdidn’tmovethatmuch”,we ratedthemas“low”.
573 574 575 576 577 578 579 580 581 582 583 584 585 586 587 588 589 590 591 592 593 594 595 596 597 598 599 600 601 602 603 604 605 606 607 608 609 610 611 612 613 614 615 616 617 618 619 620 621 622 623 624
CHI’23,Hamburg,Germany, Anon.
WealsoevaluatedtheuserexperiencequantitativelyusingtheUserExperienceQuestionnaire(UEQ)[34].Participants wereinstructedtoevaluatetheirexperienceofdesigningwithreal-timeemotionalfeedback.TheUEQassessesthe qualityofinteractiveproductsandconsistsofsixsub-scales.“Attractivenessscalemeasurestheoverallimpressionof theproduct;Perspicuitymeasuresiftheproductiseasytounderstandandlearn;Efficiencymeasuresiftheinteraction isefficientandfast;Dependabilitymeasuresiftheuserfeelsincontroloftheinteraction;Stimulationmeasuresifthe productisexcitingandmotivating;Noveltymeasuresiftheproductisinnovativeandcreative.”[51].Thescaleranges from-3to3.TheUEQhelpsusunderstandifthedesigntooloffersadecentuserexperiencetotheusersinadditionto subjectiveandobjectiveaccuracy.
4RESULTS
4.1MachineLearningClassification
Theaverageofflinevalidationaccuracywas49.3%(SD=0.078)forthe“arousal”dimensionofaffectiveresponse,and 51.8%(SD=0.060)forthe“valence”dimension.Thisoutcomewasmoderatelystrongsincewewereusingathree-fold categorizationschema(high,neutral,andlow).Thechanceofrandomlyobtaininganaccurateclassificationwouldbe only33%.Achieving49–52%accuracythusindicatesareasonablygoodpredictivecapability(Figure9).Theaverage imbalanceratioforarousalis2.71(SD=1.79).Theaverageimbalanceratioforvalenceis2.94(SD=1.44).
4.2DesignToolValidation
Inregardtotheonlinevalidation,wecollectedatotalof137responsesfortheuser-agreementmetricand129responses forself-reportedmeasuresvs.unseenpredictions(Figure8).Therewereinterestingdiscrepanciesbetweenthesemetrics forbothvalenceandarousal.Inthecaseofvalence(positiveornegativeaffect),participantsagreedwiththeAI’s prediction57.7%ofthetime—however,theirself-reportingmatchedunseenAIpredictions49.6%ofthetime.Inthe caseofarousal,participantagreementwiththeAI’spredictionwashigherat62.8%,whiletheirself-reportingmatched unseenAIpredictionsonly27.1%ofthetime.
Regardingparticipants’subjectivetrustlevels.41.7%(n=10)participantswereratedas“high”,29.2%(n=7)participants wereratedas“medium”,and29.2%(n=7)participantswereratedas“low”.Theoverallperceivedsubjectivetrustlevel wassimilartotheuser-agreementvalidation,whichdemonstratedthatparticipantsgenerallyhadamoderatelystrong subjectivetrustinourdesigntool.
4.3InterviewFeedback
Weusedaqualitativecontent-analysisapproachtoparsetheparticipants’responsesfromtheexitinterviews[16].All responsesweretranscribedbyaresearchassistant,whoalsodevelopedapreliminarycodingschemabasedoncommonly voicedthemesrelatedtotheresearchvariables.Thethreemainthemesidentifiedinthedatawere:(1)attitudesand usageperspectivestowardtheBCIdesigntool;(2)expressionsofuncertaintymodulationandmetacognitiveawareness associatedwiththetool’sfeedback;and(3)expressionsofwhyuserstrustedordidnottrustthetool.Twootherresearch assistantsindependentlyreviewedthetranscriptsandcodedthedatasegmentsbythesethemes.Aftertheresearch assistantscompletedtheirindependentcoding,theymettocollaborativelyresolveanydisagreementsabouthowthe textsegmentswereorganized.Theresultingthemesandexamplequotationsarepresentedinthefollowingsections.
625 626 627 628 629 630 631 632 633 634 635 636 637 638 639 640 641 642 643 644 645 646 647 648 649 650 651 652 653 654 655 656 657 658 659 660 661 662 663 664 665 666 667 668 669 670 671 672 673 674 675 676
Co-DesignwithMyself:ABrain-ComputerInterfaceDesignToolthatPredictsLiveEmotiontoEnhanceMetacognitiveMonitoringofDesigners CHI’23,Hamburg,Germany,
Fig.8.(A)OfflineTrainingandValidationAccuracyofAllParticipants;and(B)ROCforArousalandValenceClassificationofA SingleParticipant.
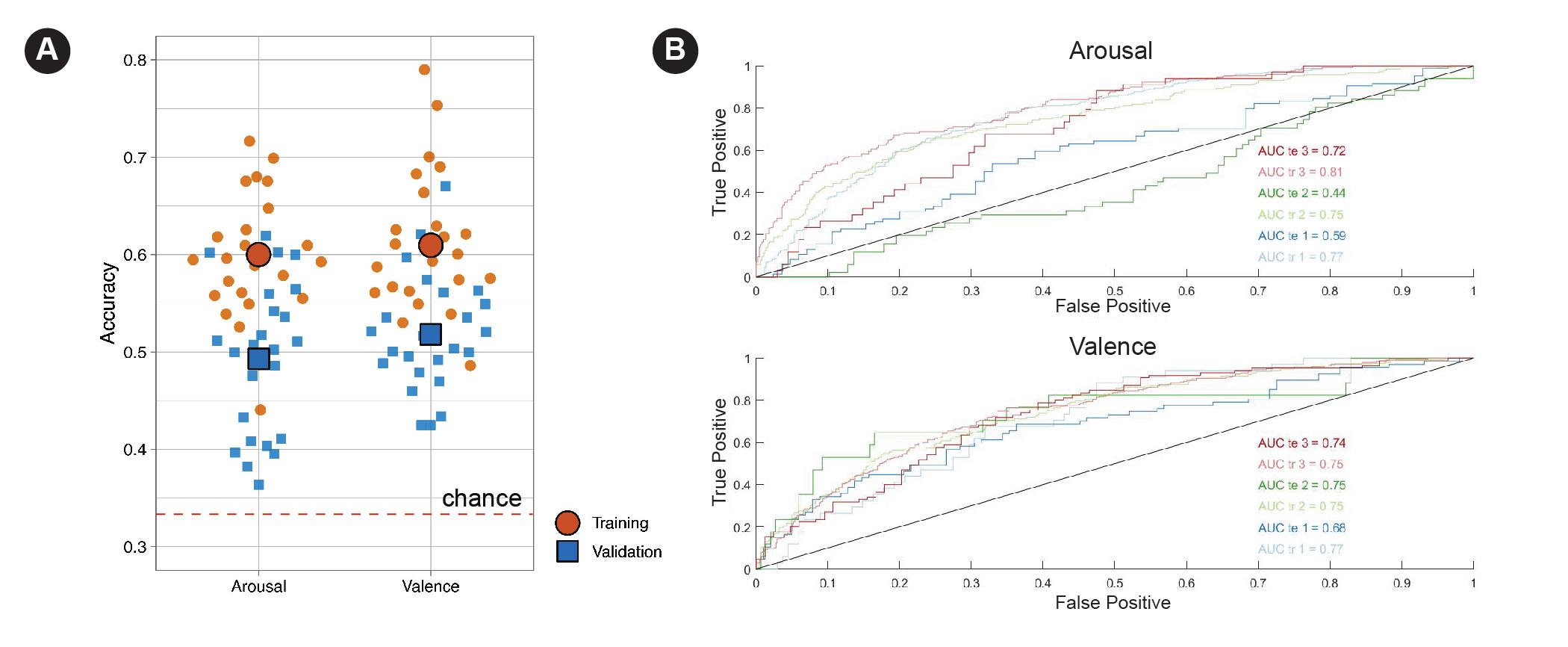
4.3.1AttitudesandUsagePerspectives. WeidentifiedfivedifferentattitudestowardstheBCIdesigntoolasexpressedby participants,whichinsomecasesledtheparticipantstousethetoolinadifferentmanner.Someparticipantsexpressed morethanoneoftheseattitudesatdifferentpointsintheirinterviews.
Thefirstattitudewastotreatthetool’sfeedbackasanimportantmetrictoguidedesigndecisions.Thisoutlookwas expressedby20.8%(n=5)oftheparticipants.Withthisattitude,participantswouldcontinuetestingdifferentdesign optionsuntilthetool’sfeedbackmovedinadirectiontheyregardedasfavorable.Forexample,Participant16said, “I woulduseittoseeifthepredictionchanged.Andifitchanged,Iwouldkeepadesign,evenifIdidn’tnecessarilywantto.” Participant18said, “Ichosethingsthatgavememorepositive[valence]andlessarousal.” Participant11said, “Myemotion predictiontellsmeIaminterestedinit,andIthoughtaboutitandeventuallydidendupsettlingonsomeofthem.” These participantsusedthetool’sfeedbackaspromptingtoreconsiderandevaluatetheirdesigndecisions.
Thesecondattitudewastousethesystem’sfeedbackasaconfirmationofindigenousfeelings.Thisoutlookwas expressedby25%(n=6)oftheparticipants.Intheseresponses,participantsindicatedthattheymadeadesigndecision firstandthencheckedthemodelforpotentialconfirmation.Participant9said, “OnceIgottoadesigndecisionthatI liked,Iwouldthenlookatthequadrant.Andifitaligned,thenIwouldalmosttakethatasasecondvoicetellingmeifthat decisionisrightornot.” Participant13said “Imadethearrangementofthefurniture,thenIsaw,likethedotmovetothe rightdirection,whichIexpect,thenIthinkit’sgood.” Essentially,theseparticipantswereusingthetoolasameansto boosttheirconfidenceintheirdesignskills.
Thethirdattitudewastousethesystemasafinaljudgmentwhentheparticipantwasundecided.Onlyasmall numberofparticipants(8.3%,n=2)fitintothiscategory.Theyindicatedthattheysimplyignoredthetoolmostofthe time,andonlycheckedforitsadvicewhentheyhadtroubleselectingbetweentwodesignoptions.Participant15said, “WhenIwasreallyhesitatingbetweentwooptions,likewhenI’mnotsure—oh,isthisbetter?Oristhisbetter?Icouldlike lookatitandbelike,oh,itsaysIlikethisbetter.Okay,soundsgood.”
Manyoftheparticipants(33.3%,n=8)expressedanattitudeofcuriosityandplayfulnesswheninteractingwiththe tool.Theyregarditasanopinionatedcompanionthatcouldprovideinsightsintodesigngoalsandpromptexploration.
677 678 679 680 681 682 683 684 685 686 687 688 689 690 691 692 693 694 695 696 697 698 699 700 701 702 703 704 705 706 707 708 709 710 711 712 713 714 715 716 717 718 719 720 721 722 723 724 725 726 727 728
CHI’23,Hamburg,Germany, Anon.
Participant6said, “Iintentionallywentagainstthepredictionstoseewhathappen.” Thisparticipantadded, “Iwouldbe happytoseeiftheAIhasthesameopinionasmine,butIstilltrustmycurrentemotions.” Participant25said, “Itriedto catertoafewdifferentemotionsbasedonhowitshowedmeIwasfeeling.”
Anotherlargegroupofparticipants(33.3%,n=8)didnotengageinanyoftheaforementionedattitudes,butinstead ignoredthetool’sfeedbackcompletely.Oneofthestatedreasonsforthisreactionwasthattheyfeltconfidentintheir emotionsanddidnotfeeltheycouldgainanyinsightsfromthefeedback.Participant20said, “Ididn’treallylookat[the affectfeedback]...IthinkIamfullyawareofmyfeelings.” Similarly,participant22said, “Ididn’tpaymuchattention toit;Imainlydesignbasedonmyownemotions.IfocusmoreonhowIfeelbymyself.” Otherparticipantsignoredthe tool’sfeedbackbecausetheybelievedemotionswerefleetingandshouldnotbeprioritizedduringarchitecturaldesign. Participant1said “Ifeltdesigningaspacewoulddependonmanyotherfactors,suchasfunction.” Othersindicatedthat theyperceivedthefeedbackasanunhelpfulanduntrustworthydistraction.Participant3said, “Iactuallydidn’tlookat theemotionfeedbackthatoftenbecauseitdidn’tchangethatmuch.”
4.3.2Metacognition. Intheinterviewdata,participantexpressionsofmetacognitionwereassociatedwiththreetypes ofexperience:(a)usingthetooltocontinuouslytrackone’sownemotionalresponses;(b)observingamismatchbetween thetoolsfeedbackandconscious/indigenousfeelings,and(c)observingamatchbetweenthetool’sfeedbackand conscious/indigenousfeelings.Someparticipantsexpressedmorethanoneoftheseattitudesatdifferentpointsintheir interviews.Intotal,45.8%(n=11)oftheparticipantsmentionedsometypeofmetacognitivereactiontothetool.
Observingcontinuousexperienceviathetool’sfeedbackencouragedtheparticipantstoviewtheirdesignsfroma third-personperspective.Thisexperiencewasmentionedby16.7%(n=4)oftheparticipants.Forexample,Participant 2said, “It’sinterestingenoughtoseethechangingofmyownexperience.” Participant5said “Thepredictioncouldturnthe designerintotheaudienceposition,andthinkaboutwhattheywouldfeelasthefirst-timeaudience.” Participant14said “I actuallydidn’tfeelmyemotionsthatwell,sothetoolactuallyencouragesmetoreflect.Itforcesmetomakeachoicelike it’srightornot.”
Thelargestportionofmetacognitivereflectionswasassociatedwithaperceivedmismatchbetweenthetool’s feedbackandindigenousfeelings.Thisexperiencewasmentionedby41.7%(n=10)oftheparticipants.Interestingly, theperceptionofmismatchledtoawiderangeofresponses.Someparticipantswerepromptedbythefeedbackto reassesshowtheywerefeelingaboutthedesign.Participant15said, “Ithoughtthatsomethinglooksgood,andit[the tool]waslike,ohyoudon’tthinkitlooksgood.Intriguing.” Otherparticipantsinthiscategorywerepromptedbythetool toassesstheirconceptualdesignanalysis.Participant9said, “Iusuallywouldn’tchoosepurecolorsbutnowIwonderif theremightbesomethinginitthatmakesmelikethedesign.” Participant16said “IwouldlookatdesignchoicesthatI probablywouldnothavemadeotherwise.Iwouldlookatthedifferent,likebrightercolors,eventhoughIprobablywouldn’t havedonethatbefore.” Ingeneral,wefoundthatexperiencesofmismatchencouragedparticipantstoexploreawider designspaceandconsideralternativesolutions.
Incontrast,however,someparticipantsfeltthatthetoolhadbeentrained“incorrectly”andwantedtoreviseitto bettermatchtheirindigenousperspectives.Participant21said, “Aftertheonlinesession,IwishIcouldchangehowI answeredinthetrainingsessionsothemodelcouldservemypurposebetter.” Afewparticipantsrejectedthetooloutright. Participant15said, “Iamlike,manthiscolorlookssobad,and[thetool]toldmeIwouldlikethiscolor.Ididn’ttrustit.” Otherparticipantsindicatedthattheytrustedthetoolonlywhenitprovidedcertaintypesoffeedback.Participant11 said, “Ifoundwhenthepredictionmovedprettyfasttothebottomright,Idisagreedwithit.” Thesameparticipantadded, “Itrustthemovementofthepredictionsratherthantheirpositionsinthequadrant.”
729 730 731 732 733 734 735 736 737 738 739 740 741 742 743 744 745 746 747 748 749 750 751 752 753 754 755 756 757 758 759 760 761 762 763 764 765 766 767 768 769 770 771 772 773 774 775 776 777 778 779 780
CHI’23,Hamburg,Germany,
Co-DesignwithMyself:ABrain-ComputerInterfaceDesignToolthatPredictsLiveEmotiontoEnhanceMetacognitiveMonitoringofDesigners
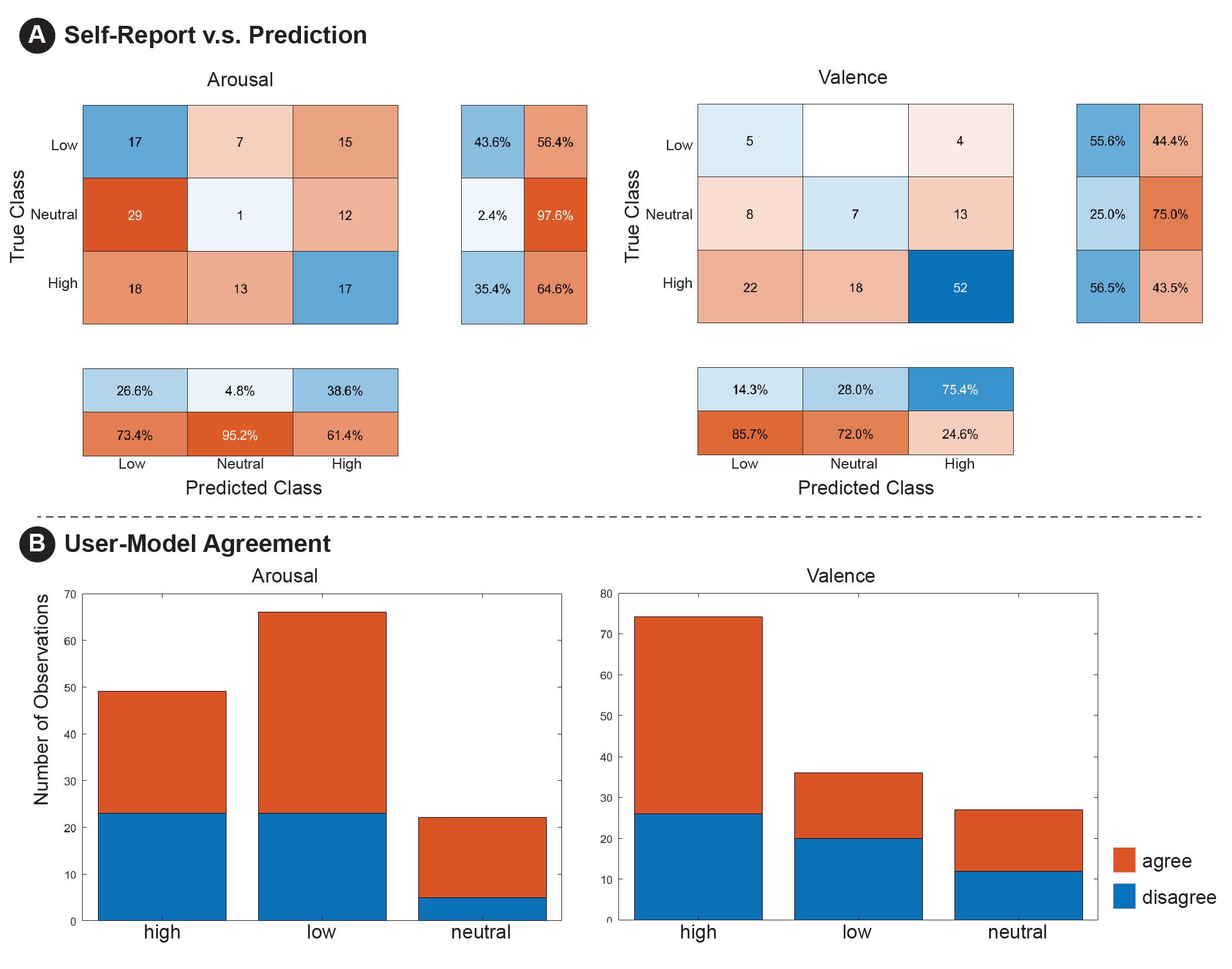
Whentheparticipantsmentionedthatthetool’sfeedbackwasaclosematchfortheirindigenousfeelings,they associatedthisexperiencewithanincreaseinconfidenceandtheabilitytonarrowdownthedesignspace.Thistypeof metacognitiveexperiencewasmentionedby8.3%(n=2)oftheparticipants.Onenotablefindinginthisareawasthat manyoftheparticipantswhodiscussedmatchingfeedbackfromthetoolalsoindicatedthattheyonlyrarelyreferred toitsoutput.Forexample,Participant13said, “ItmakesmeconfidentaboutmydecisionbutIdodesignbasedonmy preference,soIdidn’tpaymuchattentiontoit.” Apersistentpatterninthiscategoryofresponsewasthattheparticipants wereusingthetoolwhenneededtoimprovetheirconfidence,andotherwisedidnotgivemuchconsiderationtoits feedback.
reportedhigherlevelsofperceivedfeedbackaccuracyalsoprovidedmorepositivecommentaryduringtheinterviews; forexample,Participant2statedthat, “Seeingtheemotionalresponsesmotivatedmetomovethereddottothetop-right
781 782 783 784 785 786 787 788 789 790 791 792 793 794 795 796 797 798 799 800 801 802 803 804 805 806 807 808 809 810 811 812 813 814 815 816 817 818 819 820 821 822 823 824 825 826 827 828 829 830 831 832
CHI’23,Hamburg,Germany,
Anon.
corner.” InthiscategoryofresponsetheparticipantsfrequentlymentionedusingtheBCItoolintheintendedfashion. Participant25mentionedthatthetoolpromptedawiderdesignexploration: “Iwentthroughdifferentdesignoptionsto explorehowthepredictionwent.” Participant9emphasizedimprovedconfidenceintheirdesignchoices: “thevisualization helpedmeconfirmmyjudgement”
Incontrast,participantswhofeltthetoolwasinaccuratereportedlowerlevelsoftrustandinsomecasesoutright hostility.Participant6,forexample,saidthatthetoolwastryingtodirectthemawayfromdesignsthattheyactually likedandthattheyhadto “chooseagainsttheAI’sdecision.” Itwashardtodeterminecausalityinthesetypeof responses—whetherparticipantsreceivedmismatchedfeedbackandthuscametodistrustthetool,orwhetherinitial distrustledthemtofocusoninstancesofmismatchedfeedbackandvehementlyrejectit.
Anotherfactorthatemergedintheinterviewsinrelationtothetool’sacceptabilitywastheintuitivenessanddetail levelofthefeedbackvisualization.Participant7broughtupthequestionofBCItransparency,statingthat, “Itwouldbe moreconvincingifwecouldseetherawbrainactivitydatainsomeartisticwayatthesametime.IfIcouldunderstand howthosehigh-levelpredictionsweregenerated,thenIwouldtrustthetool’spredictionsmore.” Anothersuggestionfrom Participant7wasthatthebiofeedbackcouldbeshownonanavatarmodel: “Wewereexperiencingthearchitectureina first-personperspective.Maybeyoucouldsometimeschangeittoathird-personperspectivesowecanseetheavatarof myself,andmonitorthatavatar’sbiofeedback.”
Finally,weobservedthattheextentofparticipants’designexperiencewasnegativelyassociatedwithacceptanceof thetool.expertdesignersaremoresuspiciousofthedesigntoolandtendedtousethetooldifferentlyfromnovice designers.Amongthe10expertdesigners,60%(n=6)expressedlowtrustinthetoolandonlytwoofthemexpressed hightrust.Inacontrastingmanner,only7.1%(n=1)expressedlowtrustandeightofthemexpressedhightrustin thetoolamongthenovicedesigners.Regardinghowtheyusethetool,expertdesignersnevertreatthepredictions asimportantmetricsandfourofthemusepredictionstoconfirmtheirideasormotivateexploration.Fournovice designers,however,treatthepredictionsasimportantmetrics,andsixusepredictionstoconfirmorexplore.Despite expertdesignersdemonstratingmuchlowertrustinthedesigntool,theirmetacognitivefeelingsemergesimilarlyto novicedesigners.40%(n=4)expertdesignersand57.1%(n=8)novicedesignersreportedmetacognitivefeelings.
4.4UserExperienceQuestionnaire
Regardingtheuserexperienceofdesigningwithreal-timeemotionalfeedback,fivesub-scalesofUEQwerewithinthe "aboveaverage"or"good"rangebasedontheUEQbenchmark[51]: Attractiveness (M=1.22,SD=0.81),Efficiency(M= 1.14,SD=0.81),Perspicuity(M=1.76,SD=0.82),Stimulation(M=1.32,SD=1.01),andNovelty(M=0.94,SD=1.01). Dependabilitywasratedas"belowaverage"(M=0.79,SD=0.63),whichwasnotsurprisingsincethedesigntoolwas meanttointroduceuncertaintytothedesignprocess.
5DISCUSSIONANDCONCLUSION
Thecurrentresearchwasfocusedondevelopingaclose-loopBCI-VRdesigntooltoaugmentusers’real-timeawareness ofaffectlevelsbasedontheirbraindynamicsasrecordedbyEEG.Theresultsofthestudycanbediscussedinthree categories,includingEEGclassificationresults,userexperiencesininteractionwiththesystem,andapplicationofthis novelapproachinthedesignprocess.
Thevalidationaccuracyofoursystemin3-classpredictionofthearousaldimensionwas49.3%(range36.4%to62.0%; chancelevel:33.3%).Alongthevalencedimension,theaccuracyis51.8%(range67.0%to42.5%;chancelevel:33.3%). OuraccuracyiscomparabletopreviousstudiesfocusedonpredictingvalenceandarousalbasedonEEGdata[58].This
833 834 835 836 837 838 839 840 841 842 843 844 845 846 847 848 849 850 851 852 853 854 855 856 857 858 859 860 861 862 863 864 865 866 867 868 869 870 871 872 873 874 875 876 877 878 879 880 881 882 883 884
Co-DesignwithMyself:ABrain-ComputerInterfaceDesignToolthatPredictsLiveEmotiontoEnhanceMetacognitiveMonitoringofDesigners CHI’23,Hamburg,Germany,
accuracyshouldalsobeconsideredinthecontextoftheoverallusesituation—whereaspriorstudiesinthisareahave focusedonenvironmentsintendedtoprovokestrongaffectiveresponses,weevaluatedresponsesduringanordinary designtask.Thus,thedegreeofaccuracyachievedisnotablewithinthecurrentstate-of-the-artoffield.However,while theEEGclassificationperformedmuchbetterthanthechancelevel,itshouldprobablyberegardedasstillinsufficientto supportwidespreadsatisfactoryuserexperiencesinreal-lifescenarios.Infuturework,thereareavarietyofoptionsfor potentiallyimprovingperformance,suchasimprovingthetrainingstimuli,increasingthenumberofstimulitrials,and longerpracticesessions.MoreadvancedBCIsmayeventuallybeabletoadapttoindividualusersoveralongduration oftime,leadingtoincreasinglyaccurateandhelpfulfeedbackastheuserlearnsthetoolandvice-versa.
Despitetheaccuracylimitations,itisnotablethatforalargepercentageofparticipantstheBCItooldidachieve itsintendedpurposeofpromotingmetacognitiveevaluationsandmodulatingtherangeofuncertainty.Someusers intentionallytriedtoaltertheAIfeedbackthroughtheirdesigndecisions,thususingitasamodeofdesignexploration, whileothersprimarilyreliedonitwhentheywerefacedwithuncertaintyorneededaconfidenceboost.Evenforthose participantswhoconsciouslydecidedagainsttheAIfeedback,thetoolappearedtopromptgreaterreflectionandgreater confidenceintheuser’sultimatedesignconclusions.Thosebehaviorsdemonstratethatuserswereencouragedtotake morerisks,evaluatethedesignoutcomes,andasaresultachievemorethought-outconfidenceintheirwork(Figure 10).Onenotableaspectoftheuserfeedbackwasthatparticipantsreportedawiderangeofindividualdifferencesin toolperformanceandinattitudestowardthetool.Designexperienceseemedtobearelevantfactorhere,withmore experienceddesignersbeinglesslikelytotrustorappreciatethetool’sfeedback.Itseemslikelythatthisisaresultof experienceddesignershavinggreaterconfidenceintheirdesignevaluations—possiblyduetohavingdevelopedmore effectiveintuitions,butalsopossiblyduetobeingmoregroundedintheircareersandmoreaccustomedtothepositive receptionoftheirwork.Inanycase,themorepositiveresponsereceivedfromnovicedesignerspositionsthetoolas havingaspecificpotentialutilityineducationalsettings.Theresearcherssuspectthatnaturalhumandiversityinterms ofneuralresponsesmayalsocontributetoindividualdifferencesinresponsetothetool.AsBCItechnologycontinues toexpandandimprove,itislikelythatwewilldiscoveradditionalcustomizationoptionsandmodalitiesforidentifying responsestodesignandforvisualizingthoseresponsesbacktousers.
ThefindingsofthisstudyadvancetheuseofBCIsinanoveldirection,takinganewstepintheapplicationofthis technologytocreativedesignwork.Whilethisworkisstillpreliminary,ithastremendouspotential,bothinenhancing thedesignprocessandinotherapplicationssuchastestingtheimpactofdesignparametersonuserresponses(e.g., designingalobbyspecificallyforrestorativepotential).Ifexpandedtomultiplesimultaneoususers,theMulti-Self approachcouldhelpdesignerstoidentifycollectiveresponsesandresponserangestomoreeffectivelyevaluateadesign acrossalargeruser-base.Theresultingdataabouttheimpactsofarchitecturaldesignfeaturescouldenhancethestate ofknowledgeofinfieldssuchasenvironmentalpsychologyandnumerousdesigncontexts(urbanexteriors,residential design,officedesign,videogamedesign,etc.).
5.1LimitationsandFutureWork
Despitethelargerangeofdesignoptionsthatwepresentedtoparticipantsinthisstudy,thelobbydesigntaskwasfar lesscomplexthantrulyopen-endedreal-worldscenarios.Itispossiblethatthissimplifiedtaskmayhavebeenunable toinciteahighdegreeofsubjectiveuncertainty,makingparticipantslessmotivatedtousetheBCItoolforguidance.In futureworkwewillseektoevaluatetheuseofthetoolinmorechallengingdesigncontexts,whichcanbedoneby expandingthedesignpossibilityspaceandsettingupcomplexdesignconstraints.
885 886 887 888 889 890 891 892 893 894 895 896 897 898 899 900 901 902 903 904 905 906 907 908 909 910 911 912 913 914 915 916 917 918 919 920 921 922 923 924 925 926 927 928 929 930 931 932 933 934 935 936
CHI’23,Hamburg,Germany,
Fig.10.DesignOutcomesSelectedbyParticipantsfromCo-DesignSessionswith"Multi-Self".
AnotherpossibilityforfutureworkinthisareaistoexpandbiofeedbackbeyondEEGdatatoincreaseprediction robustness,byintegratingmetricssuchasskinconductance,heartrate,andfacialexpressionrecognitionintothe classificationprocess.Itmayalsobebeneficialtovisualizethebiofeedbackinamoreintuitivefashion,forexample byreplacingthemovingdotonacoordinateplanewithambientfeedbacksuchashaptic,lighting,orsound.Some participantssuggestedusingamorepersonifiedvisualizationsuchasthephotooftheusertopresentthevisualization. Ratherthanhavingtoconsciouslytracksuchfeedback,asmootherassistivesystemcouldbedesignedtogentlynotify thedesignerwhenthereisapositiveornegativeresponse.
Interactionsbesidesbiofeedbacksystemshouldalsobeconsidered.Participantssuggestedenablingtheswitch betweenfirst-personandthird-personperspectives.Furthermore,thesystemcouldruninthebackstageandprovide participantswithemotionhistoryinsteadofrealtimenotification.
Finally,thepilotstudywaslimitedinthequantityanddiversityofparticipants.Manyofourparticipantswere experienceddesigners,whomighthaverelativelylowlevelsofuncertaintyorreducedneedforreflectiveassistance. Thoughweincludedsomenovicedesignersintooursample,futureuserstudiesorientedtowardindividualdifferences ofbothexpertandnovicedesignersmightoffermoreinsights,andwouldbeinlinewiththepositioningofMultiSelf asaneducationalorearly-careerfeedbacktool.Inthebroaderpicture,wearealsoconsideringtheuseofreflective designassistanceasameansofdemocratizingdesign,providingenhancedusercustomizabilityfordesignproducts, andencouraginghuman/AIcollaborationsthatcanbroadenthehorizonsofthefield.
REFERENCES
[1] RakefetAckermanandValerieAThompson.2017.Meta-reasoning:Monitoringandcontrolofthinkingandreasoning. Trendsincognitivesciences 21,8(2017),607–617.
[2] EricCAnderson,RNicholasCarleton,MichaelDiefenbach,andPaulKJHan.2019.Therelationshipbetweenuncertaintyandaffect. Frontiersin psychology 10(2019),2504.
[3] LindenJBallandBoTChristensen.2019.Advancinganunderstandingofdesigncognitionanddesignmetacognition:Progressandprospects. DesignStudies 65(2019),35–59.
[4] MaryamBanaei,AliAhmadi,KlausGramann,andJavadHatami.2020.Emotionalevaluationofarchitecturalinteriorformsbasedonpersonality differencesusingvirtualreality. FrontiersofArchitecturalResearch 9,1(2020),138–147.
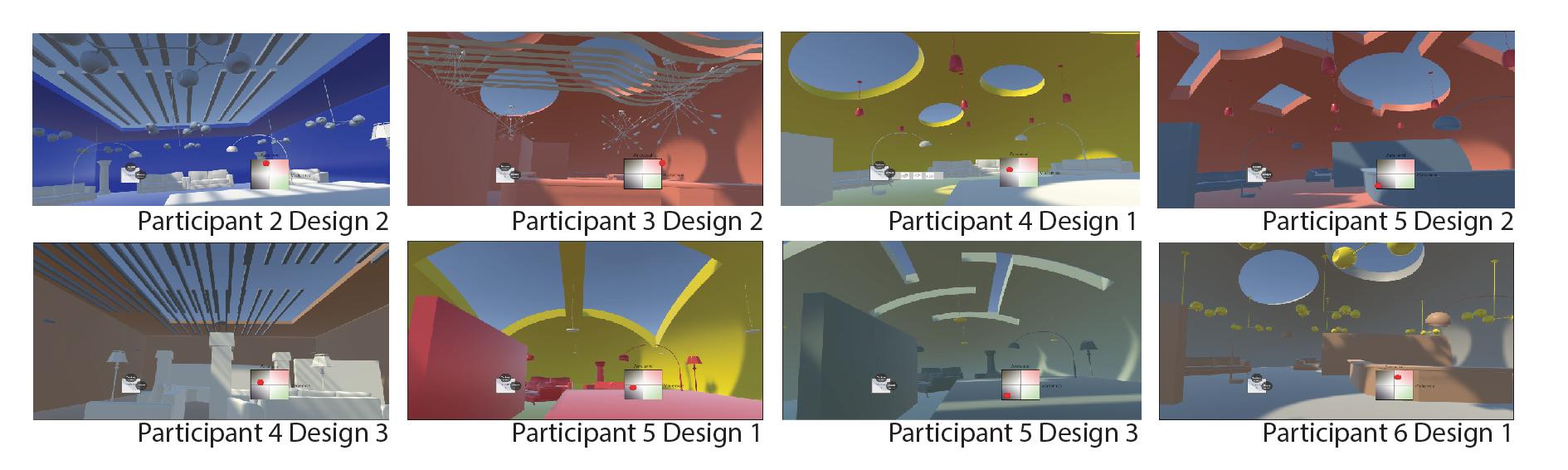
[5] Sung-WooByun,Seok-PilLee,andHyukSooHan.2017.Featureselectionandcomparisonfortheemotionrecognitionaccordingtomusiclistening. In 2017internationalconferenceonroboticsandautomationsciences(ICRAS).IEEE,172–176.
[6] SpencerECarlson,DanielGReesLewis,LeeshaVMaliakal,ElizabethMGerber,andMatthewWEasterday.2020.Thedesignrisksframework: Understandingmetacognitionforiteration. DesignStudies 70(2020),100961.
937 938 939 940 941 942 943 944 945 946 947 948 949 950 951 952 953 954 955 956 957 958 959 960 961 962 963 964 965 966 967 968 969 970 971 972 973 974 975 976 977 978 979 980 981 982 983 984 985 986 987 988
Co-DesignwithMyself:ABrain-ComputerInterfaceDesignToolthatPredictsLiveEmotiontoEnhanceMetacognitiveMonitoringofDesigners CHI’23,Hamburg,Germany,
[7]
[8]
AliceChirico,PietroCipresso,DavidBYaden,FedericaBiassoni,GiuseppeRiva,andAndreaGaggioli.2017.Effectivenessofimmersivevideosin inducingawe:anexperimentalstudy. Scientificreports 7,1(2017),1–11.
BoTChristensenandLindenJBall.2017.Fluctuatingepistemicuncertaintyinadesignteamasametacognitivedriverforcreativecognitive processes.In Analysingdesignthinking:Studiesofcross-culturalco-creation.CRCPress,249–269.
[9] AlexanderCoburn,OshinVartanian,YoedNKenett,MarcosNadal,FranziskaHartung,GregorHayn-Leichsenring,GorkaNavarrete,JoséL González-Mora,andAnjanChatterjee.2020.Psychologicalandneuralresponsestoarchitecturalinteriors. Cortex 126(2020),217–241.
[10] NathanCrillyandCarlosCardoso.2017.Wherenextforresearchonfixation,inspirationandcreativityindesign? DesignStudies 50(2017),1–38.
[11] HarshDabas,ChaitanyaSethi,ChiragDua,MohitDalawat,andDivyashikhaSethia.2018.EmotionclassificationusingEEGsignals.In Proceedings ofthe20182ndInternationalConferenceonComputerScienceandArtificialIntelligence.380–384.
[12]
AlwindeRooij,HannaSchraffenberger,andMathijsBontje.2018.Augmentedmetacognition:exploringpupildilationsonificationtoelicit metacognitiveawareness.In ProceedingsoftheTwelfthInternationalConferenceonTangible,Embedded,andEmbodiedInteraction.237–244.
[13] ArnaudDelorme,TimMullen,ChristianKothe,ZeynepAkalinAcar,NimaBigdely-Shamlo,AndreyVankov,andScottMakeig.2011.EEGLAB,SIFT, NFT,BCILAB,andERICA:newtoolsforadvancedEEGprocessing. Computationalintelligenceandneuroscience 2011(2011).
[14] AnastasiaEfklides.2006.Metacognitionandaffect:Whatcanmetacognitiveexperiencestellusaboutthelearningprocess? Educationalresearch review 1,1(2006),3–14.
[15] AnastasiaEfklides.2011.Interactionsofmetacognitionwithmotivationandaffectinself-regulatedlearning:TheMASRLmodel. Educational psychologist 46,1(2011),6–25.
[16] SatuEloandHelviKyngäs.2008.Thequalitativecontentanalysisprocess. Journalofadvancednursing 62,1(2008),107–115.
[17] SeymourEpstein.2010.Demystifyingintuition:Whatitis,whatitdoes,andhowitdoesit. PsychologicalInquiry 21,4(2010),295–312.
[18] JingFan,JoshuaWWade,AlexandraPKey,ZacharyEWarren,andNilanjanSarkar.2017.EEG-basedaffectandworkloadrecognitioninavirtual drivingenvironmentforASDintervention. IEEETransactionsonBiomedicalEngineering 65,1(2017),43–51.
[19] HaakonFaste.2017.IntuitioninDesign:ReflectionsontheIterativeAestheticsofForm..In CHI.3403–3413.
[20] JérémyFrey,GiladOstrin,MayGrabli,andJessicaRCauchard.2020.Physiologicallydrivenstorytelling:conceptandsoftwaretool.In Proceedings ofthe2020CHIConferenceonHumanFactorsinComputingSystems.1–13.
[21] FilipeGalvão,SoraiaMAlarcão,andManuelJFonseca.2021.PredictingexactvalenceandarousalvaluesfromEEG. Sensors 21,10(2021),3414.
[22] KailingGuo,JunmingHuang,YicaiYang,andXiangminXu.2019.EffectofvirtualrealityonfearemotionbaseonEEGsignalsanalysis.In 2019 IEEEMTT-SInternationalMicrowaveBiomedicalConference(IMBioC),Vol.1.IEEE,1–4.
[23] NavaHaghighiandArvindSatyanarayan.2020.Self-Interfaces:UtilizingReal-TimeBiofeedbackintheWildtoElicitSubconsciousBehaviorChange. In ProceedingsoftheFourteenthInternationalConferenceonTangible,Embedded,andEmbodiedInteraction.503–509.
[24] KentaHidaka,HaoyuQin,andJunKobayashi.2017.Preliminarytestofaffectivevirtualrealitysceneswithheadmountdisplayforemotion elicitationexperiment.In 201717thInternationalConferenceonControl,AutomationandSystems(ICCAS).IEEE,325–329.
[25] MarkoHorvat,MarkoDobrinić,MatejNovosel,andPetarJerčić.2018.AssessingemotionalresponsesinducedinvirtualrealityusingaconsumerEEG headset:Apreliminaryreport.In 201841stInternationalConventiononInformationandCommunicationTechnology,ElectronicsandMicroelectronics (MIPRO).IEEE,1006–1010.
[26] XinHu,JianwenYu,MengdiSong,ChunYu,FeiWang,PeiSun,DaifaWang,andDanZhang.2017.EEGcorrelatesoftenpositiveemotions. Frontiersinhumanneuroscience 11(2017),26.
[27] Yu-ChunHuangandChung-HayLuk.2015.HeartbeatJenga:abiofeedbackboardgametoimprovecoordinationandemotionalcontrol.In InternationalConferenceofDesign,UserExperience,andUsability.Springer,263–270.
[28] AngelHsing-ChiHwang.2022.TooLatetobeCreative?AI-EmpoweredToolsinCreativeProcesses.In CHIConferenceonHumanFactorsin ComputingSystemsExtendedAbstracts.1–9.
[29] RezaKhosrowabadiandAbdulWahabbinAbdulRahman.2010.ClassificationofEEGcorrelatesonemotionusingfeaturesfromGaussianmixtures ofEEGspectrogram.In Proceedingofthe3rdInternationalConferenceonInformationandCommunicationTechnologyfortheMoslemWorld(ICT4M) 2010.IEEE,E102–E107.
[30] AeleeKim,MinhaChang,YeseulChoi,SohyeonJeon,andKyoungminLee.2018.Theeffectofimmersiononemotionalresponsestofilmviewingin avirtualenvironment.In 2018IEEEConferenceonVirtualRealityand3DUserInterfaces(VR).IEEE,601–602.
[31] NishaVishnupantKimmatkarandVijayaBBabu.2018.HumanemotionclassificationfrombrainEEGsignalusingmultimodalapproachofclassifier. In Proceedingsofthe2018InternationalConferenceonIntelligentInformationTechnology.9–13.
[32] SanderKoelstra,ChristianMuhl,MohammadSoleymani,Jong-SeokLee,AshkanYazdani,TouradjEbrahimi,ThierryPun,AntonNijholt,and IoannisPatras.2011.Deap:Adatabaseforemotionanalysis;usingphysiologicalsignals. IEEEtransactionsonaffectivecomputing 3,1(2011),18–31.
[33] PeterJLang.1995.Theemotionprobe:Studiesofmotivationandattention. Americanpsychologist 50,5(1995),372.
[34] BettinaLaugwitz,TheoHeld,andMartinSchrepp.2008.Constructionandevaluationofauserexperiencequestionnaire.In Symposiumofthe AustrianHCIandusabilityengineeringgroup.Springer,63–76.
[35] RenLi,JaredSJohansen,HamadAhmed,ThomasVIlyevsky,RonnieBWilbur,HariMBharadwaj,andJeffreyMarkSiskind.2020.Theperilsand pitfallsofblockdesignforEEGclassificationexperiments. IEEETransactionsonPatternAnalysisandMachineIntelligence 43,1(2020),316–333.
[36] Tian-HaoLi,WeiLiu,Wei-LongZheng,andBao-LiangLu.2019.ClassificationoffiveemotionsfromEEGandeyemovementsignals:Discrimination abilityandstabilityovertime.In 20199thInternationalIEEE/EMBSConferenceonNeuralEngineering(NER).IEEE,607–610.
989 990 991 992 993 994 995 996 997 998 999 1000 1001 1002 1003 1004 1005 1006 1007 1008 1009 1010 1011 1012 1013 1014 1015 1016 1017 1018 1019 1020 1021 1022 1023 1024 1025 1026 1027 1028 1029 1030 1031 1032 1033 1034 1035 1036 1037 1038 1039 1040
CHI’23,Hamburg,Germany, Anon.
[37]
JavierMarín-Morales,JuanLuisHiguera-Trujillo,AlbertoGreco,JaimeGuixeres,CarmenLlinares,EnzoPasqualeScilingo,MarianoAlcañiz,and GaetanoValenza.2018.Affectivecomputinginvirtualreality:emotionrecognitionfrombrainandheartbeatdynamicsusingwearablesensors. Scientificreports 8,1(2018),1–15.
[38] SusanVMcLarenandKayStables.2008.Exploringkeydiscriminatorsofprogression:Relationshipsbetweenattitude,meta-cognitionand performanceofnovicedesignersatatimeoftransition. DesignStudies 29,2(2008),181–201.
[39] AlbertMehrabianandJamesARussell.1974.Thebasicemotionalimpactofenvironments. Perceptualandmotorskills 38,1(1974),283–301.
[40] MarkRomanMiller,FernandaHerrera,HanseulJun,JamesALanday,andJeremyNBailenson.2020.Personalidentifiabilityofusertrackingdata duringobservationof360-degreeVRvideo. ScientificReports 10,1(2020),1–10.
[41] LennartErikNacke,MichaelKalyn,CalvinLough,andReganLeeMandryk.2011.Biofeedbackgamedesign:usingdirectandindirectphysiological controltoenhancegameinteraction.In ProceedingsoftheSIGCHIconferenceonhumanfactorsincomputingsystems.103–112.
[42] RabNawaz,HumairaNisar,andVooiVoonYap.2018.Recognitionofusefulmusicforemotionenhancementbasedondimensionalmodel.In 2018 2ndInternationalConferenceonBioSignalAnalysis,ProcessingandSystems(ICBAPS).IEEE,176–180.
[43] RaymondSNickerson.1999.Enhancingcreativity.(1999).
[44] DanNie,Xiao-WeiWang,Li-ChenShi,andBao-LiangLu.2011.EEG-basedemotionrecognitionduringwatchingmovies.In 20115thInternational IEEE/EMBSConferenceonNeuralEngineering.IEEE,667–670.
[45] SusannahBFPaletz,JoelChan,andChristianDSchunn.2017.Thedynamicsofmicro-conflictsanduncertaintyinsuccessfulandunsuccessful designteams. DesignStudies 50(2017),39–69.
[46] HanchuanPeng,FuhuiLong,andChrisDing.2005.Featureselectionbasedonmutualinformationcriteriaofmax-dependency,max-relevance,and min-redundancy. IEEETransactionsonpatternanalysisandmachineintelligence 27,8(2005),1226–1238.
[47] MirjanaPrpa,EkaterinaRStepanova,TheclaSchiphorst,BernhardERiecke,andPhilippePasquier.2020.Inhalingandexhaling:Howtechnologies canperceptuallyextendourbreathawareness.In Proceedingsofthe2020CHIConferenceonHumanFactorsinComputingSystems.1–15.
[48] RogelioPuente-Díaz,JudithCavazos-Arroyo,andFernandaVargas-Barrera.2021.Metacognitivefeelingsasasourceofinformationintheevaluation andselectionofcreativeideas. Thinkingskillsandcreativity 39(2021),100767.
[49] YannRenard,FabienLotte,GuillaumeGibert,MarcoCongedo,EmmanuelMaby,VincentDelannoy,OlivierBertrand,andAnatoleLécuyer.2010. Openvibe:Anopen-sourcesoftwareplatformtodesign,test,andusebrain–computerinterfacesinrealandvirtualenvironments. Presence 19,1 (2010),35–53.
[50] JoanSolRoo,RenaudGervais,andMartinHachet.2016.Innergarden:Anaugmentedsandboxdesignedforself-reflection.In Proceedingsofthe TEI’16:TenthInternationalConferenceonTangible,Embedded,andEmbodiedInteraction.570–576.
[51] MartinSchrepp,JorgThomaschewski,andAndreasHinderks.2017.Constructionofabenchmarkfortheuserexperiencequestionnaire(UEQ). (2017).
[52] AliceSchut,MaartenvanMechelen,RemkeMKlapwijk,MathieuGielen,andMarcJdeVries.2020.Towardsconstructivedesignfeedbackdialogues: guidingpeerandclientfeedbacktostimulatechildren’screativethinking. InternationalJournalofTechnologyandDesignEducation (2020),1–29.
[53] NathanSemertzidis,MichaelaScary,JoshAndres,BrahmiDwivedi,YutikaChandrashekharKulwe,FabioZambetta,andFlorianFloydMueller.2020. Neo-Noumena:AugmentingEmotionCommunication.In Proceedingsofthe2020CHIconferenceonhumanfactorsincomputingsystems.1–13.
[54] NathanArthurSemertzidis,BettySargeant,JustinDwyer,FlorianFloydMueller,andFabioZambetta.2019.Towardsunderstandingthedesignof positivepre-sleepthroughaneurofeedbackartisticexperience.In Proceedingsofthe2019CHIConferenceonHumanFactorsinComputingSystems 1–14.
[55] CeliaShahnaz,SMShafiulHasan,etal 2016.EmotionrecognitionbasedonwaveletanalysisofEmpiricalModeDecomposedEEGsignalsresponsive tomusicvideos.In 2016IEEERegion10Conference(TENCON).IEEE,424–427.
[56] AvishagShemesh,GerryLeisman,MosheBar,andYashaJacobGrobman.2021.Aneurocognitivestudyoftheemotionalimpactofgeometrical criteriaofarchitecturalspace. ArchitecturalScienceReview 64,4(2021),394–407.
[57] TengfeiSong,WenmingZheng,PengSong,andZhenCui.2018.EEGemotionrecognitionusingdynamicalgraphconvolutionalneuralnetworks. IEEETransactionsonAffectiveComputing 11,3(2018),532–541.
[58]
NazmiSofianSuhaimi,JamesMountstephens,JasonTeo,etal 2020.EEG-basedemotionrecognition:Astate-of-the-artreviewofcurrenttrends andopportunities. Computationalintelligenceandneuroscience 2020(2020).
[59] NazmiSofianSuhaimi,ChrystalleTanBihYuan,JasonTeo,andJamesMountstephens.2018.Modelingtheaffectivespaceof360virtualreality videosbasedonarousalandvalenceforwearableEEG-basedVRemotionclassification.In 2018IEEE14thInternationalColloquiumonSignal Processing&ItsApplications(CSPA).IEEE,167–172.
[60] NaotoTerasawa,HirokiTanaka,SakrianiSakti,andSatoshiNakamura.2017.Trackinglikingstateinbrainactivitywhilewatchingmultiplemovies. In Proceedingsofthe19thACMinternationalconferenceonmultimodalinteraction.321–325.
[61] HabibUllah,MuhammadUzair,ArifMahmood,MohibUllah,SultanDaudKhan,andFaouziAlayaCheikh.2019.Internalemotionclassification usingEEGsignalwithsparsediscriminativeensemble. IEEEAccess 7(2019),40144–40153.
[62] OshinVartanian,GorkaNavarrete,AnjanChatterjee,LarsBrorsonFich,JoseLuisGonzalez-Mora,HelmutLeder,CristiánModroño,MarcosNadal, NicolaiRostrup,andMartinSkov.2015.Architecturaldesignandthebrain:Effectsofceilingheightandperceivedenclosureonbeautyjudgments andapproach-avoidancedecisions. Journalofenvironmentalpsychology 41(2015),10–18.
1041 1042 1043 1044 1045 1046 1047 1048 1049 1050 1051 1052 1053 1054 1055 1056 1057 1058 1059 1060 1061 1062 1063 1064 1065 1066 1067 1068 1069 1070 1071 1072 1073 1074 1075 1076 1077 1078 1079 1080 1081 1082 1083 1084 1085 1086 1087 1088 1089 1090 1091 1092
Co-DesignwithMyself:ABrain-ComputerInterfaceDesignToolthatPredictsLiveEmotiontoEnhanceMetacognitiveMonitoringofDesigners CHI’23,Hamburg,Germany,
[63] ItsaraWichakamandPeeraponVateekul.2014.AnevaluationoffeatureextractioninEEG-basedemotionpredictionwithsupportvectormachines. In 201411thinternationaljointconferenceoncomputerscienceandsoftwareengineering(JCSSE).IEEE,106–110.
[64] ShiyiWu,XiangminXu,LinShu,andBinHu.2017.EstimationofvalenceofemotionusingtwofrontalEEGchannels.In 2017IEEEinternational conferenceonbioinformaticsandbiomedicine(BIBM).IEEE,1127–1130.
[65] JinghanXu,FujiRen,andYanweiBao.2018.EEGemotionclassificationbasedonbaselinestrategy.In 20185thIEEEInternationalConferenceon CloudComputingandIntelligenceSystems(CCIS).IEEE,43–46.
[66] BinYu,NienkeBongers,AlissaVanAsseldonk,JunHu,MathiasFunk,andLoeFeijs.2016.LivingSurface:biofeedbackthroughshape-changing display.In ProceedingsoftheTEI’16:TenthInternationalConferenceonTangible,Embedded,andEmbodiedInteraction.168–175.
[67] WenzhuoZhang,LinShu,XiangminXu,andDanLiao.2017.Affectivevirtualrealitysystem(AVRS):designandratingsofaffectiveVRscenes.In 2017InternationalConferenceonVirtualRealityandVisualization(ICVRV).IEEE,311–314.