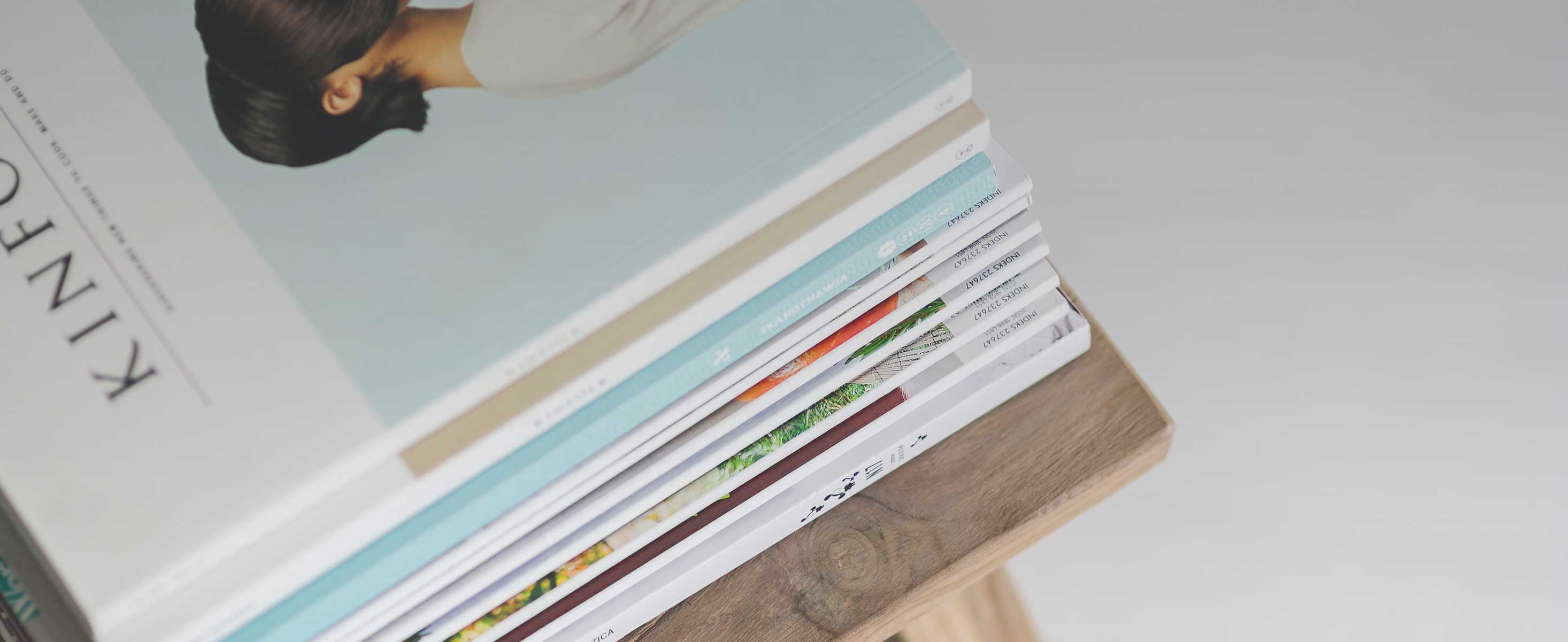
4 minute read
The rapid deployment of new technologies
Automated decision making (ADM): ADM is the process of making a decision by automated means without any human involvement. Decisions can be made based on factual data or inferred data. An example is a system counting correct answers on a multiple choice test sheet and then attributing a pass or fail mark based on the number of correct answers. It could also include an online decision to award a loan or provide a quote for the cost of a service.5 Data analysis: combining data from a variety of sources and applying organisational, statistical, mathematical or machine learning calculations to retrieve data, find a pattern, produce a conclusion or make a prediction. Machine learning (ML): ML is a branch of AI that allows a system to learn and improve from examples without all its instructions being explicitly programmed. ML systems are provided with large volumes of different categories of data, and identify patterns that distinguish one category from another. Thus, they ‘learn’ to process future data, extrapolating its knowledge to unfamiliar situations. Applications of ML include virtual assistants (such as ‘Alexa’), recommender systems, and facial recognition.6 Technological solution: a method by which data, digital software tools, AI and/or new technologies can be used (fully or partially) to provide a service, provide information, undertake a task, make a decision or change the way something is done.
The rapid deployment of new technologies 3. When we began our inquiry, we were aware of several technological solutions used in the application of the law. We had heard of ‘predictive policing’ which we understood as the use of historic data to predict where and when certain types of crime may be more likely to occur, and using those predictions to plan policing priorities and strategies. We were also aware of visa streaming algorithms—processing tools used by visa-issuing authorities to triage applications and decide which should be investigated. The use of facial recognition was of concern to us as well, especially in relation to privacy rights and risks of discrimination which have been widely reported.7
Advertisement
4. Written evidence from Dr Miri Zilka, Dr Adrian Weller and Detective
Sergeant Laurence Cartwright laid out some categories of tools used in the justice system. While our scope is wider, most of the tools we heard of fit into these categories:
(a) Data infrastructure: software and tools primarily used to record, store, organise, and search data.
5 Information Commissioner’s Office, ‘What is automated individual decision-making and profiling?’: https://ico.org.uk/for-organisations/guide-to-data-protection/guide-to-the-general-data-protectionregulation-gdpr/automated-decision-making-and-profiling/what-is-automated-individual-decisionmaking-and-profiling/ [accessed 6 February 2022] 6 The Royal Society, Machine learning: the power and promise of computers that learn by example (April 2017): https://royalsociety.org/~/media/policy/projects/machine-learning/publications/machine-learn ing-report.pdf [accessed 6 February 2022] 7 ‘UK’s facial recognition technology ‘breaches privacy rights’’, The Guardian (23 June 2020): https:// www.theguardian.com/technology/2020/jun/23/uks-facial-recognition-technology-breaches-privacyrights [accessed 6 February 2022]. Also see Harvard University, The Graduate School of Arts and
Sciences, ‘Racial Discrimination in Facial Recognition Technology’ (24 October 2020): https:// sitn.hms.harvard.edu/flash/2020/racial-discrimination-in-face-recognition-technology/ [accessed 6
February 2022].
(b) Data analysis: software and tools primarily used to analyse data to create insights.
(c) Risk prediction: software and tools primarily used for predicting future risk based on analysis of past data. This category can include predictions on an individual level (whether an individual poses a risk), or on a wider societal level (when and where a risk is higher).8
5. In the course of our inquiry, it became apparent that new technologies are used by a wide range of agencies to achieve a wide range of different purposes.
We heard the most about tools used by the Home Office, the Ministry of
Justice, HM Prisons and Probation Service, and individual police forces.
They were being used for a variety of purposes, of which the following are examples:
• To provide efficiency. Eastern region police bodies have created a ‘bot’ to run procedural checks on vetting enquiries, passing key information to an officer for assessment and decision.9
• To provide new insights. Qlik Sense is a tool used by Avon and
Somerset Police, which presents data in an interactive way. A police officer can see an increasing crime trend in their area, and find out quickly what crime types are driving that increase, along with specifics of the relevant offence.10
• To process large volumes of material. The Serious Fraud Office has used machine learning to pre-screen “several thousands of documents”, thereby “saving up to two years and significant costs” compared to manual processing.11
• To screen documents. The Home Office uses an algorithm to help review applications for marriage licences. The tool can review applications and raise flags in the system to assist in launching investigations into a potential ‘sham marriage’.12
• To provide risk assessments. Durham Constabulary has been using a
Harm Assessment Risk Tool (HART), which uses data analytics and machine learning to provide a prediction of how likely an individual is to commit a violent or non-violent offence over the next two years.13
• To identify people. Automated facial recognition uses algorithmic technology to recognise people from pictures.
• To draw conclusions about people’s emotions or deceptiveness. We were told, for example, about the use of polygraphs to monitor sex offenders on parole and manage their level of compliance with parole conditions.14
8 Written evidence from Dr Miri Zilka, Detective Sergeant Laurence Cartwright and Dr Adrian Weller (nTL0040) 9 Written evidence from Association of Police and Crime Commissioners, national Police Chiefs’
Council and Police Digital Service (nTL0049) 10 Written evidence from Avon and Somerset Police (nTL0052) 11 Written evidence from the Serious Fraud Office (nTL0034) 12 Written evidence from Public Law Project (nTL0046) 13 Written evidence from Liberty (nTL0020) 14 Written evidence from Dr Kyriakos n Kotsoglou (nTL0007)