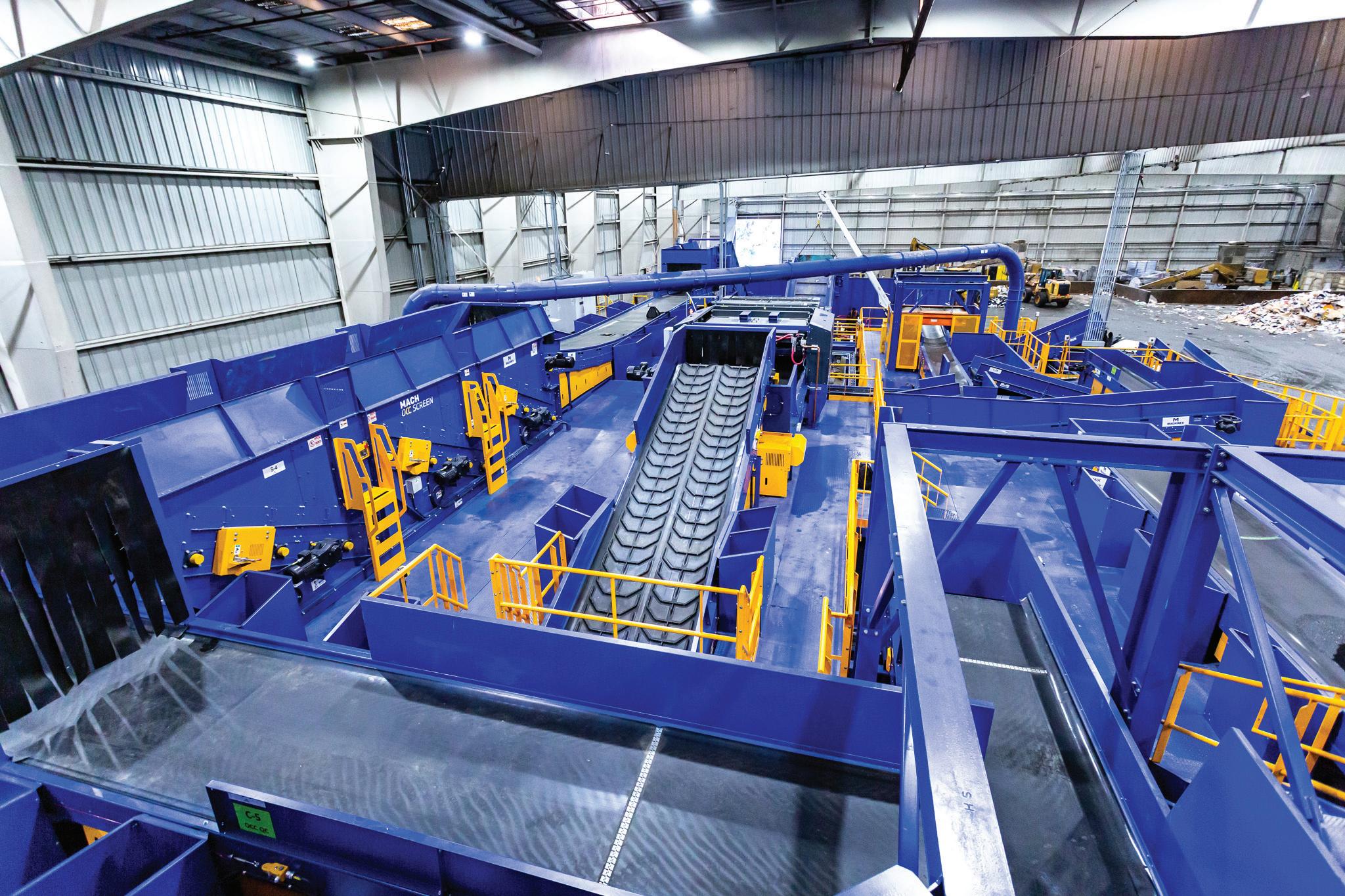
5 minute read
AI, DATA CAPTURE, AND THE DEVELOPMENT OF THE INTELLIGENT MRF
VISION EQUIPMENT GIVES OPERATORS THE INFORMATION NEEDED TO ENSURE PROFITABLE AND EFFICIENT OPERATIONS
Within the recycling and waste industry, artificial intelligence (AI) and data capture are increasingly seen as the future for the intelligent MRF, taking the industry to a new level of efficiency. Today’s intelligent MRFs are made up of various pieces of vision equipment such as optics, robots, or simple cameras to capture data throughout the system and provide supervisory control and data acquisition (SCADA) systems with the information needed to ensure profitable and efficient operations.
Traditionally, the role of an operator at a materials recovery facility was simply to manage the flow of materials. As the industry continued to develop and progress over time, equipment reliability, facility throughput, operational hours, and output quality became the main driving factors behind the develop- ment of smarter systems that provide reliable data to accommodate these needs. This has since evolved to include even more benefits for MRFs, such as increased recycling rates, the ability to recycle more types of materials, and sustainability initiatives.
These changes can be attributed to three main factors:
Environmental benefits reducing the amount of waste that goes into landfills helps conserve natural resources, conserves energy, and reduces greenhouse gas emissions and pollution.
Economic benefits MRFs save money by reducing costs associated with waste disposal and generate increased revenue from the sale of recycled materials.
Social benefits creating jobs and supporting local economies promotes a culture of sustainability and environmental responsibility.
Working Toward An Intelligent Mrf
MRFs – whether for material recovery or for waste-to-energy purposes – are now very much part of modern industry. The goal of the intelligent MRF is to produce valuable, high-quality output materials that can then be used in ongoing manufacturing processes to help reduce reliance on foreign markets while also meeting sustainability goals put in place by both local authorities and waste management companies.
While AI brings the industry one step closer to that goal, there’s still more to do. The next step in the process includes using a vision system at numerous locations within a sorting system to continually assess the volume and composition of the materials as they travel through the system, both before and after the sorting processes. This allows the equipment within the system to be controlled and adjusted in real-time, resulting in optimal performance for the entire facility. In a way, it’s like having several plant managers constantly looking at different areas of the facility and comparing what they see.
There are many benefits of a technology-reliant system, and according to Jeff Snyder, director of recycling at Rumpke Waste & Recycling, the technology will also dramatically reduce the reliance on a manual labour workforce.
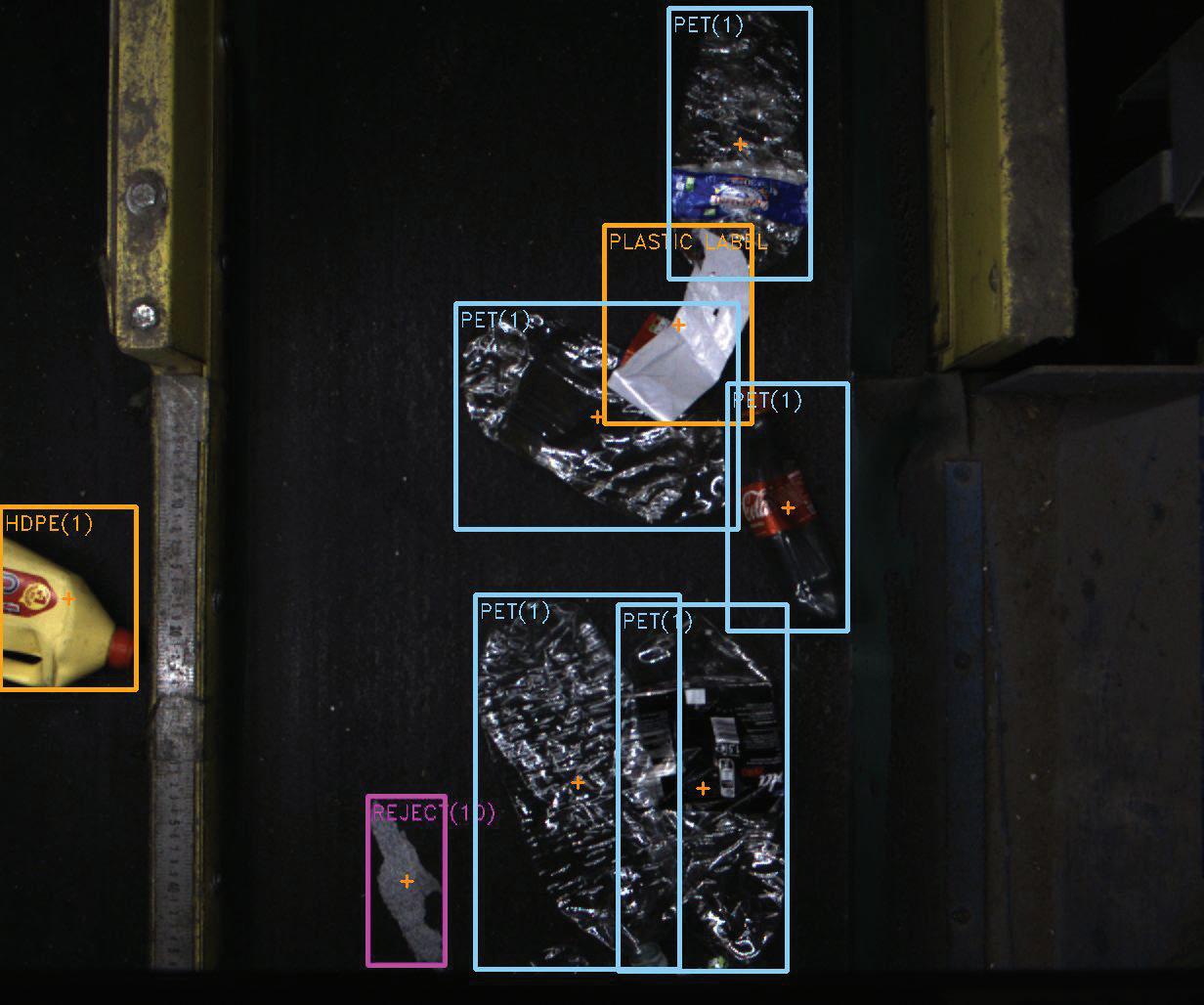
“We purchased a system with a guaranteed throughput of 56 tons per hour of recyclables, with staffing ranging from a minimum of 10 to a maximum of 20 manual sorters, depending on the inbound material characteristics and commodity focus,” says Snyder. “The intelligence of this system significantly minimizes factors such as worker fatigue, safety risks, and illness, while providing present and future flexibility enabling us to keep the highest rate of recovery and extremely high purity.”
After a competitive bidding process run by Rumpke, plant-building company Machinex, based in Plessisville, Quebec, won the contract to supply and install the processing equipment for the plant. Machinex supplied 19 optical sorters that are completing a total of 35 tasks through various ejection patterns at the facility. According to Chris Hawn, CEO of Machinex, the combination of optics and AI throughout the system will offer increased levels of accuracy and efficiency compared to traditional MRFs, and combining high-tech equipment with traditional sorting technologies can offer further benefits.
“Principally, the output you get is much higher in recov- ery and purity,” says Hawn. “This makes the recyclate more attractive to markets and attracts a higher price tag. This is very important in terms of making the project cost-efficient and therefore achieving the projected ROI.”
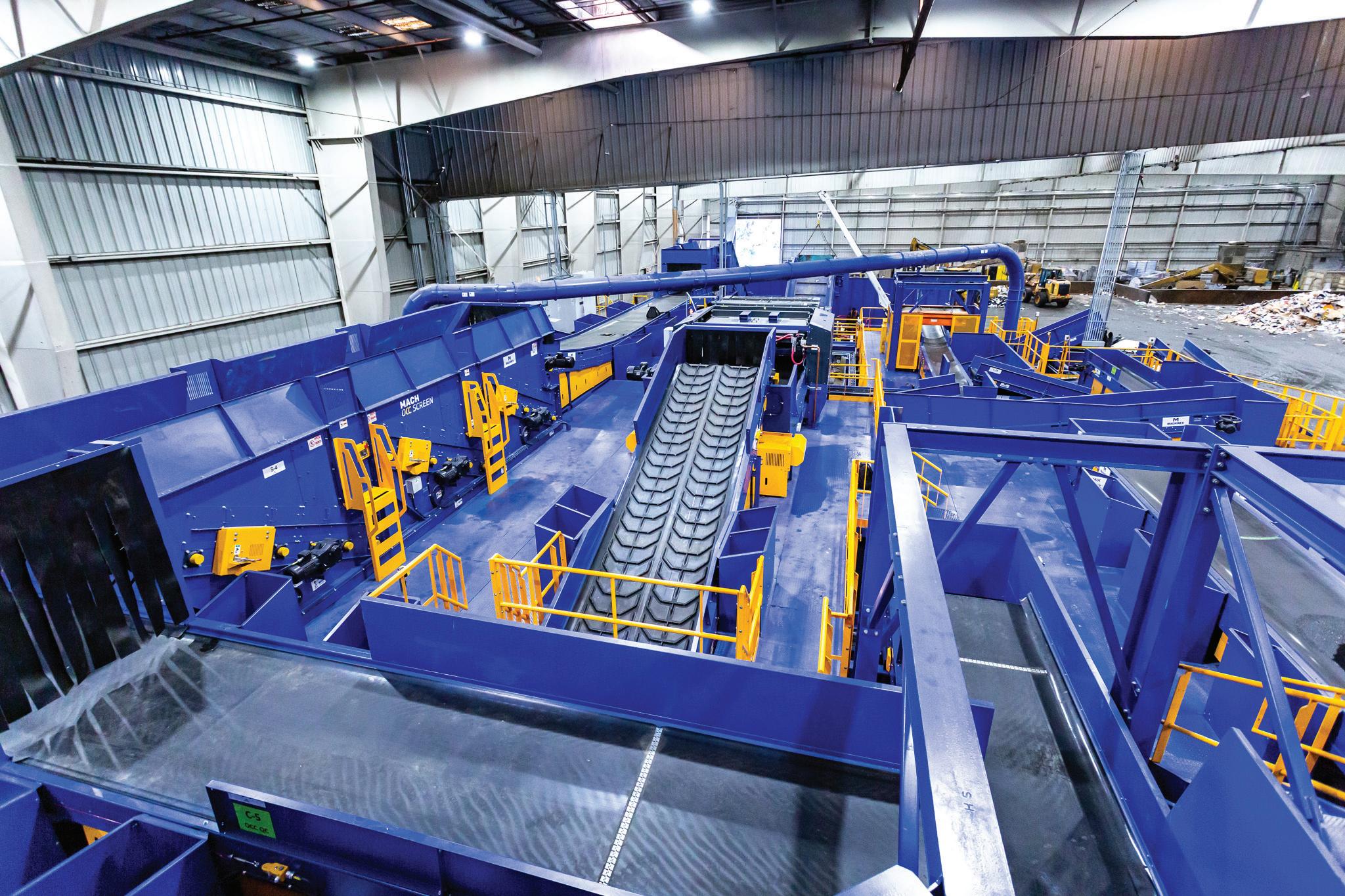
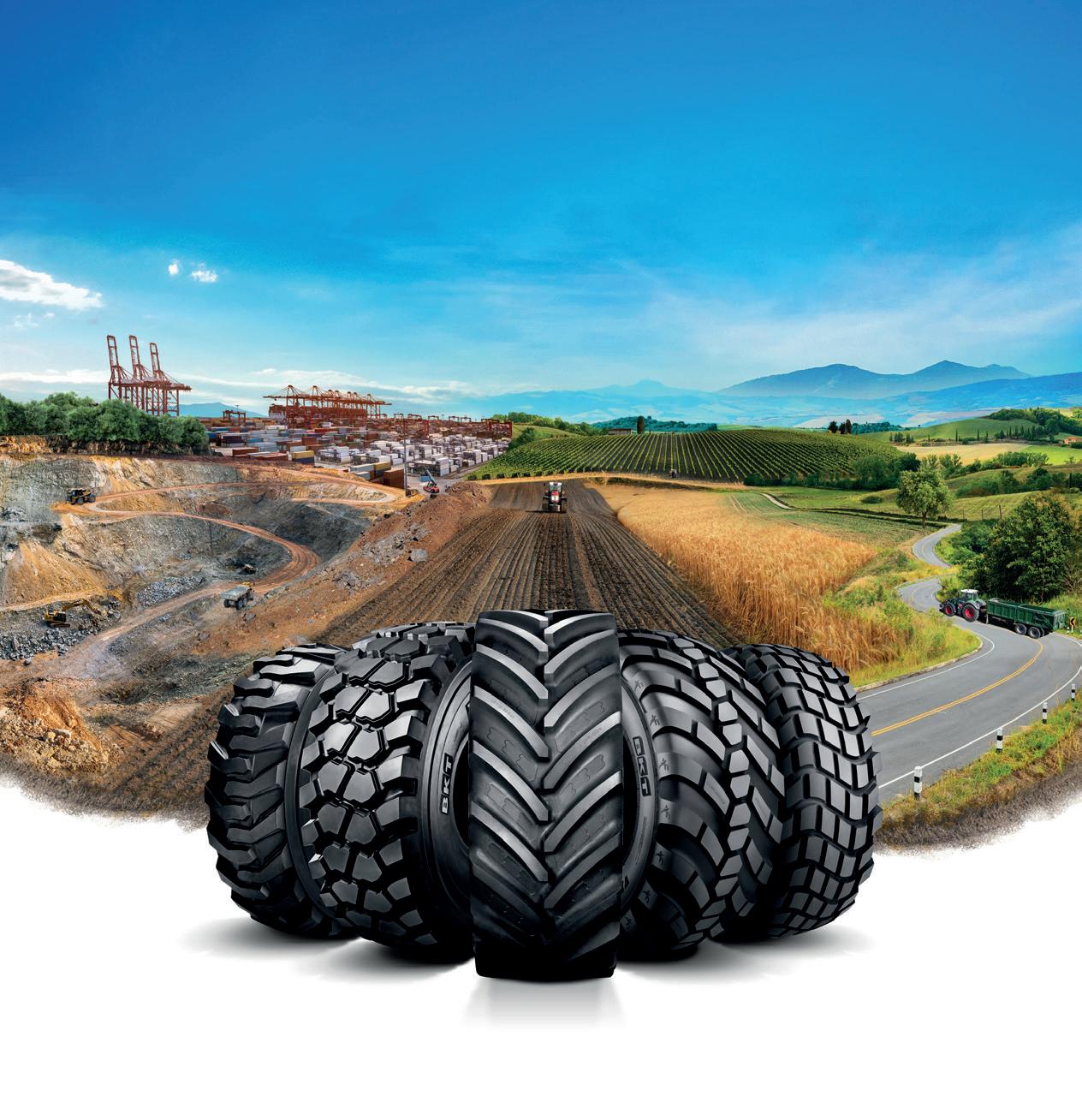
Data Capture As A Starting Point
When building an intelligent MRF, the first step is to capture data through the use of high-tech sorting equipment. Machinex offers a range of equipment for this purpose, such as the Mach Hyspec optical sorter, the SamurAI sorting robot, and Mach Vision. An optical sorter features a hyperspectral camera to identify the actual material (i.e., clear PET bottles), allowing for high-performance material sorting. While this equipment is meant for sorting, it’s also an entry point for data collection to optimize the MRF as a whole. Machinex’s Mach Intell platform is able to detect information such as infeed material, material composition breakdown, belt coverage, and more. This information is ideal when it comes to monitoring the performance of the optical sorter by itself, but can have an even greater impact when this data is analyzed in tandem with data from other equipment within the facility.
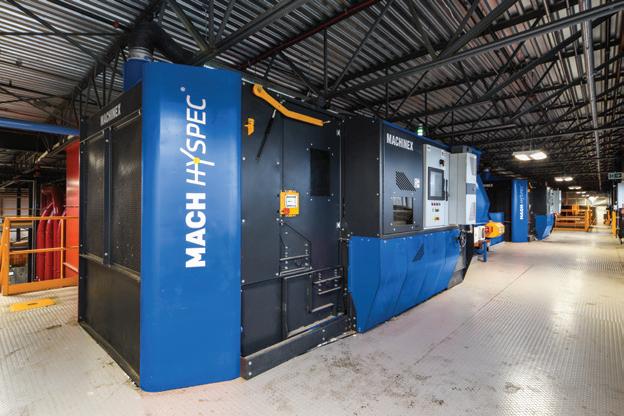
When discussing data collection, sorting robots often come up early in the conversation. These robots are powered by AI to identify materials for accurate, positive product recovery, or a precise quality control function. For Machinex, the SamurAI sorting robot can integrate the Mach Intell platform, gaining access to data such as material coming in and out of the robot, material breakdown, ejects, and more. Even though a sorting robot can be useful in accomplishing tasks, it is not required in order to capture data. A vision system, such as a Mach Vision, is able to collect valuable information within the sorting system and provide needed insight.
Data capture is key for improving system and facility performance, but information alone does not create an intelligent MRF. It’s what is done with the data that makes a difference. The core of the intelligent MRF is filtering and analyzing all of the data through a “brain” and automatically making adjustments to the sorting equipment across the facility. The different capture points are then connected to a SCADA system for further compilation and analysis.
Neural Network Development
Using high-tech equipment such as AI-powered robotic sorters and vision systems within a MRF environment facilitates the reduction of manual input when it comes to labelling materials. Labelling materials is an ongoing and necessary process that helps build a library of which materials are in certain locations, a key component of a neural network. Machinex has developed its approach to automatically label material rather than rely solely on manual labelling. This autonomous technology maximizes efficiency when developing a neural network.
A neural network for image classification is a deep learning model designed to learn and recognize patterns in images. This model consists of multiple layers of interconnected neurons – much like how a human brain is structured – that process image data and calculate the probability of different variables such as recovery, quality control, and refund operations.
To achieve ultimate AI performance, operators can collect data prior to deployment and train the equipment with the same materials found in the facility. This also allows operators to create a breakdown of materials within the system and pinpoint the ideal solution for efficient sorting. The material information gathered at this point also allows operators to have a model in place for the day the AI sorting is turned on, giving instant results.
Taking into account the continuous evolution of incoming material in MRFs, the neural network can evolve in parallel through assisted learning. Data extracted from the various technologies within the MRF, or even at the curb, will open doors that haven’t even been considered yet. In today’s environment, data such as material composition at various locations in the MRF and automated picks per minute can improve system efficiency, material purity, and material recovery, but further solutions need to be developed to ensure that there will be a notable impact within the system. It’s possible to capture an infinite amount of data, but without equipment connectivity, there will be no real improvement in the end.
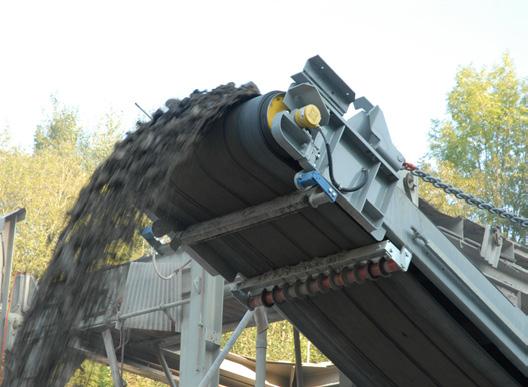