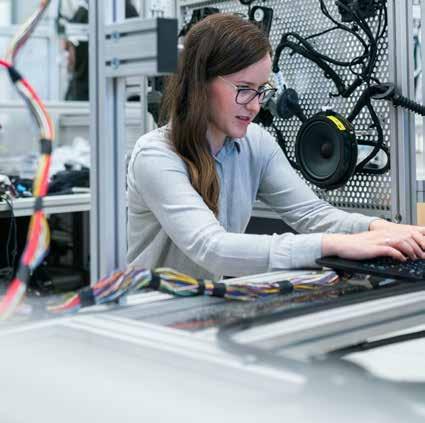
7 minute read
How predictive biometrics systems are changing the mining industry for the better
from BBMC Yearbook 2020
by bbminingclub
Alex Moss, CEO, Canaria Technologies
One of the most exciting outcomes of the mining sector implementing Industry 4.0 is that it opens the door to completely new species of technologies.
Industry 4.0 is usually only associated with drones, blockchain and asset management, and while this is true, the most interesting part of this shift is simply the move towards consistent high-speed internet access. This allows emerging technologies in sectors not traditionally associated with mining to find new life-solving problems that have plagued the industry for decades: technologies like Predictive Biometrics Systems.
What are predictive biometrics systems?
You have probably never read the term ‘predictive biometrics system’ before, which is understandable because even within its homestead of the medical sciences it is still relatively unknown.
But what happens when we make the leap from being able to measure someone’s vital signs to being able to predict disruptions in the patterns of their vital signs data?
What if it were possible to use these patterns to know that someone was at risk of a heart attack, 10 minutes before it happened?
What if we could alert a user of their fatigue risk prior to an issue arising? 70% of all accidents in the heavy industries are caused by cognitive fatigue and in 2019, cognitive fatigue was cited as the underlying cause of 144 fatalities in Australia, where 43, or more than 30%, were machinery operators and drivers.
Developing these self-learning systems which tailor themselves to every person’s individual biometric thresholds becomes a valid field of study and although this may all sound fantastical, not only do these technologies exist; they have been being quietly piloted and refined in the mining industry for the past two years.
How do predictive biometric systems work?
In order to understand predictive biometrics systems, we first need to clarify what ‘biometric’ data is. Any data that is gathered about the way the human body functions can be described as biometric.
The best-known types are:
• fingerprint and iris scans
• electroencephalography (EEG) readings, taken using skullcaps in hospitals and research institutes
• electrocardiogram (ECG) readings where pads are placed on the chest to measure electrical heart activity
• pulse plethysmography (PPG) which measures oxygen levels in the blood taken from the wrist, finger or ear
What makes biometric systems predictive is the ability to make the leap from recognising a pattern in data, to being able to predict the next step in that pattern.
Predictive biometrics systems usually consist of:
• a digital monitoring device (such as a miniature electronic vital signs monitor or core body temperature monitor)
• a processing device such as a smartphone (though highly advanced systems may feature some computing power on the monitors themselves)
• a cloud data-transfer infrastructure connecting to a powerful computer network capable of running machine learning techniques to maintain self-learning systems.
The most widely-used examples of predictive biometrics systems consist of Medtronic’s MiniMed 770g system for predicting glucose levels in diabetic patients and automatically adjusting the levels of insulin delivered to the user in real-time, and a company called Natural Cycles which has developed a system that predicts women’s fertility cycles based on core body temperature.
Predictive biometric systems follow the same principles as most other datacentric predictive systems, requiring large amounts of highly accurate historic data, computer-heavy machine learning techniques and high-speed internet connections to function properly.
Whereas the software capabilities and mathematical models used in these cases have been up to the task of prediction for a while; it is the recent breakthroughs in micro-computing hardware which has facilitated the recent emergence of predictive biometric systems, thanks to battery miniaturisation.
Along with deeper understandings of how biometrics such as heart rate variability and core body temperature reflect physiological conditions, this new field can be used proactively to solve human performance and safety issues. Predictive biometrics systems are generally anchored to one core biometric (similar to Natural Cycles’ use of core body temperature), however more and more are utilising the interplay of multiple metrics simultaneously.
One example is the work of the University of Oxford in investigating multiple vital signs from wrist and ear-based monitors including heart rate variability, blood oxygen levels and human movement.
Why aren’t predictive biometrics systems already common?
The use cases for building predictive biometric systems are obvious. With the potential for machine learning and deep learning techniques, combined with advances in edge computing, why aren’t predictive biometric systems a common part of every-day life yet?
Although in theory it’s (relatively) easy to apply AI to data about the human body to create predictive systems, there simply isn’t enough high accuracy biometric data in existence yet with which to build accurate models. The great difficulty is that very little medical data is accessible to researchers and coders, and those that are, are often sporadic and inaccurate. In addition, a surprising majority of medical data is still recorded by hand. On the other hand, there is plenty of consumer grade biometric data around (courtesy of Apple Watch and Fitbit), however this data is too inaccurate to build successful AI systems with.
Even as little as 2% inaccuracy in vital signs readings can have a compound effect when fed into a neural network which can lead to a system that is 20% inaccurate in its predictions. This level of inaccuracy simply isn’t appropriate for use in life-or-death situations. This is why many companies have moved to make their own high-accuracy monitors to get to medical levels of accuracy (~99%), so they're able to get the ball rolling on systems for solving high-stakes problems.
How predictive biometrics could change the mining industry
To date, predictive biometrics have mainly been used in medicine, and in a somewhat experimental capacity. These systems are particularly useful in mining applications because the working environments are more extreme than, say, office-based work. There's a much higher mortality rate and higher asset damage costs if a worker passes out whilst operating machinery due to fatigue. Sites are also more remote, so it's harder to access medical facilities when accidents do happen, meaning there's an extra incentive to make sure people never get past their physical limits in the first place.
Predictive biometrics mean the difference between getting a phone call that your colleague has just had a microsleep or knowing that your colleague is 10 minutes away from having a microsleep. It also means that, although you can implement predictive biometrics based on group metrics, the alerts can be individualised for each user.
As technologies become more automated over the next few years, especially with the rise of driverless vehicles, predictive biometric systems will ensure that remote operators work in optimal physical conditions to prevent costly supply chain mistakes. This is particularly important in a post-drone landscape where operators are likely to work in confined, dark conditions for long periods of time. In the short-term, there are issues in mining which can be solved today like cognitive fatigue, heat exhaustion, man-down incidents and asphyxiation prediction - problems that are perfect for the use of predictive biometrics systems.
Perhaps most topically, these systems may also be used to solve critical ready-for-work assessments such as whether someone is showing symptoms of viral infection via elevated pulse, breathing, body temperature and blood oxygen levels. However, as such implementations become more the norm, systems already in use in the field may utilise software updates to produce additional diagnostics capabilities into pre-existing predictive HSSE applications.
The five-year horizon sees predictive biometrics systems becoming multifaceted as standard, fully integrated into mining operations. The live data from these systems should also update into mine management software and synchronise with emergency response off-site, such as hospitals. These systems can continually optimise workforces in a way that improves safety and productivity, whilst allowing workers more time off.
From an operations and financial view, an emerging field allows the adoption of business models from other industries to help streamline the adoption cycle. To date, a subscription approach has been used most successfully for consumer software, and it makes sense for predictive biometrics approaches in the mining industry as it keeps both CAPEX and OPEX expenditure minimal.
\Another interesting financial advantage is that, as the benefits of this new type of risk mitigation are quantified, we can expect insurance companies to offer reduced rates to companies who are early adopters. The advent of predictive biometrics systems opens up a whole new world of opportunity to establish a point of truth by collecting and analysing data. This new species of technology enables life-threatening safety incidents to be predicted before they happen, whilst the individualisation for each person allows for optimised rostering. What this means for the mining industry is that workers go home safely.