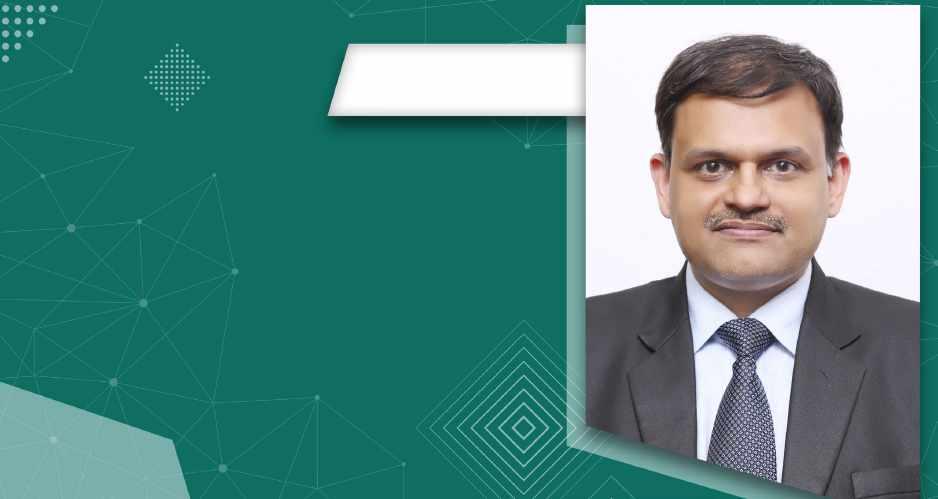
13 minute read
Sambit Sengupta
OCTOBER 2020
Sambit Sengupta
Advertisement
Associate Director – Solution Sales, Avnet Asia
What is a Smart factory, what it takes to build a Smart Factory, Why Companies are opting for Smart Factories? Finding answers for the pondering answers, Niloy from BISinfotech gets alongside for an extensive exchange of words with
Sambit Sengupta, Associate Director – Solution Sales, Avnet
Asia. Sambit defines the potential of Smart Factories solutions in India as enormous. From security to Avnet’s expertise and leadership in this nascent yet bullish sector, Sambit underlines the de facto future of Smart Factories. Edited Nub Below.
According to you, what is a perfect Smart factory (implementation, technology barriers, and cost) and how promising is this sector?
A smart factory is all about increasing efficiency in different functional areas of the existing factory. In working closely with our customers, we understand from them that despite multiple technology advancements (both in-house or collaborative work with vendors), some pain points remain at the execution level. They include: 1. Legacy industrial control systems that are prone to cyber threats when connected online 2. Lack of real-time data and disparate data systems 3. Keeping abreast of regulations and managing compliance 4. Balancing maintenance with throughput
Smart factory implementation requires on-the-go access with cloud-based system, optimized efficiency and most importantly a seamless integration of different sensors and edge devices, on-premises data gateways and finally, data ingestion into the cloud. After which, there is a huge amount of analytics required. This is a promising sector with growth prospects as there is ongoing innovation. Furthermore, with the advancement in smart sensors and cloud technologies, companies will enjoy more intelligent services at a cheaper cost. But often, the analytics portion is done as an afterthought and becomes a major technology barrier. The cost escalates if stakeholders do not see a return on investment (ROI), and ROI is determined not just by costs. Many times, we think that smart factory implementation will reduce cost, which is the eventual outcome, but if the business outcome is tallied with increased efficiency or throughput, then ROI becomes visible. All factories need to be smarter and the digital innovators of each company are working on KPIs which needs very agile implementation. A typical smart factory implementation does not concentrate on cost saving by eliminating jobs but eliminates the pain points, improves efficiency exponentially and that makes the business profitable.
Impact of Industrial Robotics is assessing, executing operations while providing productivity and efficiency?
Industrial robotics has been around since the early 1960s and has been used in welding, machine tending, and assembling in factories. The automation needs of smart factories have accelerated the growth of industrial robots from being standalone robots to collaborative and mobile robots. More integration of AI has made these industrial robots as efficient value adder in factory lines. Mobile robots or AGVs (Automatic guided vehicles) are becoming commonplace in modern factories in handling intralogistics.
Avnet for long has been a partner-first company. Given this sector, where solution providers to play the lead role, how Avnet can help to make Smart Factory a reality for customers?
Avnet works with system integrators as an end to end consulting partner to implement smart factory solutions. It is always a ‘partner first’ play for us. Partners appreciate the fact that Avnet has nearly 100 years of supply chain management expertise and in-house capability to integrate various devices to the cloud (device-to-cloud or D2C), as well as provide sophisticated and customized dashboards (cloud-to-device or C2D). We can also help our partners implement various AI and machine learning models which we have developed in
the past few years. A robust IIoT platform which is open to be white-labelled helps partners scale up a working proof of concept (POC) and deploy it in weeks. Our deep relationships with various sensor manufacturers assure customers of quality devices. We offer many options for our partners to choose from during the POC stage. Partners can onboard their sensor devices in our Partner Program’s DevLab as test-drive and can be risk-free during actual deployment. Furthermore, customers can tap into the Avnet ecosystem — from design to production to supply chain and logistics — throughout the lifecycle of their solution.
Omnipresence of IIOT underlines new challenges putting Cyber-security into the hot-spot. Critical security challenges faced by customers, common pitfalls in IIoT security implementation and any best practices?
Through strategic collaborations with partners, Avnet provides an end to end security implementation. The main challenge in implementing security in a connected world is to think ahead and remain one step ahead than attackers. We like to take a security approach from a “device up” manner. IIoT devices are often the sitting duck for side-channel and malware attacks. At every step of the implementation, we have to check where the probable attacks can happen- at the device level, at gateways, at data ingestion to cloud level. Avnet can deliver a complete hardware, software, cloud and middleware solution that delivers insights tailored to the customer’s specific needs.
How do you witness the holistic growth of Smart Factories in India comparing to other APAC countries?
The potential for smart factory solutions being deployed in India is enormous. Some of the APAC countries such as China, Singapore, Malaysia, and Thailand have invested in smart factory solutions much earlier than Indian factories. Many core industries in India have already deployed smart factory solutions. Sectors like pharma, large manufacturing in India have different digital initiatives going on and we have a large number of talented engineers who can speed up the deployments. These deployments are world-class and have a great scope of scaling up further.
The IIOT paradigm has certainly made the market competitive, where ensuring efficient supply chain, integration and improving transparency, safety and reliability are critically important. What challenges, scopes wherein keeping the point of relevance do it incur for semiconductor players?
All semiconductor players have varying degrees of efficient supply chains and highest standards of safety and reliability. The main challenges for end customers (OEMs making endpoint devices) of semiconductors are time to market, making them dependent on the reference designs and off-the-shelf solutions including software support. Semiconductor players need to be mindful about OEM’s varied use cases, especially in new product management and efficiency improvement. Based on various market research - 32-bit MCU, a discrete application processor, image sensors, IIoT sensors, wireless connectivity and MPUs with inbuilt security is going to be the highest revenue earners. The OEMs will look up to semiconductor players to provide them not only with reliable semiconductors/ chips but also the software support. These software has to be specific for the targeted IIoT applications. Avnet plays a major role in providing these SaaS solutions where our customers or partners can simply customise them for their specific needs.
The impact of COVID-19 pandemic on Smart factories segment, as it already enabling more contactless and biometric solutions, preventive and remote maintenance vital?
Factories globally were not fully prepared for the sudden pandemic. However, solutions were quickly deployed for contactless applications, such as face recognition and temperature sensor-based solutions. Many smart factories already have preventive and remote maintenance solutions. As we speak, we see a spurt of real-time location systems (RTLS) and remote-predictive maintenance solutions.
How has been Avnet helping, assisting and strategizing to tap this nascent market?
Avnet has developed a strong ecosystem to support our partners. We are focusing on business outcomes that matter to our partners and their end customers. Through our suite of advisory services and innovative workshops, we help the factory management identify the important KPIs that need to be tackled. In most cases, the defined improvement areas are in machine downtime, predictive analysis, machine idle time, the turnaround time of machines and equipment, overall equipment effectiveness (OEE) and backend integration with an internal ticketing system to monitor actual performance. Avnet’s Smart Factory solution helps companies keep track of production on the factory floor and measure production performance. By leveraging our IoTConnect platform, we generate insights for customers by collating and analyzing data across machines to make processes faster and more efficient.
What trends will foster Smart Factories and pivotal future of semiconductors designing and supply chain?
Semiconductor designing and supply chain have to recognize the software requirements of various use cases. Every use case needs to be customized. The future of supply chain will be in securing the supply chain and providing a lot of programming services.
Lastly, Avnet’s solutions and portfolio complementing this sector?
Avnet believes that each smart factory solution has to be bespoke. We have deployment experience in a wide variety of use cases. For example, Avnet supported a large pharmaceutical company to set up smart factory automation by leveraging our ecosystem and IoTConnect platform. We deployed centralized remote management, predictive analytics, real-time telemetry and analytics along with performance anomaly diagnosis for the customer’s production lines that span across many countries. We tailor the solutions along with our partners with a goal of making our partners and factories profitable.
The easier way of making your IoT data more effective
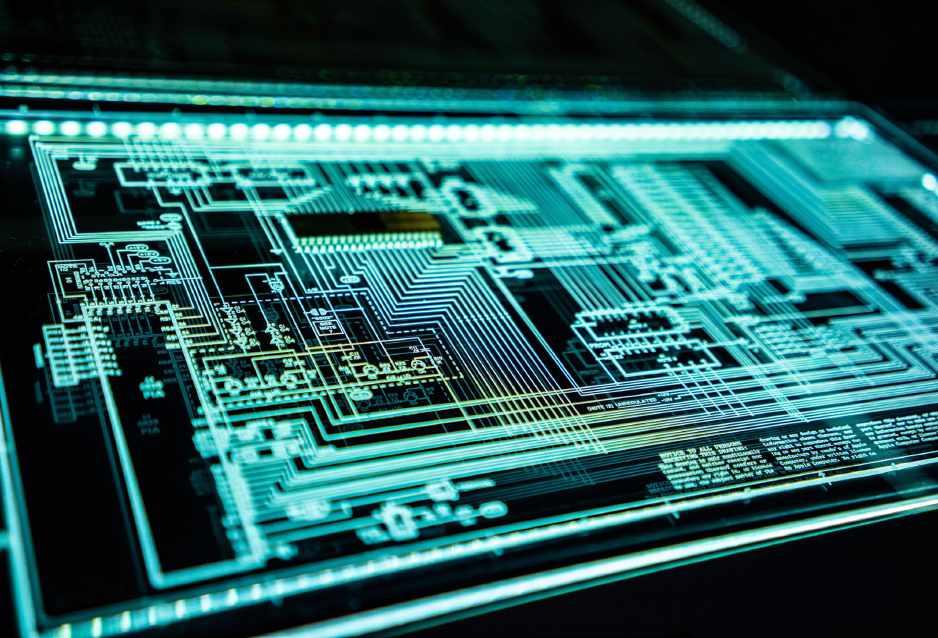
Mark Patrick Mouser Electronics
With the ongoing and massive deployment of smart sensors, the IoT is hitting its stride. Data on this scale is enabling entirely new business insights, that can translate to increased profit margins or new business opportunities for manufacturers and suppliers. Those manufacturers already using sensor data in new ways will appreciate the value of capturing information about their business. As an example, industrial plants can now use sensor data to support predictive maintenance models that lower their downtime and increase their productivity. This succinctly describes the attraction of the Industrial IoT (IIoT). In simple terms, predictive maintenance uses data captured by sensors to infer what may happen in the future, thereby equipping operators with the information they need to execute any steps appropriate to avoid the worst. In adopting this approach, maintenance can be planned, and take place at an earlier stage, avoiding unnecessary downtime caused by complete failure. When we repair, rather than replace, the cost of maintenance also comes down, so it's not surprising that manufacturers who are now embracing the IoT want to adopt preventative maintenance to achieve the best possible return on their investment, in terms of reduced downtime and
lower maintenance bills. This is just one example of how operational data can improve efficiency. It can also be applied to the supply chain, for example, in a manufacturing facility that has multiple complex stages pipelined together. Even the cost of a marginal delay between processes can threaten profitability; this is another example of how actionable data can deliver higher profitability.
The data needed to support these initiatives can come from anywhere and almost any process. The key here is linking all that data together in a way that makes sense and becomes actionable. For example, by monitoring the output of a chemical processing plant it might become clear that the presence of a certain chemical, gas, or other by-product has risen unexpectedly. This could indicate many things, including
the introduction of a contaminant at an earlier stage, reduced efficiency in a later stage, or an imminent failure somewhere else in the process.
Making sense of all the data now available is becoming something of a hurdle; one that manufacturers won't have encountered before the IIoT. If the number of sensors is small, then managing the data is relatively simple. A single sensor on a piece of machinery monitoring something like the amount of power being used isn't going to require too much management. However, if more sensors are added to monitor other aspects, such as vibration, temperature, or humidity, the data soon increases.
As the number of sensors increases and the relationships between them becomes more complex, analysing the relevance of the data they produce also becomes more complex. This is typically where cloud-based analysis steps in, where high-performance processing resources and access to data analysis algorithms can quickly relieve that burden and deliver valuable insights responsively.
Before the current resurgence in artificial intelligence, its fundamental building blocks were already being used, often in the form of Decision Engines. Many of those engines are based on an algorithm that dates back to the 70s and was developed by Dr. Charles Forgy; the Rete Algorithm. Its success is probably most notable in business systems, where data has always been plentiful, but now it is being applied to the IoT.
With its feet firmly in the camp of inference engines, the algorithm infers, rather reiterates, conditions based on the data presented. Without delving too deeply into the mechanics, it uses a rules-based approach to decision making, which makes it fast and extremely scalable. When applied to data coming from the IIoT, this approach would allow many tens of thousands of data points to be evaluated in real-time and, effectively, concurrently, without consuming huge amounts of processing resources.
This is the approach taken by UrsaLeo, a cloud platform provider offering analysis of IIoT data. Its solution is based on technology developed using the principles of the Rete algorithm. Through a user-friendly online interface, the rules used by the algorithm can be created by the user, based on their application. This can include alerts, sent when something unexpected happens, or, conversely, when an expected event doesn't happen. Alerts can also be scheduled, sending data regularly at a frequency appropriate to the user's needs. Assets being monitored do not need to be static; moving assets can also be monitored and, in this case, geofencing can be applied. This would send an alert when an asset moves inside or outside a defined geographical space; such as a forklift truck being used in an area where it isn't supposed to be. Sensor status can also be tracked, meaning that if a sensor continuously sends the same data, which may indicate a fault, an engineer can be informed and deployed to address the issue. With the data analysis in place, attention now turns to the data acquisition. Here, UrsaLeo has chosen to work closely with Silicon Labs and leverage the power of the Raspberry Pi ecosystem. It does this by combining a Raspberry Pi 3B+ running the Yocto distribution of Linux (which comes ready loaded with this version of the Pi board) with the Thunderboard Sense 2 evaluation and development module from Silicon Labs. This tiny board features seven individual sensors, some of which perform multiple functions, including a 6-axis motion sensor, air, and pressure sensors, temperature, and humidity sensing, and proximity-based on the Hall effect. The Thunderboard Sense 2 is based on a wireless Gecko microcontroller, which provides wireless connectivity to the Raspberry Pi providing the role of an internet gateway, to send all of the sensor data to the cloud-based service providing the analysis.
The analysis provided by the Rete algorithm approach means it isn't limited by the number of sensors used in a system. This scalability allows it to easily handle as much as 500,000 messages per second on the default service level, with the option to reserve more server resources if greater capacity is needed. The key to this performance is the tree-like structure of the algorithm, which uses pattern matching to deliver results. The decisions generated are not subject to the latency inherent with an 'If… Then…' approach to parsing conditions. It this innovative approach that gives the algorithm its performance.
Figure 1: UrsaLeo IoT hardware - comprising a Thunderboard 2 module and Raspberry Pi 3B+.
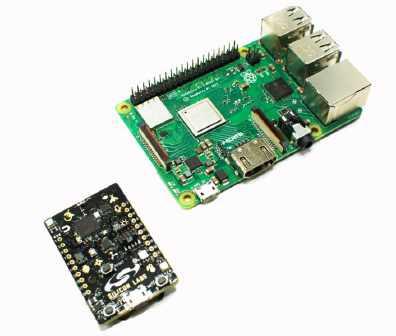
Bringing cloud services and optimized hardware platforms together makes it easier for manufacturers to start accessing the full power of the IoT. With highly capable boards like the Thunderboard Sensor 2, along with the power of the Raspberry Pi, it becomes much simpler to put technology to work for you. Every vertical sector could benefit from these innovative solutions, with barely any upfront cost. Generating actionable data has never been more accessible.