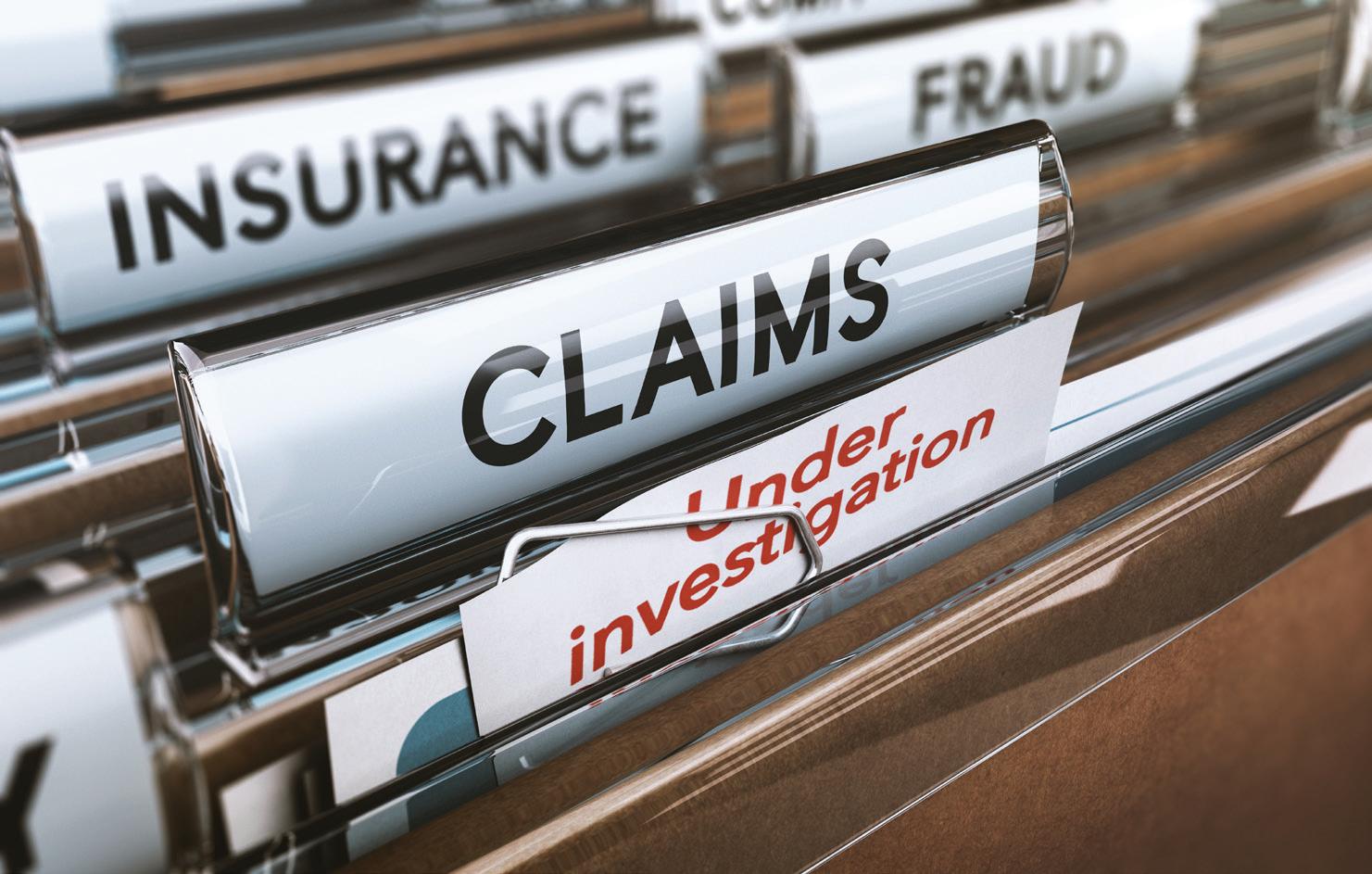
7 minute read
Expert view: AI and the detection of insurance claim fraud
NZSM sits down with Cara Carpenter, National Case Manager at ISACORP Corporate & Insurance Risk Solutions, to gain insights into the double-edged implications of emerging technologies for insurance fraud.
NZSM: Insurance companies are moving full steam towards automated customer experience, this means moving from paper to digitised processes, real-time claims processing and generally greater use of technology. What are the risks in terms of claim fraud?
CC: It is already noted in the USA that there has been an increase in insurance fraud since 2014, which coincides with the surge in insurance tech popularity. With automated processing, claim fraud can begin at the inception of the policy, as the information given is driven by the customer. Risk comes into it when this is automated and contained within standard parameters but without the ability to question or determine other relevant factors for risk and premium profiles.
An example is vehicle purchasing from a car yard. Registration, ownership, financial and insurance needs are automated and initially determined by ‘honest Joe’ the car salesman.
Either no or bogus information and (intentional or not) lax fact checking, followed by three months of payments for legitimacy, and then a claim for a stolen vehicle in South Auckland. The fraudster is laughing all the way to his bank app.
Money laundered or drug debt paid by vehicle or goods are services offered by local narcotics providers. The insurer may have little avenue to explore or recoup losses when the legitimacy of the client is not initially screened by insurance professionals.
Automated claim processes look for flags inside or outside set parameters or business rules. However, with the advent of image and document manipulation technologies and digital identity theft, fraudsters can circumvent these controls. Service providers to the Insurance industry, for example, have used the automated windscreen repair claim system to defraud insurers and ultimately consumers. Healthcare providers and organised criminal gangs worldwide have used insurance related automation for fraudulent gain.
Complex and involved frauds are difficult to identify and prosecute under manual fraud detection systems, especially when perpetrated by trusted professions and professionals. The automation of the claims process and the rules/parameters set can be used against insurers to commit on-going fraud.
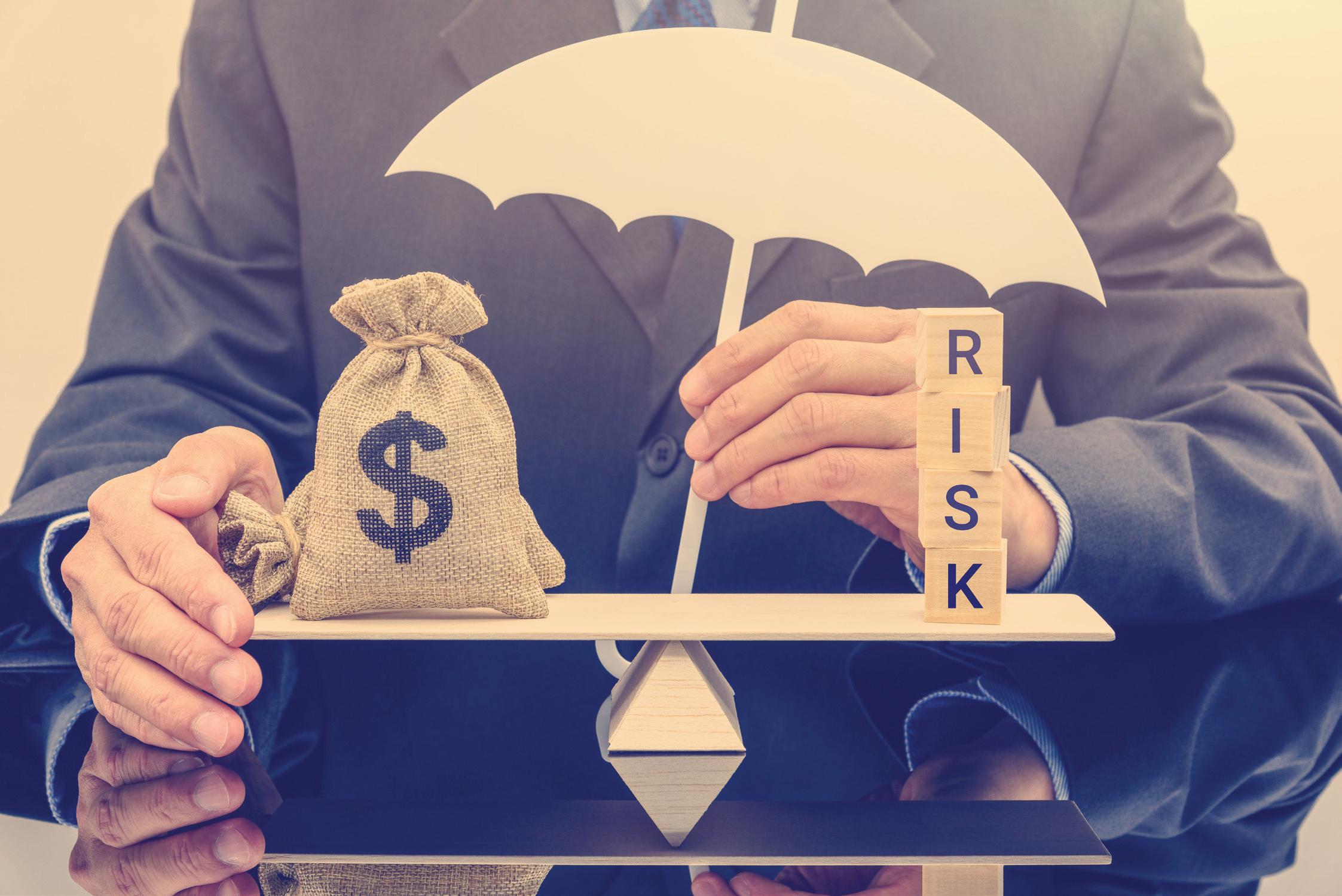
NZSM: In general, what do you see as the emerging trends in insurance fraud? Is the nature and/or incidence of insurance fraud changing?
CC: Fraud in this sector is both fluid and constant in nature. The builder will still lose his tools out of the back of his ute when tax time comes around, however tech advances now put the cost of replacement in the tens of thousands with added business interruption payouts.
With liability also in the mix in commercial insurance products, negotiated collusion between parties to obtain payment is emerging and, where possible, cultural barriers are exploited. New technologies can be exploited as new and emerging avenues for fraud.
Insurance products are becoming more tailored to the evolving personalised needs of co-sharing, ondemand and increasingly tech driven consumers’ lives. To keep up, fraud detection analysis needs to be dynamic and evolving in its capability.
Internationally, the rise of financial regulatory requirements against money laundering practices has seen emerging problems in large insurance fraud schemes. Additionally, shell insurance operations, senior care fraud, identity theft, cyber ransoming and catastrophic events are flagged as emerging problems.
The detection of fraud is generally reactive to known threats, however the evolving and fluid nature of insurance fraud schemes today requires prevention strategies and industry-wide cooperation for real gains.
NZSM: Automation will also see greater adoption of Artificial Intelligence technologies in claims processing. Can AI play a part in identifying possible fraud?
CC: Stephanie Taylor, Insurance AI Lead for Microsoft, explains that automation is allowing machines to perform repetitive monotonous tasks – e.g. a person doing exactly what they are told to do. AI, however, is mimicking the way persons think, say and do, but seeks patterns and insights from the data.
She states that AI is a superintelligent worker. Automation is the documents, scanning, form filling and chatbots, but AI is for intelligent tasks where the ability to learn and adapt is needed, such as policy decisions and fraud identification.
AI algorithms are currently being used to analyse large datasets to spot anomalies in claims and to extrapolate large scale fraud indicators. Visual analytics can be used to determine vehicle damage to repair cost accuracy. The use of cognitive and behavioural analysis of previous fraud schemes is used to detect deceptiveness in claims.
However, as with all things tech, the identification is only as good as the data input, the depth and breadth of the data set and the data analyst who reads it.
Internationally, AI has already demonstrated its usefulness as a tool for fraud detection using Insurtech partnerships. The AI Forum in New Zealand suggests this is a growth area in the sector, with 17 Insurtech companies established since 2017.
Additionally, traditional insurers are establishing AI strategies throughout their businesses. There are challenges and barriers to this for the New Zealand sector, with large costs, expected failures, lack of inter-agency pathways and cooperation, and the need to upskill employees.
Problems to be surmounted in this area are in the limited or internal datasets available, which can be skewed by systemic or unconscious bias and gender stereotyping. Additionally, the testing and learning stage required for this is a long process and can be fraught with false positives and, worse, failures leading to customer dissatisfaction.
NZSM: How will such technologies, such as automated fraud detection, work in practice?
CC: It uses rule-based learning to detect claims that indicate deceptiveness or the heightened risk of fraud. AI systems can learn rules that have been identified in previous insurance-related fraud schemes, and by using text and statistical analytics can examine assessor reports for anomalies.
Efficient cross-referencing of internal and external datasets with traditional and standardised fraud rules identifies the probability of fraudulent claims by detecting unusual patterns that fall outside or inside the parameters of these rules. Then this data is input to make algorithms smarter.
With the large amount of data we now produce, AI ‘supervised learning’ can analyse large data sets from banking data, etc. to provide more accurate personalised cover. This learning will also advance fraud detection.
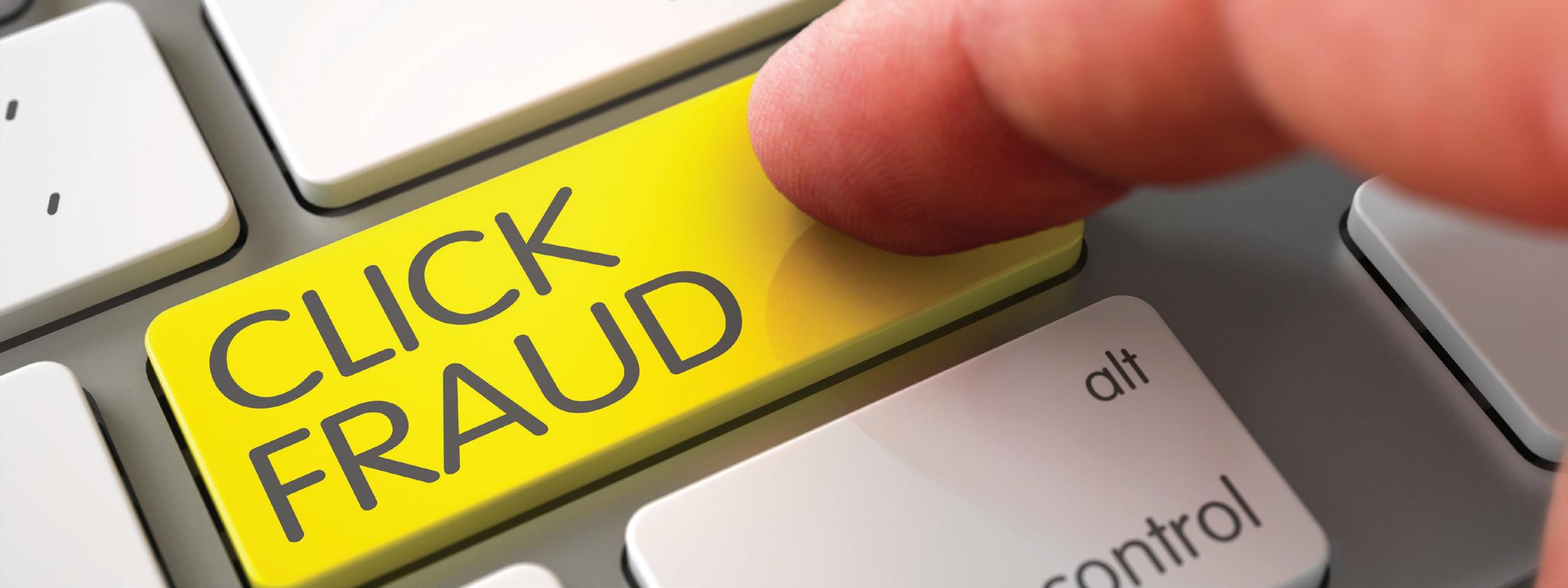
Ben Fletcher of the UK Insurance Fraud Bureau states that frauds have common traits, and these can be determined by data-sharing and analytics. “The move from reactively looking at data and intelligence at a practitioner level to using analytical tools to proactively look for trends and patterns at an industry level,” he says, “has been the single biggest step forward from the IFB’s point of view”.
NZSM: What anti-fraud technologies are emerging in the insurance sector?
CC: IDC Financial Insights states that insurance is becoming more personalised, predictive and real-time. Emerging technologies will be using deep learning techniques to assess and predict fraud patterns.
Natural language processing (NLP) uses phraseology and behaviour rules to detect the probability of fraud. In commercial and complex policies and claims, the use of NLP to harvest industry terms and meanings is emerging.
Computer vision, which is next level image recognition, can learn the difference between a concrete or wood post pixel. Understandably, this tool will be invaluable for MV accident claims when real time assistance and assessment can be just an image sent to an app and dealt with by a chatbot. Early identification of fraud is the key to success.
Emerging advances in facial recognition, fitness and health, geographic imaging and IoT data technology will all be used to detect fraud.
Stephanie Taylor of Microsoft , for example, shares a strategy she developed in the claim resolution area where her client had a 20 percent grey area on car claims where no one seemingly was at fault. By combining verbal explanations from customers and known data to a machine learning algorithm, they were more accurately able to determine the outcome of the grey area and reduced these claims to 3.9 percent.
James Breeze, Digital AI Lead at AXA XL, states that photoshopped images can now be automatically detected. When a claimant sends a photo of their car damage, the app quickly assesses the damage and highlights any similar images from stock photos on Google or elsewhere, thereby detecting potentially fraudulent activity. He emphasises that AI acts as a very necessary tool between the customer and automated and efficient app-based claim processes.
NZSM: Does fraud detection ultimately rely on the human touch?
CC: While AI can flag a claim, an analyst is required to consider whether a false positive has occurred, and an assessor and investigator is required to compile any evidence of the fraud. Additionally, when large, commercial or complex losses occur, the customer is more likely to want to pick up the phone and speak to someone.
New Zealand has a conservative insurance consumer base and customer trust in these new technologies will be a hurdle. Data protection and privacy concerns are strongly felt issues in New Zealand.
As an investigator in this space, I can see no replacement for some of the instincts applied by claims handlers on bare or seemingly benign information. While AI is the future, it remains to be seen if these instincts can be keenly mimicked.

Cara Carpenter is National Case Manager for ISACORP Limited. An intuitive professional with multisector experience in financial fraud, customs and coronial investigations, she holds a Bachelor of Criminology and Criminal Justice.
Image supplied.