Nicole Qian
Senior Thesis | 2024
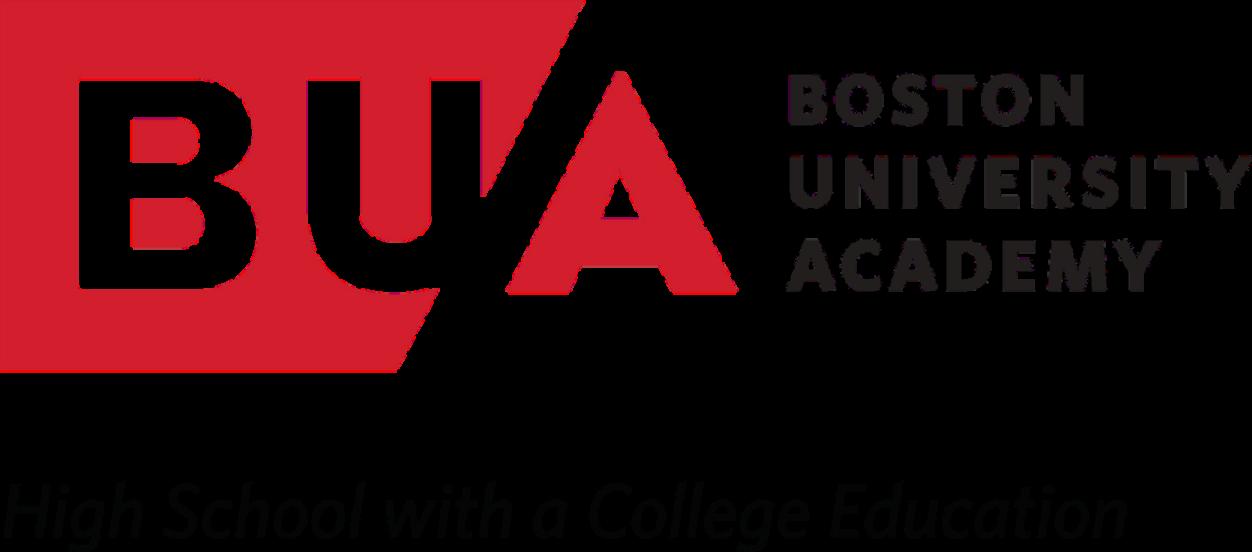
Visualizing Amyloid Fibrils: A Computational Chemistry Study
Nicole Qian, Straub Lab
Intro
Alzheimer’s disease is a neurodegenerative disease that affects millions of people in just the U.S. alone. It is the most common form of dementia, though its cause still needs more research to fully understand. Alzheimer’s symptoms typically start with memory loss, and the disease can eventually lead to further losses in thought processing. Alzheimer’s is expensive to treat and is in the top 10 leading causes of death in the United States.1 While age is currently the biggest risk factor that we are aware of, research is being done to better understand what causes the onset of Alzheimer’s. One theory that is being investigated is based around amyloid fibrils. These fibrils are also suspected to be related to other diseases like Parkinson’s disease and type 2 diabetes. In the U.S., Parkinson's affects hundreds of thousands of people,2 and almost 30 million people suffer from type 2 diabetes.3 Out of the different forms of diabetes, type 2 is by far the most common, affecting 90-95% of all people with diabetes. While Alzheimer’s and Parkinson’s seem to be strongly linked to people of older ages, type 2 diabetes is becoming increasingly common in younger adults and children.
1 (2020, October 26) What is Alzheimer’s disease? Centers for Disease Control and Prevention. Centers for Disease Control and Prevention.
2 (2023) Parkinson’s disease: Challenges, progress, and promise. National Institute of Neurological Disorders and Stroke. U.S. Department of Health and Human Services.
3 (2023, April 18) Type 2 diabetes. Centers for Disease Control and Prevention. Centers for Disease Control and Prevention.
Biological Background
There are three main branches of the amyloid family: pathological amyloids, artificial amyloids, and functional amyloids. Pathological amyloids were discovered first, and members of this branch are the fibrils that lead to amyloid diseases such as Alzheimer’s, Parkinson’s, and type 2 diabetes. Artificial amyloids were discovered second, after scientists discovered that denaturing proteins of certain structures can cause them to form amyloid-like fibrils. Functional amyloids were discovered last and have evolved from nature to serve biological roles in all kinds of organisms.4
Amyloid fibrils are made up of stacked layers of proteins. Proteins are chains of amino acids that can attach to each other to form complex structures. Each layer of an amyloid fibril is the same, but the formed structure is different from fibril to fibril. That being said, fibrils do follow a general cross-β pattern. This pattern is categorized by β sheets connected to each other but going away from the fibril’s axis. β sheets are a common secondary structure of proteins that are formed when β strands connect to each other with hydrogen bonds to form a sheet.5 This “cross-β motif” is made stronger by the connections of the side chains from side-by-side β sheets.4 While all amyloid fibrils have this general structure, the organization of individual β sheets and β strands can still produce very different formations. Amyloid fibrils flatten, so they can “stack” with nearby chains of the same structure. Even when they are in a two-dimensional folded state, amyloid proteins vary
4 Sawaya, M. R., Hughes, M. P., Rodriguez, J. A., Riek, R., & Eisenberg, D. S. (2021). The expanding amyloid family: Structure, stability, function, and pathogenesis. Cell, 184(19), 4857–4873. https://doi.org/10.1016/j.cell.2021.08.013
5 Secondary structure (2˚) beta strands. Secondary Structure - Beta strands.
greatly. For example, J-shapes are characteristic of Pick’s disease, while Alzheimer’s is caused by C-shaped formations (Figure 1).
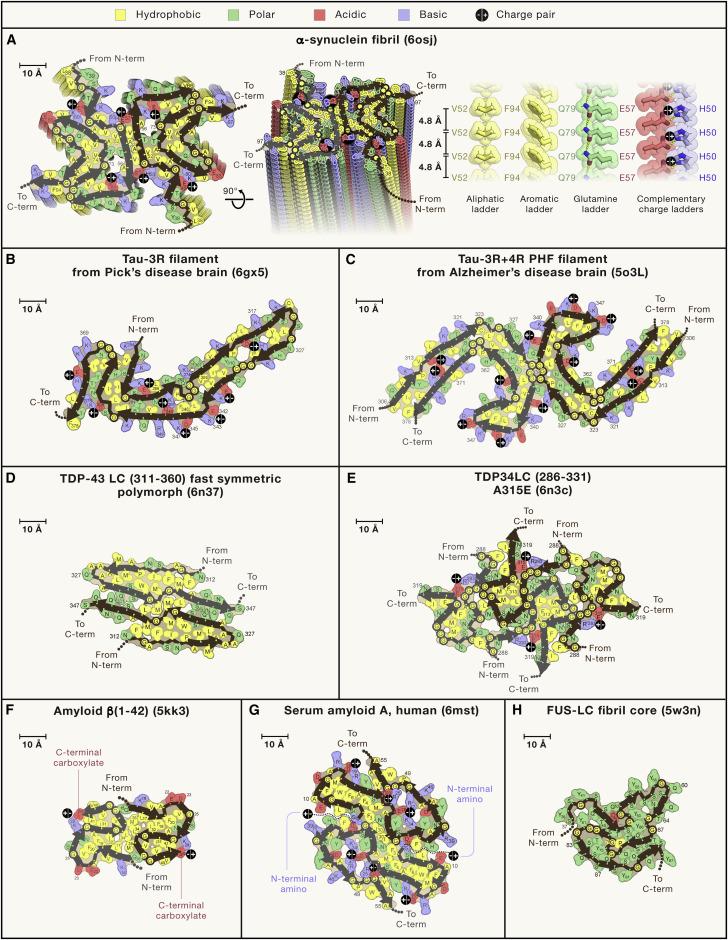
Figure 1: Shown on the left is a J-shaped tau filament; on the right are two C-shaped tau filaments.6
6 Sawaya, M. R., Hughes, M. P., Rodriguez, J. A., Riek, R., & Eisenberg, D. S. (2021). The expanding amyloid family: Structure, stability, function, and
Through all these variations, fibrils still share a “parallel” structure, meaning the N termini of protein chains align with each other, and the C termini of protein chains line are aligned as well. There are some exceptions to this parallel quality, but it is rare. Amyloid fibrils also differ in stability based on their specific structure. Pathological amyloids tend to be stable, while functional amyloids can have a wide range of stability.1
Pathological amyloids are particularly detrimental due to their potential for onset of neurodegenerative diseases. Aggregations of amyloid β and tau fibrils cause Alzheimer’s, while protein ⍺-synuclein leads to Parkinson’s.1 These two diseases alone affect tens of millions of people worldwide, but cures remain elusive without more research into their root causes. More research must be done on why pathogenic amyloids form the way they do in order to figure out how to prevent or treat these diseases. Gaining a fundamental understanding of the structure, behavior, and properties of these fibrils is crucial to making progress in this research.
Within the three main branches of amyloids, functional amyloids can be further categorized in five ways: structural amyloids, reservoir amyloids, information carrier amyloids, function-suppressing amyloids, and signaling amyloids. First, structural amyloids aid in maintaining certain shapes and providing support. This can range from keeping proteins aligned to supporting biofilms. Next, reservoir amyloids regulate protein activity when proteins “fibrilize” to serve various purposes. Information carrier amyloids serve almost an opposite purpose by maintaining a certain state and can also transmit information between cells. The fourth type of functional amyloids is functionsuppressing amyloids. These reduce the activity of the protein that
pathogenesis. Cell, 184(19), 4857–4873. https://doi.org/10.1016/j.cell.2021.08.013
forms the fibril. Lastly, signaling amyloids are formed when specific proteins fibrilize to signal for a process to begin. There are many amyloids that have multiple roles, so they may belong to several of these categorizations.
The amyloid hypothesis of Alzheimer’s Disease (AD) suggests that the formation of Aβ proteins into plaques causes the onset of AD. AD seems to develop as a result of a buildup of Aβ peptides around the hippocampus, neocortex, and the cerebrovasculature (CAA). Aβ peptides are formed from fragments of amyloid precursor protein (APP), which is a type 1 membrane glycoprotein that has many biological functions and are products of APP activity and processes. APP is made in the endoplasmic reticulum (ER), then is moved to the Golgi complex, and it eventually ends up in the plasma membrane. In humans, APP is processed with either an amyloidogenic or nonamyloidogenic pathway. Depending on the pathway, the APP is cleaved using αsecretase or β-secretase, respectively. A “cross β” structure, characteristic of amyloid fibrils that have been associated with AD, is formed from peptide chains of β-strand segments that are perpendicular to the long fibril and certain β-strand hydrogen bonds that are parallel to the axis. These cross β structures can be categorized as parallel or antiparallel. Studies on Aβ oligomers suggest that they have an antiparallel beta-turn-beta motif. The grouping of Aβ into long, uniform fibrils can indicate disease and trigger cell death and subsequently the loss of neurons. Amyloid fibrils are insoluble while Aβ oligomers are soluble and can spread throughout the brain, causing issues in memory and cognition. Research from the past few years suggests that oligomeric or prefibrillar species of the Aβ peptide cause the most damage to neurons.
In order to better understand the behaviors of potential causes of AD, such as amyloid fibrils, researchers can run simulations. There are many components to these simulations, one
especially important one being molecular dynamics. Molecular dynamics (MD) is used in many simulation software packages, including GROMACS and CHARMM, due to its potential for more extensive data on the interactions between observed molecules. The public and free availability of the GROMACS code allows for anyone to reuse or build upon its framework. The goal for GROMACS is to maximize its ability to capitalize on limited amounts of resources while still providing thorough data on the simulations it runs, such as the interactions that occur between lipids and proteins during a simulation. MD packages range in effectiveness for different sizes of molecules, as an increase in the number of atoms can lead to computational overload. Simulations can be performed with leapfrog Verlet algorithms, velocity Verlet algorithms, Brownian and stochastic dynamics, along with energy minimization, normal-mode analysis and simulated annealing calculations. GROMACS is compatible with most of the commonly used molecular dynamics force fields,7 which are AMBER (Assisted Model Building and Energy Refinement), CHARMM (Chemistry at Harvard Macromolecular Mechanics), GROMOS (GROningen MOlecular Simulation), and OPLS (Optimized Potentials for Liquid Simulations).8
Previous Computational Studies
Amyloid precursor protein (APP) has been studied through both experiment and computational work. It is an important factor to understanding Alzheimer’s, which the Straub group studies. Their most recent work studies lipid membrane biophysics through modeling lipid bilayers and theorizing about protein behavior.9
7 Force fields in gromacs#. Force fields in GROMACS - GROMACS 2024.1 documentation.
8 (2022, September 12) Force fields in molecular dynamics. CompChems. false.
9 Research focus " straub lab: Boston University. Straub Lab RSS.
APP is one of many components that may contribute to the onset of Alzheimer’s. β-secretase, also known as β-site amyloid pre-cursor protein cleaving enzyme (BACE1), the α-secretase–a disintegrin and metalloproteinase domain-containing protein 10 (ADAM10)– and APP are all key factors in researching Alzheimer’s disease (AD). One theory on the development of AD is the amyloid hypothesis of AD. This hypothesis theorizes that improper regulation of APP pathways, which are regulated by BACE1 and ADAM10, leads to more amyloidogenic βamyloid(Aβ) peptides. The greater the number of these peptides, the greater the chances of amyloid fibrils forming. Fibrils of amyloidogenic Aβ have been associated with the development of AD. In order to understand the regulation of APP pathways, it is crucial to understand what APP pathways actually do. First, APP is cleaved into a fragment which results in either APP-C99 or a Cterminal fragment, depending on which site the APP is cleaved at. The APP-C99 or C-terminal fragment is then also cleaved, with APP-C99 producing amyloidogenic Aβ and the C-terminal fragment turning into nonamyloidogenic peptides. The key question in this field of AD research is what causes the amyloidogenic Aβ to be upregulated. One proposed reason for this is high cholesterol. Some studies have found that significantly lowering cholesterol levels decreases Aβ production, which would mean a theoretically lower chance in developing AD. Results from other studies also indicate that membrane domains are a key factor in the regulation of APP processing. Simulations were performed with specific peptides with temperature replica exchange molecular dynamics (REMD) once a satisfactory state was reached. Membrane environments were then simulated with allatom (CHARMM36) and coarse-grained (Martini2.2) force fields.
Materials and Methods:
Simulation analysis was done with Python3 with Numpy, Scipy, Scikit-Learn or MDAnalysis packages. Molecular visualizations were done with Visual Molecular Dynamics (VMD), Stride, and POV-Ray, and MatPlotLib was used for generating figures.
All-Atom Proteins in Implicit Membranes:
REMD was used to simulate transmembrane congener models with juxta-membrane domains (JMDS) of different lengths for each protein in implicit membranes. Thicknesses of 30, 35, and 40 Å were used with GBSW in the CHARMM simulation program. Residues were arranged in an α-helical structure and centered in the GBSW membrane for each model.
Coarse-Grained Simulations:
Martini2.2 coarse-grained protein force fields were used for coarse-grained simulations. Each protein’s Martini2.2 congener model was first simulated in five different uniform lipid membranes: 100 mol% DPPC, 100 mol% DIPC, 100 mol% dilaurylphosphatidylcholine (DLPC), 100 mol% dioleoylphosphatidylcholine (DOPC), and 100 mol% 1-palmitoyl2-oleoyl phosphatidylcholine (POPC). They were also simulated in DIPC and DPPC membranes with various concentrations of CHOL: 10 mol% CHOL, 20 mol% CHOL, 30 mol% CHOL, and 40 mol% CHOL. Systems were made with the insane.py program.10 For each system, five replicates were simulated in GROMACS with the new-rf input parameters. Then, the steepest descent was minimized. Next, a velocity rescaling thermostat and a
10 Wassenaar, T. General purpose coarse-grained force field. insane.
semi-isotropic Berendsen barostat with a compressibility of 4.5 × 10-5 bar-1 and an integration timestep of 20 fs were used to equilibrate the systems in an isobaric-isothermal (NPT) ensemble. For production simulations, a semi-isotropic Parrinello-Rahman barostat with a compressibility of 3.0 ×10-4 bar-1 was used in the NPT ensemble for 5μs at 310K with a 0.15M ion concentration.
All-Atom Proteins in Explicit Membranes:
Proteins in 7:3 DPPC:CHOL and 9:1 DIPC:CHOL membranes from each replicate simulation were back mapped to the all-atom force field (CHARMM36) using the backwards.py and initram-v5.sh programs.11 DIPC and DPPC lipids were back mapped to di-C18:2 PC lipids and di-C16:0 lipids respectively, using Martini2.2’s one-to-four bead per atom representation. In order to use the initial ramdisk scheme with the specific version of GROMACS being used, the Verlet cutoff scheme for neighbor searching and soft-core potentials was used. In the 9:10 DIPC:CHOL system, 2nm of water was added perpendicular to the membrane of the ADAM10 in order to stop certain contact between JMDs. Systems were equilibrated in the NVT ensemble with the velocity rescaling thermostat and a 1-fs timestep for 5ns and in the NPT ensemble with a 1-fs timestep, velocity rescaling thermostat, and semi-isotropic Berendsen barostat with a compressibility of 4.5×10-5 bar-1 for 10 ns. The Nose-Hoover thermostat and semi-isotropic Parrinello-Rahmen barostat with a compressibility 4.5×10-5 bar-1 for 1μs were used for production simulations. To be able to analyze the simulations, coordinates were saved every 100 ps and also the last 900 ns of each.
11 Abraham, C. B., Xu, L., Pantelopulos, G. A., and Straub, J. E. (2023) Characterizing the transmembrane domains of ADAM10 and BACE1 and the impact of membrane composition. Biophysical Journal 122, 3999–4010.
GROMACS 2021.2 at 310K with an ion concentration of 0.15M was used for all simulations.
Results and Discussion:
REMD simulations were used to determine the minimal length congener sequences of ADAM10 and BACE1 in 30-, 35-, and 40-Å GBSW implicit membranes that were structurally similar to the membranes that would be used in other membrane simulations.
Characterization of the ADAM10 TMD and JMD:
Results from simulations show that the length of the ADAM10 TMD and TMD α-helix are not significantly influenced by membrane lipid and CHOL concentrations. The tilt angle between ADAM10’s α-helix and the membrane normal and residues’ mean oriental angles were calculated for coarse-grained DIPC: CHOL and DPPC:CHOL systems with varying concentrations of CHOL, and for the all-atom systems with 9:1 DIPC:CHOL and 7:3 DPPC:CHOL membranes. In DIPC membranes, it was found that the tilt angle of ADAM10 had an inverse relationship with membrane width.
Characterization of BACE1 TMD and JMD:
The BACE1 congener model was simulated under the same conditions as the ADAM10 simulations. For the all-atom simulations, numbers of hydrogen bonds were computed to determine the nature of interactions between the membrane and BACE’s TMD and JMDs. BACE1’s tilt angle has the same relationship with membrane width as ADAM10’s, but for both
DIPC AND DPPC membranes, rather than just for the DIPC membranes.
Conclusion:
Specific TMD and JMD residues are ideal for ADAM10 and BACE1’s transmembrane congener models. The ADAM10 congener model has protein-CHOL contacts with the membrane. The α-helix and orientation of the membrane of ADAM10’s TMD did not change in differing membranes. Liquid-disordered membrane types displayed an inversely proportional relationship between the tilt of the α-helix and the membrane width. Liquidordered membranes take a different status with a lower tilt angle due to a lower number of hydrogen bonds in various areas. There is a possibility that qualities of the liquid-ordered membranes explain the possible localization and enzymatic activity of ADAM10 in liquid-disordered domains. BACE1 is typically bound to the membrane on the C-terminal sides of the TMD and JMD, with a RCLRCLR motif. Like the ADAM10 congener model, the BACE1 congener model has protein-CHOL contacts that it uses to interact with the membrane. Changes in anchoring residues and rotations of the TMD α-helix around the membrane axis could influence BACE1’s partitioning, homodimerization, and enzymatic activity. ADAM10 and BACE1 both have arginine residues included in their TMD and JMD sequences, a fact that is particularly notable for BACE1’s RCLRCLR anchoring motif. The presence of arginine in ADAM10 and BACE1 near their TMDs may influence their membrane insertion and management. Bilayer thickness, membrane ordering, and certain interactions with CHOL all affect lipid composition on the structure and orientation of ADAM10 and BACE1’s TMDs. More specifically for BACE1, these factors affect the length of the TMD α-helix and the tilt and orientation of the helix in relation to the membrane. BACE1 is able to adapt better to wider liquid-ordered membranes than ADAM10
because of its RCLRCLR motif, whereas wider membranes in ADAM10 only affect tilt angle. Interactions between specific proteins and APP may be affected by different membrane compositions, with the membranes preventing or increasing the proteins’ APP-cleaving activity. AD research could be furthered by further research in liquid-ordered and liquid-disordered membrane domains and APP activity associated with the development of Aβ, particularly with the use of the developed TMD models and potential future models.
Alzheimer’s is the most common neurodegenerative disorder, and constitutes around 75% of cases of dementia (cite). BACE2, which is the homolog of BACE1, is a Θ-secretase and cleaves APP in the Aβ domain. Ɣ-secretase is a protein complex that cleaves APP after its previous cleaving processes. APP traffics through secretory, endo-lysosome and recycling pathways. APP trafficking is an important factor in APP processing because of APP’s relationship with certain secretases. Specific pathways and secretases can significantly influence APP processing and Aβ generation. Post-translational modifications of APP include Nglycosylation (N-Gly), O-glycosylation (O-Gly), phosphorylation, sulfation, palmitoylation, ubiquitination, and sumoylation. APP matures in the Golgi apparatus as a result of O-Gly. Once it has undergone the N-Gly and O-Gly processes, APP prefers to subsequently undergo phosphorylation. Palmitoylation is a type of protein modification that adds fatty acids to a cysteine residue, and the process is regulated by palmitoyl acyltransferases and acyl protein thioesterases. Protein palmitoylation is one of the ways in which protein trafficking is regulated. Ubiquitination is a protein modification process that attaches ubiquitin to lysine residues. This process is also included the process of protein trafficking. Protein sumoylation modifies through interactions of lysine residues on target proteins with ubiquitin-like modifiers. This modification
helps with the regulation of protein functions and is catalyzed by various enzymes. Tyrosine sulfation occurs in the late Golgi compartments and is implicated in both protein trafficking and the proteolysis process.
Computational Methods Used
GROMACS:
GROMACS is a tool to process files from the Protein Data Bank, or PDB files. PDB files are obtained first from the online RCSB database. Protein structures are modified by manually removing all the water molecules, marked as “HOH” residues, although removing water is not always necessary and depends on the molecule and circumstances. PDB files need to be inspected for entries labeled “MISSING”, which means an atom or residue is missing, as this could cause issues in future processing if the missing atom or residue is not added. PDB files are then converted to GROMACS format, which involves generating a topology file, a position restraint file, and a post-processed structure file. Force fields are then chosen with care; in this study we used the CHARMM36 forcefield. Topology files contain information about all atomic coordinates, bonds, bond angles, and dihedral angles. Upon confirming the structure of the protein in GROMACS, the system is solvated in water and ions are added to neutralize the system. Next, the solvents and ions must be equilibrated around the protein in two phases. The first phase is conducted with a constant number of particles, volume, and temperature (NVT), which allows one to stabilize the energy of the system in relation to temperature. Once the temperature has been stabilized, the pressure and density of the system must also be stabilized. This phase is conducted with a constant number of particles, pressure, and temperature (NPT) in a similar process as NVT equilibration.
Upon equilibration, molecular dynamics are run for a complete time interval of 1 ns using force fields.
CHARMM-GUI:
CHARMM-GUI is a tool that builds systems and prepares files that can be used with packages like GROMACS. CHARMMGUI is used to set the C-terminal to be neutral for all segments of the fibril. Next, the MDAnalysis12 package is used to modify the PDB file in order to extend the fibril to the desired number of layers. With the extended fibril file, the CHARMM-GUI solution builder is used to place the fibril in an octahedral water box type with an NaCl concentration of 0.15M. This solution neutralizes the charge of the fibril. The CHARMM36m13 forcefield is an all-atom protein force field that is accurate for intrinsically disordered peptides and proteins.
At this stage, the same steps for a GROMACS simulation can be taken, starting from energy minimization, as the steps before have already been covered with CHARMM-GUI.
FibMap:
The FibMap calculates hydrogen bonds using MDAnalysis’s Hydrogen Bond Analysis tool. Salt bridges are calculated by calculating the minimum distance between charged residues. Pi-stacking interactions are calculated with the methodology developed by Zhao et al.14 The positions of a central
12 (2024) MDAnalysis. Powered by MDAnalysis.
13 Home. CHARMM.
14 Zhao, Y., Li, J., Gu, H. et al. Conformational Preferences of π–π Stacking Between Ligand and Protein, Analysis Derived from Crystal Structure Data Geometric Preference of π–π Interaction. Interdiscip Sci Comput Life Sci 7, 211–220 (2015). https://doi.org/10.1007/s12539-015-0263-z
fibril layer are positioned in a way that the longest interatomic distance is aligned with the X-axis to determine the positions of the residues. The trajectory analysis is computed by the number of specific interactions per layer.
Results and Discussion
One tool that can be used to analyze trajectories from molecular dynamics simulations is MDAnalysis, which is a Python library that allows one to convert between PDB files and trajectories easily. This was a crucial step to the programming in this project. I developed a program that built on the previous computational work of Abraham et. al.,15 which processes PDB and trajectory files to visualize fibril structure. The previous program uses molecular geometries to calculate inter- and intramolecular forces and can display properties such as salt bridges as determined by the user. Given that the layers of an amyloid fibril are all identical, each fibril can be represented in a two dimensional, top-down view of one layer.
15 Abraham, C. B. (2023) Conor-B-Abraham/FibMap: Calculates and displays intermolecular forces within an amyloid fibril from a PDB or molecular dynamics trajectory. GitHub.
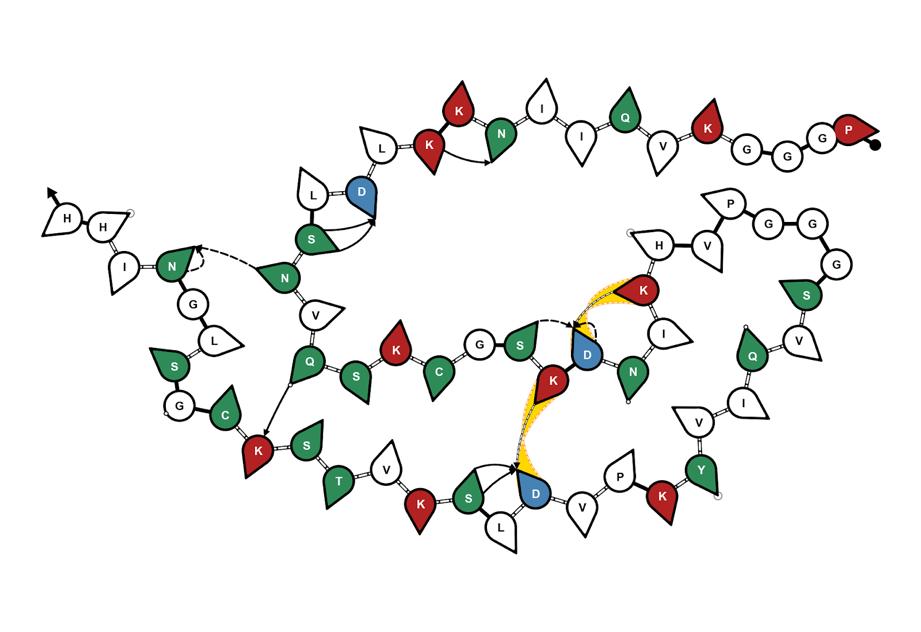
Figure 2: Fibril Map of a lysozyme.16
Using the existing computational framework for visualization of fibrils, I wrote my program to expand the visualization to include side views of interlayer hydrogen bonds. The previous work allows one to display hydrogen bonds between the molecules by processing information about donor and acceptor amino acid residues, and the probability of forming intra- and inter-layer hydrogen bonds. The input files had to be formatted in a unique way to allow for proper parsing. Upon proper formatting, the modified text files were processed to extract information about pairs of amino acid residues that have a probability of forming
16 Abraham, C. B. (2023) Conor-B-Abraham/FibMap: Calculates and displays intermolecular forces within an amyloid fibril from a PDB or molecular dynamics trajectory. GitHub.
interlayer hydrogen bonds greater than 90%. The pairs that satisfy this condition were transcribed into empty files.
Next, the program starts mapping the satisfactory bonds using the newly written files. The code parses through each pair of hydrogen-bonded residues and plots them on lines that represent the layers of the fibril. Since only interlayer hydrogen bonds were considered, lines connecting the appropriate residues are drawn to represent the hydrogen bonds that are most likely to occur. Each amino acid is also labeled by its single-letter abbreviation (with HSD being assigned ‘Z’, since it is not an amino acid and does not have a set single-letter abbreviation), and each amino acid pair is given its own color.
Donor, Acceptor
['1-ILE8-BB', '1-GLN7-BB']
['1-ILE9-BB', '1-ILE8-BB']
['1-LYS11-BB', '1-ASN10-BB']
['1-LEU13-BB', '1-LYS12-BB']
['1-VAL18-BB', '1-ASN17-BB']
['1-LYS25-BB', '1-SER24-BB']
['1-ASN27-BB', '1-ASP26-BB']
['1-GLN38-BB', '1-VAL37-BB']
['1-ILE39-BB', '1-GLN38-BB']
['1-VAL40-BB', '1-ILE39-BB']
['1-TYR41-BB', '1-VAL40-BB']
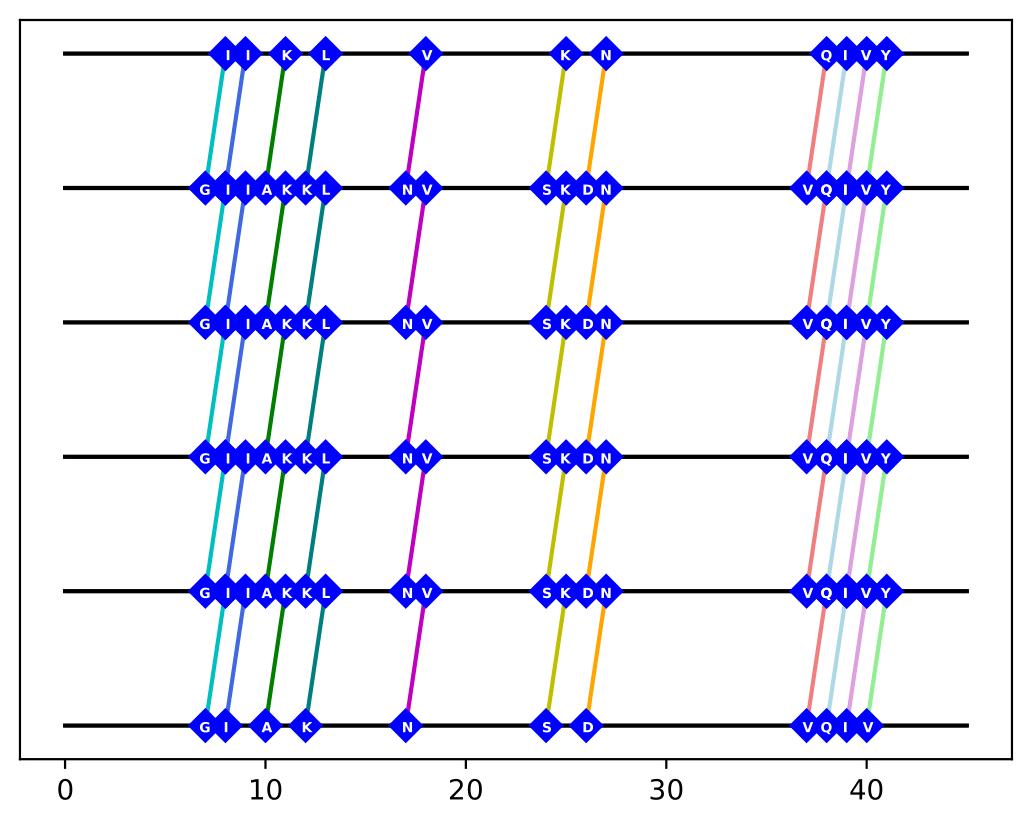
Figure 3: List of donor-acceptor interlayer hydrogen bond pairs, along with its visualized map.
This kind of visualization allows one to better understand fibril structure in more dimensions and unearth the complexity of interlayer hydrogen bonding, which is crucial to understand in determining the structure-function relationship of different amyloid fibrils. Given the amyloid hypothesis of AD, looking for possible trends in what causes the aggregation of amyloid fibrils can help us better understand AD.
Possible Future Direction of My Project:
To improve my program, there could be many more commands and functions added. While it currently only focuses on hydrogen bonds, I could add features that allow the program to also display other interlayer interactions. More generally, the program can be improved to be able to handle more data and to process it more efficiently. With increased knowledge in both Python and amyloid fibrils, I would hopefully be able to visualize helpful aspects of the fibrils with my program. These additional aspects would include an ability to more clearly display a detailed rendering of the protein structure, since structure is incredibly important to the formation of an amyloid fibril. My general hope for the program would be for it to reach a point at which it would be able to provide a different and progressive perspective on amyloid fibrils. Should my program ever reach this point, it may be able to aid in furthering research in the field of amyloid fibrils and the diseases they can lead to.
More generally, and on a larger scale, I think it would be cool to somehow incorporate more modern technology into the study of amyloid fibrils. For example, being able to interact with the kinds of models visualized in programs like VMD in a VR environment rather than simply on a screen. To go further, I think being able to somehow visualize simulations and experiments in a
VR setting could, at the very least, be interesting to experience and could even further AD research in a more applied situation. Throughout my internship, I had to learn the basics of various software packages like GROMACS and MDAnalysis and familiarize myself with the Protein Data Bank (PDB) site and VMD. Performing processes like creating trajectory and topology files and editing PDB files required following detailed tutorials and at least a general understanding of the context of these various files. Had I not had any prior coding experience, learning all these things would have been even more difficult, regardless of how much chemistry or biology knowledge I had. I think adapting these packages or creating different versions that can be more easily used by anyone that may not have any computer science background. Perhaps instead of using commands and flags in the terminal, there would be buttons or text input prompts.
Bibliography:
Abraham, C. B., Xu, L., Pantelopulos, G. A., and Straub, J. E. (2023) Characterizing the transmembrane domains of ADAM10 and BACE1 and the impact of membrane composition. Biophysical Journal 122, 3999–4010.
Sawaya, M. R., Hughes, M. P., Rodriguez, J. A., Riek, R., & Eisenberg, D. S. (2021). The expanding amyloid family: Structure, stability, function, and pathogenesis. Cell, 184(19), 4857–4873.
https://doi.org/10.1016/j.cell.2021.08.013
Chen, Gf., Xu, Th., Yan, Y. et al. Amyloid beta: structure, biology and structure-based therapeutic development. Acta Pharmacol Sin 38, 1205–1235 (2017).
https://doi.org/10.1038/aps.2017.28
Wang X, Zhou X, Li G, Zhang Y, Wu Y and Song W (2017)
Modifications and Trafficking of APP in the Pathogenesis
of Alzheimer’s Disease. Front. Mol. Neurosci. 10:294. doi: 10.3389/fnmol.2017.00294
Mark James Abraham, Teemu Murtola, Roland Schulz, Szilárd
Páll, Jeremy C. Smith, Berk Hess, Erik Lindahl, GROMACS: High performance molecular simulations through multi-level parallelism from laptops to supercomputers, SoftwareX, Volumes 1–2, 2015, Pages 1925, ISSN 2352-7110, https://doi.org/10.1016/j.softx.2015.06.001.
Straub, J. E., & Thirumalai, D. (2011). Toward a molecular theory of early and late events in monomer to amyloid fibril formation. Annual Review of Physical Chemistry, 62(1), 437–463.
https://doi.org/10.1146/annurev-physchem-032210-103526
Berndt, K. D. (1996, May 31) 3.0 Types of Secondary Structure. 3.2 sheets.
Secondary Structure - Beta strands. (2014) Secondary structure (2˚) beta strands.
Centers for Disease Control and Prevention. (2020, October 26)
What is alzheimer’s disease? Centers for Disease Control and Prevention.
Centers for Disease Control and Prevention. (2023, April 18) Type 2 diabetes. Centers for Disease Control and Prevention. (2023) Parkinson’s disease: Challenges, progress, and promise.
National Institute of Neurological Disorders and Stroke. U.S. Department of Health and Human Services. (2022, September 12) Force fields in molecular dynamics. CompChems. false.
Force fields in gromacs#. Force fields in GROMACSGROMACS 2024.1 documentation.
Secondary structure (2˚) -- beta strands. Secondary
Structure - Beta strands. Wassenaar, T. General purpose coarse-grained force field. insane. (2024) MDAnalysis. Powered by MDAnalysis.
Home. CHARMM.
Zhao, Y., Li, J., Gu, H. et al. Conformational Preferences of π–π
Stacking Between Ligand and Protein, Analysis Derived from Crystal Structure Data Geometric Preference of π–π
Interaction. Interdiscip Sci Comput Life Sci 7, 211–220 (2015). https://doi.org/10.1007/s12539-015-0263-z
Research focus " straub lab: Boston University. Straub Lab RSS.
Abraham, C. B. (2023) Conor-B-Abraham/FibMap: Calculates and displays intermolecular forces within an amyloid fibril from a PDB or molecular dynamics trajectory. GitHub.
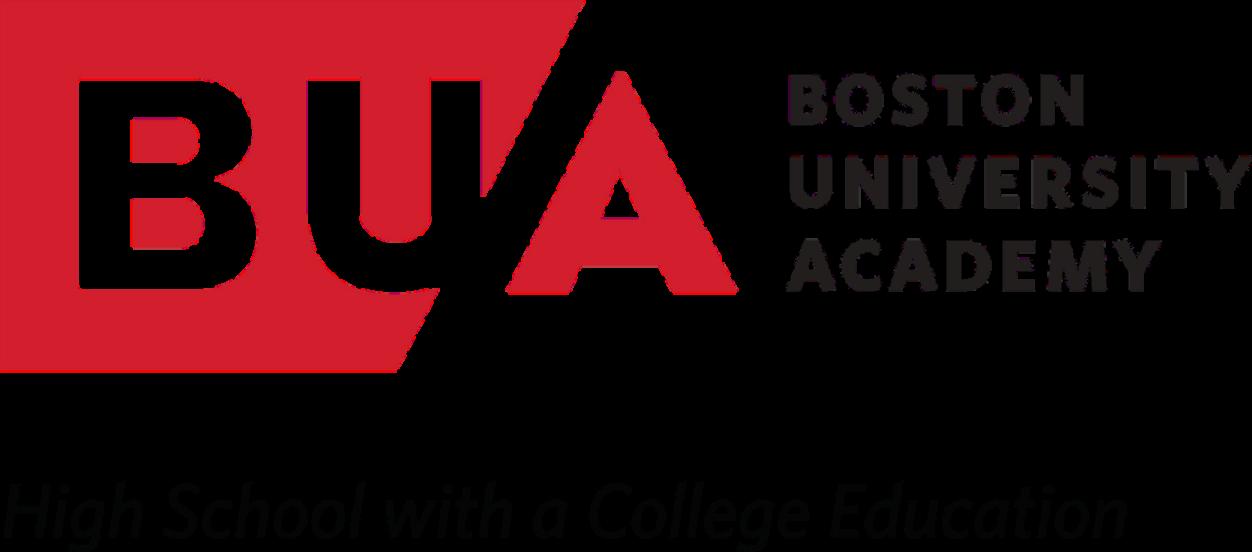