Machine learning helps detect lymphedema among breast cancer survivors Using algorithms in a clinical setting A study for advancing technology in diagnosis
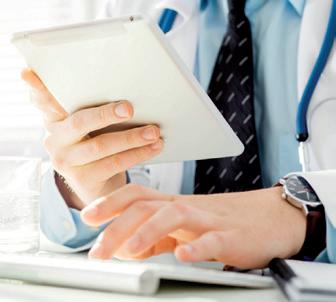
By Jeanna Qiu and Deborah Axelrod
This article is a synopsis of an innovative study led by our research team at New York University and published in the journal mHealth, titled “Machine learning for detection of lymphedema among breast cancer survivors.”
Introduction
Symptoms are subjectively perceived indicators for abnormal changes in body functioning due to illness or side effects from medical treatment. Lymphedema is associated with more than 20 distressing symptoms and is one of the most distressing and dreaded late adverse effects from breast cancer treatment. Currently there is no cure for lymphedema, but early detection can help patients receive timely intervention to effectively manage lymphedema. In our digital era, integrating technology into health care has led to advances in detecting and predicting various medical conditions. Machine learning is of interest to researchers due to its ability to construct algorithms that continually improve predictions and generate automated knowledge through data-driven predictions or decisions with incoming data—in this case, lymphedema symptom reports.
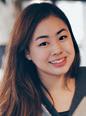
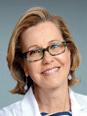
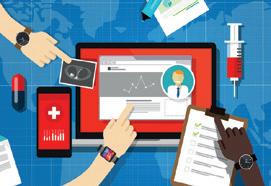
Machine learning is particularly beneficial when there are many relevant factors that are not independent, which is true for lymphedema symptoms. Real-time symptom report refers to the report of symptoms that patients are experiencing at the time of reporting. The use of machine learning integrating real-time patientcentered symptom report and real-time clinical analytics to develop real-time precision prediction may improve early detection of lymphedema and long term clinical support for breast cancer survivors who face lifelong risk of lymphedema. Our study aimed to appraise the accuracy, sensitivity, and specificity to detect lymphedema status using machine learning algorithms based on real-time symptom report.
Methods
In this study, we used a web-based tool to collect information from 355 women who had undergone treatment for breast cancer,
Jeanna M. Qiu is a junior at Harvard College studying Chemical and Physical Biology, with a Sociology secondary. Since high school, she has been a research intern for the lymphedema research team.
Dr. Deborah Axelrod is a board-certified surgeon specializing in breast diseases and breast cancer and Professor of Surgery at New York University School of Medicine. Dr. Axelrod has published over 80 articles in peer-reviewed journals on breast cancer and lymphedema.
including surgery. In addition to sharing demographic and clinical information, including whether they had been diagnosed with lymphedema, participants were asked whether they were currently experiencing 26 different lymphedema symptoms. Statistical and machine learning procedures were performed for data analysis. Five different classification algorithms of machine learning were compared: Decision Tree of C4.5, Decision Tree of C5.0, gradient boosting model, artificial neural network, and support vector machine. We also compared the algorithms with a conventional statistical approach, Youden’s method, which determines the optimal threshold for the symptom count based on the receiver operating curve.
Results
We found that all five machine-learning approaches outperformed the standard statistical approach, and the artificial neural network achieved the best performance for detecting lymphedema. The artificial neural network was 93.75 percent accurate, correctly classifying patients to have true lymphedema cases or non-lymphedema cases based on the symptoms reported. Thus, a well-trained artificial neural network classifier using real-time symptom reports can provide highly accurate detection of lymphedema. Such detection accuracy is significantly higher than that achievable by current and often used clinical methods, such as healthcare providers’ observation of swelling.
Clinical Implications
Findings of our study demonstrate that an mHealth system designed for real-time lymphedema risk detection is feasible, reliable, and valid. A well-trained Artificial Neural Network classifier using real-time symptom report provided highly accurate detection of lymphedema. Our study provides a novel, pragmatic and cost-effective realtime lymphedema risk detection employing a machine learning algorithm that could be used by patients or clinicians anywhere and anytime. Conducting such real-time
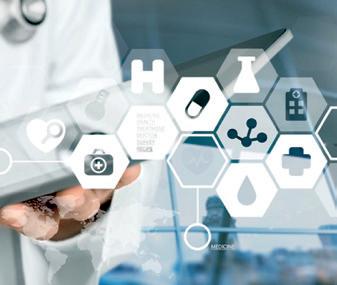
lymphedema assessment encourages patients to monitor their lymphedema status without having to visit a healthcare professional, but based on patients’ symptoms and resulting risk for lymphedema, could alert those at risk to schedule formal clinical assessment. Such an approach has the potential to reduce healthcare costs and increase the likelihood of early detection and intervention, which could reduce the risk of lymphedema progressing to more severe stages. LP
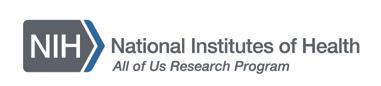
This study was supported by National Institutes of Health (NIMHD P60 MD00053803 and NCI1R01CA214085-01), Judges and Lawyers for Breast Cancer Alert, Pfizer Independent Grants for Learning & Change (13371953 The-Optimal Lymph-Flow™) and the Pless Center for Nursing Research of NYU Rory Meyers College of Nursing.
Our study provides a novel, pragmatic and cost-effective real-time lymphedema risk detection employing a machine learning algorithm that could be used by patients or clinicians anywhere and anytime.
To view the full article: Fu, M.R., Wang, Y., Li, C., Qiu, Z., Axelrod, D., Guth, A.A., Scagliola, J., Conley, Y., Aouizerat, B.E., Qiu, J.M., Van Cleave, J.H., Yu, G., Haber, J. (2018). Machine learning for detection of lymphedema among breast cancer survivors. mHealth, 4:17, 1-11. doi: 10.21037/mhealth.2018.04.02 View this article at: http://dx.doi.org/10.21037/ mhealth.2018.04.0 PubMed PMID: 29963562; PubMed Central PMCID: PMC5994440.