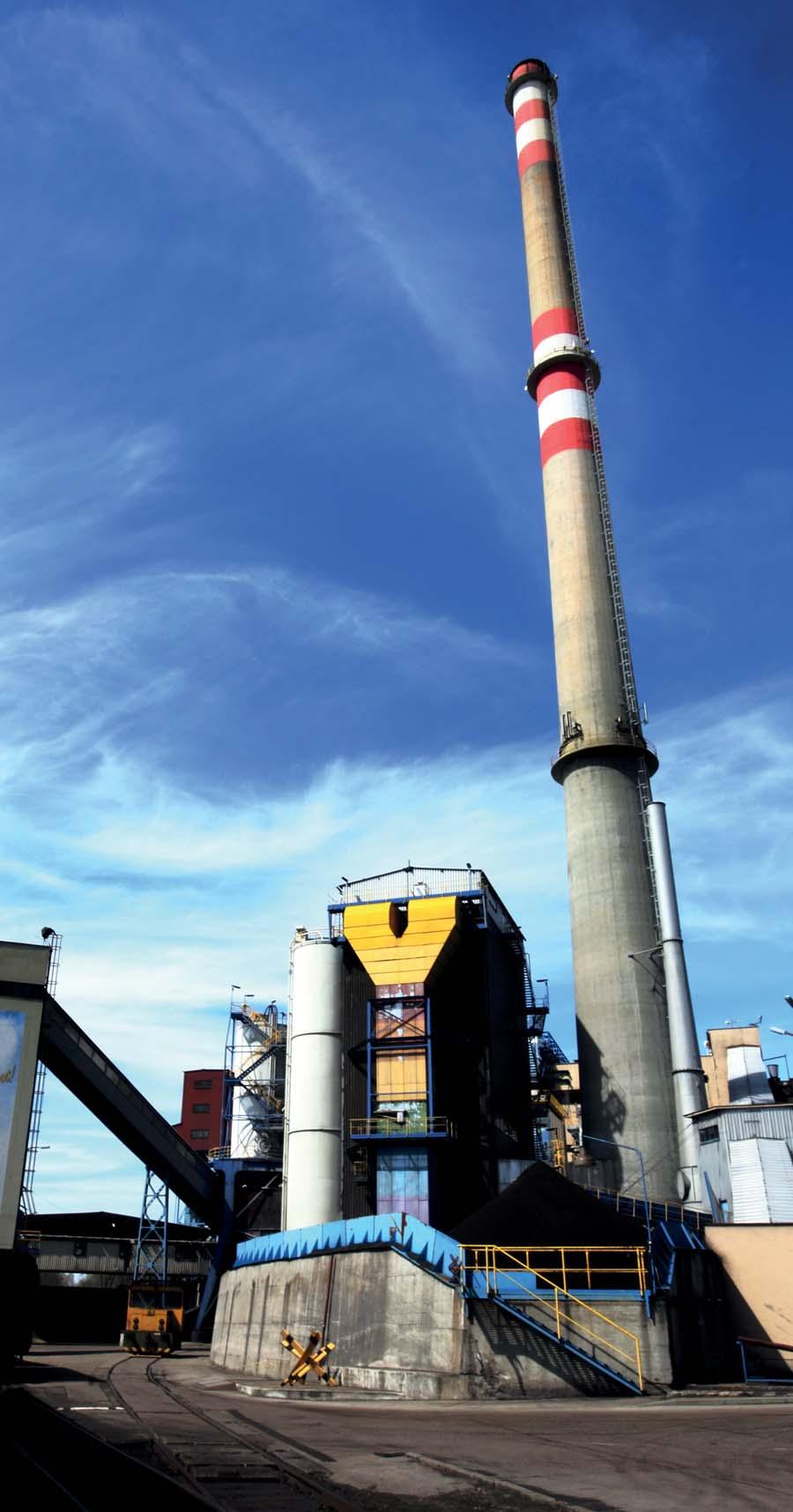
9 minute read
Digital twin of heating plant
from CITY:ONE.1.2020.EN
by CityOne
Digital twin of heating plant a challenge for Central heating
THE SYSTEM FOR INCREASING THE EFFICIENCY OF EXISTING THERMAL NETWORKS, USING CLOUD SOLUTIONS AND CONTROL OF OPERATIONAL DATA IN THE MINDSPHERE® COMPUTING ENVIRONMENT, WAS DEPLOYED FOR THE OPERATION OF THE HEATING PLANT IN THE TOWN OF STRAKONICE.
Advertisement
: Benefi ts of the digital twin
The digital twin is a basic digitization tool that allows you to optimize existing operations (economic and environmental benefi ts), identify failures and threats in a timely manner (digital records and predictions) and model possible investments before actual implementation (measure twice, cut once). The deployment of a digital twin at the Strakonice heating plant has shown that the system is suitable to reduce existing heat losses by 5%, otherwise necessarily arising from the production, treatment, and distribution of heat, which ultimately reduces overall fuel and other energy consumption in village.
: Threats to the heating industry
In connection with growing uncertainties in heat supply, municipalities and owners of houses and premises will face a fundamental decision in the next 10 years on how to continue to supply their buildings with energy, or how to operatively address their possible surpluses. Most municipalities in the Czech Republic and in neighbouring countries own a property in central heat supply systems, built up to 1990 and still more or less maintained. This means that the initial position in the decision-making of municipalities on further energy management may be infl uenced by already invested funds, often tied to the mandatory lifetime of already implemented plans in municipal or city energy.
In the current economic and legislative environment, central heating systems, especially those working with fossil fuels, especially coal, are in a very disadvantageous position. Even without a far-reaching economic analysis, it can be said that those heat productions that do not signifi cantly reduce the number of emissions (described, for example, by CO2) to produced and especially purposefully located (i.e., sold) megajoules of heat or megawatt of electricity will disappear within 20 years. The described demise of production and distribution networks will be gradual, but with devastating consequences for municipal or private investments already made in central heating systems.
There are ways to maintain and operate these systems in a competitively acceptable way in principle, the right way to centrally generate electricity and heat in our municipalities. However, this presupposes signifi cant changes in the production, distribution, but also organizational part of the existing heating systems.
: Digitization of heating industry – results from Strakonice
The basic advantage of the deployed digital system is that it does not require any investment in the heating networks themselves, their buildings or transfer stations. It is not an investment in additional „iron“, it is a matter of operating once and not always well-built heating systems in such a way as to give their maximum potential at minimum cost.
The system makes it possible to monitor the operating characteristics of the heating system constantly and systematically to prevent unnecessary outages and failures. These conditions often draw attention to us weeks in advance by certain parameter changes that are detectable in the MindSphere® environment. The result of the system‘s work is, in addition to the above prediction and optimization functions, a daily listing of problems that have occurred on the heating network in the last 24 hours.
The performed measurements and processing of measured data showed that multiple local measurements of physical quantities on heat exchangers and heat distribution of heating networks with direct data export to the cloud and its further processing in MindShere are currently a usable and suffi ciently reliable technology. Big data on important variables in the heating network will enable continuous optimization of the operation of the heating network or its sub-district. Since in this way the data will be accessible far into the past, based on this data, it is possible to create predictive models of heat consumption and use them in optimization.
no. Building Central heating [kW] Hot water [kW] Air handling unit [kW]
1273 community 20 - -
1253 Garden equipment shop 70 - -
1254 Restaurant with discotheque 70 20 150
1263 fl ats 110 110 -
1255 fl ats 110 110 -
1256 fl ats 110 110 -
1257 fl ats 110 110 -
1258 fl ats 110 110 -
1259 fl ats 180 150 -
1401 fl ats 60 90 -
Types of heat consumption and their calculated wattages - Line 1, based on VVS15 (PS)
In a specifi c case, the device is used to check the correct function of the heat distribution, with the proviso that „correctness“ in this case means not only functionality but also safety and economy. The installed system easily detects defi ciencies in the operation of heating networks every day, as well as problems with consumption in individual supplied buildings. The whole algorithm of the control of operating states is a tool for the main goals which are: the supply of heat in quantity and time course and on such parameters that the needs of customers are precisely met. No more, no less.
The system of intelligent control of heat networks with cloud outputs was developed by the research team of the Institute of Fluid Mechanics and Thermodynamics of the Czech Technical University, in cooperation with colleagues from the Technical University of Liberec and the University of West Bohemia in Pilsen. A team composed of employees of many research, production, and distribution companies, led at CTU by prof. Jiří Nožička and his colleagues, within the project TAČR TE01020036 „Centre of advanced technologies for production and distribution of electricity and heat“, in the years 2010 to 2019, developed and tested several technical solutions that are designed to increase the effi ciency of existing heating networks.
The equipment was installed, debugged and has been operated on the property of Teplárna Strakonice a.s. since the end of 2017, where the majority shareholder is the town of Strakonice. This is the district heating “Mír”, on the western edge of Strakonice, specifi cally its part, which is supplied by hot water branch No. 1 from the heat transfer station VVS15 (PS Mír).
The district heating district was selected from several possible localities based on the need for high variability of operation of individual supplied buildings. In the case of the measured hot water branch, there are 10 individually standing supply objects with very diff erent heat supply schedules. The predominance consists of residential houses of panel construction of the P1.11 system from the early 1980s, one modern apartment building of mixed concrete-brick construction from the fi rst decade of the 21st century, one community building, one technical-commercial enterprise and one restaurant with occasional weekend “disco” events. The heat inputs of individual buildings are shown in Table 1.
The aim of the measurement was to provide data from at least one year of operation of the selected hot water distribution system. The aim was also to fi nd out data from the heating of drinking water for hygienic purposes (hot water), which is being prepared in a substantial part of the supplied buildings.
Some aspects of economically optimal operation seem intuitively obvious. For example, heat losses increase with the temperature at the outlet of the source, respectively at the input of the considered subnet. However, it cannot simply be said that the economic optimum simply occurs at the lowest possible temperature at the entrance to the heating network. Too low temperature leads to the need for higher fl ow, and therefore higher output and consumption of circulating pumps.
For this reason, continuous optimization works with a criterion function that characterizes the optimization goals. If, as in this case, the optimization of one district, not the entire network including the source, is performed,
it makes sense to consider and minimize heat losses and operating costs of circulating pumps. At the same time, it can be required that the heat consumption that the main transfer station takes from the superior network is as even as possible. Such a criterion function must, of course, be supplemented by restrictive conditions which, with the use of the model, ensure that the required temperatures in the individual PCBs are maintained, heat consumption is covered, etc.
In principle, it would be possible to minimize the criterion function once with a horizon of, for example, one day. However, since the heat consumption can be predicted only with limited accuracy, it is more advantageous to repeat the optimization in each time step and always implement only the fi rst step of the schedule, i.e., use the principle of declining horizon, which is the basis of predictive control based on the model.
: Other benefi ts of measurement
A less expected benefi t of the measured data and their continuous evaluation was already (in the fi rst months of the supplemented measurement (2018)) the indication of several fi ttings inside the individual distribution sections, which did not fulfi l their function correctly. The signalling physical quantity in this case was the cyclically increasing temperature at the supply of cold drinking water to the hot water exchanger, even at a greater distance from this exchanger. A local investigation revealed a bad location of the non-return valves, which opened in the closing direction due to the expansion of the water and the air contained in the water. The non-return valves were moved to a more gravitationally suitable position and the said hygienically dangerous phenomenon was thus eliminated.
The step now done is the implementation of the above optimization tasks into the MindSphere environment. The current result is a continuous control of the main operating parameters of the hot water network (Figure 1) with the evaluation of the frequency of faults (Figure 2) and their dynamics (Figure 3) over time. Another goal is the permanent determination of the optimization goal based on the previous development of the measured quantities and the possible prediction of these quantities and the ambient conditions of the solved heating network. Here, in the MindSphere environment, the output will be the recommended setting of thermal network parameters implemented through proposals to change the settings of controlled variables, such as fl ows, temperatures, etc. This approach to control can then be compared with the method of constantly learning neural networks.
#Pavel Sláma, Jiří Ehrlich, ČVUT Prague
Figure 1: Alerts on operation failures of distribution/transferring stations for a particular day
Figure 2: Description and duration of single incorrect and ineffi cient operation states for one distribution section
Figure 3: Checking the dynamics of operating deviations from the detailed course of physical quantities measured at each of the house transfer stations.

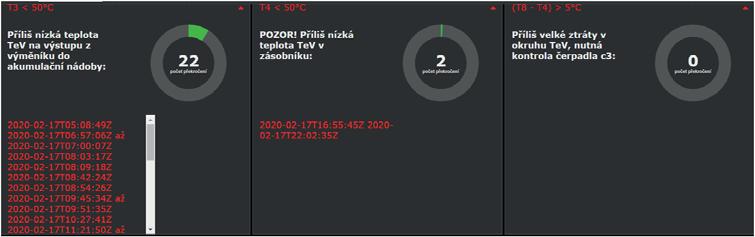
