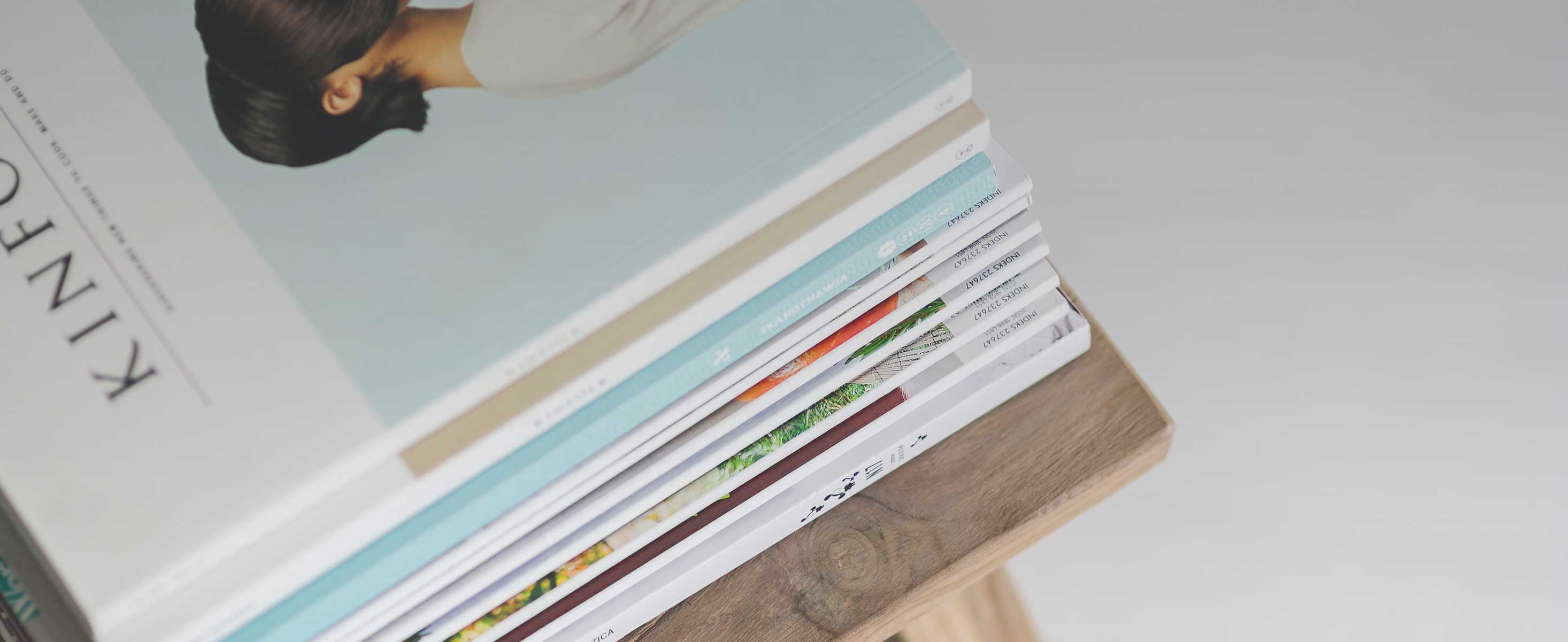
6 minute read
Table 3: Collecting data on a portfolio company’s supply chain sites
While Approach I, using firm-specific supply chain data, is the most accurate, financial institutions and companies are not (yet) able to easily gather information at scale, as firm-specific supply chain data is patchy and largely incomplete (Climate & Company, 2021). Therefore, building on existing work (Banque de France, 2021), Approach II, using IO models, offers a second complementary, less accurate, but more practical alternative, based on sector- and country-level averages. IO models are generally derived from supply and use statistics from national account databases and help to show supply chains between industries and countries.
The following subchapters introduce the two approaches identified in more detail and discuss their coverage, advantages and disadvantages as well as available data points to approximate the required WWF BRF input data. The subchapters also contain workarounds to address typical data challenges, such as missing values. It ends with an overview of the comparison and recommendations regarding the available approaches.
Advertisement
1. COMPANY-SPECIFIC SUPPLY CHAIN DATA (APPROACH I)
Table 3 below describes the required data structure to assess the supply chain-related risks faced by the company of interest.
Table 3: Collecting data on a portfolio company’s supply chain sites
1: Identify suppliers
Company name Supplier Importance of that relationship
Company A
Supplier 1 0.5
Supplier 1
Supplier 1
Supplier 2 0.5
Supplier 2
Supplier 2
Relevance Required to derive supply chain risks for Firm A
2: Collect required input data per supplier (see Guidance A)
Location of company sites Industry classification Business Importance
Lat/long Industry A %
Lat/long Industry A %
Lat/long Industry A %
Lat/long Industry B %
Lat/long Industry B %
Lat/long Industry B %
Required as input data to the WWF BRF tool
1. Identifying a company’s suppliers, including a weighting score per supplier-customer relationship
Identifying the list of suppliers
Ideally, the user receives a list of supply chain relationships for the company of interest (Firm A) along with a quantification of their (financial) importance. Different third-party data providers, such as FactSet,18 Bloomberg,19 or Refinitiv,20 provide supplier-customer relationship data in a structured format. Data is collected by leveraging information based on the target company disclosing its suppliers.21 One way to make the data set more complete is to add relationships based on reverse disclosures.22
18 Data product: FactSet Revere Supply Chain Relationships. FactSet data has been used for testing purpose. Therefore, data quality (coverage and accuracy) might differ subject to the chosen provider.
19 Data product: Bloomberg Global Supply Chain Data.
20 Refinitiv provides more information here.
21 For example, Nestle decided to disclose a list of its suppliers, covering 95 per cent of the company’s annual sourcing of raw materials.
Identifying the importance of each supplier
A logical measure of the financial importance of the customer-supplier relationship is the revenue dependency of the company on each of its suppliers as a fraction of the total revenue of that company. There are three potential ways to integrate this measure, in decreasing order of accuracy23:
• Solution 1 – Disclosed data: Third-party providers collect financial figures of supplier-customer relationships as part of their supply chain data products (example: Firm A is a customer of Firm B, generating X% of Firm B’s revenue). However, this data is rarely disclosed: for a sample of 1461 listed companies headquartered in the EU, we only retrieved (incomplete) financial figures for 13% of the sample24 . • Solution 2 – Estimates: In the absence of disclosed data, the user could derive estimated weights by determining the relative importance of the supplier-customer relationships25. FactSet, for example, provides an ordinal relevance ranking to fill the gaps mentioned in Solution 1. Based on FactSet Supply
Chain Relationships data, a proprietary algorithm scores each relationship based on some hand-picked features (i.e., mutual disclosure, industry type, geography, etc). Users could also attempt to rank such relationships themselves. They would then have to transform this ranking into a normalised list of weights by making an assumption on how financial importance behaves as a function of the firm’s place in the ordered list of suppliers. An example with four suppliers (S1, S2, S3 and S4) and an assumed linear relationship (starting with a minimal financial importance of 0.1), would be to assign the weights 0.4, 0.3, 0.2 and 0.1.
• Solution 3 – Equal weighting: The user equally weights all identified suppliers of a specific company.
2. Collecting location-specific proxy data on a supplier’s operational sites (see Guidance A)
Feeding the data on the portfolio companies’ supply chain sites into the WWF BRF (or WRF) Assess Module, requires location-specific data on the portfolio companies’ suppliers. This proxy data can be generated in a similar manner to that used for a company’s operational site. This is further explained in Guidance A.
2. INPUT-OUTPUT MODELS (APPROACH II)
In the absence of comprehensive, firm-specific supply chain data, a well-established way to gain insights into the supply chain links between different industries of the economy is to use IO models (see Figure 11). This has already been applied by some actors in this field, including Banque de France and the World Economic Forum (WEF)26 and could be used as a bridging solution (until supply chain data is collected or becomes available).
Input-output tables are generally derived from supply and use statistics from national account databases, and thereby rely on sectoral averages at state level rather than relying on more granular firm-specific data. In more recent state-of-the-art IO models, international databases as well as trade statistics are also incorporated.
23 These methods also to apply to other potential metrics such as % of cost of goods or amount paid for purchased goods.
24 To get an idea on the order of magnitude, we assessed a sample of 1461 listed companies headquartered in the EU. For 87% of the sample (1261 companies) we retrieved no financial figures on supplier-customer relationships at all. For the remaining 13% at least some financial figures were stated by the source companies (Source: based on data from FactSet’s Supply Chain Relationship database in 2022; reverse disclosures were not considered).
25 Example: a steel company is likely to be a more important supplier for an automotive company than, say, a supplier producing office materials.
26 While an extensive overview is beyond the scope of this chapter, it is informative to look at some relevant existing approaches. Firstly, the WEF and PwC
Report Nature Risk Rising (WEF, 2020) compares direct and indirect dependencies across industry sectors using a preliminary version of ENCORE’s dependency ratings. The authors used a global Multi-Region Input Output (MRIO) model to identify the sectors in the supply chain which were then weighted according to high, medium and low dependency. Secondly, the paper by Svartzman et al (2021): A ‘Silent Spring’ for the Financial System? (Banque de France, 2021) explores biodiversity-related financial risks for the French financial system (including transition risks as well as direct and indirect/upstream dependencies) using a sectoral approach which, in turn, was inspired by the pioneering study by van Toor et al. (2020). The study finds that 42 per cent of the value of securities held by French institutions are highly or very highly dependent on at least one ecosystem service. Finally, the
Science Based Targets Network’s business guide also contains an upstream/downstream dependency/impact rating, which builds on linking ENCORE with a specific MRIO called EXIOBASE (SBTN, 2020b).