BERNOULLI’S FALLACY
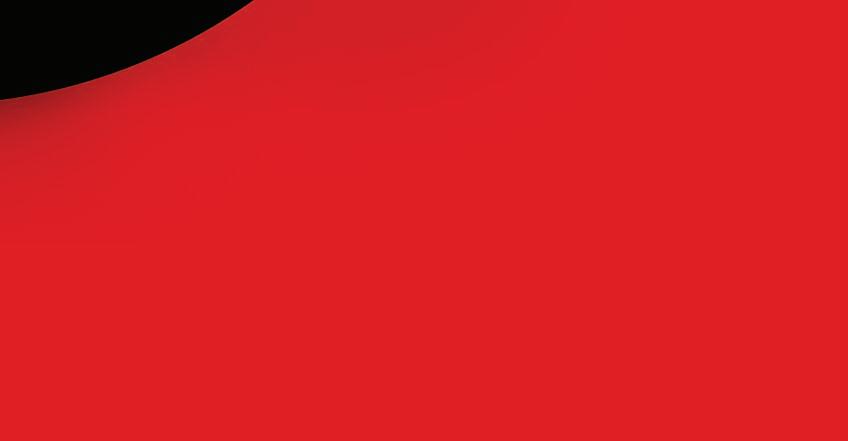
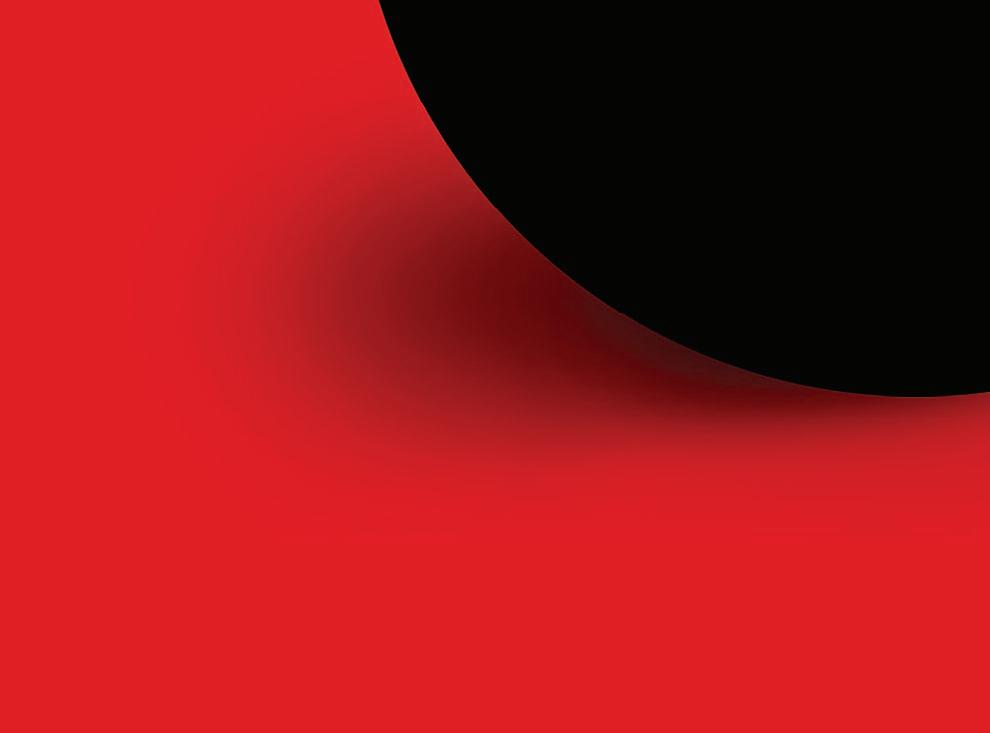
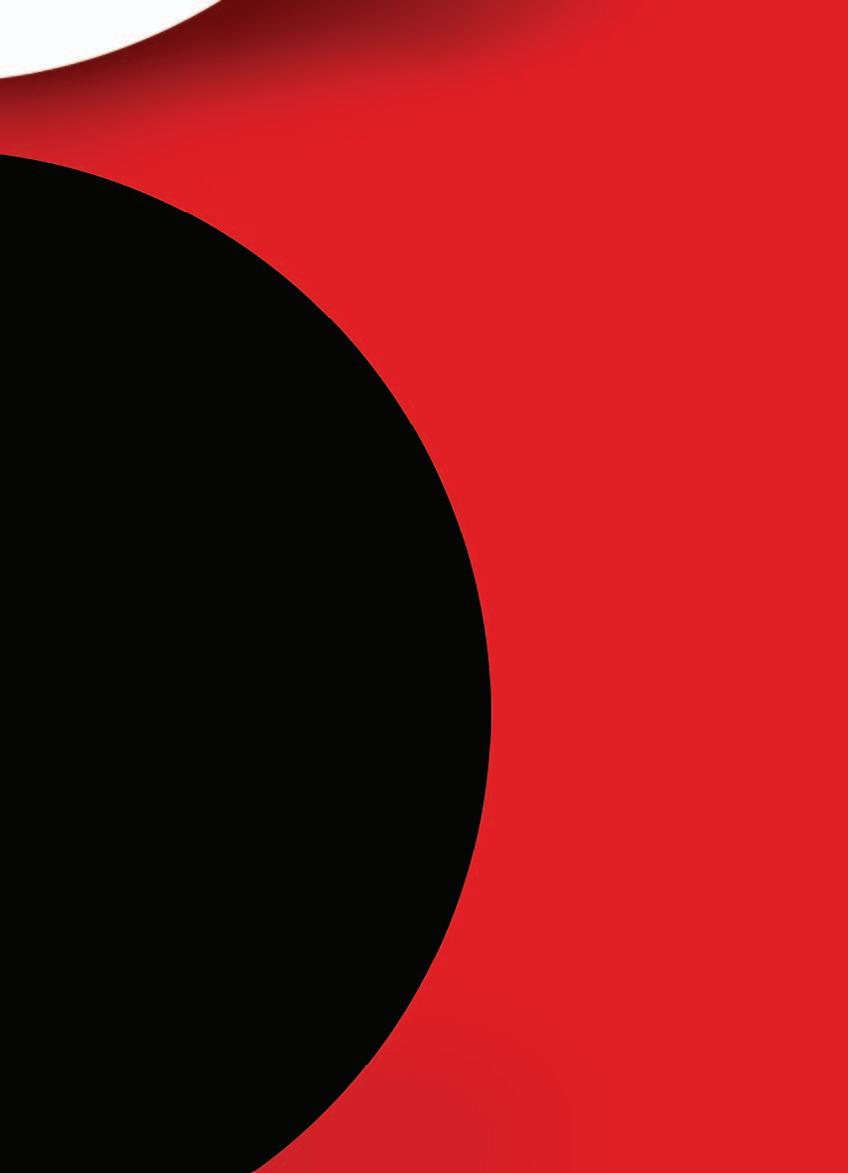
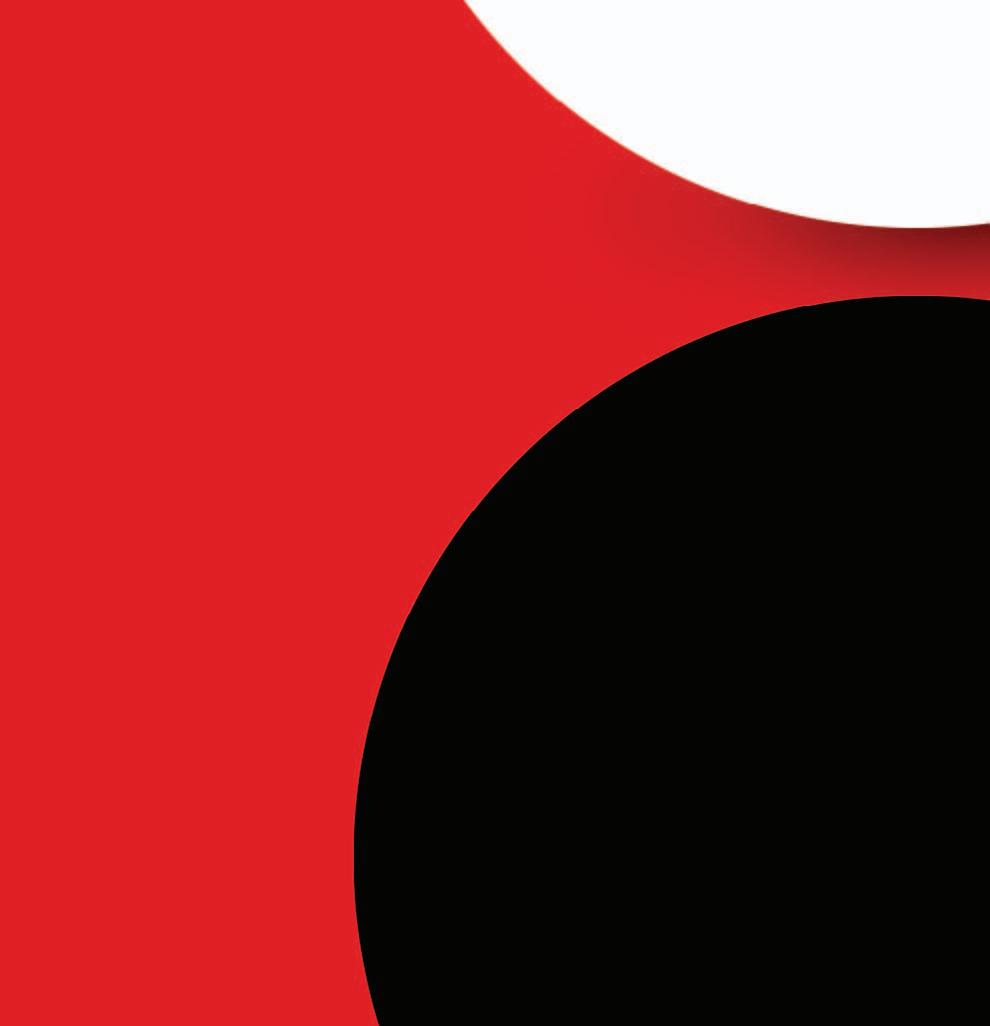
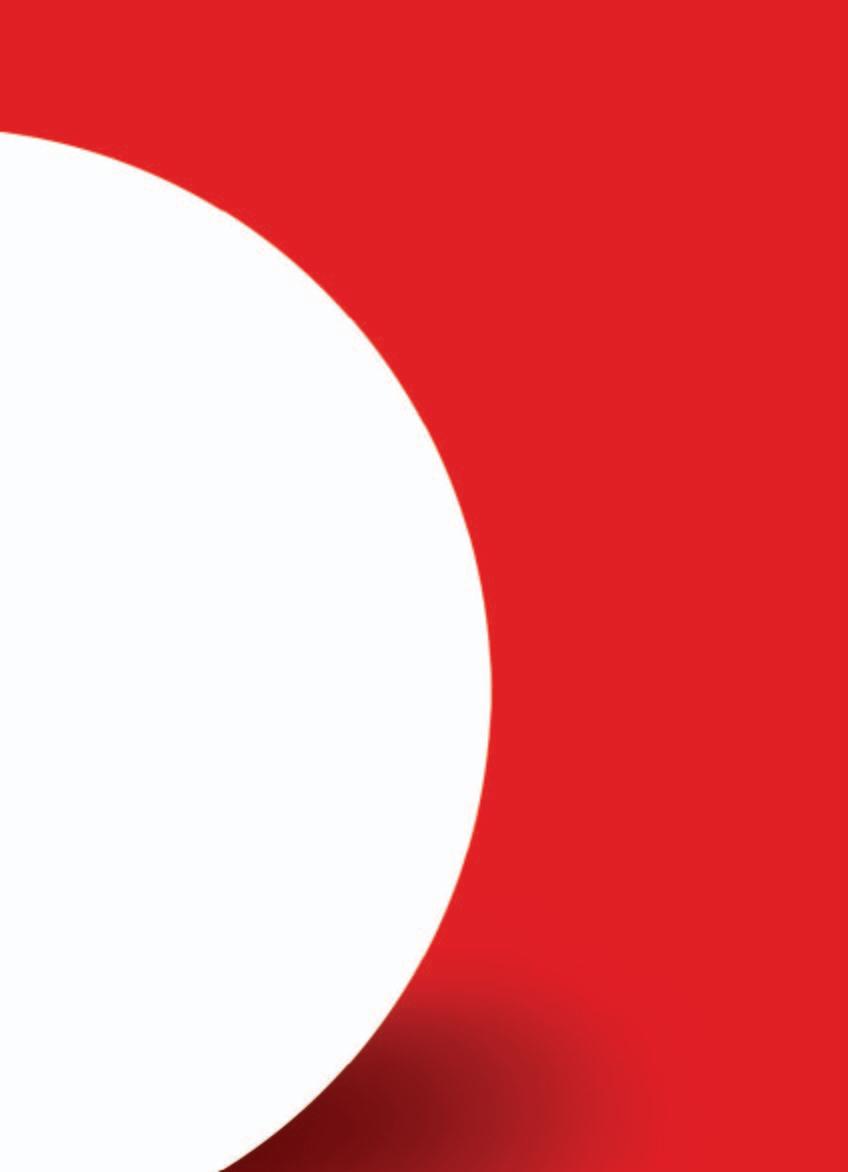
Statistical Illogic and the Crisis of Modern Science
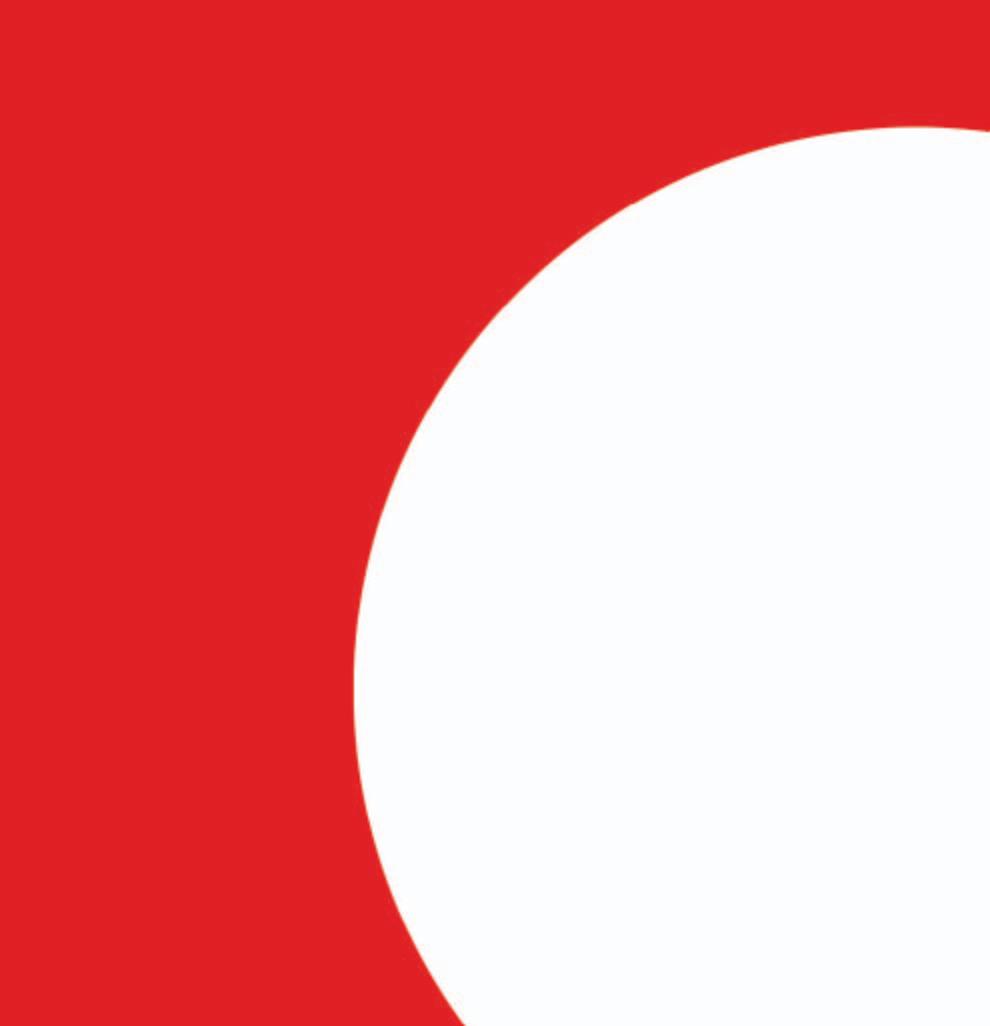
Since this book risks being accused of relitigating old arguments about statistics and science, let us first dispense with the idea that those arguments were ever settled. The “statistics wars” never ended; in some ways they have only just begun.
Science, statistics, and philosophy need each other now as much as ever, especially in the context of the still unfolding crisis of replication. Everyone regardless of ideology can likely agree that something is wrong with the practice of statistics in science. Now is also the right time for a frank conversation because statistical language is increasingly a part of our daily communal lives. The COVID- pandemic has, sadly, forced statistical terms like “test sensitivity,” “specificity,” and “positive predictive value” into our collective lexicon. Meanwhile, in other recent examples, (spurious) statistical arguments were a core component of the allegations of electoral fraud in the U.S. presidential elections, and (non-spurious) statistical arguments are central to the allegations of systemic racial bias in the U.S. criminal justice system. The largest stories of our time—in public health, education, government, civil rights, the environment, business, and many other domains—are being told using the rhetorical devices of statistics. So the recognition that statistical rhetoric might lend itself to misuse makes this an urgent problem with an ethical dimension. On that we can probably also agree.
What to do about it is another matter. In science, several proposed methodological changes (discussed in the following) have gained support as potential solutions to the replication crisis, but there are no clear winners yet. The reason consensus is hard to come by is that there are unresolved foundational questions of statistics lurking within these debates about methods. The discussions happening now can, in fact, be seen as a vibrant remixing of the same philosophical issues that have colored the controversies about statistics since
the s. In short, assessing whether a proposed change successfully fixes a problem requires one to first decide what the problems are, and such decisions reveal philosophical commitments about the process by which scientific knowledge is created. When it comes to such foundational questions, we are not all on the same page, for reasons explored in this book.
Because statistical methods are a means of accounting for the epistemic role of measurement error and uncertainty, the “statistics wars” (at least on the frequentist versus Bayesian front) are best described as a dispute about the nature and origins of probability: whether it comes from “outside us” in the form of uncontrollable random noise in observations, or “inside us” as our uncertainty given limited information on the state of the world. The first perspective limits the scope of probability to those kinds of chance fluctuations we can, in principle, tabulate empirically; the second one allows for probability to reflect a degree of confidence in a hypothesis, both before and after some new observations are considered. Unfortunately for the conflict-averse, there is no neutral position here.
As a snapshot of the ways these philosophical commitments are now playing themselves out in practice, consider that much of the current debate about statistical and scientific methods can be organized into three categories of concerns: Where does the hypothesis come from and when? If a particular hypothesis, representing a concrete prediction of the ways a research theory will be borne out in some measured variables, is crafted after peeking at the results or going on a “fishing expedition” to find a version that best suits the available data, then it may be considered a suspicious product of “post hoc theorizing,” also known as hypothesizing after results are known (“HARKing”), taking advantage of “researcher degrees of freedom,” the “Texas sharpshooter fallacy,” “data dredging,” the “look-elsewhere” effect, or “p-hacking.” Various proposals to combat this include the pre-registration of methods, that is, committing to a certain rigid process of interpreting the data before it has been gathered, sequestering the “exploratory” phase of research from the “confirmatory” one, or correcting for multiple possible comparisons, as in the Bonferroni correction (dividing the threshold for significance by the number of simultaneous hypotheses being considered) or others like it.
What caused the experiment to begin and end, and how did we come to learn about it? Subcategories of this concern include the problem of “publication bias,” or the “file drawer problem,” and the problem of “optional stopping.” If, say, an experimenter conducting a trial is allowed to keep running the experiment and collecting data until a favorable result is obtained and only then
report that result, there is apparently the potential for malfeasance. Attempts to block this kind of behavior include making publication decisions solely on the basis of the pre-registered reports—that is, based purely on the methods—to encourage publishing negative results, and for the “stopping rule” to be explicitly specified ahead of time and adhered to.
Is there enough data? Small samples are the bane of scientists everywhere, for reasons ranging from lack of resources to the phenomena of interest being inherently rare. In the standard statistical framework, this creates a problem of low power, meaning a high chance of failing to find an effect even though it’s present. It also, perversely, means that an effect—if it is found—is likely overstated and unlikely to be replicable, a paradox known as the “winner’s curse.” A different but related dynamic is at work when statistical models with many parameters are “overfit” to the available data. Too few data points are asked to carry too heavy a load, and as a result the model may look good when evaluated on a training data set and yet perform miserably elsewhere. Apart from simply collecting larger data samples (easier said than done), the emerging best practice recommendations are to facilitate collaboration by sharing resources and materials, incentivize replication studies and meta-analyses, reserve some amount of data for “validation” or “out-of-sample testing” of any model being fit, and perform power analyses to determine how large a sample is needed to find a meaningfully sized effect with high probability.
All three appear at a glance to be legitimate causes for concern, and the proposed solutions appear to be sensible countermeasures, but only if the standard (non-Bayesian) mode of doing statistical inference is assumed as a given. As we’ll see, Bayesian statistics provides natural protection against these issues and, in most circumstances, renders them non-issues. The safeguard, missing completely from the standard template, is the prior probability for the hypothesis, meaning the probability we assign it before considering the data, based on past experience and what we consider established theory.
Upon further reflection, it becomes apparent that many of these proposed limitations are at odds with common sense about the way hypotheses are usually formed and tested in light of the evidence. For example, a doctor measuring a child’s height might report that measurement including some error margins, indicating the best guess for the true value and a probability distribution around it, but strictly speaking that hypothesis would be made after the results were known—violating the rules established to protect us from concern (). Is the doctor now obligated to measure the child again as a “confirmatory” analysis? Many of the prototypical examples of basic statistics like surveying
a population by random sampling would not pass such scrutiny. Nor would, say, the application of probability to legal evidence. If a suspect to a crime is identified based on evidence gathered at the scene, can their probable guilt also be established by that same evidence, or must all new evidence be gathered? Is a reviewer for a scientific journal, in the course of critiquing a paper, allowed to conjure up alternative explanations to fit the reported data, or must those alternatives also be pre-registered?
The problem of publication bias—the bulk of concern ()—is exacerbated by the standard statistical methods because they unnaturally level the playing field between all theories, no matter how frivolous and outlandish. So, of course, among the theories that meet a given standard of publication-worthiness, the more surprising and counterintuitive ones (and those least likely to actually be substantive) will tend to get more attention. Requiring more surprising hypotheses to meet a higher standard of evidence would realign publication incentives and remove much of the chaff. Similarly, as we’ll see, “optional stopping” is a source of anguish for standard methods only because they’re sensitive to the possibility of what could have happened but did not. Bayesian inferences are based only on what was actually observed, and the experimenter’s plans for other experiments usually do not constitute relevant information.
Similarly, the problems in ()—low statistical power and overfitting of models—are only problems if the answer from a statistical procedure is interpreted as final. In the Bayesian mode, hypotheses are never definitively accepted or rejected, nor are single estimates of model parameters taken as gospel truth. Instead, uncertainty can change incrementally as more data is collected; a single observation can be useful, two observations more so, etc. The more simultaneous questions being answered, in the form of many adjustable dials in a given model, generally speaking the more data will be required to reduce that uncertainty to manageable levels, but all along the way we will be reminded where we “are,” inferentially speaking, and how much further we have to go. Reserving data to use for validating models is a waste of perfectly good data from which we could have learned something.
All of the above and more is possible if we’re simply willing to let probability mean uncertainty and not just frequency of measurement error. So, first we need to get over that philosophical hump. To put the case another way: compared with Bayesian methods, standard statistical techniques use only a small fraction of the available information about a research hypothesis (how well it predicts some observation), so naturally they will struggle when that limited information proves inadequate. Using standard statistical methods is like driving a car at
night on a poorly lit highway: to keep from going in a ditch, we could build an elaborate system of bumpers and guardrails and equip the car with lane departure warnings and sophisticated navigation systems, and even then we could at best only drive to a few destinations. Or we could turn on the headlights.
So, while the floor is open for a discussion of “questionable research practices,” it’s the perfect time to question whether some of those practices are questionable, or whether there might be a better way to think about the whole scheme.
To that end, contained in this book are suggestions concerning probability and statistical inference that at first will appear heretical to anyone trained in statistical orthodoxy but that might, with some meditation, seem more and more sensible. The common theme is that there would be no need to continually treat the symptoms of statistical misuse if the underlying disease were addressed.
I offer the following for consideration:
Hypothesizing after the results of an experiment are known does not necessarily present a problem and in fact is the way that most hypotheses are ever constructed.
No penalty need be paid, or correction made, for testing multiple hypotheses at once using the same data.
The conditions causing an experiment to be terminated are largely immaterial to the inferences drawn from it. In particular, an experimenter is free to keep conducting trials until achieving a desired result, with no harm to the resulting inferences.
No special care is required to avoid “overfitting” a model to the data, and validating the model against a separate set of test data is generally a waste.
No corrections need to be made to statistical estimators (such as the sample variance as an estimate of population variance) to ensure they are “unbiased.” In fact, by doing so the quality of those estimators may be made worse.
It is impossible to “measure” a probability by experimentation. Furthermore, all statements that begin “The probability is . . .” commit a category mistake. There is no such thing as “objective” probability.
Extremely improbable events are not necessarily noteworthy or reason to call into question whatever assumed hypotheses implied they were improbable in the first place.
Statistical methods requiring an assumption of a particular distribution (for example, the normal distribution) for the error in measurement are perfectly valid whether or not the data “actually is” normally distributed.
It makes no sense to talk about whether data “actually is” normally distributed or could have been sampled from a normally distributed population, or any other such construction.
There is no need to memorize a complex menagerie of different tests or estimators to apply to different kinds of problems with different distributional assumptions. Fundamentally, all statistical problems are the same.
“Rejecting” or “accepting” a hypothesis is not the proper function of statistics and is, in fact, dangerously misleading and destructive.
The point of statistical inference is not to produce the right answers with high frequency, but rather to always produce the inferences best supported by the data at hand when combined with existing background knowledge and assumptions.
Science is largely not a process of falsifying claims definitively, but rather assigning them probabilities and updating those probabilities in light of observation. This process is endless. No proposition apart from a logical contradiction should ever get assigned probability , and nothing short of a logical tautology should get probability .
The more unexpected, surprising, or contrary to established theory a proposition seems, the more impressive the evidence must be before that proposition is taken seriously.
Of course, I’m far from the first person to write down such theses and nail them to the door of the church of statistics. What follows will not be anywhere near a comprehensive account of the history or current status of the statistics debates, nor the multitude of unique perspectives that have been argued for. No two authors, it seems, have ever completely agreed on the foundations of probability and statistics, often not even with themselves. Roughly speaking, though, the version presented here descends most closely from the intellectual lineage of Pierre-Simon Laplace, John Maynard Keynes, Bruno de Finetti, Harold Jeffreys, Leonard Jimmie Savage, and Edwin Jaynes.
I have tried wherever possible, though, to avoid taxonomizing the camps of people who have contributed to that vast body of literature because I don’t think it adds much to the story. If anything, reducing someone’s position via shortcuts like “[X]ive [Y]ist [Z]ian” and so on can convey the wrong idea since, depending on context, the meanings of [X], [Y], and [Z] may imply shifting and contradictory conclusions as they’ve been used differently over centuries. There is, in particular, a danger in allowing various subcamps to be labelled “objective” and “subjective,” as these have historically been used mostly as ad copy to sell the different schools of thought.
For our purposes, most of the nuanced differences between the positions staked out by these camps are irrelevant, anyway. What concerns us is one essential question: Is it possible to judge hypotheses based solely on how likely or unlikely an observation would be if those hypotheses were true? Those who answer in the affirmative, whether they be Fisherians, neo-Fisherians, NeymanPearsonians, equivalence testers, “severe testers,” and so on, commit the fallacy that is our subject. Those who answer in the negative have at least avoided that trap, though they may fall into others.
Nor should this book be interpreted as a work of original scholarship. All the main ideas presented here exist elsewhere in higher resolution detail. I’ve attempted as much as I can to provide the necessary references for an interested reader to follow any thread for more detail. (The answer, in the majority of cases, is Jaynes.)
Consider this, instead, a piece of wartime propaganda, designed to be printed on leaflets and dropped from planes over enemy territory to win the hearts and minds of those who may as yet be uncommitted to one side or the other. My goal with this book is not to broker a peace treaty; my goal is to win the war. Or, to use a less martial metaphor, think of this as a late-night infomercial for a genuinely amazing product, pitched at those researchers cursed with sleepless nights spent crunching statistical analyses and desperately hoping for a “p < .” ruling from an inscrutable computer judge. Over a black-and-white video of such a researcher grimacing and struggling to get their appliance to simply function while the complex, clunky machinery of significance tests, power analyses, and multiple comparison corrections finally falls apart in a chaotic mess, a voice asks knowingly, “Are you tired of this always happening to you?” Our exasperated scientist looks up at the camera and nods. “Don’t you wish there were a better way? Well now, thanks to Bayesianism, there is . . .”
“An entertaining mix of history and science.”
“I like it! Anything that gets people thinking about the uses and abuses of statistics is important and Aubrey Clayton’s book does just this. Fifty years ago, E. T. Jaynes opened my eyes to the importance of Bayesian ideas in the real world and this readable account brings these ideas up to date.”
“This story of the ‘statistics wars’ is gripping, and Clayton is an excellent writer. He argues that scientists have been doing statistics all wrong, psychology, the social sciences, and other empirical disciplines. Few books accessible to a broad audience lay out the Bayesian case so clearly.”
—ERIC-JAN WAGENMAKERS, COAUTHOR OF BAYESIAN COGNITIVE MODELING: A PRACTICAL COURSE