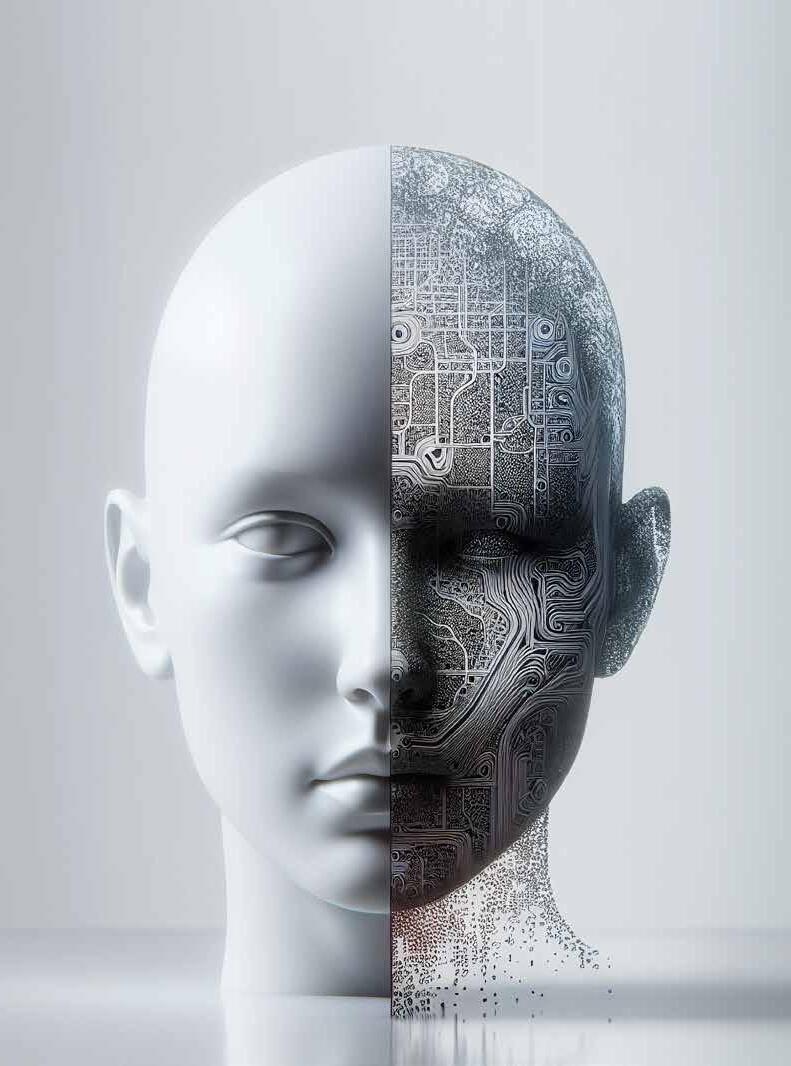
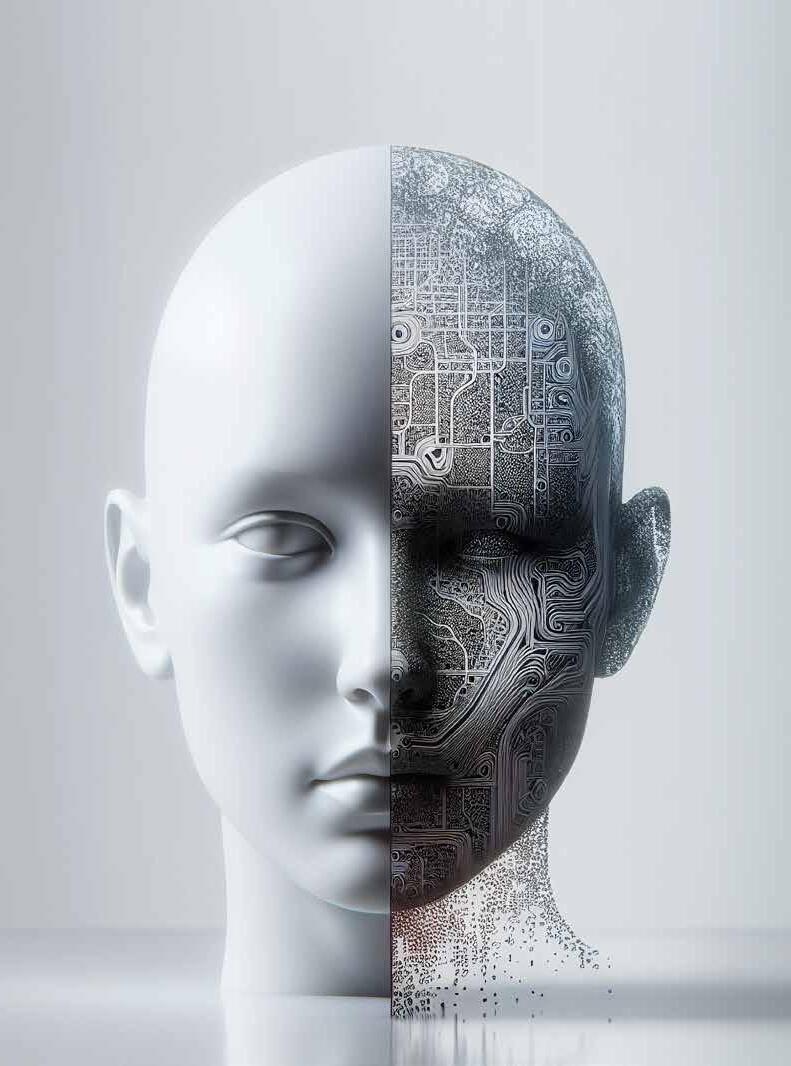
IMPACT OVER HYPE
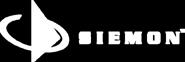
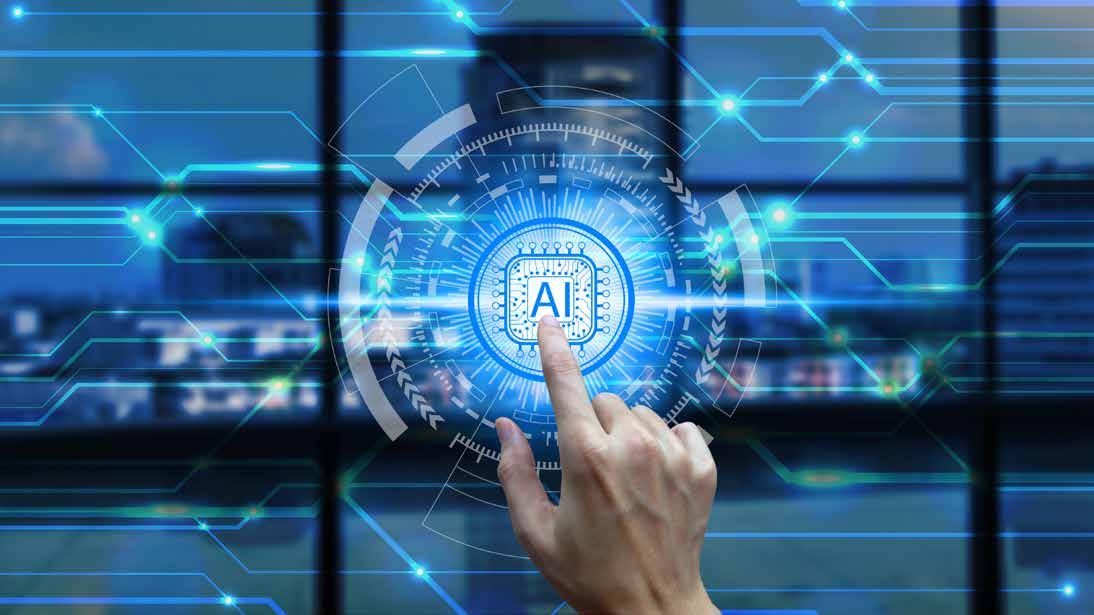
800G 400G High Speed Cable Assemblies
Siemon is AI-Ready
AI ready Fiber Cabling Solutions
Data Centre Design Services
Siemon is at the frontier of the GenAI revolution and through Collaboration with clients, w e’ve developed a range of next-generation AI-Ready solutions that are ready to suppor t your IT Network deployments. Are
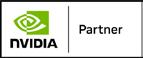
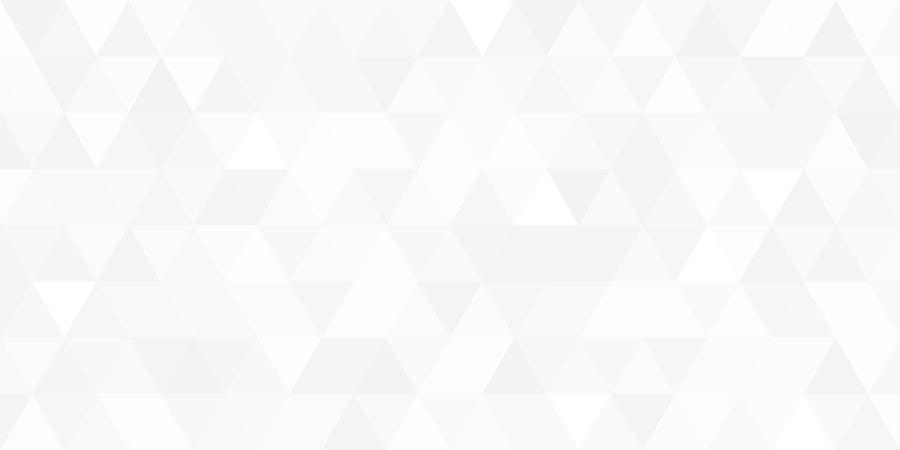
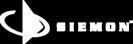
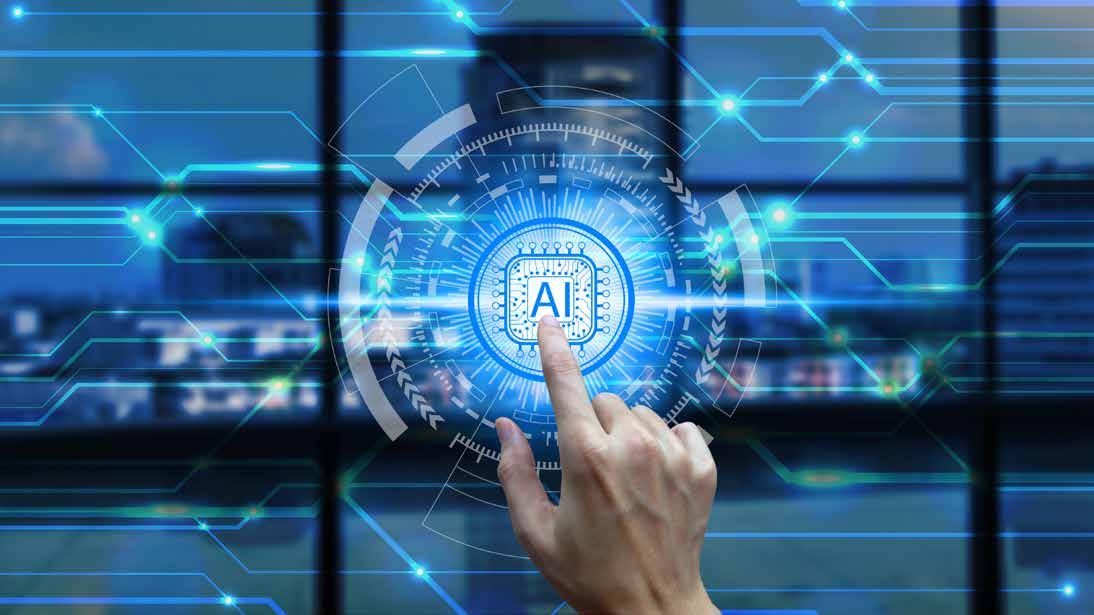
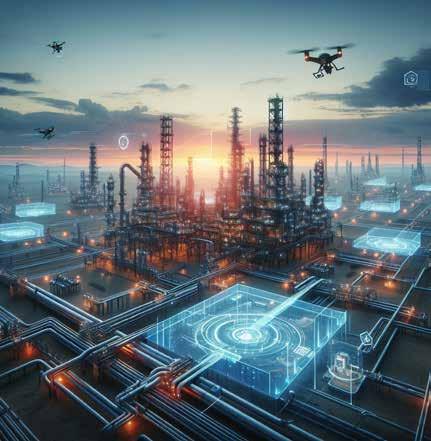
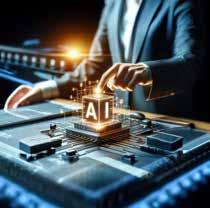
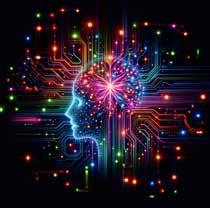

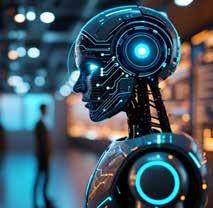
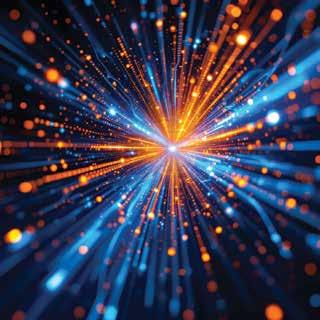
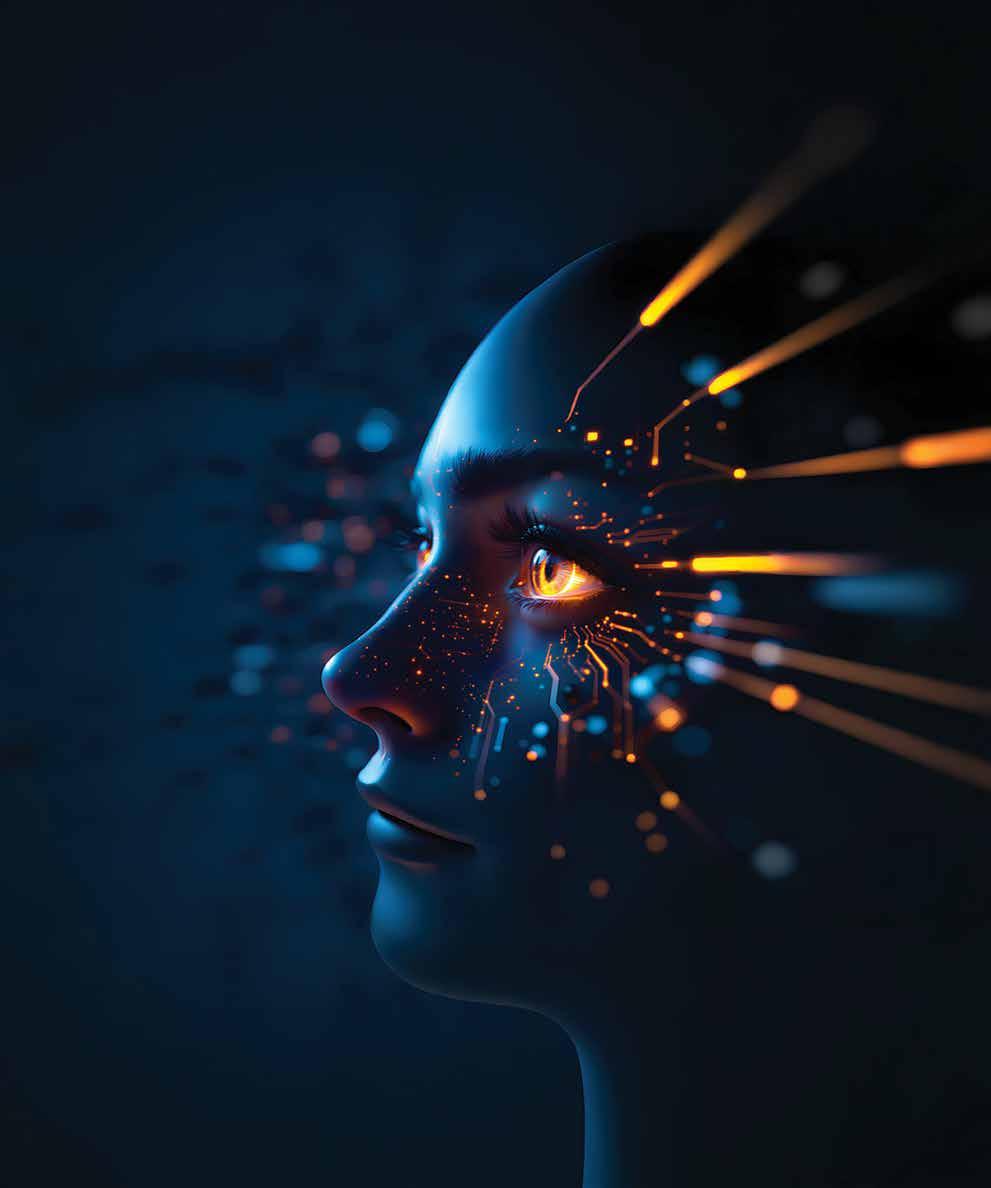

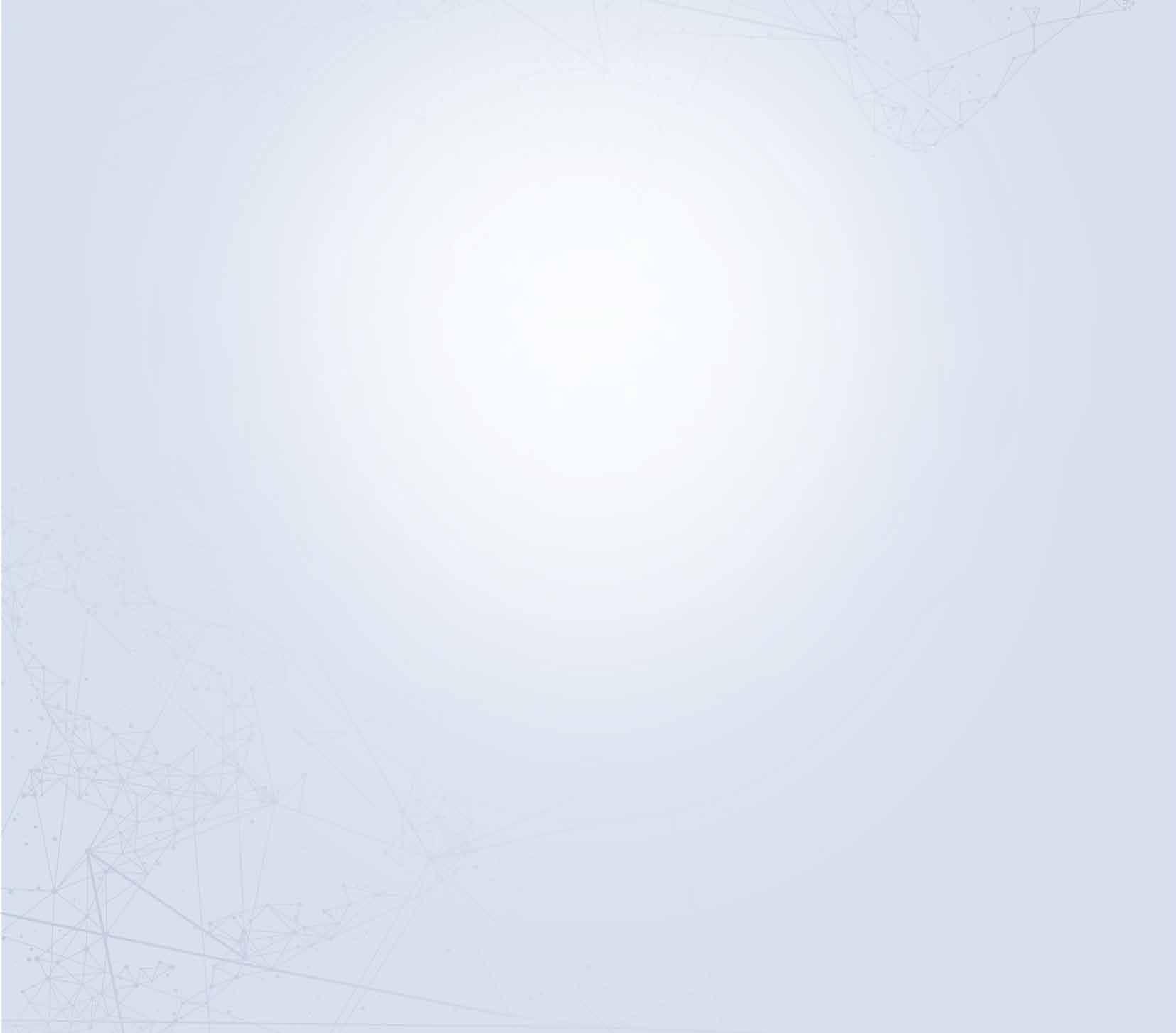
Published by
Publication licensed by
AI in action
Welcome to the inaugural edition of the AI Insights booklet—a curated exploration of how artificial intelligence (AI) is redefining leadership priorities and unlocking new sources of enterprise value.
This edition brings together voices from across the AI spectrum—from pioneers building scalable data infrastructure to leaders championing transparency, contextual intelligence, and human-machine collaboration.
According to IDC, global spending on technology to support AI is expected to reach $337 billion in 2025, underscoring the growing urgency for enterprises to operationalise AI across the value chain. This shift from experimentation to execution is a central theme throughout the thought leadership shared in this booklet. The C-suite is no longer asking if AI should be adopted, but how best to integrate it into their business models— securely, responsibly, and at scale.
AI Insights invites reflection on how we lead, build, and grow in the age of intelligent technologies. It challenges business leaders to shape not just digital strategies, but digital futures.
Fittingly, the imageries featured in this booklet was generated using AI—an artistic nod to the very technologies shaping the ideas within these pages.
Happy reading!
Managing Editor
Adelle Geronimo
adelleg@insightmediame.com +97156 - 4847568
Production Head
James Tharian
jamest@insightmediame.com +97156 - 4945966
Commercial Director
Merle Carrasco merlec@insightmediame.com +97155 - 1181730
Administration Manager
Fahida Afaf Bangod fahidaa@insightmediame.com +97156 - 5741456
Operations Director
Rajeesh Nair
rajeeshm@insightmediame.com +97155 - 9383094
Designer
Anup Sathyan
While the publisher has made all efforts to ensure the accuracy of information in this magazine, they will not be held responsible for any errors
The CXO Insight ME team

Jim Chappell / AVEVA
Powering Industry 5.0
Jim Chappell, Global Head of AI at AVEVA, explores how Agentic AI is reshaping industrial innovation—bridging human expertise with autonomous intelligence
The industrial sector has always been a benchmark for progress— pushing the limits of scale, precision, and efficiency. From assembly lines to smart factories, every leap has been driven by technology. Today, the sector stands at the cusp of another transformation: shifting from automation to proactive intelligence.
For over a decade, Industry 4.0 has delivered gains through automation, IoT, and digitisation. But today’s demands—sustainability, resilience, operational complexity, and the need for more human-centric environments— are driving the adoption of smarter, more adaptive technologies.
Artificial intelligence is central to this evolution. Once primarily used for task automation and retrospective data analysis, AI is now a strategic enabler of real-time decisionmaking and adaptive operations. In the context of Industry 5.0, AI is emerging as a true collaborator. What’s unique about Industry 5.0 is the emergence of Agentic AI—a new generation of intelligent systems that go beyond processing information to actively learning, reasoning, and making decisions with minimal human intervention. These AI agents are capable of adapting to changing conditions, amplifying human expertise, and unlocking new levels of performance, sustainability, and efficiency.
“AI is evolving into dynamic partners, acting as ‘intelligent agents’ capable of autonomous action, continuously learning from complex data,” says Jim Chappell, Global Head of AI at AVEVA. “This marks a significant step toward Agentic AI and brings us closer to Artificial General Intelligence (AGI), or true human-like intelligence.”
While AGI remains a longer-term ambition, Agentic AI represents a turning point in how industrial enterprises approach efficiency, sustainability, and resilience.
Take digital twins, for example. AI now plays a key role in automating data mapping across different systems, dramatically cutting down manual workloads and speeding up deployment.
“AI can identify, and link equipment labelled differently across systems, achieving 70–80 percent accuracy and allowing human reviewers to focus on complex cases,” explains Chappell.
Such breakthroughs are redefining how engineers and operators interact with technology.
Chappell cites AVEVA’s own AI assistant as a case in point. “Currently, it answers basic queries, but with Agentic AI, it will conduct deeper analytics, autonomously collecting data, running calculations, and generating insights.” Future iterations will not just assist—they’ll monitor systems, detect anomalies, and trigger alerts when operational thresholds are exceeded.
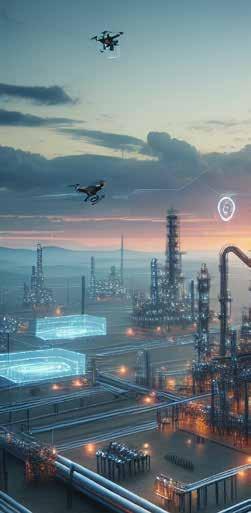
Responsible AI: Ethics Meets Innovation
As AI’s capabilities grow, so does the responsibility to ensure it remains accountable, explainable, and aligned with human values. “With technologies evolving towards Industry 5.0, the focus will shift to Responsible AI, ensuring that AI applications are built on safe, trustworthy, and ethical principles,” says Chappell.
He adds that the industry can expect to see frameworks for Responsible AI to ensure AI decisions are equitable, understandable and accountable. “These frameworks will use practices like capturing citations to document inputs and outputs while protecting data and maintaining user privacy,” he explains Responsible AI not only builds trust but is increasingly vital for regulatory compliance, especially in
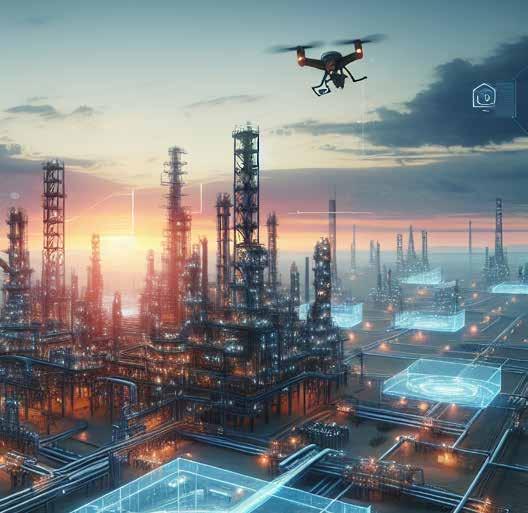
sectors like energy, manufacturing, and infrastructure.
Agentic AI is also transforming sustainability efforts. While AI has long supported energy efficiency, recent innovations allow organisations to scale these initiatives more effectively.
“AI is much more than just one technology – it includes expert systems, machine-learning (ML) programmes, prescriptive and prognostic models, reinforcement learning, right up to Large Language Models (LLMs) and GenAI,” says Chappell. “AI-infused solutions can turbocharge industries’ progress towards efficiency and sustainability.”
These solutions, when combined with cloud and data analytics, can optimise energy use and reduce emissions. “These types of AI solutions do not involve training
massive LLMs… AI actually runs substantially faster, thus requiring significantly less energy,” he says.
Chappell highlights the partnership between AVEVA and TotalEnergies as a practical example. “AVEVA is working with TotalEnergies, to monitor over 110 greenhouse gas reduction projects using the AVEVA PI System. Data from global sites is fed into dashboards at TotalEnergies’ headquarters, where teams analyse performance against key KPIs. This system enables them to monitor 85 percent of emissions accurately and leverage AI to forecast the impact of operational changes.”
In one case, optimising power delivery at a single site led to a 15 percent reduction in carbon emissions annually. Scaled across TotalEnergies’ operations, the potential impact is enormous—tens of thousands of tonnes in CO₂ savings every year.
“AI IS EVOLVING INTO DYNAMIC PARTNERS, ACTING AS ‘INTELLIGENT AGENTS’ CAPABLE OF AUTONOMOUS ACTION, CONTINUOUSLY LEARNING FROM COMPLEX DATA”
Building Trust in Intelligent Agents
For all its promise, Agentic AI brings its own set of challenges—chief among them being reliability and user trust. As AI systems become more complex, ensuring they behave consistently and transparently is a top priority.
“Deploying Agentic AI comes with challenges. One major concern is reliability—unlike traditional software, AI models can produce varying results, making it difficult to build consistent test frameworks,” Chappell explains. “Additionally, user acceptance remains a hurdle, as many AI systems still require human validation. As AI becomes more autonomous, fostering trust and ensuring transparency will be critical to adoption.”
To address this, AVEVA places strong emphasis on human-in-theloop design, where human judgment remains central. Trust is also built through clear communication, robust documentation, and co-development with customers.
Looking ahead
As we step into the era of Industry 5.0, the integration of Agentic AI marks a shift from automation to true intelligence—where systems evolve alongside human decision-makers. Organisations that are ready to lead will be those that combine visionary thinking with grounded, responsible AI practices—shaping not just what comes next, but how it’s built.

Yasser Hassan / AWS
The intelligent shift
As generative AI transitions from hype to meaningful adoption, Yasser Hassan, Managing Director – MENAT at Amazon Web Services (AWS), explores how it’s driving real business outcomes and transforming enterprise strategy
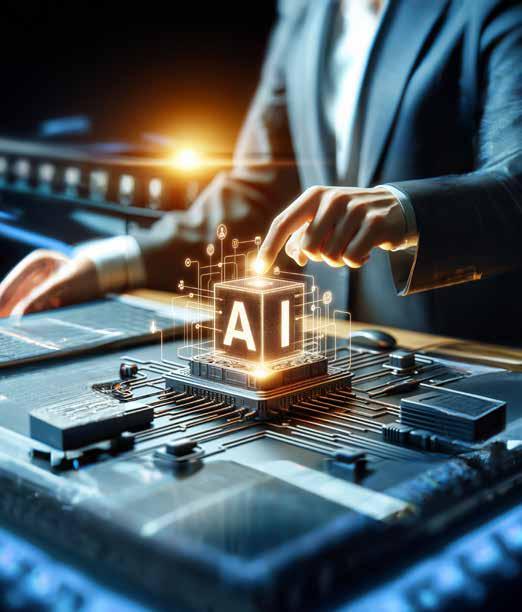
Artificial intelligence (AI) has quickly evolved from an experimental concept to a businesscritical capability. Since the release of ChatGPT in 2022, organisations worldwide have accelerated their AI journeys, shifting their focus from innovation pilots to largescale deployment. At the centre of this transformation is generative AI (GenAI), now emerging as a foundational pillar of modern business strategy.
Generative AI (GenAI) is accelerating this shift through a wide range of applications—spanning content creation, code generation, intelligent summarisation, and conversational agents. These use cases are helping organisations embed intelligence across functions, resulting in a tangible shift in how enterprises drive efficiency, personalisation, and speed-to-market.
“GenAI is fast becoming a core driver of strategic transformation, with business leaders recognising its potential to reshape operations, reimagine customer engagement, and unlock new growth opportunities,” says Yasser Hassan, Managing Director – MENAT, AWS.
Now that the AI race is in full swing, companies must differentiate between superficial experimentation and meaningful innovation.
“Organisations should look for solutions that address all the pieces needed to scale AI applications, not just one core model,” says Hassan. “This includes workflows, guardrails, and cost optimisation strategies.”
AWS’s perspective on meaningful innovation comes down to two key ideas: flexibility and scalability. By embracing a model-agnostic approach, enterprises can avoid being locked into a single solution. “Genuine innovation
involves having the flexibility to choose the right model for the specific task and being able to adapt as new models emerge,” he explains.
The company’s flagship GenAI service, Amazon Bedrock, allows customers to build and scale applications using foundation models from AWS and third-party leaders such as Anthropic, Meta, Mistral AI, and Cohere. This broad choice is essential for leaders aiming to tailor AI to their specific business needs without compromising agility.
Responsible AI by design
As GenAI integrates deeper into business and society, ethical considerations and responsible AI governance have become central to technology adoption.
“Technology providers have a responsibility to set ethical standards for AI,” says Hassan. “Since they’re the pivotal power plays of its widespread deployment, it’s their duty, along with government regulators and academia, to put in place the checks and balances that help ensure responsible and ethical AI proliferation.”
Accelerating AI momentum in the Middle East
The Middle East is quickly becoming one of the world’s most exciting AI frontiers. Visionary governments and ambitious enterprises are setting a bold pace for digital transformation. AWS is helping drive the momentum to position the region as a global hub for AI.
“TECHNOLOGY PROVIDERS HAVE A RESPONSIBILITY TO SET ETHICAL STANDARDS FOR AI”
Infrastructure purpose-built for AI As models become more sophisticated and compute-intensive, the ability to scale depends heavily on infrastructure. Traditional systems are often ill-equipped to handle the demands of GenAI, which requires high performance and cost-efficient operations.
“It’s extremely critical, even as costs start to decrease with scale, and here in the Gulf region, there’s an abundance of energy— and motivation—to power AI infrastructure,” says Hassan.
To address this, AWS has developed its own custom chips—Trainium and Inferentia—designed specifically for GenAI workloads. These chips deliver significantly better price performance compared to general-purpose instances, enabling customers to reduce the cost of inference while supporting faster training times.
Combined with AWS’s scalable cloud architecture, this gives enterprises the tools they need to handle large volumes of data and complex model deployment across regions.
AWS has integrated a range of responsible AI features into its platform. These include configurable guardrails, toxicity filters, and automated systems for detecting sensitive data like personally identifiable information (PII).
One particularly innovative safeguard is AWS’s method for detecting hallucinations—a wellknown challenge with large language models.
“We’ve implemented contextual grounding checks to verify that the output of a model is relevant to the input and grounded in the context. We’re able to filter 75 percent of hallucinations this way by applying guardrails,” says Hassan. “We also emphasise configurability, allowing customers to customise the ethical controls and policies that are applied to their AI applications.”
The company’s partnership with Anthropic further underscores its responsible AI approach. “Through this collaboration, we’re helping customers scale GenAI with privacy, safety, and security built in from day one,” says Hassan.
In addition to providing access to Anthropic’s foundation models via Amazon Bedrock, AWS also supports Anthropic’s model training through its purpose-built Trainium chips—demonstrating a shared focus on innovation grounded in ethical principles.
“Here in the Gulf, we’re very fortunate to be aligned with visionary digital-first governments that are leading the way in cross-border investment collaborations that are transforming the likes of the UAE and Saudi Arabia into AI supernations,” says Hassan.
AWS has already launched dedicated cloud regions in Bahrain and the UAE, with a third slated to open in Saudi Arabia by 2026. Collectively, AWS is investing over US$10 billion in the UAE and KSA alone. These investments are expected to contribute significantly to national GDPs and job creation. For instance, the AWS Middle East UAE Region is projected to support nearly 6,000 full-time jobs and generate AED 41 billion (US$11.16 billion) in economic impact by 2037. Strategic partnerships with regional powerhouses such as e&, stc Group, Careem, Lulu Group, and Property Finder underscore AWS’s deep involvement in accelerating digital transformation across sectors.
The road ahead
For AWS, the future of AI goes beyond technological leadership to building ecosystems that foster sustainable, secure, and inclusive innovation.
“Ultimately, GenAI is no longer a side project or innovation pilot,” concludes Hassan. “It’s a foundational pillar of modern business strategy. Companies that successfully integrate it into their core are the ones shaping the next chapter of digital transformation.”

Ranjith Kaippada / Cloud Box Technologies
Scaling momentum
Ranjith Kaippada, Managing Director at Cloud Box Technologies, discusses how enterprises are accelerating the wave of AI maturity in the Middle East, turning strategic ambitions into scalable impact
The global AI race is accelerating, but few regions are leaning in as decisively—or as strategically—as the Middle East. With bold national agendas like Saudi Arabia’s Vision 2030 and the UAE’s National AI Strategy 2031, AI has moved beyond aspiration to a strategic imperative woven into the region’s future.
“AI investments in the Middle East, particularly in the UAE and Saudi Arabia, are accelerating due to government initiatives and the growing demand for automation. The Saudi Vision 2030 and the UAE’s National AI Strategy 2031 are driving AI adoption across industries such as cybersecurity, healthcare, and finance, unlocking vast opportunities for businesses,” says Ranjith Kaippada, Managing Director at Cloud Box Technologies.
Among the trends gaining momentum are generative AI for content creation, intelligent automation, and personalised customer experiences powered by data analytics. Predictive insights and advanced cybersecurity capabilities are also emerging as key differentiators for organisations looking to future-proof operations.
“Key trends gaining traction include generative AI for content creation, personalised customer experiences powered by automation, and predictive
“AI IS THE FOUNDATION OF THE NEXT ERA OF DIGITAL TRANSFORMATION, EMPOWERING BUSINESSES TO OPERATE FASTER, SMARTER, AND MORE SECURELY”
“In the digital era, data is considered the new gold,” explains Kaippada.
“With enterprises holding vast amounts of sensitive data, cybercriminals can exploit vulnerabilities, potentially causing irreparable damage.”
He further underscores that Responsible AI frameworks are quickly becoming essential. Enterprises are expected to audit their AI models, ensure explainability in decisionmaking processes, and enforce strict data governance policies to mitigate risk and regulatory exposure.
“To achieve compliance while fostering innovation, enterprises should establish AI governance frameworks, conduct regular audits of AI models, and implement robust data governance policies. These measures help organisations leverage AI’s full potential while aligning with evolving regulatory requirements,” he says.
analytics. AI-driven cybersecurity solutions are also becoming essential, as cyber-attackers increasingly leverage AI to target multiple devices simultaneously,” says Kaippada.
Responsible AI: Navigating the regulatory tightrope
As AI becomes a more integral part of daily operations—from automating workflows to making data-driven decisions—it brings with it a heightened need for responsibility. Transparency, fairness, and compliance are becoming just as important as performance and efficiency.
With regulations like the UAE Data Protection Law and Europe’s GDPR influencing how companies collect, process, and secure data, AI implementation must go beyond functionality—it needs to be explainable, auditable, and ethical.
In sectors such as finance, healthcare, and public services, where decisions can impact livelihoods, the demand for ethical, interpretable AI is even more urgent.
Closing the capability gap
Amid the AI revolution, technology is only half the story. The other half –people.
As demand for AI capabilities outpaces supply, regional organisations are increasingly investing in workforce development and ecosystem-wide enablement. This includes partnerships, certifications, and hands-on programmes that upskill both internal teams and external collaborators.
“As a leading system integrator, we understand the importance of continuous upskilling in the evolving AI landscape. To support our partners and customers, we offer AI-focused training programs, certifications,
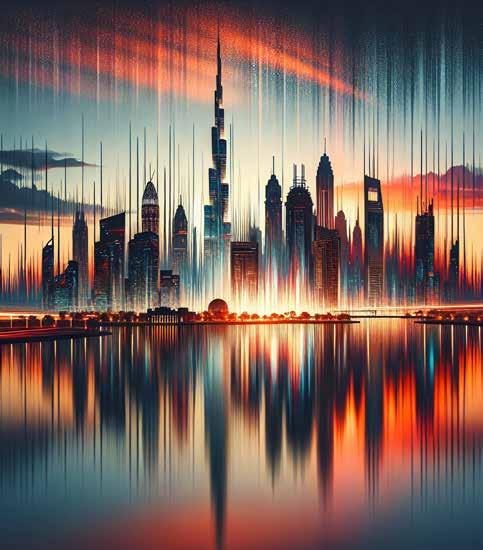
workshops, and industry-specific seminars. These initiatives provide essential knowledge and expertise to keep businesses aligned with emerging AI trends.”
More broadly, collaboration between global technology providers and regional players is helping make AI more accessible and industryrelevant. This includes delivering scalable cloud AI services, sectorspecific frameworks, and customised deployment strategies.
“To further accelerate AI adoption, we conduct hands-on workshops, AI certification programs, training sessions, and seminars for our employees and partners. These initiatives equip stakeholders with the latest AI skills and knowledge.”
AI-powered security: Reinventing resilience
Cybersecurity has always been critical—but with threats becoming faster, smarter, and more complex, traditional security tools are simply no longer sufficient. This is why enterprises are increasingly shifting from reactive security models to proactive detection and real-time response powered by machine learning and behavioural analytics.
“Traditional security solutions struggle to keep pace with today’s evolving threat landscape, where organisations face relentless cyber-attacks, including malware, ransomware, and unauthorised system access. AI has revolutionised cybersecurity by enabling faster,
more accurate threat detection while minimising false positives,” says Kaippada.
Next-generation Security Operations Centres (SOCs) are at the forefront of this transformation. AI-powered SOCs ingest massive volumes of data, identify anomalies, predict attack patterns, and respond to incidents before they escalate.
“Cloud Box Technologies’ AIpowered Security Operations Centre (SOC) analyses vast amounts of security data daily. Using machine learning, behavioural analytics, and advanced AI algorithms, it identifies attack patterns and fortifies defences against brute-force attempts,” says Kaippada.
These SOCs play a critical role not only in enhancing detection and response times but also in ensuring regulatory compliance, conducting proactive threat hunting, and delivering continuous risk assessments.
He adds, “Our AI-driven SOC also ensures compliance, proactive threat hunting, and comprehensive risk assessments. With real-time monitoring and proactive incident response, we provide businesses with resilient, AI-enhanced cybersecurity solutions that mitigate financial and reputational risks.”
AI as the core of enterprise transformation
As the Middle East advances toward becoming a global AI hub, the region’s enterprises face a pivotal opportunity: to embed intelligence at the heart of how they operate, serve, and grow. “AI is the foundation of the next era of digital transformation, empowering businesses to operate faster, smarter, and more securely. We continue to invest in AI-driven applications such as intelligent automation, cybersecurity, and predictive analytics to enhance enterprise resilience,” says Kaippada.

Sid Bhatia / Dataiku
Redefining value
Sid
Bhatia, Area VP & General Manager, Middle East, Turkey & Africa at Dataiku, explores
how enterprises can bridge the gap between GenAI innovation and real-world impact
through strategic governance and scalable frameworks
There is no denying that generative AI has captured the collective imagination of enterprises worldwide. In just over a year, it has gone from a curiosity to a strategic imperative. Across industries, organisations are looking for ways to use it for everything from automating content creation to improving customer experiences and streamlining operations. The potential is exciting but turning that potential into something tangible is proving to be more complicated than many expected.
Sid Bhatia, Area Vice President & General Manager for the Middle East, Turkey & Africa, points to a growing appetite for enterprise AI— but also a reality check on what it takes to get there.
“The biggest opportunity lies in leveraging generative AI to boost productivity, enhance customer experiences, and automate knowledge work—especially through use cases like
summarization, content generation, code assistance, and intelligent document processing,” he says.
The enthusiasm, though, is often accompanied by a misunderstanding.
“A common misconception is that deploying a powerful LLM alone guarantees value. Enterprises often underestimate the need for governance, data integration, fine-tuning, and responsible AI practices to make these models enterprise-ready and impactful at scale,” explains Bhatia.
In reality, the model is just one part of a broader ecosystem. Its success hinges on how well organisations can operationalise it within dynamic and complex environments. As generative AI evolves, so do expectations—especially around security, transparency, performance, and scalability.
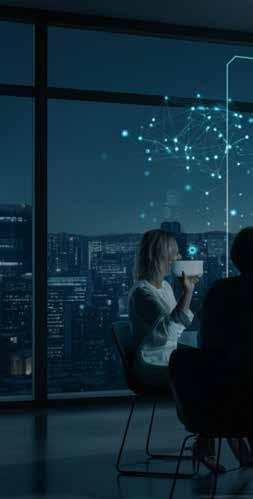
AI responsibly across functions— without losing visibility or control.
To meet this need, Dataiku introduced LLM Mesh—a unified framework to orchestrate, monitor, and govern multiple LLMs.
“DATAIKU’S MISSION IS TO DEMOCRATISE AI AND MAKE IT PART OF THE EVERYDAY FABRIC OF DECISION-MAKING”
“The pace of innovation in generative AI has raised expectations significantly. Enterprises now expect solution providers to offer out-ofthe-box support for LLMs, seamless integration with multiple models (open-source and proprietary), and tools to operationalise GenAI use cases quickly and safely,” Bhatia adds.
This marks a shift away from isolated pilots toward enterprise-wide AI orchestration. Businesses now seek platforms that empower them to scale
“At Dataiku, this is exactly why we introduced LLM Mesh—a framework that allows enterprises to orchestrate, monitor, and govern multiple LLMs through a unified framework, ensuring flexibility without compromising on compliance or control.”
Its value is twofold: enabling agility by supporting model experimentation without infrastructure overhaul, and establishing a governance backbone to ensure compliance, data protection, and sustained stakeholder trust.
Industry momentum
While the AI maturity curve varies by sector, certain industries are
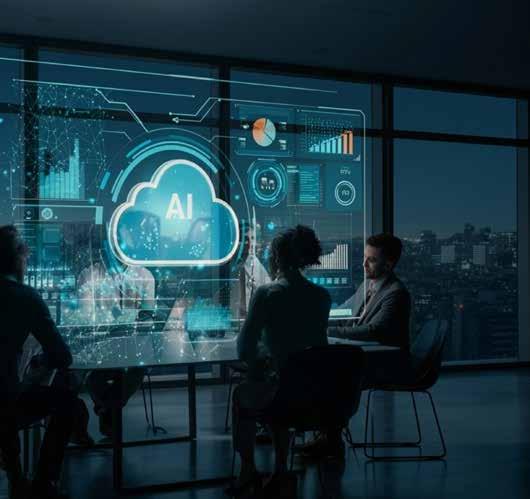
already proving what’s possible when innovation meets execution.
According to Bhatia, financial services, telecommunications, and manufacturing are leading the way.
“These sectors have matured data infrastructures and clear ROI-driven use cases—from fraud detection and risk scoring to predictive maintenance and network optimisation,” he explains.
He also sees growing momentum in the public sector and healthcare, where the emphasis on explainability, fairness, and responsible deployment is driving innovation within structured guardrails.
What unites these frontrunners is clarity of purpose. AI success in these sectors stems from strong alignment between business outcomes and data strategies, supported by the right platforms and people.
Redefining ROI in the age of AI
For AI to mature from tactical tool to strategic asset, organisations must rethink how they measure success. Traditional ROI metrics like cost savings are no longer sufficient. Instead, forward-looking enterprises are tracking impact across a broader set of dimensions: timeto-insight, marketing conversion uplift, reduced risk exposure, and operational efficiency.
“Measuring ROI in AI requires a multi-dimensional approach. It’s not just about cost savings, but also revenue generation, risk reduction, and productivity gains,” explains Bhatia.
To support this, Dataiku encourages customers to define key performance indicators early and monitor them throughout the MLOps lifecycle.
“Our platform helps monitor impact in real-time and align AI outcomes to business goals,” he says.
This approach ensures that AI initiatives remain focused, measurable, and outcome-driven— critical ingredients for long-term enterprise adoption.
What’s next?
Looking ahead, Bhatia is motivated by the shift from AI as an isolated capability to AI as an embedded function within core business processes. This evolution is being accelerated by trends like composable AI, retrieval-augmented generation (RAG), and AI agents that can act autonomously across systems.
“Beyond generative AI, we’re excited about composable AI, retrievalaugmented generation (RAG), and AI agents that autonomously complete tasks across systems. These innovations will accelerate the move from experimentation to truly embedded AI in business operations,” says Bhatia.
But with great power comes great responsibility. As these technologies mature, responsible AI frameworks will be the cornerstone of trust, especially in regulated industries and public-facing sectors.
As generative AI becomes a foundational layer in the enterprise tech stack, its success will depend on the ability to empower crossfunctional teams—from data scientists to business analysts—with tools that are intuitive, governed, and aligned with strategic priorities.
“Dataiku’s mission is to democratise AI and make it part of the everyday fabric of decisionmaking,” Bhatia affirms.
“Our focus remains on empowering diverse personas—from data scientists to business analysts—to co-create and operationalise AI in a governed, scalable, and responsible way. We don’t just help companies adopt AI; we help them industrialise it.”
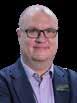
Kalle Björn / Fortinet
At the frontlines
Kalle Björn, Senior Director, Systems Engineering - ME at Fortinet, highlights how AI
is reimagining cybersecurity for a new era of threats
As cyber threats grow in scale and sophistication, enterprises are being forced to confront a new reality: artificial intelligence (AI) is both a potent weapon and a critical line of defence. From deepfake phishing and adversarial AI to autonomous malware campaigns, attackers are leveraging AI in increasingly complex ways. In this dual-threat environment, organisations can no longer rely on traditional tools and manual processes. The future of cybersecurity lies in intelligent, adaptive systems that combine AI’s analytical power with human oversight and strategic intent.
“Cybersecurity is at an inflection point, with AI being used both offensively and defensively,” says Kalle Björn, Senior Director, Systems Engineering - ME at Fortinet. “AIdriven security solutions can be a force multiplier when it comes to keeping pace with the speed and sophistication of modern threats. They can also play a vital role in keeping an organisation’s dynamic, hybrid networks safe and online.”
This evolution is shaping security strategies across sectors. For enterprises navigating the complexities of hybrid networks, distributed workforces, and expanding digital footprints, the stakes have never been higher.
AI as an amplifier
As AI becomes more embedded in attack methodologies, the ability to detect and respond in real time is fundamental.
The conversation has moved beyond whether to adopt AI in cybersecurity to how best to integrate it. Solutions powered by machine learning and GenAI are increasingly seen as force multipliers that can help security teams stay ahead of emerging threats. This is particularly critical in today’s dynamic environments where the attack surface constantly shifts across endpoints, cloud platforms, and edge devices.
To stay ahead of this complexity, Björn highlights two essential capabilities: “The first is AI-driven threat detection to proactively identify and stop attacks before they can compromise your organisation.”
He adds: “The second is GenAI.
As the complexity of today's hybrid network security increases the complexity of operations, GenAI can help simplify certain tasks for NOC/SOC engineers by allowing natural language queries for quick, accurate insights. GenAI can also help automate tasks like updating configurations to reduce the risk of misconfigurations and strengthen overall security.”
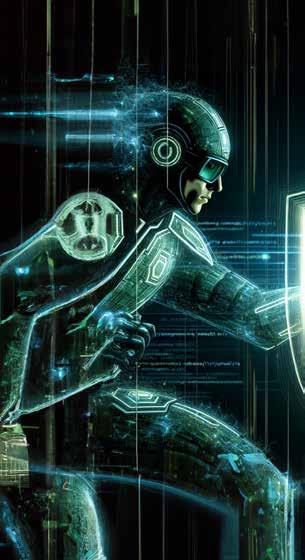
Mind the gap
Despite the promise of AI, a foundational gap remains: the global cybersecurity skills shortage. AI cannot succeed in a vacuum. It needs informed users, skilled teams, and decision-makers who understand the implications of deploying intelligent systems across business-critical infrastructure.
“Perhaps the largest issue that all organisations face in these areas is a lack of AI-specific training and awareness,” says Björn. “Research estimates a global shortage of around 4.8 million cybersecurity professionals, which Fortinet is actively addressing by offering free cybersecurity training for IT teams.”
This skills gap has knock-on effects. Without skilled employees, organisations can be slow to adopt AI-based security solutions that are
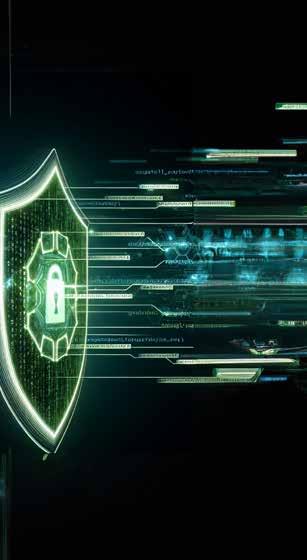
essential for effectively detecting and responding to AI-driven threats.
While AI excels at scale and automation, it cannot replace the human element entirely. Strategic decision-making, policy design, and ethical oversight still require human judgement.
“Automation is particularly important in cybersecurity given the ongoing shortage of expert security staff. However, human oversight will still be important,” says Björn.
He further notes that security teams will need to be equipped with the knowledge and skills to understand, interpret, and manage AI-driven security systems effectively. “AI excels at tactical responses based on
predefined rules. However, defining security policies, understanding risk tolerance, and making strategic decisions still require human expertise and intuition,” he says.
To ensure trust in AI systems, explainability and auditability must be built in. Björn advises organisations to “focus [on] opting for transparent AI models whose decision-making processes can be understood and audited by human experts. Organisations should also implement robust validation and testing, rigorously testing AI models with diverse datasets to identify and mitigate biases or inaccuracies and following any local and global regulations around AI.”
Integrating intelligence
To be effective, AI can’t be bolted on—it must be embedded into the architecture of security itself. Fortinet’s approach reflects this shift.
“Incorporating AI into our Security Fabric, Fortinet centres on an integrated approach, leveraging AI-driven technologies for proactive threat detection and response,” Björn explains. “FortiGuard AI-Powered Security Services integrate with security solutions across Fortinet's broad portfolio to provide marketleading security capabilities that protect applications, content, web traffic, devices, and users located anywhere.”
Unlike competitors that rely on third-party feeds, Fortinet’s intelligence is built in-house. “While many of our competitors OEM their security intelligence from different vendors, FortiGuard Threat Intelligence has been built in-house, allowing us to apply AI consistently across different sources to expand the scope and scale of how and where it can be used.”
These innovations extend to Fortinet’s cloud-native and edge
offerings. AI powers web filtering, intrusion prevention, and DLP within FortiSASE to identify and block threats in real-time. When it comes to ZTNA, FortiClient uses AI-driven endpoint telemetry to assess device posture and enforce least-privilege access, strengthening ZTNA security.
What’s ahead
Looking forward, the role of AI in cybersecurity will continue to deepen— especially as the industry moves toward self-healing ecosystems capable of identifying, isolating, and remediating threats without human intervention.
“As we continue to imagine AI in every aspect of cybersecurity, we're witnessing a revolution that's reshaping the industry, making it more proactive, responsive, and adaptive than ever before,” says Björn.
Generative AI is expected to play a key role. Beyond summarising alerts or assisting analysts, it is now being used to automate decision-making in environments where speed is critical and context is complex. Real-world applications, like customer service automation at scale, offer a glimpse of what’s possible in cybersecurity.
But innovation can’t happen in isolation. The future of cyber defence will depend on cross-industry collaboration, shared intelligence, and coordinated responses. No single vendor or organisation can secure the digital world alone. Partnerships— across sectors and borders—will be key to dismantling sophisticated cybercrime operations.
“Innovation is in our DNA and we are constantly looking for new ways to better protect people, data, and devices everywhere,” says Björn. “As cyber risks continue to grow, we’ll continue to empower our customers with solutions that streamline security processes, improve decisionmaking, and bolster resilience against evolving threats.”
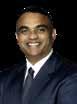
Jacob Chacko / HPE Aruba Networking
Shaping intelligent networks
Jacob Chacko, Regional Director – Middle East & Africa, HPE Aruba Networking, highlights how AI is redefining future-ready networks
Networks have evolved beyond being the connective tissue of enterprise IT to a foundation on which business agility, innovation, and security rest. As organisations shift toward cloud-native architectures, edge computing, and the Internet of Things (IoT), network complexity is growing exponentially. Additionally, artificial intelligence (AI) is stepping in as the defining enabler, turning traditional networks into intelligent, adaptive ecosystems.
According to a Jacob Chacko, Regional Director – Middle East & Africa, HPE Aruba Networking, this convergence of AI and networking is already transforming how enterprises manage performance, user experience, and security. “Enterprise networks are growing more diverse and distributed. This makes managing and protecting them with traditional, manual techniques is becoming almost impossible. That’s where AI flips into support mode.”
The AI imperative for modern networking Organisations are now leveraging AI to take control of their networks with granular, real-time insights that power operational efficiency and resilience.
“The opportunity for IoT to provide organisations with rich data to train and activate Generative AI models is huge,” says Chacko. “But it also
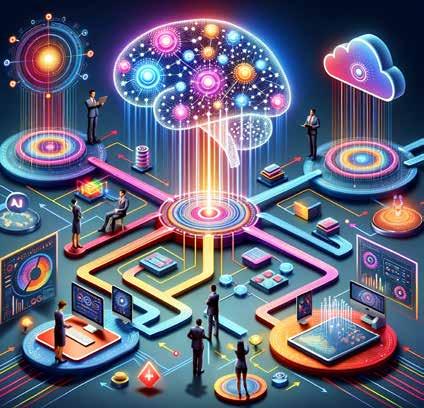
increases the critical need to detect changes in network traffic patterns, connection status, or dynamic device attributes that could signal a successful compromise.”
This is where Artificial Intelligence for IT Operations (AIOps) plays a vital role. It enables IT teams to proactively identify, diagnose, and even resolve network issues — often before end users are
aware of them. For example, HPE Aruba Networking’s AIOps can automatically pinpoint the root cause of Wi-Fi, wired, or WAN issues, and execute self-healing actions to resolve them. This closed-loop automation model is what sets it apart.
“Built-in self-healing workflows go beyond identifying the problem — they fix it automatically. And detailed reports show when problems were
encountered and what was done to resolve them. This is real operational transformation,” he says.
Unlocking speed, scale, and simplicity
AI offers an opportunity to shift from reactive, labour-intensive network operations to proactive, insightdriven models that deliver real-time optimisation at scale. Instead of waiting for issues to escalate, teams can now anticipate and fix problems before they impact business outcomes. In large, decentralised organisations, where network environments span hundreds of locations and thousands of endpoints, this AI-driven shift is already yielding tangible benefits.
“We’ve seen AI-trained models reduce the time and effort it takes to plan, deploy, manage, and optimise networks,” says Chacko. “In some cases, what used to take weeks across hundreds of sites can now be done in a matter of hours.”
This is more than automating networks, it’s about applying AI models trained on telemetry data to make smarter, faster decisions.
Take the case of HPE Aruba Networking Central, where AI-powered insights derived from vast real-time telemetry — drawn from millions of managed devices and endpoints — support more intelligent network planning and troubleshooting. Such platforms illustrate how AI can reduce the cognitive load on IT teams by automatically flagging anomalies, recommending configuration adjustments, and initiating remediation workflows, all without human intervention.
Going one step further, the platform has introduced expanded Digital Experience Monitoring (DEM) by integrating HPE Aruba Networking’s User Experience Insight (UXI) sensors, thereby extending intelligence to the user experience. “By integrating UXI sensors with digital experience
“LARGE LANGUAGE MODELS (LLMS) ARE IMPROVING HOW TEAMS SEARCH, DIAGNOSE, AND UNDERSTAND WHAT’S HAPPENING ACROSS DISTRIBUTED ENVIRONMENTS”
monitoring, we’re continuously tracking SLA performance,” he adds. “This helps IT address issues before users are even aware of them.”
Additional tools like OpsRamp add a new layer of contextual observability. “These kind of solutions helps eliminate blind spots across heterogeneous networks by bringing in insights from network devices. They also accelerate common health monitoring and troubleshooting tasks, making operations much more efficient,” he says.
These developments mark a transition from network monitoring to true observability.
The challenge of autonomy
The prospect of autonomous networking — where AI systems govern end-to-end operations — is compelling. But the journey toward that vision is complex and fraught with practical and regulatory considerations.
“One of the biggest concerns is the risk of automating actions on critical national infrastructure,” he says. “There are regulatory, compliance, and practical implications that need to be carefully considered.”
Cybersecurity is another critical concern. Autonomous networks, if not governed correctly, could become lucrative targets for threat actors. In addition, as AI becomes part of the solution, it can also become part of the problem. “Adding new AI applications means adding potential
new vulnerabilities. AI can be abused as a tool for attacks,” says Chacko.
To address this duality, the HPE Aruba Networking follows a “securityfirst, AI-powered” philosophy grounded in Zero Trust.
This vision is central to HPE Aruba Networking’s approach. “It offers organisations security from edge to cloud and improves the overall security posture by applying a rigorous set of best practices and controls to previously trusted network and cloud resources.”
“Security and networking should not be separate domains. The best customer experience will be when network and security are delivered together in one common platform,” he says. “And that’s the model we’re building.”
The road ahead
Looking forward, the future of AI and networking is not a question of if but how fast. AI will be critical to maintaining performance, security, and resilience in the face of escalating complexity.
“The role of IT is changing. It is crucial to business operations as it plays a significant part in production, strategy, and time to market of services and commodities,” he says. “AI helps IT teams to cope with the volume of events and data, and frees up resources for more strategic tasks.”
Yet the relationship is symbiotic. While AI makes networking more intelligent, AI itself depends on robust, scalable infrastructure. According to the Dell’Oro Group, network traffic for AI workloads is expected to increase tenfold every two years. As a result, infrastructure must evolve to support extremely high bandwidth and low latency — from 100 Gb to 800 Gb and beyond.
The conclusion is clear: AI and networking are now inextricably linked. “Success in AI means embracing these interlinked technologies simultaneously,” he says.
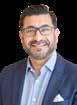
Vijay Jaswal / IFS
The autonomous frontier
As industrial leaders rethink strategies in the age of AI, Vijay Jaswal, Chief Technology Officer, APJ, ME&A at IFS, discusses how industrial organisations are redefining resilience and preparing for a more autonomous future
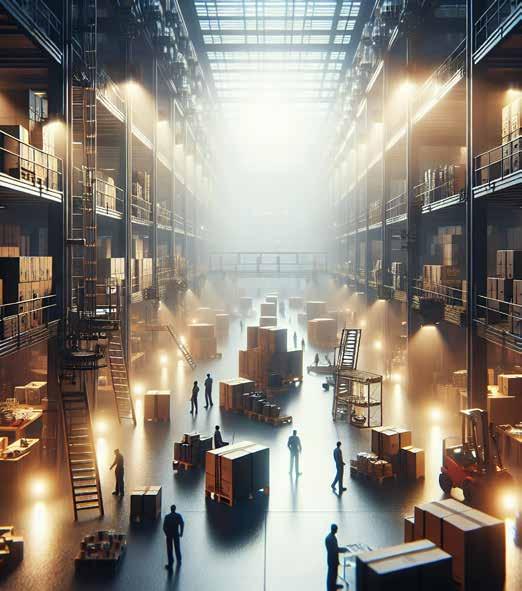
The convergence of artificial intelligence (AI), automation, and connected systems is pushing industries to move beyond traditional efficiency metrics and into a realm of self-optimising, adaptive operations. Yet, even as the promise of AI grows, the reality on the ground is often more complex.
“Industrial sectors face challenges such as unplanned asset downtime, workforce shortages, supply chain disruptions, and increasing pressure for sustainability and cybersecurity,” says Vijay Jaswal, Chief Technology Officer, APJ, ME&A at IFS. These issues are not new—but they’re intensifying. And with each challenge comes the opportunity to rethink operations through the lens of intelligent systems.
For many industrial organisations, the road to AI adoption is slowed by long-standing structural issues— siloed data, legacy infrastructure, and limited internal expertise. As Jaswal points out, “Companies face key obstacles such as data silos, legacy system integration, lack of AI expertise, and change resistance.”
Despite widespread interest in AI, many enterprises still struggle to operationalise it across functions. The problem isn’t just about adopting the latest algorithms—it’s about embedding intelligence into
workflows and decision-making processes in a way that’s seamless and impactful. According to Jaswal, platforms that “provide a unified, composable foundation” are critical for helping businesses overcome these barriers and unlock AI’s true potential.
This shift from isolated tools to integrated intelligence represents a defining pivot in how AI is viewed—not as a bolt-on, but as a foundational capability for industrial competitiveness.
From data to decision
Modern industrial environments are complex ecosystems of machines, data streams, human operators, and supply networks. Making sense of this complexity in real time—and acting on it—is where AI delivers tangible value.
“AI-driven intelligence can automate data analysis, provide real-time insights, improve asset reliability, optimise supply chains, and enhance workforce productivity,” says Jaswal. These capabilities are no longer aspirational—they are fast becoming essential in a world where every minute of downtime translates to lost revenue and eroded customer trust.
Predictive maintenance, intelligent automation, and AI-driven supply chain resilience are among the most impactful use cases emerging today.
These aren’t just buzzwords—they’re measurable shifts in how industrial leaders respond to disruption, manage risk, and drive performance.
Empowering the workforce of tomorrow
One of the more profound impacts of AI is how it is reshaping workforce dynamics. As industries become more digital, the skills needed on the ground are evolving—faster than many organisations can reskill. In this context, AI is not about replacing
workers—it’s about enabling them.
“AI-driven automation, Large Language Models (LLMs), and smart technology—like tablets or wearables—can deliver realtime, context-aware guidance to the workforce,” Jaswal explains.
“When combined with smart glasses, employees can receive hands-free, AI-powered assistance, overlaying step-by-step instructions, diagnostics, and predictive insights onto their field of view.”
This blend of human expertise and machine intelligence is particularly powerful in environments where safety, speed, and precision are critical. It reduces training overhead, bridges the generational knowledge gap, and supports workers as they navigate more complex digital workflows.
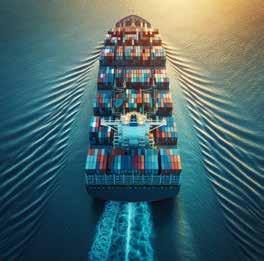
industries toward self-healing systems and autonomous decisionmaking.”
“AI IS THE FOUNDATION FOR THE JOURNEY TOWARD FULLY AUTONOMOUS INDUSTRIAL OPERATIONS”
Quantum computing, for instance, could radically enhance supply chain modelling, energy efficiency simulations, and predictive maintenance—solving problems beyond the reach of today’s classical systems. Meanwhile, AI-enabled circular economy models will help industries minimise resource waste and maximise reuse.
Vision to value
The road to autonomy
The endgame, for many, is a future where industrial systems are not only smart, but autonomous. Jaswal sees this shift accelerating over the next decade, as AI technologies mature and converge with innovations like digital twins and quantum computing.
“AI is the foundation for the journey toward fully autonomous industrial operations,” he says. “Over the next decade, breakthroughs in AI-powered automation, predictive intelligence, digital twins, and quantum computing will drive
The industrial AI conversation is evolving—from experimentation to execution, from siloed pilots to enterprise-wide orchestration. As Jaswal frames it, success lies not in AI itself, but in how intelligently it’s embedded across the business.
Furthermore, the challenge now is not whether to adopt AI—but how to do so in a way that creates sustainable, measurable impact.
“As industries embrace AI-driven autonomy and quantum-powered insights, our goal is to help businesses transition from manual oversight to AI-powered orchestration, unlocking new levels of resilience, innovation, and value creation,” says Jaswal.
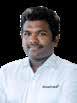
In clear view
Ramprakash
Ramamoorthy, Director of AI Research at ManageEngine, unpacks how enterprises can unlock real value from AI by prioritising transparency, contextual intelligence, and human-machine collaboration
Today, the conversation around AI has shifted from “if” to “how fast.” As digital ecosystems expand and threat surfaces grow more complex, organisations are recognising AI not as an experimental edge, but as an operational necessity. Still, this transition is not without friction—especially in fragmented environments where data silos, talent shortages, and compliance requirements complicate AI deployment.
“AI is a driving force of modern IT management,” says Ramprakash Ramamoorthy, Director of AI Research at ManageEngine. “Once purported to be overstatements, the claims of AI proactively preventing, detecting, and handling security flaws, cybersecurity attacks, and threats have become a reality.”
However, operationalising AI at scale remains a struggle. Overburdened IT teams face alert fatigue, and AI models often underperform in siloed environments, leading to false positives and reduced trust.
“Enterprises contend with tons of complexities and exorbitant expectations,” he explains. “The
challenges start with fragmented ecosystems where AI models struggle to understand and learn from siloed data sources.”
Opening the ‘black box’
As AI becomes more embedded in decision-making, it’s not just about what the system does—but whether we can understand why it does it.
One of the most significant adoption barriers is the black box problem: opaque, unexplainable processes that undermine confidence and accountability.
“For AI to truly add value in enterprise IT, its decision-making must be transparent, traceable, and auditable,” says Ramamoorthy.
“AI-driven systems may generate numerous anomaly alerts, but IT teams must understand why an event was flagged rather than blindly acting on notifications.”
This lack of interpretability introduces operational risk—from missteps in security response to non-compliance in regulated industries. Ramamoorthy advocates for explainable AI not just to build trust, but to strengthen performance.
“Explainable AI empowers security
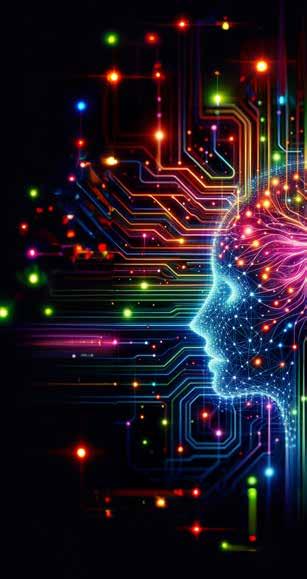
teams to trace decision-making processes, reduce false positives, and improve incident response,” he notes. Building such clarity requires both technical and governance guardrails.
“Organisations should focus on adopting governance policies that define bias detection protocols, ethical AI guidelines, and human-inthe-loop oversight,” he says. “Models that deliver transparent, actionable insights and openly disclose their data and prediction techniques are more effective than guarded models.”
Rethinking AI architecture
While large language models (LLMs) have captured public imagination,
Ramprakash Ramamoorthy / ManageEngine
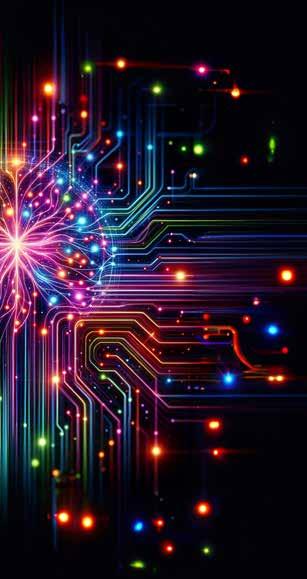
Ramamoorthy is quick to point out their limitations in enterprisegrade IT environments. “While the industry primarily focuses on LLMs, their accuracy in structured IT environments is limited due to the over-optimisation of natural language tasks,” he notes.
The future, he argues, lies in hybrid AI frameworks that go beyond language tasks to deliver contextual, real-time intelligence tailored to enterprise workflows. This is where agentic systems—AI agents that bridge structured and unstructured data—play a critical role.
ManageEngine’s contextual AI strategy, built on this multi-layered
architecture, is shaped by years of operational learning and security insights. But as Ramamoorthy stresses, what matters most isn’t the model—but the outcomes. “At the end of the day, AI should reduce friction, not add more complexity. It should help teams operate with more clarity, speed, and confidence.”
Intervention to orchestration
As AI takes on more responsibility across IT operations, the conversation around automation often turns to displacement. But Ramamoorthy frames the shift differently. Rather than
managing systems rather than being overwhelmed by alerts and interventions,” Ramamoorthy explains.
This human-AI partnership is also a crucial piece of the trust equation. When organisations retain the ability to review, refine, and override AI outputs, they’re better positioned to scale automation without sacrificing accountability.
Moving forward
Looking ahead, Ramamoorthy sees the enterprise IT landscape moving from automation to decision intelligence—where AI systems continuously learn, adapt, and optimise in real time.
“FOR AI TO TRULY ADD VALUE IN ENTERPRISE IT, ITS DECISION-MAKING MUST BE TRANSPARENT, TRACEABLE, AND AUDITABLE”
“In the next three to five years, AIpowered IT management will evolve into self-optimising ecosystems that unify IT, security, and business workflows,” he says. “AI will advance beyond task execution to decision intelligence, resolving complex incidents.”
replacing talent, AI presents an opportunity to redefine roles— freeing professionals from reactive firefighting and enabling them to lead with strategic intent.
“IT professionals should transition from manual task execution to AI-augmented decision-making,” he says. “The goal is not to replace human expertise but to enhance it with AI-driven insights.”
This vision is one of collaboration— where AI handles repetitive, timesensitive tasks like incident triage, while humans focus on judgment, exception handling, and policydriven decisions. “IT professionals will evolve into AI orchestrators,
This evolution will demand more robust integration of AI across the stack—merging observability, incident response, access management, and analytics. But it will also demand a greater commitment to ethical guardrails, contextual performance, and adaptive learning.
“ManageEngine will focus on shaping this evolution through a hybrid framework, combining IT-native foundational models, agentic systems, and LLMs to deliver context-aware solutions,” he says. “By prioritising explainability, privacy, and ethical AI, ManageEngine will empower enterprises to ride the tech waves that matter confidently.”
As enterprise IT moves into an AIfirst era, leaders must navigate more than just a technology shift—they must rethink roles, responsibilities, and the very fabric of decisionmaking.
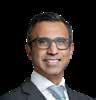
Abhijit Dubey / NTT Data
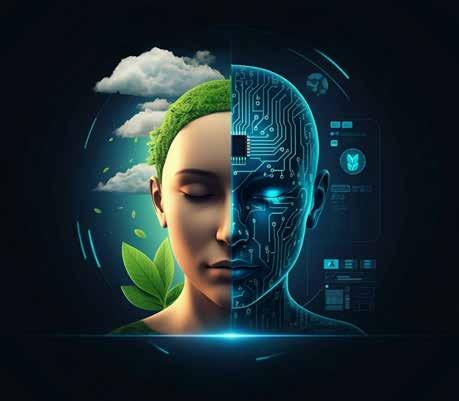
THE AI RESPONSIBILITY CRISIS
Abhijit Dubey, CEO at NTT Data, discusses why senior leaders need to align AI innovative with ethical responsibility
AI has hit fever pitch. It’s perhaps the most pervasive and disruptive technology of this millennium, yet it’s still in its infancy. It’s transforming industries in ways we couldn’t have anticipated, reshaping how organisations operate, fueling new economies and changing the workforce.
This explains why more than 60 percent of organisations surveyed for NTT DATA’s landmark Global GenAI Report believe GenAI will be a game changer within two years, and almost 70 percent are optimistic about the technology. Furthermore, nearly two-
thirds of respondents plan to invest significantly in GenAI in 2025 to 2026, proving there is no slowdown in its growth.
In the C-suite, specifically, 64 percent of executives expect significant transformation in their industry in 2025 thanks to major investments in GenAI. This is significant because leaders’ commitment to AI and GenAI will determine the success of the technology as their belief in its power trickles down to the entire organisation, shaping how it is embraced, integrated and scaled as a strategic priority.
In short, GenAI — and AI more broadly — is transforming the DNA of core organisational strategies and forcing most enterprises to fundamentally reevaluate their technological opportunities. Following a period of experimentation and ideation around AI in organisations, this year will see an increasing focus on identifying tangible GenAI successes that can be taken into production.
Balancing innovation and responsibility
Based on responses from more than 2,300 senior leaders in diverse roles and industries across 35 countries, the perspective presents concrete data that showcases the urgent need for a leadership-driven mandate to align AI innovation with ethical responsibility. Without it, we compromise security, introduce ethical bias and miss opportunities to grow AI sustainability. While one in three in the C-suite says responsibility matters more than innovation when it comes to AI and GenAI, nearly the same number say innovation matters more — and the remainder believe innovation and responsibility are equally important. This shows the C-suite is at odds with itself about where the balance between innovation and responsibility should lie. One trend is evident, though: as investment in GenAI surges, the gap widens.
Our perspective explores the C-suite’s views on the extent of the gap and its impact on society and business, as well as the main reasons for organisations leaning more toward innovation than responsibility and vice versa. There’s also the rising need for training the workforce as investment increases — an ongoing evolution that requires constant management.
The findings are a clear call to action for the business community: it’s beyond time to act. Embed responsibility into the foundations of AI. Unlock its full potential. Become a part of the solution.
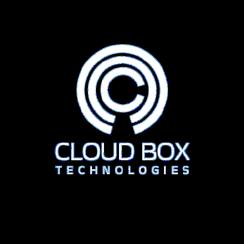
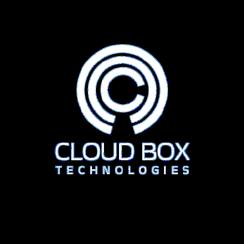

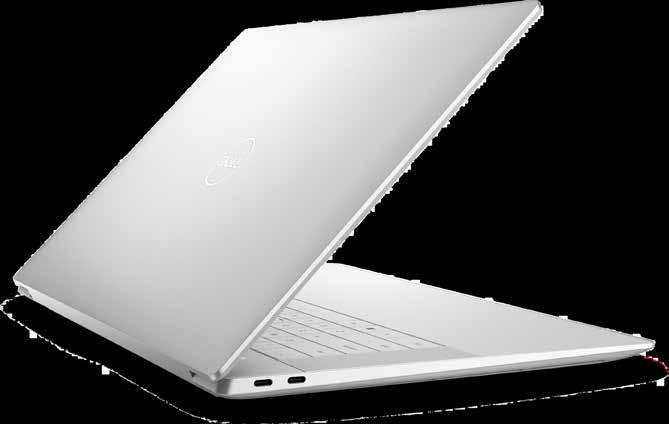

Walid Gomaa / Omnix International
From blueprint to bottom line
Walid Gomaa, CEO at Omnix International, explains how organisations can move from AI ambition to real business results by focusing on the right use cases, data readiness, and strategic execution
The success of enterprise AI will not be determined by models or algorithms—it will be defined by how effectively organisations turn ambition into architecture. In regions like the Middle East, where national strategies have fast-tracked AI as a pillar of economic transformation, the spotlight has now shifted to execution: how to operationalise AI at scale, align it with measurable value, and embed it into real workflows.
This shift exposes a hard truth: many enterprises are pursuing AI without a clear understanding of where to begin, how to measure success, or what outcomes to target. Strategy often outpaces structure— and without disciplined use case identification, AI risks becoming just another siloed initiative.
“Business leaders want AI, but they don’t know how to start,” says Walid Gomaa, CEO at Omnix International. “Even if they start, they don’t know how to create a proper ROI to get money and get funding… unless you have a customer who's ready to fund without ROI, then the implementation of AI is going to be a challenge.”
This ambiguity around return on investment is one of the main
“AI IS HERE TO ENHANCE THE SKILLS WE ALREADY POSSESS—IT’S NOT ABOUT REPLACEMENT. IT’S ABOUT PARTNERSHIP”
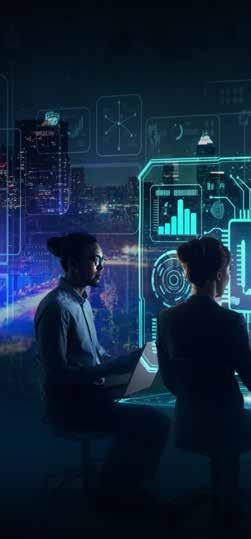
reasons AI initiatives stall, Gomaa explains. Organisations may launch pilot projects based on industry hype or vendor promises, only to realise later that business value was never clearly defined. “Sometimes, they say AI didn’t help. But the reality is, maybe the wrong use case was implemented—or there was no proper ROI model to begin with.”
Bridging this disconnect requires building a business-driven foundation—starting not with a proof of concept, but with a clear monetisation strategy. It calls for solutions that shift the focus from technical experimentation to commercially grounded execution. Services like Omnix’s AI Monetisation offering have emerged to address this need.
“This service is only about making sure that you break the gap between
the business users and IT users,” says Gomaa. “The outcome is a clear strategy for AI, clear implementation of use cases, clear ROI—and it’s all coming from business users.”
By transforming fragmented efforts into enterprise-aligned roadmaps, the service enables organisations to approach AI not as a siloed project, but as a strategic capability— embedded with purpose, prioritised for value, and engineered for scale.
The need for an AI talent strategy
AI is reshaping how work gets done— but without the right skills, even the most advanced technology will fall short. Gomaa sees two layers to this challenge. On one hand, there’s a shortage of deep AI expertise, such
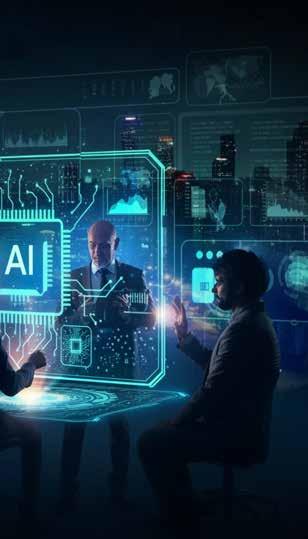
as data scientists, machine learning engineers, and AI strategists. On the other, there’s a readiness gap across business functions.
“You need a data engineer, data scientist, strategist. They were not there before... now these guys are becoming very important to the implications,” he adds. “To find them and afford them is a question... because they are very few and very expensive.”
This cost barrier has led some organisations to delay their AI ambitions. But Gomaa argues that the solution isn’t simply hiring—it’s about enabling existing teams to work effectively alongside AI.
Whether it’s a doctor interpreting AI-assisted diagnostics or an engineer responding to a predictive
maintenance alert, humans remain central to the process. Gomaa stresses that the future lies in human-machine collaboration, not replacement.
“AI is here to enhance the skills we already possess—it’s not about replacement. It’s about partnership. In this hybrid model, AI handles part of the work, while human expertise completes the picture. The true power of AI lies in collaboration, not substitution,” he says.
The unseen barrier to AI success
While talent and strategy are essential, AI adoption will fail without one critical ingredient: clean, consistent data.
“If the data is inaccurate, the whole thing will collapse. You have to have data management, data governance, and make sure that the data is accurate.”
Data inconsistencies are especially common in large organisations, where conflicting datasets reside across departments. This makes it difficult to determine which version is accurate—and feeding this ambiguity into an AI system only compounds the issue.
“In large organisations, you can find the same data coming from different sources, and they are all different. So which one of them is the real data?”
To address this, Gomaa advocates for building data governance frameworks early in the AI journey— before experimentation begins. Data cleansing, integration tools, and quality controls must be treated as foundational infrastructure, not afterthoughts.
Augmentation in action
Beyond the headlines about AI replacing jobs, Gomaa sees a more pragmatic and valuable application: intelligent augmentation. Tools like conversational agents and copilots
demonstrate how AI can accelerate productivity while keeping human judgement in the loop.
“You need to analyse the feedback [from AI] and take action on that... It’s all about making my work better, not replacing my work.”
Even in areas like predictive analytics, machines offer probabilities—but the decision ultimately rests with the human.
“Machines cannot take a decision. An engineer will take a decision based on the input, but now he's adding more quantitative data to base his decision, rather than depending only on his 80/60.”
This is the model of the future: AI as an advisor, a companion in problem-solving—not a standalone authority.
The way forward
As enterprises move beyond experimentation, the focus is shifting from generic AI tools to purposebuilt, context-aware solutions. Offthe-shelf AI tools can demonstrate potential, but domain-specific use cases are what ultimately unlock real business value.
“ChatGPT is a generic engine. But the real value is in understanding— how can I use the ChatGPT engine for real estate? How can I use it for manufacturing? Then this becomes the use case specifically for this industry,” says Gomaa.
This perspective will shape Omnix’s AI strategy going forward, with a focus on developing solutions tailored to industry-specific needs, compliant with regional regulations, and capable of running closer to the data—whether on laptops, desktops, or secure on-premises infrastructure.
Gomaa believes this direction is especially relevant in the Middle East, where national AI strategies and data sovereignty mandates are heavily influencing how and where AI can be deployed.

A NEW DAWN FOR AI
Patrick Smith, Field CTO EMEA at Pure Storage, unpacks why the future of AI hinges on data—its quality, accessibility, and the storage performance required to fuel both foundational training and domain-specific fine-tuning

Patrick
Smith / Pure Storage
The 30th of November 2022 was a monumental day. That was the day that ChatGPT was released to the world by OpenAI, the rest is history; literally. It’s been two years since then and we’ve seen a meteoric rise in the interest in AI. This has led to an almost 10x increase in the market capitalisation of Nvidia, the leading maker of GPUs, and wild predictions around the potential total investment by businesses in AI, as well as the impact it will have on society.
This feels very different to the previous AI dawns we’ve seen over the last 70 years, from the Turing Test, defeats of chess grand masters, to autonomous driving and now the Generative AI explosion. The game has well and truly changed, but it’s still based on certain fundamental concepts. For many years, AI advancements have been built on three key developments; 1) more powerful compute resources — in the form of GPUs; 2) improved algorithms or models — in the case of Generative AI the Transformer architecture and large language models (LLMs), and finally; 3) access to massive amounts of data. At a very high level the phases of an AI project include data collection and preparation, model development and training, and model deployment also known as inference.
It’s all about the data
Successful AI projects rely on highquality, relevant, and unbiased data. However, many organisations struggle with understanding their data, defining
“Successful AI projects rely on highquality, relevant, and unbiased data
ownership, and breaking down silos. Without good data, AI initiatives are likely to fail. Increasingly, AI projects use multimodal data—text, audio, images, and video—driving significant storage demands.
Training the model
There are two primary approaches to AI model training. Foundational model training builds a model from scratch using vast datasets and resources, typically by tech giants. For instance, Meta trained its open-source Llama 3.1 model with 405 billion parameters using 15 trillion tokens—reportedly taking 40 million GPU hours. This scale demands highperformance, high-capacity storage for checkpointing to recover from failures.
The alternative is fine-tuning existing models with domain-specific data. This allows organisations to leverage pretrained models and customise them without starting from zero.
Regardless of approach, model training requires massive GPU parallelism, demanding storage systems with high throughput, fast access speeds, scalability, and reliability to keep GPUs supplied with data and avoid costly disruptions.
Into production
Once a model has been trained, and its performance meets requirements, it’s put into production. This is when the model uses data it hasn’t seen before to draw conclusions or provide insights. This is known as Inference, and is when value is derived from an AI initiative. The resource usage and cost associated with inferencing dwarfs that of training because inferencing has demands on compute and storage
on a constant basis and potentially at massive scale; think about millions of users accessing a chatbot for customer service.
The underlying storage for inferencing must deliver high performance as this is key to providing timely results as well as easy scaling to meet the storage requirements of the data being fed into the model for record-keeping and to provide retraining data. The quality of the results from inferencing is directly related to the quality of the trained model and the training data set. Generative AI provided a twist to the accuracy of inferencing; the nature of Generative AI means that inaccuracies are highly likely, known as hallucinations. These inaccuracies have caused problems that have frequently hit the headlines.
Improving accuracy
Users of ChatGPT will realise the importance of the query fed into the model. A well-structured comprehensive query can result in a much more accurate response than a curt question. This has led to the concept of “prompt engineering” where a large well-crafted dataset is provided as the query to the model to yield the optimal output.
An alternative approach that is becoming increasingly important is retrieval augmented generation, or RAG. RAG augments the query with an organisation’s own data in the form of use-case specific context coming directly from a vector database such as Chroma or Milvus. Compared to prompt engineering, RAG produces improved results and significantly reduces the possibility of hallucinations. Equally important is the fact that current, timely data can be used with the model rather than being limited to a historic cut-off date.
RAG is dependent on vectorising an organisation’s data, allowing it to be integrated into the overall architecture. Vector databases often see a significant growth in the dataset size compared to the source — as much as 10x — and are very performance sensitive given that the user experience is directly related to the response time of the vector database
query. As such the underlying storage in terms of performance and scalability plays an important part in the successful implementation of RAG.
The AI energy conundrum
The past few years have seen electricity costs soar across the globe, with no signs of slowing down. In addition, the rise of Generative AI means that the energy needs of data centres have increased many times over. In fact, the IEA estimates that AI, data centres, and cryptocurrency power usage represented almost two percent of global energy demand in 2022 — and that these energy demands could double by 2026. This is partly due to the high power demands of GPUs that strain data centres, requiring 40-50 kilowatts per rack — well beyond the capability of many data centres.
Driving efficiency throughout the datacenter is essential meaning infrastructure like all-flash data storage is crucial for managing power and space, as every Watt saved on storage can help power more GPUs. With some all-flash storage technologies, it’s possible to achieve up to 85 percent reduction in energy usage, and up to 95% less rack space than competing offerings, providing significant value as a key part of the AI ecosystem.
Data storage part of the AI puzzle
AI’s potential is almost unimaginable. However, for AI models to deliver, a careful approach is needed across training, whether foundational or fine tuning, to result in accurate and scalable inference. The adoption of RAG can be leveraged to improve the output quality even further. It’s clear that in all stages data is a key component, flash storage is essential in delivering AI's transformative impact on business and society, offering unmatched performance, scalability, and reliability. Flash supports AI's need for real-time access to unstructured data, facilitating both training and inference, while reducing energy consumption and carbon emissions, making it vital for efficient, sustainable AI infrastructure.
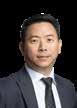
Thomas Pramotedham / Presight AI
Purpose over hype
Thomas Pramotedham,
CEO of Presight AI, he
discusses how contextual intelligence, synthetic data, and agent-driven models are unlocking AI opportunities at scale
As the AI landscape matures, enterprises and governments are moving beyond questions of capability and turning toward one defining challenge: operational relevance. In a world where artificial intelligence is expected to integrate with national infrastructure, industrial systems, and policy-driven mandates, the models that matter most are not the largest or most novel—they are the ones that work, consistently, and in context.
This shift calls for a new breed of AI—models engineered to perform specific tasks with domain awareness, regulatory compliance, and measurable efficiency. It marks a transition away from generalpurpose language models and toward applied, agentic AI: systems that understand their environment, execute targeted functions, and collaborate with human decisionmakers to accelerate outcomes.
“In our mind, the team’s mind, AI is in how we apply it,” says Thomas Pramotedham, CEO of Presight AI. “The whole conversation around applied intelligence, applied technology, is what drives us.”
That mindset underpins Presight’s strategic approach across sectors such as energy, national resilience, and public services. The goal is not to
build AI that does everything—but to build models that do the right things extremely well.
From Generalist Models to Purpose-Built Agents
Presight’s work in the energy sector highlights the growing importance of specialisation in AI design. In collaboration with ADNOC through its joint venture AIQ, the company developed a domain-specific large language model tailored for upstream oil and gas operations. What set this project apart was not just the model’s technical capability—but its purposedriven training.
“We literally had it trained three weeks over… to be able to do specific tasks for upstream oil and gas or reservoir analysis and so forth,” Pramotedham explains. “That becomes proof of the pooling that you need to train agent.”
Rather than relying on broad, generalist intelligence, Presight prioritised training the model on highly specific workflows—validating the emerging view that targeted, contextualised models are more effective than vast, open-ended ones when it comes to enterprise use.
“You don’t need large language models that know everything,” he adds.
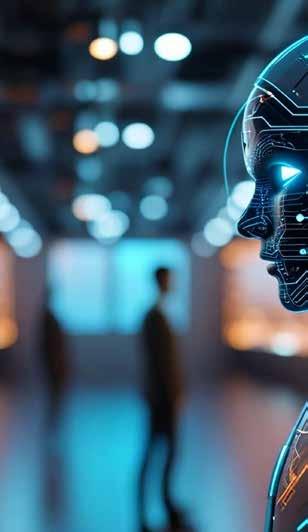
“You need specialised models that do something really well.”
These models are designed to operate within human workflows— not to replace them. Pramotedham envisions a future in which intelligent agents and domain experts exchange tasks in a loop, each contributing what they do best. “The future is the agent handing a task to me, I complete my part and pass it back, and the agent continues the workflow—eventually even coordinating with other agents. We will become part of a collaborative digital workforce.”
Operationalising AI in government This collaborative philosophy is equally evident in Presight’s work with the public sector, particularly
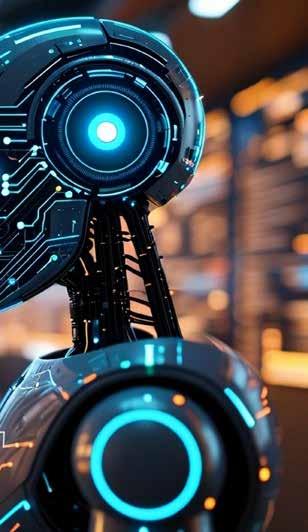
in Abu Dhabi, where the company has supported AI-native governance initiatives since the pandemic. From national emergency response to urban planning, the company’s systems are deployed in real-world environments with high sensitivity, high stakes, and evolving policy needs.
“Our involvement has always been right-sizing the technology to fit the use case,” Pramotedham says. “And the way we have organised ourselves is always learn about the use cases—not just the technology.”
When supporting the digitisation of workflows for entities such as the Abu Dhabi Accountability Authority, the objective was simple: replace manual processes with scalable, intelligent systems. But as Pramotedham points
“YOU DON’T NEED LARGE LANGUAGE MODELS THAT KNOW EVERYTHING. YOU NEED SPECIALISED MODELS THAT DO SOMETHING REALLY WELL”
out, what matters is not how advanced the technology is—but whether it solves the problem at hand.
“They just need to move this stack of paper to a database that I can use,” he says. “What you use in between? You know, it’s not ‘do my workflow’—it’s make the output usable.”
This pragmatic approach—starting from the need, not the tool—has become critical for governments seeking to modernise at speed while maintaining trust, compliance, and cost-efficiency.
Data integrity and the rise of synthetic environments
AI adoption at scale also raises the perennial question of data—how it is accessed, governed, and protected. In regulated sectors like healthcare, energy, and national security, the balance between innovation and privacy is delicate. Presight operates under a strict model: it builds platforms, but never accesses customer data.
“The data doesn’t belong to us… The data privacy, data usage laws are governed by the government. So we don’t access the data,” says Pramotedham.
This approach has accelerated the company’s investment in synthetic data generation. In the development of Arabic LLMs, Presight faced significant challenges in sourcing high-quality, annotated, domain-specific datasets. The solution was to simulate them.
“By the time we released the 130-billion parameter model… most of
it was synthetic data,” Pramotedham says. “There’s just no data to grab.”
This innovation is becoming standard practice across the industry.
Synthetic data not only addresses scarcity—it also ensures models can be trained in a secure, controlled manner while meeting compliance requirements across jurisdictions.
Navigating AI's ethical boundaries
As AI agents become more autonomous and media generation tools more sophisticated, the line between real and synthetic continues to blur. The risk of misinformation, identity manipulation, and deepfakes now demands serious attention from policymakers, developers, and platforms alike.
“We could have had this completely done in AI and not known what’s true or not,” says Pramotedham. “That scares me a little, because the deepfakes are so strong.”
This risk, however, doesn’t outweigh the opportunity. When developed responsibly, AI can be a powerful leveller—expanding access to services, accelerating healthcare outcomes, and improving infrastructure planning across regions.
“It will help solve many of the problems in health… It will make advancement equitable… You don’t have to be privileged to have access,” he says.
The path forward
As AI moves from labs to missioncritical systems, organisations must shift focus—from building powerful models to building relevant ones. Scale, alone, is no longer the benchmark. Precision, trust, and impact are.
AI that is designed for the environments it serves—governed responsibly, trained efficiently, and deployed strategically—will define the next phase of digital transformation.
This is the model Presight AI is working to shape: applied intelligence, built for the real world.
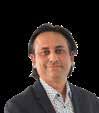
Narender Vasandani / Siemon
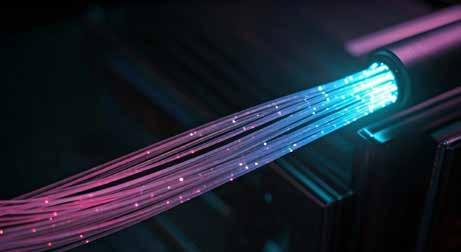
WIRED FOR THE NEXT
Narender Vasandani, Technical Services Group Manager for India, Middle East and Africa at Siemon, explains why businesses need to adapt new structured cabling technologies to prepare for today's and tomorrow's AI needs
Artificial intelligence (AI) is putting tremendous strain on existing data centre infrastructure. To support the increasingly complex workloads that AI applications generate, today’s data centres must deliver network speeds of 400Gb/s, 800Gb/s and even 1Tb/s. Data centre cabling infrastructure is therefore becoming a strategic asset which must not only meet the performance needs of today but also remain scalable in the future.
Growing demands
Facilitating increased network speeds is not the only challenge that data centre owners and operator are facing. Demands for improved latency and network scalability as well as increased port density and associated power and cooling needs are moving into focus too. For the cabling infrastructure this means
that it must be of the highest quality.
With AI workloads relying on realtime data processing, small defects in fibre optic components for example can introduce latency and signal loss, impacting performance.
Delivering the right performance at higher speeds also means that optical loss must be at a minimum. The larger the number of connections in an optical link, the greater the attenuation will become. Low-loss OM4 multimode and OS2 single-mode fibre solutions are meeting these performance requirements today. At the same time, they offer a seamless migration path to even higher speeds in the future.
Selecting cables and components from a trusted manufacturer that offers a comprehensive warranty is key but managing them correctly is equally important.
High speed interconnects for the front end
At the data centre edge, where speeds are now exceeding 100Gb/s in server-toswitch connections, a design approach that deploys short-reach, high speed cable assemblies in a top of rack configuration is a valuable option. These cable assemblies support faster speeds but also offer lower latency and better power efficiencies.
High speed cable assemblies can support transmission speeds from 10GB/s all the way to 800Gb/s, which means that data centre facilities can easily upgrade their network equipment to higher speeds as and when required in the future.
Amongst these point-to-point high-speed cabling options are Direct Attach Cables (DACs), Active Optical Cables (AOCs) and transceiver assemblies. DACs are best suited for in-rack connections. They consume hardly any power (up to 0.05W only), support high density server connections and offer low latency. AOCs support lengths to up to 30 metres, feature a smaller cable diameter and are well suited for higher density inner cabinet breakout connections in a ToR topology. Transceiver assemblies can cover up to 100 meters in length and are suitable for connecting rows. Due to a small cable diameter, they support better airflow. For next generation networks DACs, AOCs and transceiver assemblies offer a very flexible deployment option.
High density fibre for the back end
For switch-to-switch connections in the data centre backbone, a highdensity fibre infrastructure will prepare data centres for the bandwidth needs required by AI. Network professionals however must consider the requirements of their applications first. A high-density (HD) fibre connectivity system, which supports between 72 and 96 LC fibres per rack unit, will most likely serve the majority of applications, whereas an ultra-high density (UHD) connectivity solution that supports at least 144 LC fibres per rack, will serve the demands of heavy compute AI.
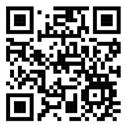
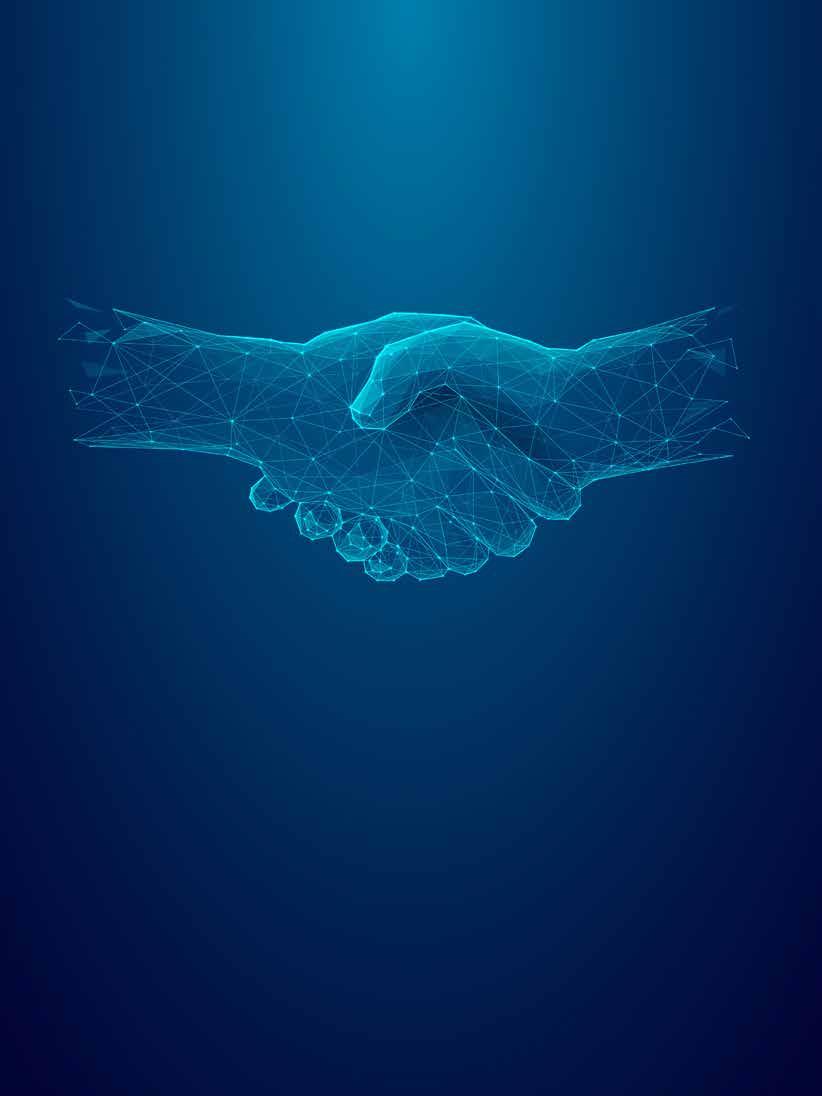
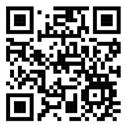
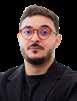
Dr.
Chaouki Kasmi / TII
Future forward
Dr. Chaouki Kasmi, Chief Innovation Officer at the Technology Innovation Institute (TII), shares how AI is moving from the lab to the heart of enterprise operations
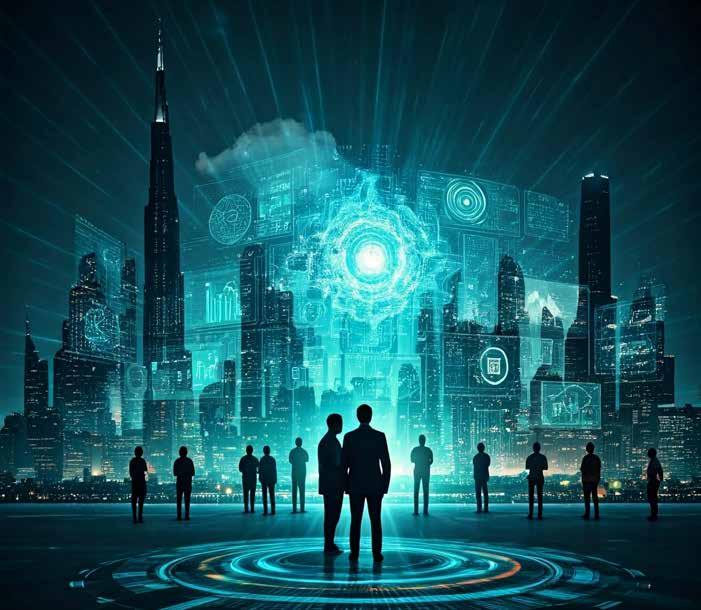
Across every sector, AI is shifting from a supporting role to a central driver of transformation. No longer confined to R&D labs or innovation teams, it is being embedded into the core of operations—from accelerating product design in manufacturing to enhancing clinical decision-making in healthcare and reimagining engagement in education. The question is no longer whether to adopt AI, but how to integrate it in ways that are scalable, secure, and
aligned with strategic outcomes. This shift demands more than just technological readiness—it calls for a rethinking of entire operating models. At the Technology Innovation Institute (TII), this philosophy is shaping how AI is developed, deployed, and governed.
“Most of the time, when you talk to people, they think that it's a new application that they install… and this will do the job for them,” says Dr. Chaouki Kasmi, Chief Innovation Officer at TII. “But they need to understand that AI is more than a plug-and-play solution. It’s an opportunity to fundamentally rethink how our operating models work.”
AI and automation are fundamentally altering how industries operate—from factory floors to hospital wards to classrooms.
“In manufacturing, smart systems are streamlining production processes, requiring workers to develop more advanced technical skills. Robotics is making these processes more adaptive, transforming how products are made and managed,” says Dr. Kasmi.
Healthcare, too, is evolving rapidly, as AI enables better diagnostics and surgical precision. Yet, the technology is only as impactful as the people working with it. “AI-powered diagnostics and robotic surgeries are improving patient outcomes, but they demand healthcare professionals who can adapt to and work with new technologies.”
Education is no exception. AI is changing how teachers teach and how students learn, creating new roles for educators in digital-first environments. “This shift is creating a need for educators who are digitally skilled and prepared to innovate in the classroom.”
AI is becoming a catalyst for a new kind of workforce—one that is techfluent, agile, and adaptable.
“AI IS MORE THAN A PLUGAND-PLAY SOLUTION. IT’S AN OPPORTUNITY TO FUNDAMENTALLY RETHINK HOW OUR OPERATING MODELS WORK”
Beyond AI
While AI continues to dominate the innovation agenda, Dr. Kasmi believes 2025 will also bring other paradigm-shifting technologies to the forefront. “AI will continue to be a major driver of innovation, but 2025 is shaping up to be a big year for quantum computing, especially with the International Year of Quantum shining a spotlight on its incredible potential.”
Quantum computing is expected to solve problems beyond the reach of classical systems, particularly in cryptography, logistics, and complex optimisation tasks. “Quantum computing can tackle problems that traditional systems simply can’t handle, making it a game-changer.”
Momentum in space exploration is also growing, driven by increased investments from both governments and private entities. “These efforts are pushing forward satellite technology and interplanetary missions, bringing us closer to new discoveries and opportunities beyond our planet.”
Biotechnology, too, is on the cusp of transformation. Dr. Kasmi highlights advancements in gene therapy, regenerative medicine, and bioengineering that are “transforming healthcare and agriculture, offering solutions to some of the world’s most urgent challenges.”
The UAE’s Bold Bet on AI
At the heart of this innovation surge is the Middle East—particularly the
UAE—which is fast emerging as a global technology powerhouse.
“The Middle East, particularly the UAE, is set to play an important role in the global tech ecosystem in 2025,” says Dr. Kasmi. “With significant investments in technology and infrastructure, the region is becoming a vibrant innovation hub.”
This momentum is fuelled by the UAE’s government-led digital vision and a strong appetite for international collaboration.
“Strategic collaborations with international tech firms and research institutions are helping integrate the UAE further into the global technology landscape.”
TII is a cornerstone of this national ambition, leading research and innovation efforts that address realworld challenges while promoting sustainability and inclusivity.
This extends beyond developing technologies—it’s about building capabilities. “We have put 100% of our staff into AI training programs, whether we talk about support services, human resource, functionality and finance to technicians, managers, C-level… everyone went to tailor training… to really understand the technology, apply the technology and develop the technology.”
The result is a workforce primed to drive AI adoption across disciplines— an essential pillar of the UAE’s vision to become a global AI leader.
As AI scales, Dr. Kasmi emphasises the importance of pairing innovation with ethical responsibility and deeper societal understanding. That means addressing questions around safety, transparency, and trust—especially in high-stakes applications. “When we bring AI in critical technologies or sensitive systems where human [lives are] involved, then you talk about the safety of AI, functional safety of AI… how we are sure that the AI will perform the way it should be.”
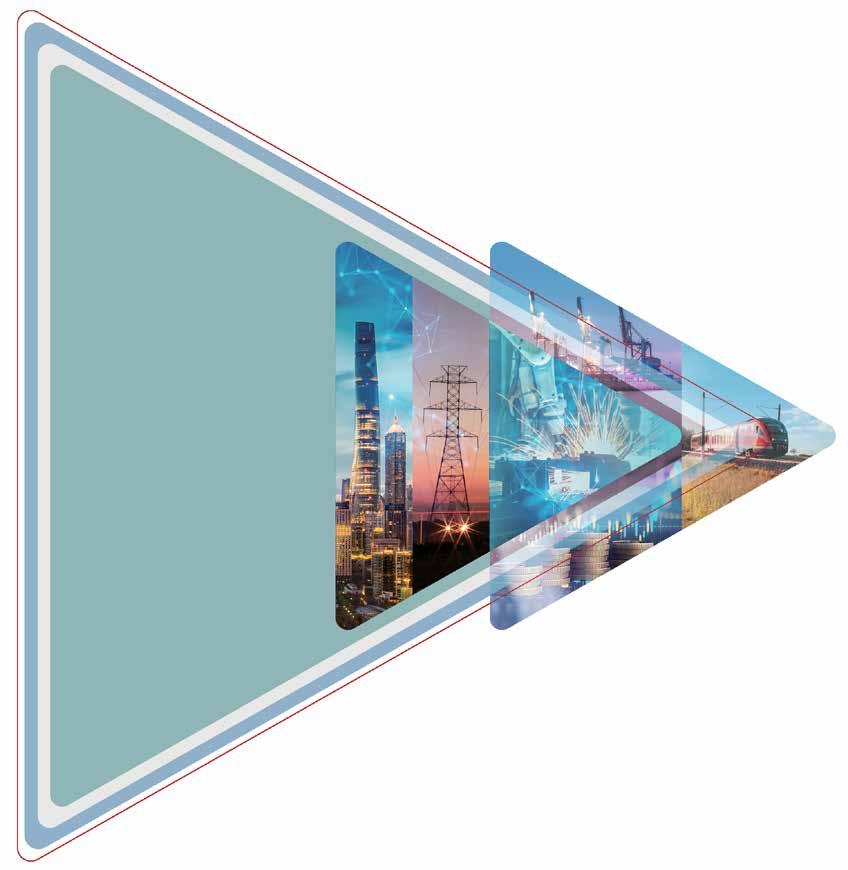
Accelerating the Intelligent World

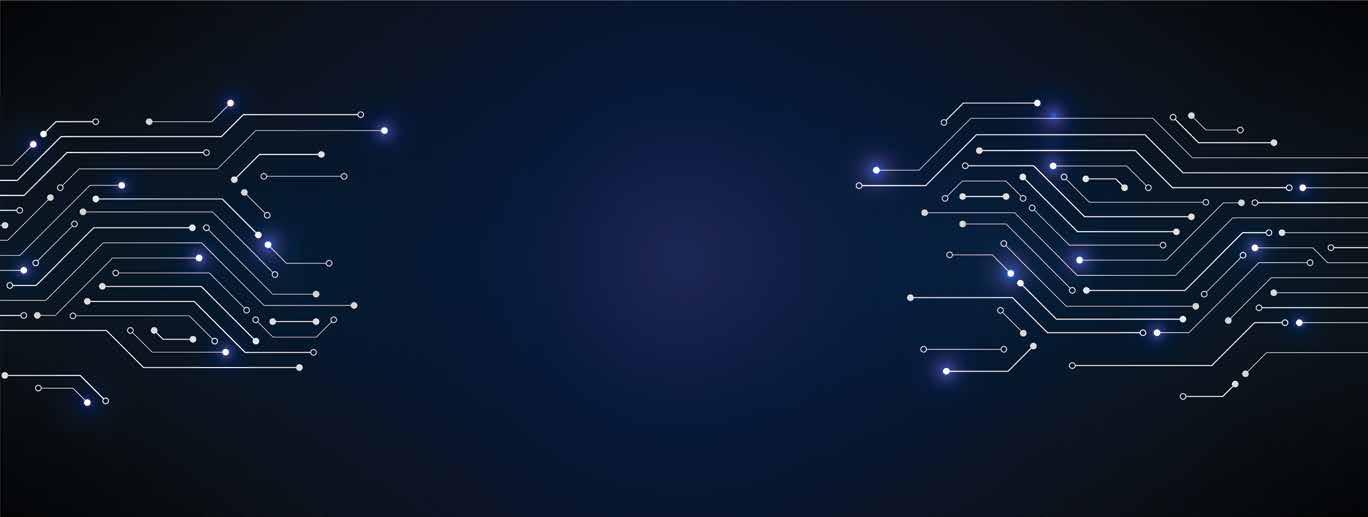
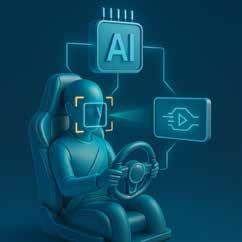
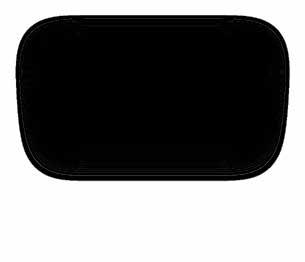
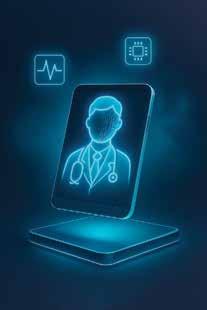
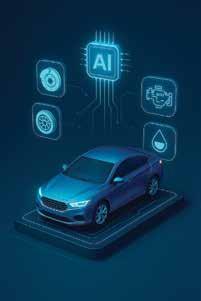
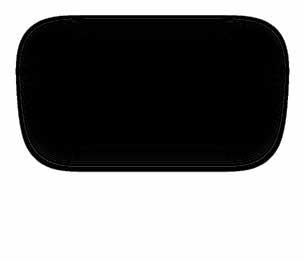

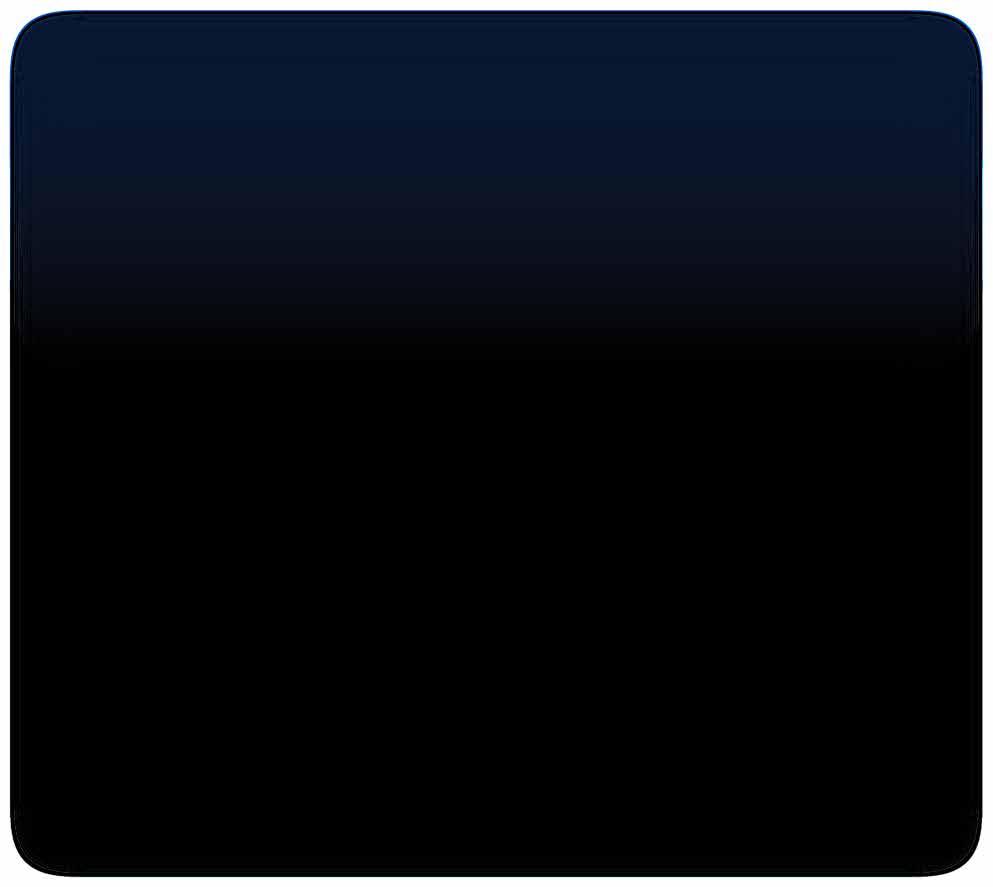
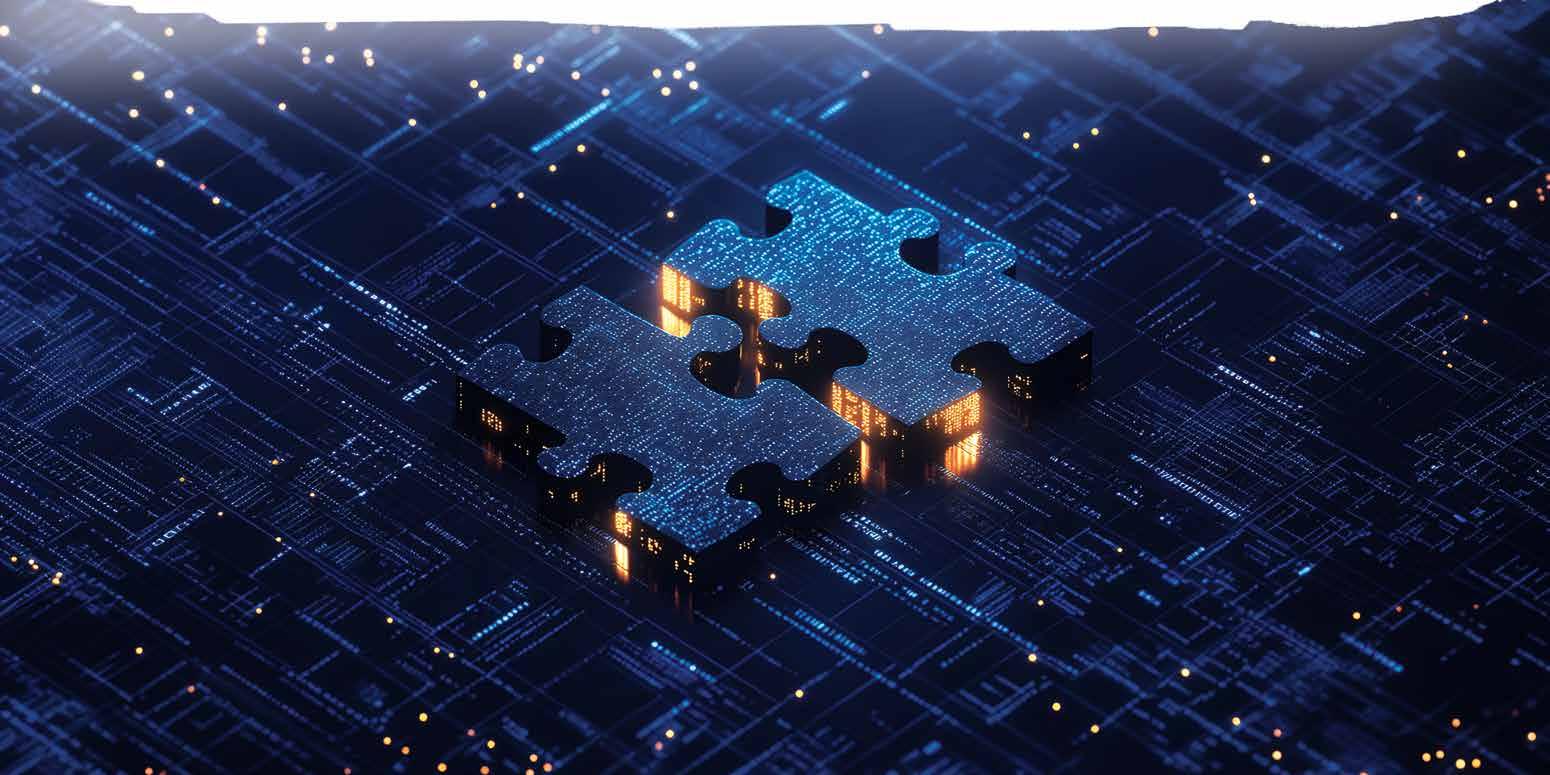
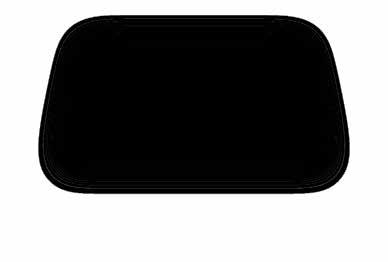