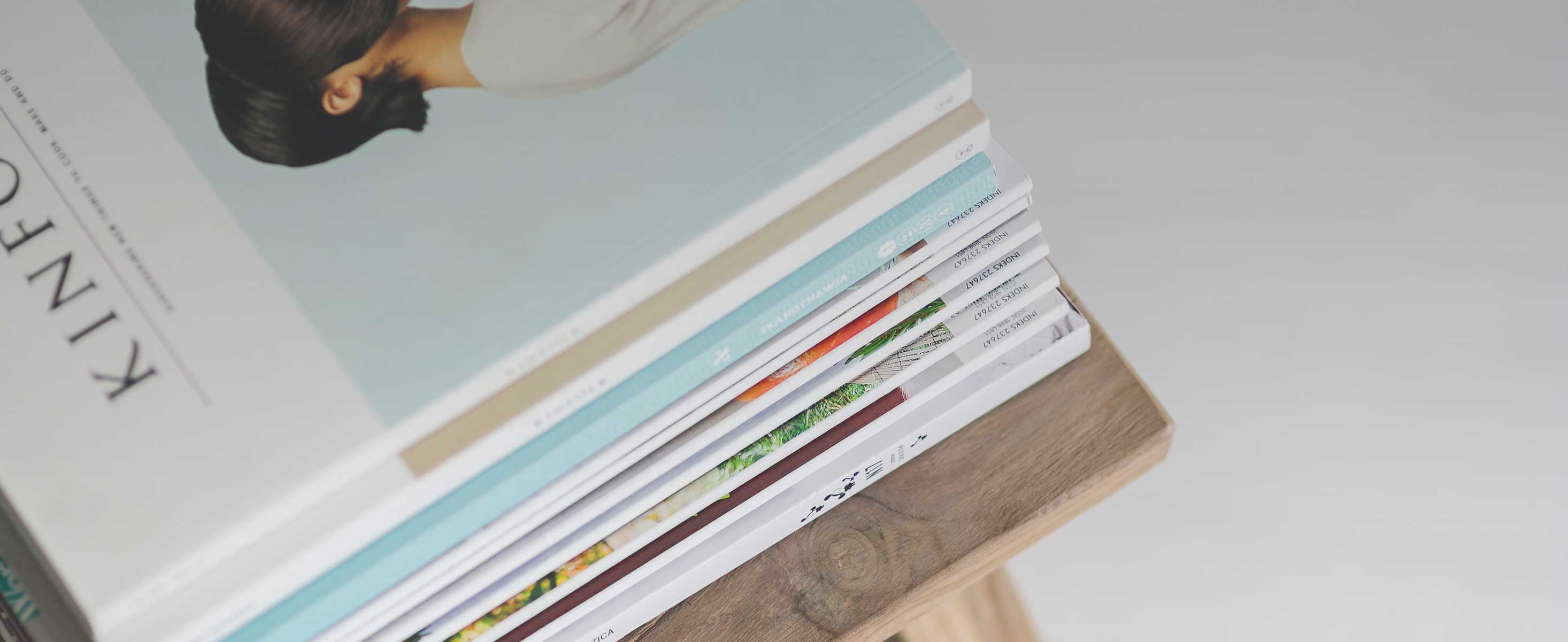
3 minute read
THE TRUE COST OF AI
from 50 CHANGE MAKERS
by cxoinsightme
GREGORY HERBERT, SVP & GM – EMEA AT DATAIKU, ON THREE STEPS TO REDUCING THE COST OF AI PROJECTS
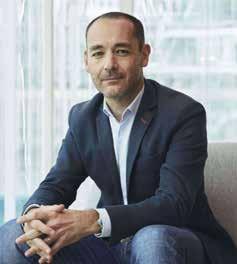
Advertisement
These days, every regional business leader spends at least some of their time each day thinking about AI — its uses, its implications, its potential. The United Arab Emirates (UAE) can be thought of as a pioneer in artificial intelligence. It was the first country to appoint a minister of state to oversee the field. It is home to organisations like Dubai Electricity and Water Authority (DEWA) and Etisalat (by e&) that use bots for customer service. And it has a national Strategy for Artificial Intelligence. Enterprises here have even shown a willingness to commit to the deep and broad culture changes required to deliver Everyday AI, where AI becomes second nature to the entire workforce.
But despite the will of the innovators, AI projects can be stubborn when it comes to delivering their expected value. According to a March 2022 global report from Gartner, the ROI on AI projects varies from 20% to more than 800%. The problem arises from early cases classically being quick wins. The law of diminishing returns then applies to subsequent initiatives, but this is aggravated by increasing maintenance and execution costs.
So the challenge for organisations that want to create an Everyday AI culture becomes controlling costs while deploying at scale. There are three main steps to reducing the costs associated with AI projects.
1. Reuse and recycle
Every project is broken into manageable chunks for ease of implementation. In IT solutions development, everything from the smallest code snippet to data cleaning and even entire solutions can be repurposed and reused. Within an
Everyday AI enterprise, there will be professional coders who will know the basic resource economies that accompany reuse. But there will also be citizen developers who may need training in how to build code libraries and consolidate work into a repository for future reuse.
When it comes to data-cleaning and preparation, the repository model does not apply directly but the basic concept is the same. Prep work is labor-intensive, and organisations should devote some time to ensuring such tasks are not repeated. A centralised catalog of prepped data, similar to code libraries, will save a lot of time.
It makes sense to procure tools and establish rules and processes that ensure data can be prepared, and code written, just once and used many times. This can be of particular use to nontechnical business users who would otherwise have to wait for a developer or data scientist before creating value.
2. Many use cases for the price of one Once reuse procedures are nailed down, earlier projects can share their costs across all those that follow. The Everyday AI model calls for transparency and a breakdown of information and departmental silos. If we follow this concept and combine it with the newly introduced practice of reuse, we could envisage a marketing employee taking a use case developed in the customer service function and building something else of value on top it with only a fraction of the effort that the original required.
This is the value of transparency. Of knowing what others have done and how it applies to the next use case and the one after that. And the tools are out there to facilitate such openness and allow all roles — from the most experienced data scientist to business analysts with basic spreadsheet skills — to feel the power of AI. This is often referred to as “data democratisation” and is a critical pillar of Everyday AI.
3. Efficiency across the AI lifecycle
AI projects do not tend to be linear, and they involve a rich community of job titles. If this pipeline is improperly managed, repetition is bound to occur, and keep occurring. There are three main areas where simple efficiency can help control costs, and the first is operationalisation, where AI solutions are released into the wild. Packaging and deploying take time and in an Everyday AI culture these releases can run into the hundreds. If possible, enterprises must make one-click deployment available to all professional and citizen developers.
Secondly, once deployed, a model must be maintained to ensure it remains effective and does not, in the worstcase scenario, do harm to the business. MLOps is one way of controlling the cost of maintenance, by turning it into a systematised, centralised task.
The third area to consider for an efficiency overhaul is architecture changes, including changes to AI tools themselves. A scalable approach to architecture that allows for the adding of new users, including citizen developers is required to ensure that the Everyday AI culture will last. Cloudnative AI platforms are the answer here, especially those that can integrate easily into a hybrid workspace.