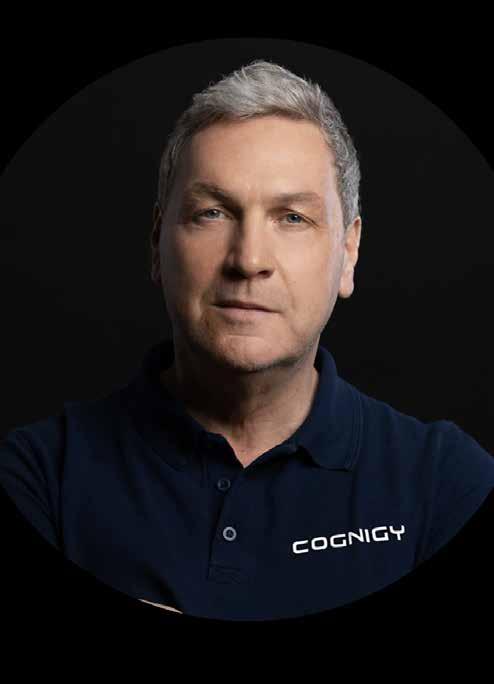
4 minute read
THE FUTURE CHANGING
from 50 CHANGE MAKERS
by cxoinsightme
BERND WUERMSEER, SVP GLOBAL SALES AT COGNIGY, EXPLAINS WHY CONVERSATIONAL AI IS A GAME CHANGER FOR DIGITAL TRANSFORMATION AND AUTOMATION.
What is the future of CAI?
Advertisement
Well, first I’d say the future is very bright. The market is growing rapidly because both the value and return on investment are huge and also continue to be proven over and over again. The two main components of CAI are the AI-powered natural language understanding and process automation and orchestration. Large Language Models will bring significant change to the language understanding side of things bringing in better understanding and generation capabilities, particularly in terms of personalisation. On the automation side, I think enterprises will realise that the same value that CAI delivers to customers can be leveraged internally as well and see growth there too.
What is driving the demand for CAI?
Most self-service experiences suck, and customers are becoming more impatient with the mediocre service they’re receiving. That’s a huge driver to be honest, and the technology is already here to help improve customer experience. Additionally, the orchestration and automation capabilities play a major role because it enables companies to deploy CAI on top of their existing tech stack, bringing dozens of siloed channels and systems together into a single well-oiled machine. Finally, the number of service channels continues to increase making it challenging for businesses to keep up and serve them all accurately and consistently. With CAI, companies can tackle all the above mentioned issues with a single platform.
What is the difference between CAI and chatbots?
Chatbots typically mean narrowly scripted question and answer bots found on websites. They don’t use artificial intelligence and are only able to deliver information such as FAQs or take customer information and relay that information to a marketing or sales process for follow-up. Essentially, chatbots give customers the ability to fill out a web form via chat. You can think of them as first-generation bots where the idea and vision were still in the development stage and the functionality was very limited.
CAI, as is in the name, uses artificial intelligence and natural language understanding to create a conversational interface to software, in the same way your mouse, keyboard and monitor provide an interface for you to interact with say Microsoft Word or Firefox. A key difference here is the idea of an interface, i.e., a road into key backend systems like your CRM, case management or say a reservations system.
Once you enable users to find information and carry out tasks and actions via language, you’ve got much more than a chatbot. Instead of calling a customer service agent who will navigate through multiple systems on their end and do what you need, the customer is suddenly able to do all of this themselves. So, CAI is the combination of AI as it pertains to language and process automation and orchestration which is far more powerful. It’s what enables Lufthansa for example to fully automate millions of conversations a year even on complex topics like flight refunds or rebooking instead of a classic chatbot that would just return a link to their policy or provide a phone number. It empowers customers to solve problems themselves, across dozens of channels.
How can enterprises use tools such as ChatGPT and GPT-3?
Right now, the business-ready use cases are limited. That’s not to say there aren’t plenty more great ideas that still need to be tested or further developed but putting something into the product is a much higher bar than letting people play unfiltered with ChatGPT. The first way to use large language models like GPT-3 is in building virtual agents. We’ve already built that into Cognigy and currently have an open beta program with many customers using it. Since LLMs are designed to generate languages, a conversation designer can use them to build chat flows in seconds, based only on a short description of what it should do or on a customer conversation. Additionally, they can quickly generate training data for intents and lexicons such as lists of city names or food items.
Second, on the customer-facing side of things, which is also something available now within Cognigy.AI, you can use GPT to rephrase standard responses into unique customer-specific ones by including the conversation history and context. That way you can ensure your business’s process is followed and the correct information is given, but it can be phrased in a personalised way for every single customer. It can also be used to extend natural language understanding capabilities by having it process customer inputs to improve the models understanding of what customers are writing or saying. If a virtual agent asked me how many people are flying and I say “Four people” then it’s straightforward. But if I say “That’s me, my wife and our two kids” it becomes much harder. That’s where GPT comes into play. So, it’s truly a better together story because CAI ensures consistency that your business processes and offers all the orchestration, automation and integration opportunities while GPT enables once unthinkable natural language understanding and generation to personalise every experience at scale.
What are some of the risks lurking behind ChatGPT?
Right now, the biggest risks come from customers directly accessing GPT-3 which opens up the possibilities of things like prompt injection attacks, hallucinations where the model confidently and convincingly gives false answers, as well as the risk of it saying hateful, discriminatory or other controversial things. To be clear, this is the exact same risk if you try ChatGPT directly which is why the current use cases are either on the backend or using GPT to augment processes but not take independent action. Another lesser discussed risk is cost on the customer-facing side. While LLMs offer some amazing advantages, every API call comes at a cost so when you’re thinking about scale, say millions of conversations a year, the additional cost of say rephrasing every single response rises quickly. So, it’s important not to be blinded by the advantages and carefully consider the situations where the added value is worth the added cost.