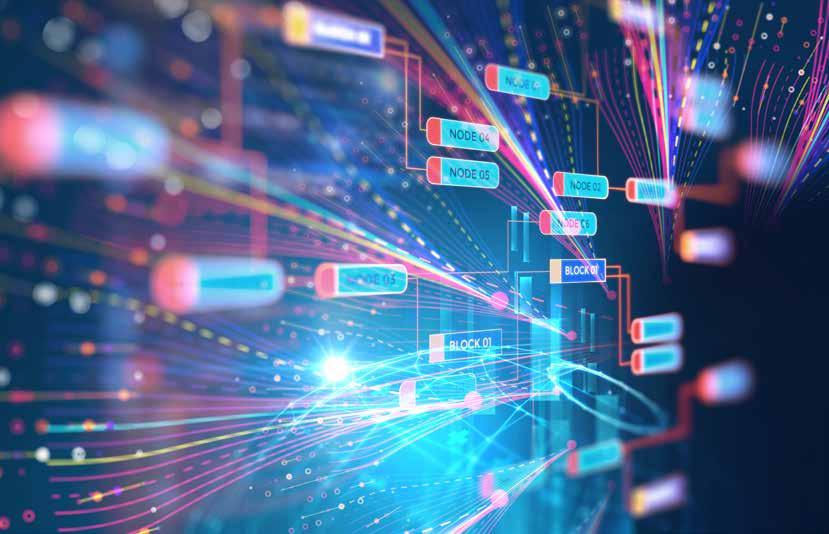
9 minute read
THE ART OF DATA SCIENCE
from AN OPEN APPROACH
by cxoinsightme
HOW DATA SCIENCE CAN POWER YOUR BUSINESS
For the uninitiated, data science is the process of gleaning business insights from structured and unstructured. It collects, analyses, and interprets large volumes of data, using various methods ranging from statistical analysis to machine learning, to improve business operations, reduce costs and enhance customer experience.
Advertisement
Though the term has been in use for decades, there is a sudden surge in demand for data science platforms as enterprises continue to amass enormous volumes of data in both structured and unstructured formats. This has created opportunities to transform data into value by gaining actionable insights into business challenges.
“The abundance of big data originating from web applications, mobile, and Internet of Things (IoT) has brought opportunities and challenges for business. Companies have the opportunity to get insights from this data to optimise processes, foster SAS, says the need to operationalise and realise the value of data science is now booming, underpinned by the need to manage and deploy models effectively. “Gartner reported that less than 40% of models created, with the intention of productionalising them, are ever put into production. Bain & Company reported that 70% of enterprises view analytics as a critical strategy, but less than 10% of enterprises are realising the benefits. Managing all models, no matter the language, in one place is key. This allows organisations to take advantage of automation to create repeatable deployment processes and monitor models once they’re in production to ensure the highest level of performance is maintained.”
innovation, and create new business opportunities,” says Hadj Batatia, Director of B.Sc. Data Sciences, Mathematical and Computer Sciences, Heriot-Watt University Dubai
He says the science behind working with data is becoming more accessible. Until recently, limited numbers of people graduating from some universities were able to master the needed mathematics, statistics, and computational models; but they were coming from different programmes, making knowledge sharing and cooperation difficult. Today, universities offer integrated data science programmes; various tools, platforms and technologies are available on the cloud. On-line courses ad onthe-job training are offered to re-skill employees. These factors lead to the democratisation of data science, with the aim of allowing companies of any size to benefit from this revolution.
Celal Kavuklu, Customer Advisory Director for Middle East and Africa at
What is the difference between data science and AI/ML?
Data science and AI are frequently used interchangeably. Data science is the discipline that aims at scaling machine

Dr. Hadj Batatia
learning to deal with big data in order to solve real business problems. This new and fast-developing discipline creates methods, tools and techniques for this purpose. Data science brings together mathematics, statistics, machine learning, and computer science.
“A way to understand the work of data scientists is to compare with software engineers who start from an information problem, design a solution, scale and manage projects, and develop and operate software. Data scientists also start from a business problem. They design data collection technologies and strategies, transform and analyze data, develop and validate data models, and integrate solutions within company information systems or industrial systems,” says Batatia.
Sid Bhatia, Regional Vice President & General Manager for Middle East & Turkey at Dataiku, adds: “When it comes to defining data science, which is frequently lumped together with machine learning, it is described as a field that uses processes, scientific methodologies, algorithms, and systems to gain knowledge and insights across structured and unstructured data. Moreover, data science definitions vary widely based on business function and role — different people across an organisation might have bespoke definitions for what makes good data science. It might be tangible business impact for data leaders, while for people like data scientists or engineers, it might be more detailed and nuanced — like the quality or accuracy of the mode.”
Kavuklu from SAS says data science uses AI, and most AI projects today rely on multiple data science technologies. “So we’re talking about two broad fields that have a lot in common, and it may be difficult to set clear boundaries between them.”
Top data science programming languages
A data science project involves a workflow of activities including data collection and management, data cleaning and preparation, data analysis and visualisation, data modelling and validation, and model integration and exploitation. Each of these stages requires different and complementary technologies and languages. The world of data science is awash with many programming languages, including PyTorch, TensorFlow, Python and R. Of these, the last two are more prevalent in data science projects.
“Both Python and R are suited for data science tasks — from data analysis and data wrangling all the way to model development and automation. Both languages are supported by large communities and are continuously extending their libraries and tools. While R is mainly used for statistical analysis, Python provides a more general approach to data wrangling and machine learning.
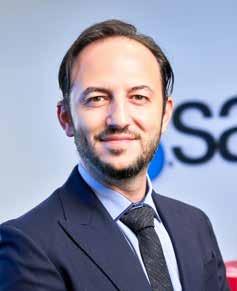
Celal Kavuklu Sid Bhatia
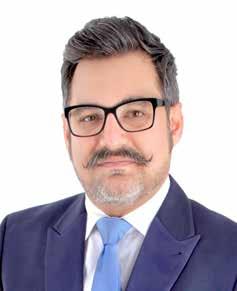
The choice of the language to use in a data science project depends on many factors, including the team’s familiarity with either technology and the use case to be implemented,” says Bhatia from Dataiku.
Batatia says data collection and management make use of NoSQL technologies with specific programming and query languages. Data cleaning and preparation can use simple spreadsheet tools when data has low volume. But when data is big, usually Python is used to write transformation pipelines. R is the best choice for statistical modelling when analysts want to uncover patterns and correlation. When developing predictive machine learning models, data scientists often resort to Python due to the large user community, the availability of libraries and frameworks, and the ease of development. System integration usually require more structured languages to enforce maintainability, reusability, interoperability, and other quality factors.
Kavuklu argues users should be allowed to choose their language of choice, the language they have spent years becoming experts and highly efficient in. “Being able to provide users with a technology that allows them to use a language of choice, or even dip between one and another will allow them to be as efficient and create the most performant data science models they can. A key part of this is allowing data scientists to collaborate and work in the way they prefer,” he sums up.
HOW TECHNOLOGY CAN TRANSFORM RECRUITMENT
GARRY TAYLOR, CHIEF TECHNOLOGY OFFICER, THE DATAFLOW GROUP, EXPLAINS HOW DISRUPTIVE TECHNOLOGY IS MITIGATING THE RISKS OF MAKING THE WRONG HIRES
Recruitment is an expensive process. And a risky one.
While recruiting the right talent should ultimately increase a company’s revenue generation, bad hiring practices are incredibly expensive. As economies around the world recover from the pandemic, many organisations are faced with the challenges of The Great Resignation, adding complexity and urgency to the already critical task of finding, engaging and employing the right people for the growing number of roles they need to fill.
In addition to the time it takes to advertise a role, review applications, interview applicants and onboard new hires, organisations incur expenses related to obtaining the relevant visa and work permit, medical insurance and training. Estimates of how much hiring the wrong person costs vary, but it could be as high as 30 per cent of the employee’s salary for the first year, if not more. In critical sectors such as healthcare, beyond the financial cost, risks of the wrong hire extend to patient care, or the lack of competent care, which could result in malpractice or even death. According to the
Patient Safety Movement, as many as three million people die globally each year as a result of medical errors. A likely contributing factor to this number is inadequately qualified or trained medical personnel.
To mitigate the risks associated with recruitment, especially in the healthcare sector, many governments mandate that practising professionals have licenses awarded which are dependent on credentials and experience. However, determining the accuracy and truthfulness of license applications is a time-consuming and often challenging process. Primary Source
Verification (PSV)—the process in which a third party, such as the DataFlow Group, handles license applications and verifies whether the information provided is true— is helping government bodies and employers find qualified and experienced talent faster and with greater confidence.
DataFlow has partnered with several government authorities, which leverage the organisation’s specialised PSV solutions to screen the credentials of healthcare professionals. Applicants upload their application for licenses and provide supporting documents, including education certificates and evidence of past employment, to an online portal. DataFlow then validates this information through its network of over 100,000 issuing authorities across more than 200 countries.
To further streamline this process, DataFlow launched TrueProfile.io, a career hub for healthcare professionals. Applicants using this platform only need to verify their data once rather than verifying their credentials every time they change jobs or relocate. Once an applicant’s details have been validated, their data is stored on the Ethereum blockchain, ensuring the verifications are accessible when required while also being completely secure and tamper-proof.
The benefits to applicants are clear; they need only complete the verification process once, saving them time and money for future applications. However, benefits extend to authorities who issue licenses and potential employers. They can be confident the data they are receiving is accurate and has been verified by a trusted partner. While increasing confidence in the applications they receive, this system also reduces the number of applications they will need to review. DataFlow only provides applications in which the applicant meets all of the required criteria, selected by its proprietary technology, so employers can be confident that applicants, at least on paper, are competent and capable of carrying out the role. DataFlow’s PSV and TrueProfile. io also pre-qualify applicants before they have entered the sector, meaning there is a verified talent pool ready to engage with when positions become available. This has the potential to reduce time-to-hire dramatically, further delivering cost savings.
Taking a broader view, if patients know their doctors, nurses and healthcare practitioners have been through this rigorous recruitment process in which all of their credentials and experience have been validated, driven by technology and an objective review, confidence in the healthcare sector increases and medical errors decrease.
Finding the right talent goes beyond just finding someone capable of doing the job—organisations are also looking for people who are a good cultural fit. Again, technology is being used to provide a more detailed picture of candidates, extending to analysing their online presence. DataFlow’s Digital Footprint Verification scans social media platforms, the web and the dark web to identify applicants’ online behaviour, even identifying and tracking pseudonyms. This background screening process protects hiring organisations from unnecessary exposure to risk, flagging violence, prejudices and extreme tendencies and generating a report that provides both historical and real-time data.
While PSV has not yet become fully automated, strides are being taken to eliminate the need for manual human input. While some countries still rely on paper-based records, many institutions are now digitising records, which will enable the seamless flow of information and the faster-automated verification of documents. Further down the line, artificial intelligence (AI) will play an even more prominent role in assessing an applicant’s suitability for a position. In essence, recruitment is based on asking the same questions and completing the same processes, so there is no reason why, given the advances in technology and quantum computing, AI-driven solutions would not be able to process these applications independently of human interaction.