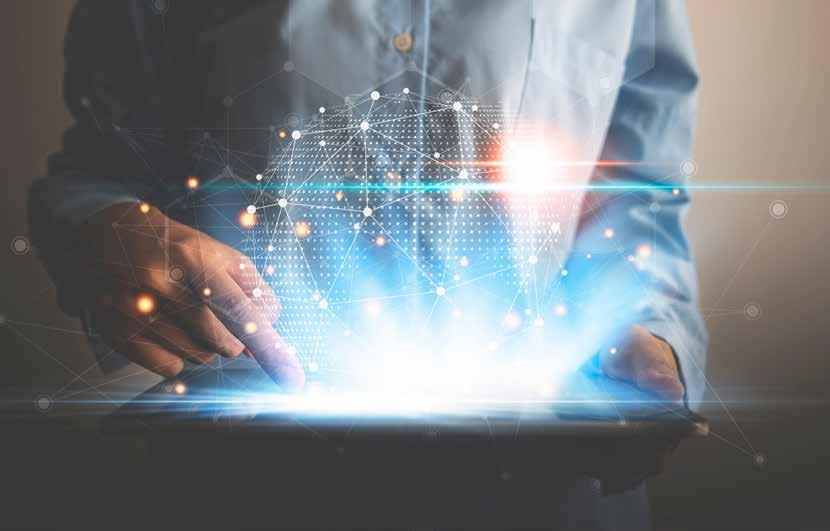
14 minute read
CRITICAL CAPABILITIES OF A MODERN DLP
from AN OPEN APPROACH
by cxoinsightme
SUNDARAM LAKSHMANAN, CTO AND HEAD OF ENGINEERING, SASE PRODUCTS AT LOOKOUT, EXPLAINS THE IMPORTANCE OF HAVING A MODERN DLP SOLUTION FOR DIGITAL ORGANISATIONS.
In some ways, IT teams had a great life in the early 2000s. Data was stored inside data centers and accessed through known ingress and egress points like a castle with a limited number of gates. As a result, organisations had control over exactly whom and what devices could access company data.
Advertisement
This is no longer the case. With users accessing cloud applications with whatever networks and devices are at their disposal, those defence mechanisms have become inadequate. To ensure their sensitive data is secure, organisations have to rethink their security model — including the way Data Loss Prevention (DLP) technology is implemented.
While DLP has been around for decades, it has reinvented itself in this remote-first environment which is why I think it is important to understand how modern DLP solutions, integrated into a cloud-delivered platform, can help organisations prevent data breaches, comply with regulations, while providing secure access to remote workers.
Why do organisations need a modern DLP solution?
Back when network architecture was centred around data centers, monitoring technologies like DLP existed on the edges of corporate perimeters or at the data exchange points. This worked because there were only a small number of apps and resources and organisations used relatively homogenous endpoints that were corporate-owned or managed.
About a decade ago, that castle-andmoat cybersecurity model started to break down. IT had to start accounting for other endpoints that didn’t use Windows such as MacOS, iOS and Android devices. It got even more complicated when corporate data migrated from corporate perimeters to private clouds and softwareas-a-service (SaaS) apps, where each of them had their own unique configurations and security measures.
Now that security requirements have turned inside out, with users, apps and data residing mostly outside data centers, DLP has to expand beyond the perimeter’s edge. And with data moving so quickly, simple user errors or misconfiguration that were once harmless can now cause serious harm to an organisation.
Differentiating between modern and traditional DLP solutions
One of the most important differences between a modern DLP solution and its traditional counterpart is its ability to understand both the content and the context of a data exchange, which enables an organisation to make smart access decisions that safeguards data without hindering productivity.
Know the risk levels of endpoints and users
With users and data no longer residing inside perimeters, the context by which data is accessed — such as who is accessing the data, their behavioural patterns and what risks are on the device they’re using — has become critical. In the spirit of Zero Trust, organisations shouldn’t provide any entity access until its risk level has been verified. But to do so efficiently, security teams must write policies that take into account the sensitive nature of the data as well as the risk level of the user and data.
A modern DLP has the insight to understand whether an account is compromised, or an insider threat based on a user’s behaviour, or the presence of risk apps on an endpoint. With those telemetry, it would be able to, for example, disable downloading privileges depending on whether the endpoint is managed or not or shut down access altogether if the user or endpoint is deemed high risk.
Identify, classify and encrypt data on the fly
In addition to context awareness, modern DLP solutions also have more advanced capabilities to identify and secure sensitive data. For example, an advanced DLP would have optical character recognition (OCR) and exact data match (EDM) to precisely identify data across any document type including image files, which is where data such as passport or credit card information is commonly found.
To ensure data doesn’t fall into the wrong hands, organisations also need integrated encryption capabilities to take automated actions. With integrated enterprise digital rights management (E-DRM) as part of a modern DLP, organisations can encrypt data when it moves outside sphere of influence, so that only authorised users have access.
Modern DLP is the key to data protection, compliance and productivity
Modern DLP enables organisations to set up countless remediation policies based on the merit of the context being accessed and the context by which the exchange occurs. This means DLP is critical both to the productivity of remote workers as well as data protection and staying compliant to regulations.
Protect data and remain compliant
Whether it’s sensitive intellectual property or data protected by regulatory requirements, organisations need to ensure that data is accessible but secure.
A modern, cloud-delivered DLP has the capabilities to efficiently identify the types of data you own across your entire organisation — in data centres, on private clouds or in SaaS apps. It can also enforce policies with varying degrees of granularity by using E-DRM and technologies such as Cloud Access Security Broker (CASB) or Zero Trust Network Access (ZTNA) to block intentional and unintentional insider threats and compromised accounts from leaking or stealing your data.
Empower Productivity
In theory, an organisations’ data would be secure if everything was locked down — but that would be detrimental to productivity. To tap into the full potential of cloud apps and mobile devices, organisations need to be able to make smart Zero Trust decisions.
By using DLP in conjunction with secure access solutions like CASB, ZTNA and endpoint security, you can give employees access to the data they need without introducing unnecessary risks to your organisation.
Modern Data Protection Requires an Integrated Approach
In today’s complex hybrid environment, data goes wherever it’s needed. This means organisations need the visibility and control they once had inside their perimeters. A modern DLP that is delivered from the cloud is central to this.
But one final thought — DLP shouldn’t be deployed in isolation. To truly secure data in a remote-first world, DLP needs to be integrated into a larger platform that can provide telemetry data about your users and endpoints and have the ability to enforce granular and consistent policies.

CUTTING THROUGH CHAOS
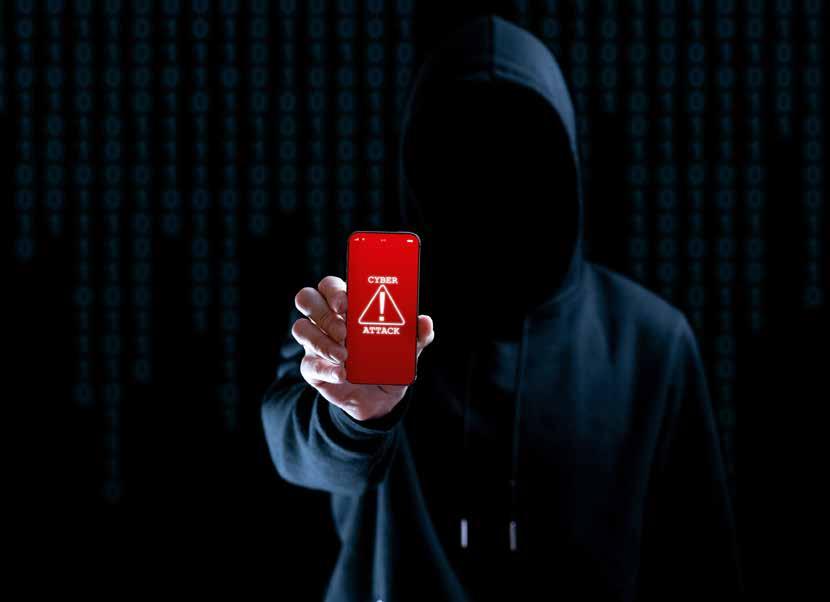
HADI JAAFARAWI, MANAGING DIRECTOR – MIDDLE EAST, QUALYS, WRITES CONTEXT XDR IS THE BEST RESPONSE YET TO THE MODERN THREAT LANDSCAPE
Regional cybersecurity chiefs have their hands full — they are understaffed and they face skills gaps. These are challenges that threat actors don’t face. And the increase in IT complexity, combined with many employees working from home on private networks with personal devices, means it has become a steep challenge to keep sensitive apps and data safe.
The Middle East and Africa cybersecurity market hit US$ 1.9 billion in 2020, and is projected to reach US$ 2.9 billion by 2026. The spending surge can be attributed to a staggering increase in cyber incidents, brought about by the stayat-home work trends that emerged from the pandemic. In late 2020, in the United Arab Emirates, the nation’s cybersecurity chief described a 250% year-on-year increase in attacks as a “cyber pandemic”.
Something must be done, and one of the most popular approaches to the much-desired, catch-all cybersecurity platform in the industry today is extended detection and response (XDR), a cloud-native solution capable of peering into every crevice in the technology stack, to detect and respond to incidents in real time.
Interpretations of the form
But as with many products in many industries, not all XDR is created equal. There are many interpretations of the form. Here, I will argue that only context-driven XDR can adequately support security analysts in their prioritisation of threats and the reduction of alert fatigue.
Because of the regional skills gap in digital security, teams need all the advantages they can get when it comes to identifying and mitigating threats. However, too often the alerts that prompt the hunt offer very little supporting information about the users, assets, and behaviors that
triggered the initial warning. Threat hunters need to know a range of things relating to operating systems, vulnerabilities, and the configuration of assets, as well as an initial assessment of how likely the attack is to succeed.
If the attack was already successful, where in the MITRE ATT&CK (Adversarial Tactics, Techniques, and Common Knowledge) framework did it fall? If it is ongoing, can it be mitigated by automation, or a junior analyst, or will it take a team of internal or external experts to address? Whether successful or not, it helps analysts to have a rich journal of events leading to the flagged event, including a post-event analysis of business impact.
A pyramid of needs
Compiling all this information in a timely manner is one of the key challenges for the region’s beleaguered security professionals. Incomplete information from multiple sources can leave analysts struggling to understand their organisation’s risk exposure and asset criticality. Travelling from dashboard to dashboard, they will try their best with what they have to hand, but the time they spend chasing false positives is time spent away from more productive activities, such as addressing genuine threats that pose real risk.
The modern SOC has three fundamental needs when it comes to threat assessment. The first is immediacy, where responses can occur at scale in real time. The second, criticality, calls for the understanding of impacts and potential impacts, for the purposes of prioritisation. And third is response, which represents the means to take effective action, such as killing processes and quarantining files.
To deliver on this pyramid of needs, XDR solutions must break down security data silos to deliver a unified view of the enterprise technology stack and the threats it faces. Effective XDR should bring the tapestry of security solutions and functions together in a single platform. In doing so, contextoriented XDR can help to dial out the white noise that varied telemetry creates and present a real-time view for the user of the business impact of a given alert. Context, in short, leads to more effective response.
Many tentacles
Context XDR brings together available information on risk posture, asset criticality, and the threats themselves to deliver a clearer picture. It leverages comprehensive vulnerability and exploit insights for a threatened asset’s OS and for third-party apps. Insights must include misconfigurations and end-of-life (EOL) flags. This uninterrupted vulnerability mapping will provide a more complete picture of the organisation’s risk posture than simple risk-scoring based on how OS-patch statuses relate to common vulnerabilities.
Active asset discovery is vital in context XDR. Policy-driven criticality assignments can evolve with an asset’s current state more easily if information on the asset is up to date. The right security and business context can help security teams to prioritise, say, an executive’s laptop or a database server that stores sensitive intellectual property.
Everything XDR hopes to accomplish hinges on the quality and availability of the right data at the right time. This is not only true of assets, but of the potential attacks themselves. Threat intelligence on current exploits and attack methods can deliver the actionable insights that can help security teams prevent and mitigate the perils beyond the digital gates. Where possible, XDR solutions should look to data from third-party solutions within the technology stack and combine it with asset risk posture, criticality, and direct threat intelligence to create even higher fidelity in alerts.
The future is context XDR — a fullfledged, many-tentacled sentinel with access to every surface and crack in the digital estate. Threat actors may have us outgunned, but with context XDR in our arsenal, the advantage will finally be ours.
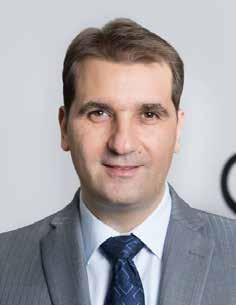
DEBUNKING MLOPS MYTHS
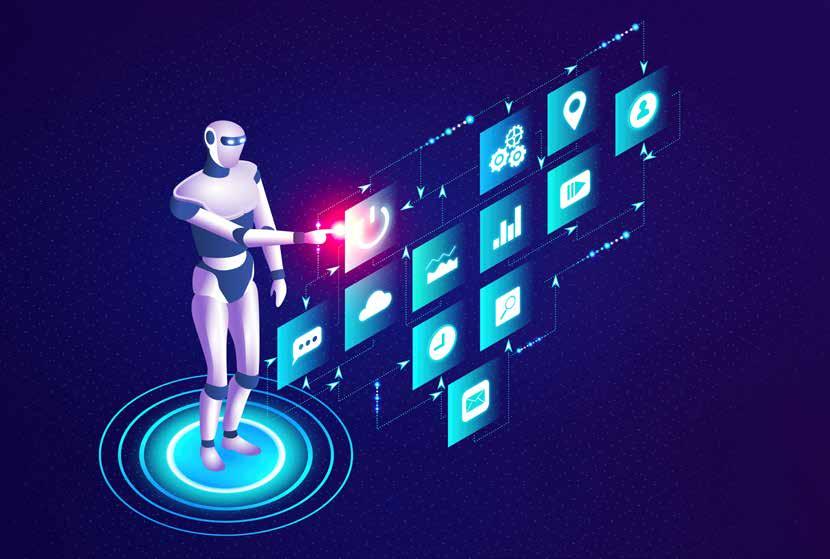
JAD KHALIFE, SALES ENGINEERING DIRECTOR – MIDDLE EAST, DATAIKU, ON SEVEN COMMON MACHINE LEARNING OPERATIONS MYTHS WE SIMPLY MUST BUST.
Machines that learn have always been fascinating. But the graduation of machine learning from frontier gimmick to mainstream tool is now complete. The region’s cloud migration seems likely to generate more and more interest in ML. And in an age where businesses increasingly recognise the need for formal workflows and best practices across the IT function, machine-learning operations (MLOps) will play a fundamental role in delivering actionable intelligence to stakeholders.
There is a lot of confusion and misunderstanding around MLOps.
Standardisation takes time, but for now, we can think of it as a family of best practices geared towards the efficient and rewarding deployment and maintenance of machinelearning models into production environments.
Underneath every misinterpretation of MLOps lie a host of miscalculations driven by memes. So let’s examine the seven main myths that surround MLOps and often lead to disappointment in its implementation.
1The model is what matters
Organisations tend to obsess over the model itself as being the primary, or even the sole, deliverable of an AI-based data project. But modeling is just part of the journey. The vast majority of a project team’s time will be absorbed by data preparation. Tasks include configuration, data collection, feature extraction, data verification, and the selection of analysis tools and process-management tools, as well as infrastructure and resource considerations.
2The design environment mirrors the real world
A common point of failure for MLOps is the misconception that design environments are (or can be) carbon copies of production environments. Pipelines need to function in both, but often this oversight can break the model as it exits the gate. A design team’s focus on performance at the expense of portability must be tempered, so deployment is streamlined to include all artifacts required in each operating environment.
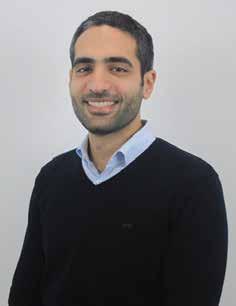
3Model lifecycles are straight lines
Some MLOps teams treat models with a fire-and-forget approach, but MLOps is not about initial deployment; it is more about maintaining models over time. The design process may only take weeks, but the model will most likely run for years. If its value-add is to remain strong, then the relevance of its insights must be maintained. So, do not expect models to operate in the long term without nurturing. Some organisations may want to build environments where models have multiple versions that can be swapped in and out cleanly, as needed.
4Accuracy is the number-one metric
In this case, the temptation to believe the myth is obvious. Doesn’t ROI naturally emanate from an accurate model? To an extent, the answer is “yes”, but MLOps has many other important metrics. For example, pipeline, service health and data-drift detection are arguably more important in the long run. The data drift — the measurable variation of a dataset over time — between, say, training and production data can be an important indicator of future performance, and so may deserve more attention than current accuracy levels.
5Fixes can be ad hoc
Taking a “cross that bridge when we come to it” attitude to broken models implies that in MLOps, model breakdowns are rare. They are not. From data drift to changes in upstream systems, there are many things that can disrupt a model. So, the break-fix lifecycle for MLOps requires a plan. Organisations must have baseline models that can serve as fallbacks, along with predetermined workflows to introduce backups without disrupting downstream services. And audit trails, event logs, and monitoring data from other production systems must be in place to accelerate the fix time.
6Production teams don’t need to understand ML models
Models cannot just be deployed without contextual knowledge of their design and proposed operation. If MLOps teams try to work this way, decisions by the deployment team that seem technically sound in isolation could induce anomalies in model behavior and even lead to unwelcome biases in the system. Production teams’ understanding of the underlying behavior and expectations of a model will better equip them in fixing issues and deploying models that behave as expected. For this reason alone, it is recommended that MLOps teams have access to tools that automatically produce rich model documentation.
7With MLOps, AI governance is redundant
MLOps is not a part of AI governance. Yes, the two are related, but they cover many functions that do not overlap, and have entirely different outlooks and priorities when it comes to systems, data, and roles. Both MLOps and AI governance oversee the operation of projects in production environments. But where AI governance is concerned with managing risk and ensuring compliance, MLOps looks after the systems and processes of a digital business, optimising value and uptime. For example, while an AI governance team will use audit trails for assuring senior executives and regulators that the organisation is dotting its Is and crossing its Ts, MLOps will use such data for troubleshooting.
Knowledge and action
MLOps can certainly deliver value to an organisation that has the capacity and will to become a digital business and can arm its decision-makers with all the real-time actionable insights this implies. But the myths must be overcome.
Stakeholders must remember that MLOps is about more than models and relies on strong data integrity and agile infrastructure. Project leaders must run a tight ship on documentation and cross-team information sharing. And they must never forget to operate AI governance and MLOps separately while ensuring they cooperate on scaling AI. For those that bust the myths and get it right, MLOps will add immense value and form the foundation of an enviable powerhouse of knowledge and action.