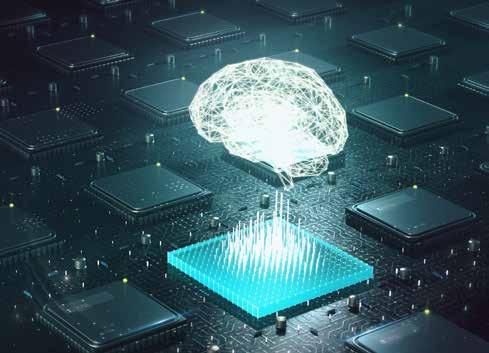
5 minute read
THE RISE OF MACHINE LEARNING
ENTERPRISES ACROSS VIRTUALLY EVERY INDUSTRY ARE ADOPTING MACHINE LEARNING SYSTEMS. HERE IS WHAT YOU CAN DO WITH ML TODAY.
Leveraging AI and machine learning to better use data and improve decision-making has become a strategic priority for enterprises in the Middle East looking to remain competitive in the post-Covid world.
Advertisement
Though the concept of machine learning is not new, digital businesses are adopting this disruptive technology driven by the availability of sensor data and cloud-based platforms. Gartner says
ML has become so pervasive that most of us use it daily without even noticing.
The research firm says whether you are searching the web or using speech recognition on a smartphone, you are most like benefiting from ML.
“With the developments being made in machine learning, the practical uses of the technology are adding up to be endless. One of the unique features of ML is that it is highly versatile, as ML models can be deployed across a variety of industries. They are especially useful in situations where quickly analyzing numerous amounts of data to predict outcomes is key,” says Ahmed Eid, Director – Presales, META, Dell Technologies.
In the future, machine learning systems will be further enhanced to help anticipate trends and identify problems, thereby playing an important role in supporting decision-making processes across various industries.
“We can also expect the capabilities of machine learning to significantly increase due to quantum computing, where ML will acquire the capability to create systems that execute multi-state operations simultaneously, thus tackling complex issues in a split second,” says Eid.
By establishing human-machine partnerships, businesses will be primed to improve productivity and employee and customer satisfaction. Consequently, they will be able to focus on what matters and explore new valuegeneration opportunities.
“Machine Learning (ML) is one of the most versatile and powerful technologies of our time and one that is creating huge strides. Amazon, Netflix, Facebook, and more leading companies have aspects of their businesses or the main product heavily using ML techniques,” says Talal Shaikh, Associate Professor, Director of Undergraduate Studies for the School of Mathematical and Computer Sciences, Heriot-Watt University Dubai.
Quantum Computing and Automated Machine Learning (AutoML) are among the future trends of machine learning. Quantum Computing has the potential to boost machine learning capabilities through faster data processing and performance enhancement. It can improve data analysis and develop profound insights, enabling companies
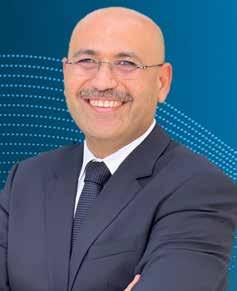
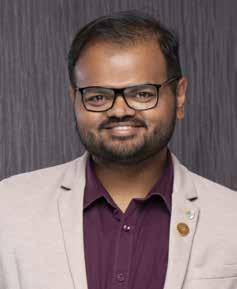
Talal Shaikh
to get better and faster results than traditional methods. Currently, no commercially ready quantum computing is available, but with tech companies investing in this technology, we should expect its rise soon.
“Another rising trend is automated machine learning or AutoML, which can simplify complex machine learning models and techniques, allowing wider use even by individuals with little experience in developing AI models. The technology can allow data preprocessing, algorithm selection, feature engineering and more,” says Shaikh.
Almost every industry is powered by ML, including healthcare, manufacturing, and automotive. In fact, the global machine learning market is predicted to grow from $8.43 billion in 2019 to $117.19 billion by 2027.
“Overall, AI and ML can automate repetitive tasks and enable humans to deliver maximum impact in their fields of work. In healthcare, there has been an acute shortage of healthcare personnel in most geographies. ML can help fill this gap by providing insights from smart devices. For example, it can infer results from tests, such as ECGs, without requiring a medical specialist to interpret them. ML can also help develop vaccines and drugs faster than traditional techniques,” says Ramprakash Ramamoorthy, director of AI research at ManageEngine.
He adds the manufacturing industry can use ML techniques like computer vision for better quality control of finished goods. It can also help reduce workplace hazards for the personnel on the manufacturing floor and increase efficiency, and decrease costs.
In the automotive sector, ML can help build fail-proof components, thereby reducing the cost of ownership. In addition, AI can ensure greater vehicle safety. Techniques like advanced driverassistance systems are increasingly using AI to provide safer commutes. ML can identify driver safety issues, such as those related to drowsiness, by constantly monitoring driving parameters.
Benefits of ML in the cloud
Major cloud providers have built ML platforms to support the complete lifecycle – from planning to production, which can be quite resource-intensive if you do it on-prem.
“Today, most machine learning (ML) and AI workloads depend on cloud data centers’ massive computational, processing, analytics, volume storage, memory, and graphic processing capabilities. It is generally agreed that the cloud is a cost-efficient platform to host ML and deep learning (DL) workloads without investing in expensive hardware. With cloud, it is also easy to scale up, and therefore organizations just starting-out or experimenting with ML can easily test and deploy smaller projects,” says Eid from Dell.
Additionally, organizations typically turn to cloud service providers (CSP’s) for ML and AI workloads because they have a reservoir of development tools and other resources readily available such as pre-trained deep neural networks for voice, text, image, and translation processing. Moreover, for organizations without an expert staff for AI projects, these platforms build DL neural networks automatically, saving weeks or sometimes months of labor, he adds.
Sid Bhatia, Regional Vice President & General Manager, Middle East & Turkey, Dataiku, says in traditional IT contexts, the risk and cost of lock-in are critical in assessing and adopting new technologies. In the age of Enterprise AI, this phenomenon is magnified exponentially as AI/ML technologies come and go with no sign of a reduction in the pace of innovation. In addition, the shift to cloud providers continues to create interdependencies between different components of the full AI stack. This necessarily means that companies have to become more elastic, able to adapt and drop technologies as they arise.
“It also means that in order to provide an experience for end users who are interacting with data and AI systems that is unchanging despite underlying architecture, organizations need to have a layer that sits on top of existing systems. The elastic scale of the cloud is an enabler and accelerator for AI/ML adoption,” he sums up.
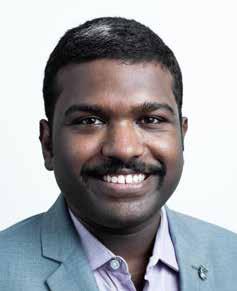
Ramprakash Ramamoorthy Sid Bhatia
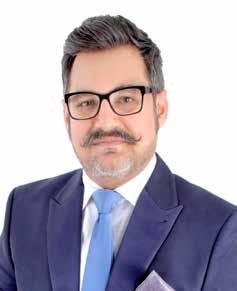