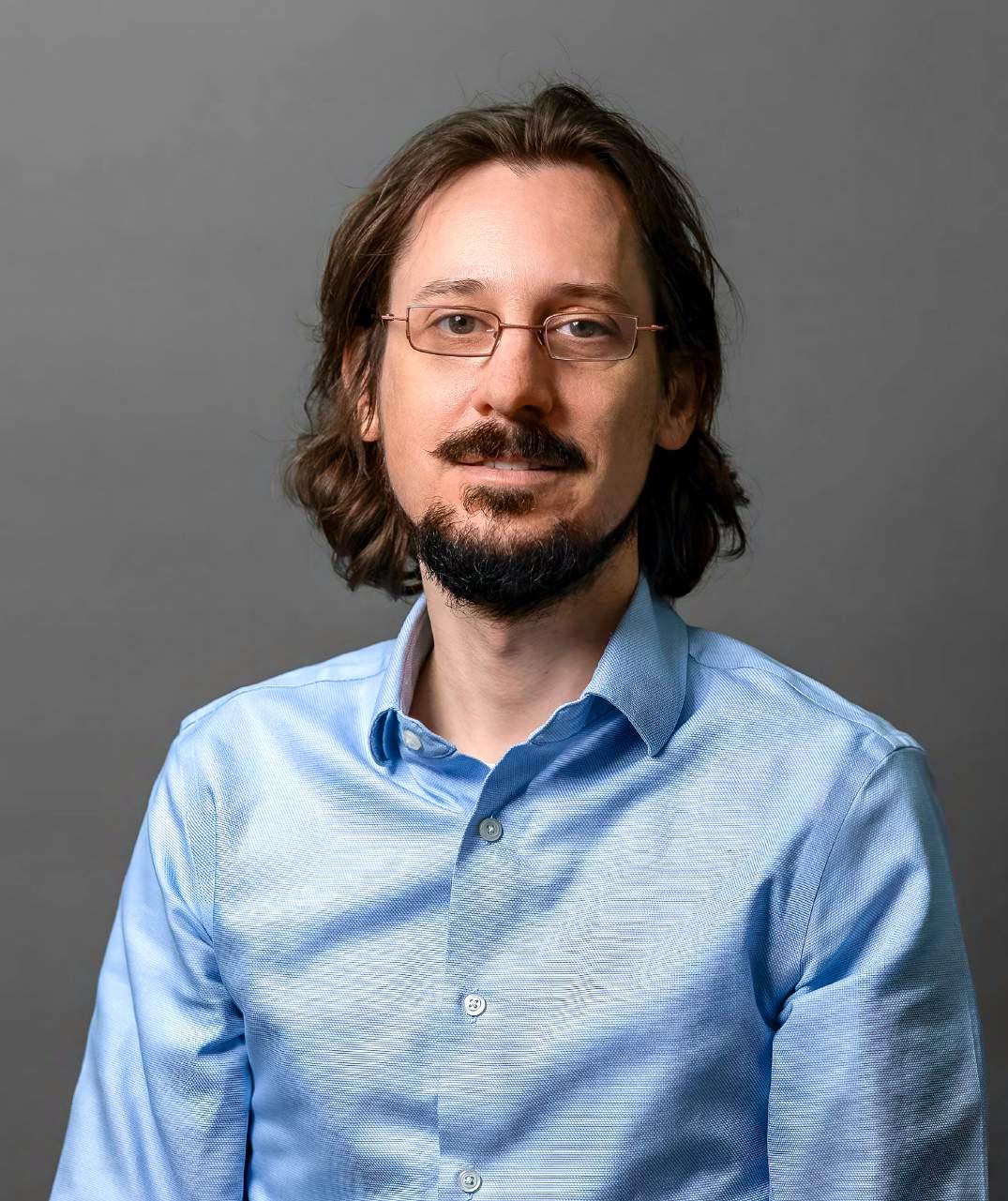
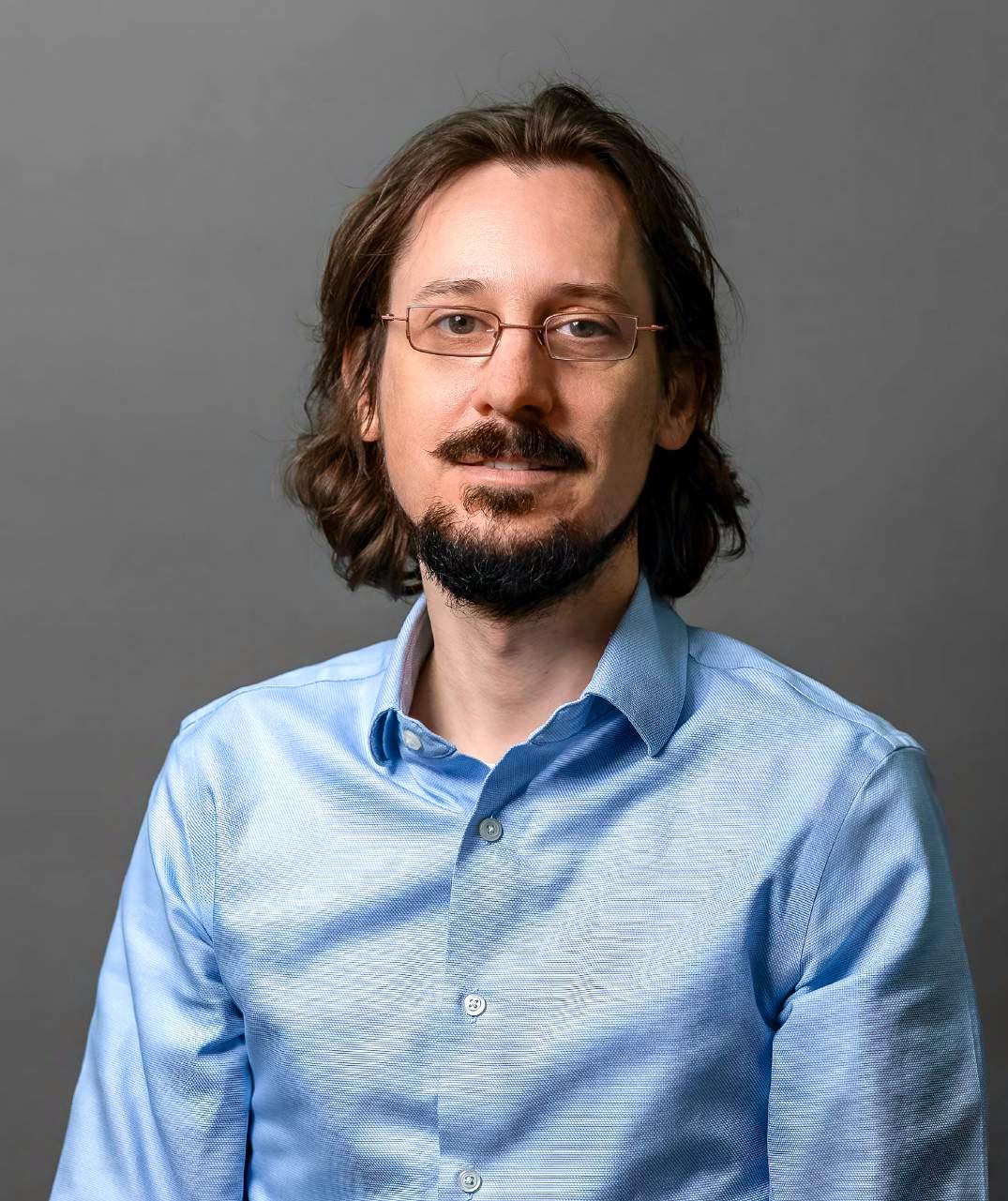
GABRIEL STRAUB
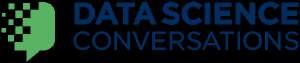
THE SMARTEST MINDS IN DATA SCIENCE & AI
Expect smart thinking and insights from leaders and academics in data science and AI as they explore how their research can scale into broader industry applications.
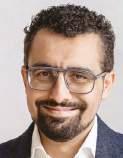
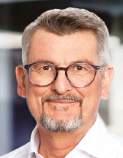
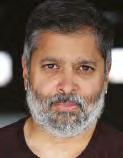
Key Principles for Scaling AI in Enterprise: Leadership Lessons with Walid Mehanna
Maximising the Impact of your Data and AI Consulting Projects by Christoph Sporleder
How AI is Reshaping Startup Dynamics and VC Strategies by KP Reddy
Helping you to expand your knowledge and enhance your career.
Hear the latest podcast over on
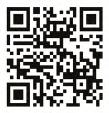
KP REDDY
WALID MEHANNA
CHRISTOPH SPORLEDER
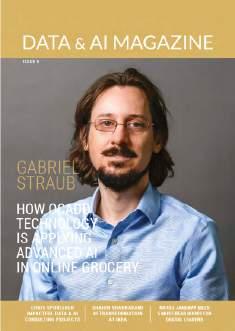
CONTRIBUTORS
Gabriel Straub
Christoph Sporleder
Shahin Shahkarami
Guillaume Desachy
Ben Taylor
Nicole Janeway Bills
Francesco Gadaleta
Jhanani Ramesh
Rohan Sharma
Gustavo Polleti
Anthony Alcaraz
Philipp Diesinger
Gabriell Fritsche-Máté
Andreas Thomik
Stefan Stefanov
Martin Musiol
Charmaine Baker
Glenn Hapgood
EDITOR
Damien Deighan
DESIGN
Imtiaz Deighan imtiaz@datasciencetalent.co.uk
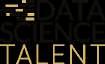
Data & AI Magazine is published quarterly by Data Science Talent Ltd, Whitebridge Estate, Whitebridge Lane, Stone, Staffordshire, ST15 8LQ, UK. Access a digital copy of the magazine at datasciencetalent.co.uk/media.
DISCLAIMER
The views and content expressed in Data & AI Magazine reflect the opinions of the author(s) and do not necessarily reflect the views of the magazine, Data Science Talent Ltd, or its staff. All published material is done so in good faith. All rights reserved, product, logo, brands and any other trademarks featured within Data & AI Magazine are the property of their respective trademark holders. No part of this publication may be reproduced, stored in a retrieval system, or transmitted in any form by means of mechanical, electronic, photocopying, recording or otherwise without prior written permission. Data Science Talent Ltd cannot guarantee and accepts no liability for any loss or damage of any kind caused by this magazine for the accuracy of claims made by the advertisers.
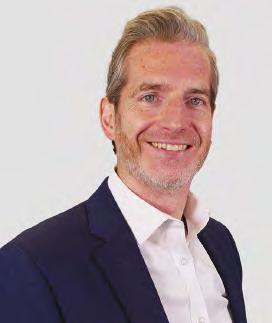
WELCOME TO ISSUE 9 OF DATA & AI MAGAZINE
YOUR QUARTERLY INTO THE EVOLVING WORLD OF ENTERPRISE DATA SCIENCE AND ARTIFICIAL INTELLIGENCE
IN THIS ISSUE
Our articles discuss both the challenges and innovations within the data and AI space, highlighted by our cover story featuring Gabriel Straub of Ocado Technology. Gabriel provides an in-depth look at why advanced technology and AI are pivotal for the future of online grocery shopping. His insights show how AI continues to reshape industries far and wide.
Alongside our cover story, we feature a diverse lineup of thoughtprovoking articles. Chris Sporleder from Rewire discusses the impact of strategic data and AI consulting on transforming businesses. Shahin Shahkarami at IKEA explores the company’s journey through AI transformation, emphasising practical applications in retail.
For those looking to deepen their leadership and knowledge base, Nicole Janeway Bills offers a curated list of five essential reads for digital leaders, empowering our readers with the tools to lead in a digitised economy.
On the governance front, Rohan Sharma provides a practical guide for investors navigating the complex landscape of AI governance, a mustread for stakeholders aiming to balance innovation with responsibility. Meanwhile, Martin Musiol engages us with a conversation about the evolution of generative AI – from generative adversarial networks (GANs) to multi-agent systems – offering a glimpse into the future of AI technology.
Each article, while unique in its focus, threads a common narrative about the transformative power of AI and the critical need for an informed approach to technology adoption and integration in various sectors.
AN ESSENTIAL ENTERPRISE AI EVENT: REWIRE LIVE (FRANKFURT)
Thursday 10th April 2025 – Dealing with AI’s Last Mile Problem
We are thrilled to co-promote an event with Rewire, designed to connect industry leaders, innovators, and thinkers in the field of data & AI. This must-attend gathering promises to be at the forefront of solving AI’s last mile problem. It will be an invaluable opportunity to network, share ideas, and learn from some of the brightest minds in the industry.
Rewire LIVE 2025 is set to be a pivotal day for corporate executives and business leaders who want to address critical aspects of AI implementation in the corporate sphere. Focusing particularly on ‘the last mile’ of driving scale and adoption to truly harness the transformative potential of data & AI.
Why attend?
The landscape of data & AI is fast-evolving, and despite the substantial promise it holds, the actual deployment and scaling of AI within organisations presents numerous challenges. From technical integration hurdles to ethical and regulatory considerations, the path to effective AI implementation is complex.
Rewire LIVE aims to cut through the AI hype to provide clear guidance on these challenges, offering a mix of technical expertise and strategic guidance from a panel of distinguished speakers and experts from both corporate and academic backgrounds.
Who should attend?
This event is specifically designed for senior executives: CEOs, CTOs, heads of departments, and directors across technology, strategy, analytics, and data-intensive sectors like supply chain and marketing. There will be the chance to network with peers, gain insights from industry leaders, and bring back knowledge that can shape your organisation’s future.
Registration is simple:
Visit Rewire LIVE 2025 registration to express your interest.
Our events team will review your application and confirm your place. Given the strategic importance of this event, ensuring your seat is crucial for those who wish to lead in the AI-driven corporate world.
Thank you for your continued engagement with Data & AI Magazine. We hope this issue enlightens and inspires you, and we look forward to meeting you in Frankfurt for what promises to be a pivotal gathering.
Damien Deighan Editor
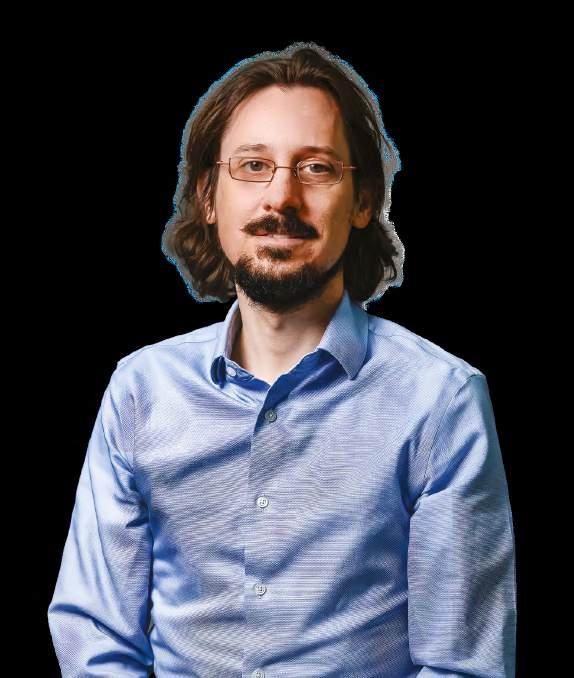
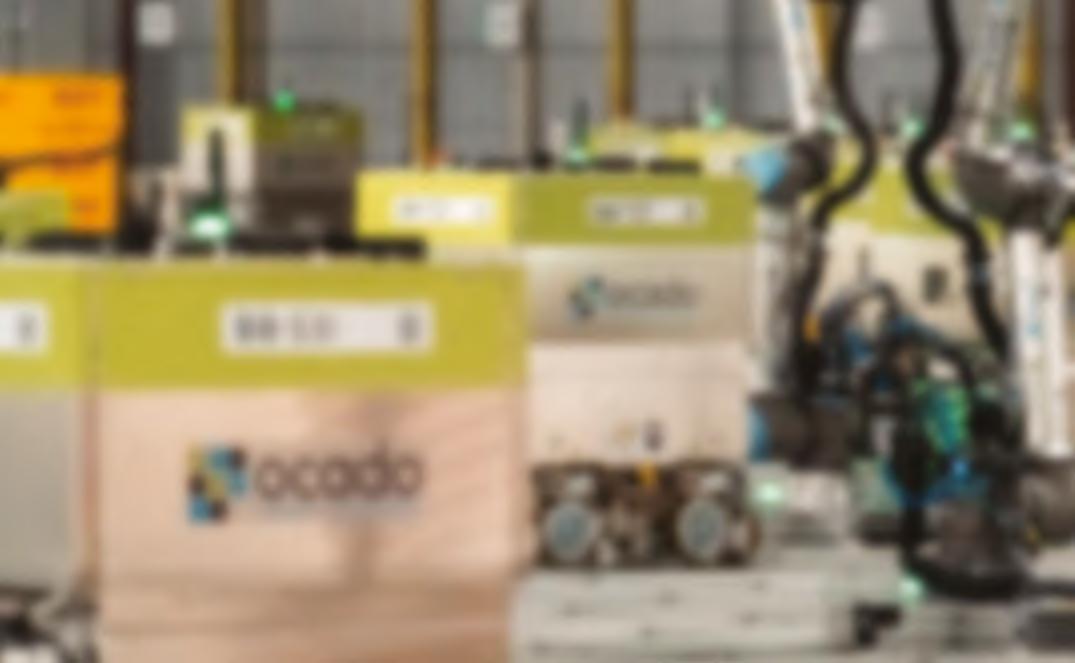
WHY ONLINE GROCERY NEEDS ADVANCED TECHNOLOGY
When Open AI launched Chat GPT in November 2022, artificial intelligence suddenly shot to the top of the agenda in every boardroom. LinkedIn was flooded with hot takes about the hundreds of ways that AI would change the way we live, work and interact. Many media outlets portrayed AI as a brand-new technology that had come out of nowhere and taken us all by surprise.
But in the online grocery space, AI isn’t as new as you may think. In fact, at Ocado Technology, it’s a crucial element of the e-commerce, automation and logistics technology that we develop and deploy for retailers all over the world. Shoppers have been experiencing the benefits of AI working behind the scenes for many years without even realising.
GABRIEL STRAUB joined Ocado Technology in 2020, bringing over 10 years’ experience in leading data teams and helping organisations realise the value of their data. At Ocado Technology, his role as Chief Data Officer is to help the organisation take advantage of data and machine learning so that we can best serve our retail partners and their customers.
Gabriel is also a guest lecturer at London Business School and an Independent Commissioner at the UK’s Geospatial Commission. He has advised startups and VCs on data and machine learning strategies. Before Ocado, Gabriel held senior data roles at the BBC, Not On The High Street and Tesco.
Gabriel was named AI Expert of the Year by Digital Leaders and one of the top people in data by both DataIQ and HotTopics. Gabriel has an MA in Mathematics from Cambridge and an MBA from London Business School.
So why the hype now?
Wide-scale and free access to generative AI tools such as ChatGPT is definitely an exciting development. If your job involves content creation or processing large amounts of documents, I’m sure you will have experienced a change in how you do things.
But for the online grocery space, generative AI is just one tool in our toolbox. We use a vast array of AI techniques to solve business problems for our clients. From deep reinforcement learning to train robot arms to pick and pack thousands of different items, to optimisation algorithms which can make sure deliveries arrive on time using the fewest number of vans.
Wait, why do you need AI and robotics to deliver my tomatoes?
When Ocado was founded, many believed that the vision of building a feasible online grocery service was an almost unsolvable challenge because of its complexity.
Handling tens of thousands of orders that contain over 50 items every day is complex enough as it stands. When you combine this with the fact that the products need to be in and out of the warehouse very fast for freshness, and you need to arrive perfectly on time so that your customer can refrigerate their groceries, the logistics challenge gets bigger and bigger.
Without getting smart with automation, data and AI early on, we were never going to achieve a viable model. We quickly found that technology from companies who specialise in the less complex general merchandise space could not be repurposed for the online grocery use case. The tech simply couldn’t handle the need for managing different temperature regimes and perishable goods. There was no template for what we wanted, and no off-the-shelf product that would help us, so we built the technology ourselves.
A technology-powered online grocery operation creates a lot of data. Shoppers log millions of data points as they interact with the webshop, and every shipment that comes in from the supply chain, every movement of goods around the warehouse, and every move our logistics vehicles make create flows of valuable information.
they can offer a much wider range of products to their customers. For example, Ocado.com in the UK has a range of around 50,000 items. This means, we need to make it simple to navigate that large range for customers. We don’t want shoppers to ‘get lost’ in the virtual aisles.
On average, 70% of shoppers abandon their shop after adding products to their baskets (source: baymard.com/lists/cart-abandonment-rate). AI is critical to overcoming the friction that leads shoppers to abandon. We need to make their lives easy.
For example, many shoppers use our AI-based ‘instant shop’ that automatically populates a whole order with items based on their data and history. It means a shopper can go from login to checkout in just a few clicks.
We also use AI to inspire shoppers with ML features that recreate the fun of spontaneously grabbing something new in the supermarket with personalised recommendations.
An example of this is flash sales, where AI technology triggers price drop sales of products with a risk of purge advertised to shoppers. This not only helps us reduce waste, but helps inspire our customers to try (and continue with!) new products. It turns out new-to-you products bought through flash sales have a significant chance of being repurchased in following shops so clearly it’s a proposition that shoppers benefit from for inspiration.
We also use AI in our search function, where our models are designed to improve the accuracy of matching, which massively increases the regularity of preferred brands and products appearing at the top of customers’ search results. AI models can suggest search terms that closely align with a customer’s search history, enabling them to find what they’re looking for without needing to type a letter.
Thanks to AI, every single customer has a different experience and interacts with a unique storefront tailored to their needs.
Thanks to AI, every single customer has a different experience and interacts with a unique storefront tailored to their needs.
We use artificial intelligence to find patterns, insights and optimisations in this data that allow us to improve the customer experience and make efficiency gains.
How does AI help shoppers fill baskets quickly and inspire customers to try new products?
Using large centralised fulfilment centres that store products in giant grid structures, means that the retailers we work with don’t have the same capacity constraints that a supermarket store has. This means
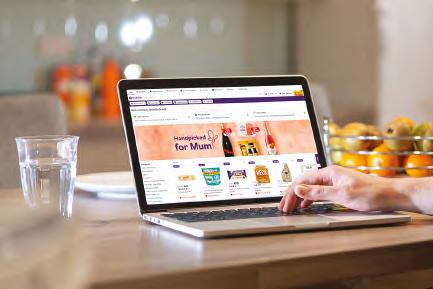
What other factors aside from the unique storefront play an important part in the customer experience?
Customers want their shop to arrive in full, at the right time and all for a competitive price. One of the consumer frustrations with online grocery shopping is that when their order arrives, the retailer has made substitutions that they are not happy with.
For us, this isn’t an acceptable customer experience. We set up data flows through our platform so that we know exactly what’s in our warehouse, when new stock will be arriving, and which supplier orders can still be modified. That means we only show customers products on the webshop that will be in stock at the time that their order is prepared so we rarely need to make substitutions.
But we can only offer a top-level service if the model is operationally efficient. AI plays a crucial role in eliminating waste and driving down cost.
Can you explain how AI allows retailers to order exactly the right amount of stock?
Deep learning models are able to forecast what customers will buy. Retailers have found that our deep learning models are up to 50% more accurate than their traditional forecasting systems. The AI recommends the optimal amount of stock to buy from suppliers to guarantee availability of products for shoppers without creating waste from unsold products. For our partners using our supply chain AI, over 95% of stock is ordered automatically without the need for any manual intervention.
The models benefit from the latest developments in transformer models, which are at the heart of the current Iarge language model and generative AI phenomenon. This innovation means that in the UK, only around 0.7% of stock we order goes unsold compared to an industry average of 2-3%.
But once we have inbounded our stock from the supply chain, we need to get it in and out of the warehouse in the most efficient manner.
Can you talk about how Ocado’s AI enables highly efficient picking and packing of orders?
In our automated customer fulfilment centres that we provide for retailers worldwide, hundreds of retrieval robots whizz around giant storage grids to fetch containers containing the items that people have ordered.
These ‘bots’ are orchestrated by an AI ‘air traffic control’, which controls every move the bots make. AI helps make decisions like: which stock storage box to use for the next order, how to efficiently path the bots around each other, and where to put boxes down once we’ve finished picking from them.
Compared to a typical manual warehouse, the storage and retrieval bot system and advanced automation
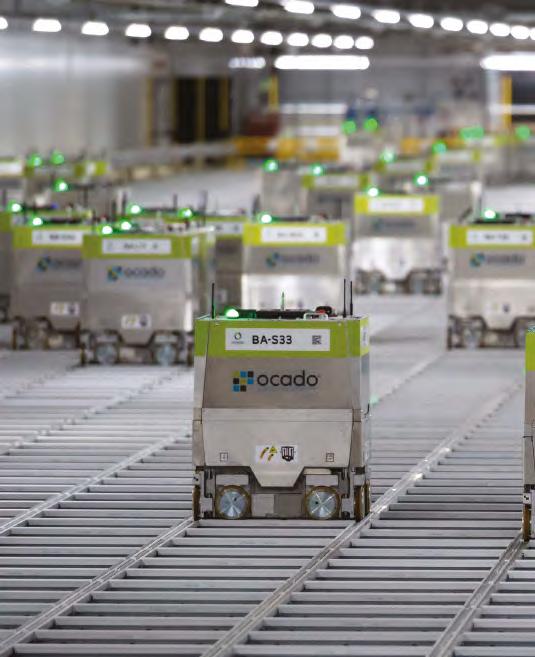
Compared to a typical manual warehouse, the storage and retrieval bot system and advanced automation reduces the end-to-end labour time to complete one order from over an hour to under 10 mins.
reduces the end-to-end labour time to complete one order from over an hour to under 10 mins.
What role does computer vision play in improving your engineering operations?
Our fleets of bots travel tens of millions of km every year, and occasional mechanical wear can mean there is a failure. AI plays a crucial role in minimising the impact. If a bot ceases to provide updates to the air traffic control system, we use computer vision on the 360-degree fisheye cameras above the grid to locate the bot for maintenance attention. This makes our engineering operations more efficient.
Can you give an overview of how Ocado’s robotic packing of shopping bags works without the need for human touch?
The bots deposit stock next to robot arms to be picked into customer bags. Our retail partners can stock up to 50,000 different products each. Robot arms need to be
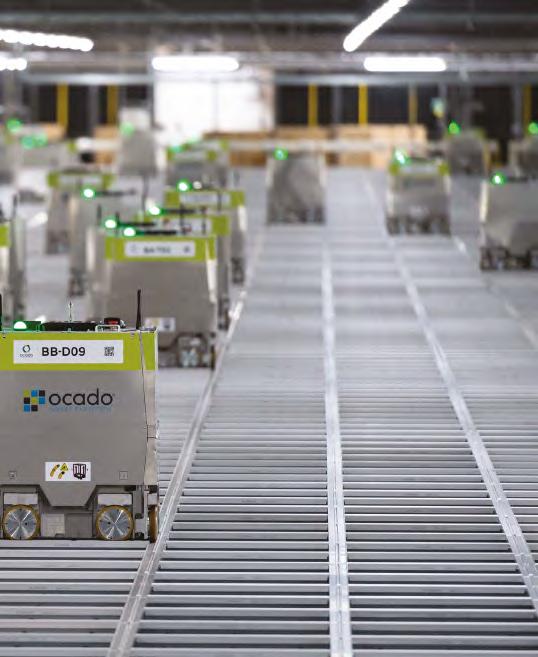
able to deal with a huge variety of weight, size, shape, surface, fragility and packaging.
We use the latest AI and machine vision algorithms to solve this challenge. The system takes inputs from the cameras and sensors on the robot and outputs the motor-control instructions to move the robotic arm and the end effector.
We used imitation learning techniques where human pilots demonstrate how picking and packing should be done with a controller and the robot arms learn from this data, generalise, and improve over time.
Now that robotic pick is live in operations, it’s supervised by remote teleoperation crews who can assist the arm in identifying grasp points for unfamiliar products or scenarios. If the AI gets ‘stuck’ it calls for a person to assist remotely. Every time we use teleoperation we gather data which, combined with the information of the failed pick attempt, helps train the AI to handle similar scenarios in the future.
How are you using technology to optimise the delivery journey on the roads?
Unlike more traditional courier services, grocery orders cross multiple temperature regimes, take up significant space, and are expected within
narrow time windows. As a result, a grocery delivery van may deliver 20 or so orders in a shift, whereas a van delivering parcels might deliver hundreds. This makes the labour cost in the last mile far more significant on a per-order basis.
Minimising delivery costs is therefore vital to achieve the margins we want. Without AI, it would be difficult for us to deliver groceries to your door on time for the right cost.
Firstly, AI helps us minimise the number of bags we use, and therefore vans and routes. 3D packing algorithms mean we can densely fill shopping bags so they fit into a lower number of vans, whilst ensuring bags aren’t too full so fragile items might be damaged.
Our AI delivery optimiser calculates the optimal distribution of all of our orders onto available vans taking into account the order volume, weight, availability of vans and drivers and much more. It also plans the optimum path for all of these vans to take in order to minimise fuel consumption, number of drivers needed, and ensure everything gets delivered on time.
It takes into account traffic conditions, and learns from past drive time and stop time data for every road and every customer address.
Data from vans is used both for the real-time monitoring of the routes and for feeding into our routing systems so that the routes we drive tomorrow will be even better than the ones we drove today – e.g. the best place to park on a Wednesday afternoon during school term time may be very different to the best location on a Sunday morning.
How important is human critical thinking in your approach to deploying AI?
AI is embedded throughout our tech estate. We have over 130 distinct AI use cases across Ocado. Many of our robotics products deal with highly unstructured environments. We can’t pre-programme a robot to deal with the many millions of scenarios it could be faced with. Due to the high number of unknown factors and almost infinitely variable environments, we must have AI systems based on data that can learn, adapt and generalise.
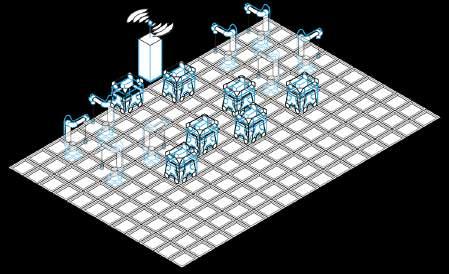
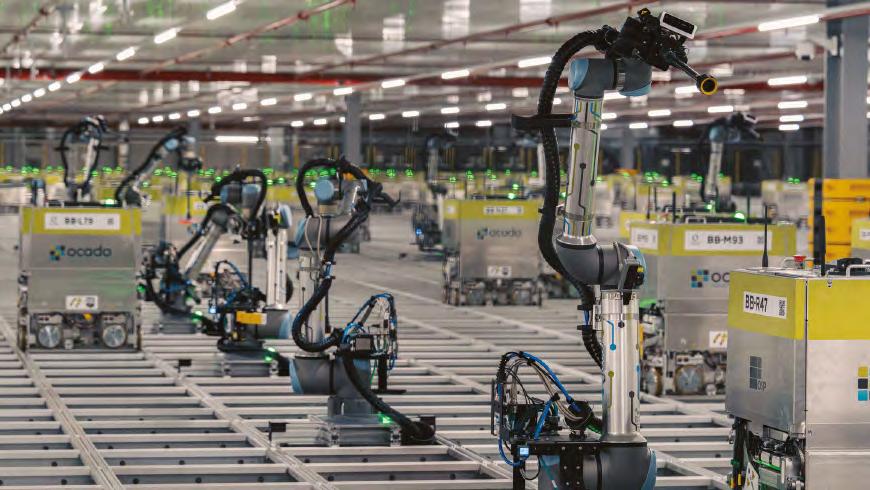
But any AI we use is always augmented with great engineering in other crafts. ML engineers and data scientists work alongside mechanical engineers, controls, mechatronics, electronics and software engineering. Our data team is embedded in our product streams, rather than being a totally separate function.
Despite our wide-scale use of AI, our mantra is to always choose the right tools for the job. We need to remain critical when we use this technology. AI is not the right approach for every product domain. There are many areas where we will always have hardcoded software systems.
We take a similar approach with the latest generative AI tools. Generative AI, or any sophisticated AI
approach, can be very exciting, but there is only a small subset of business problems that are best solved by very sophisticated techniques.
More often, the most important thing is good data and an understanding of what it means. It might be very trendy to develop complex algorithms to achieve certain tasks, but often there’s a simpler maths approach which will be just as effective. And likewise, small, faster and cheap-to-run machine learning models beat massive parameter large language models at a lot of tasks.
What is Ocado’s approach to ensuring responsible decisions are made with AI technology?
With so much AI technology embedded across our platform, it’s critical that we develop and deploy these technologies as robustly as possible. We want our partners and their users to feel confident about using our AI and robotics products and services. They want to know we’ve thought about performance, safety and privacy. This is even more important as we scale internationally and support new businesses beyond the online grocery space.
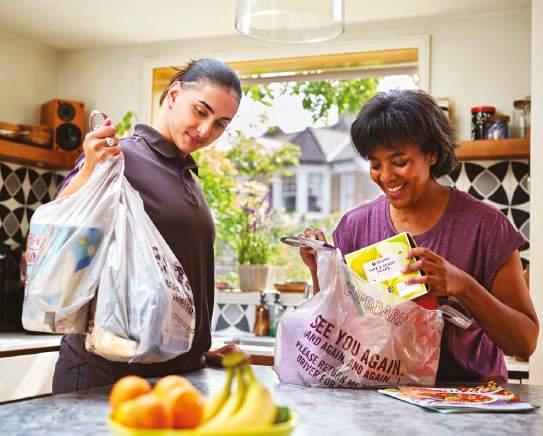
This is why we have committed to design and deploy AI systems responsibly – always considering fairness, accountability, transparency and explicability throughout the AI lifecycle. This will ensure systems function as intended and prevent mistakes, meaning responsible AI is essentially successful AI.
CERTAIN
If you could build precise and explainable AI models without risk of mistakes, what would you build? Rainbird solves the limitations of generative AI for high-stakes applications.
CHRISTOPH SPORLEDER
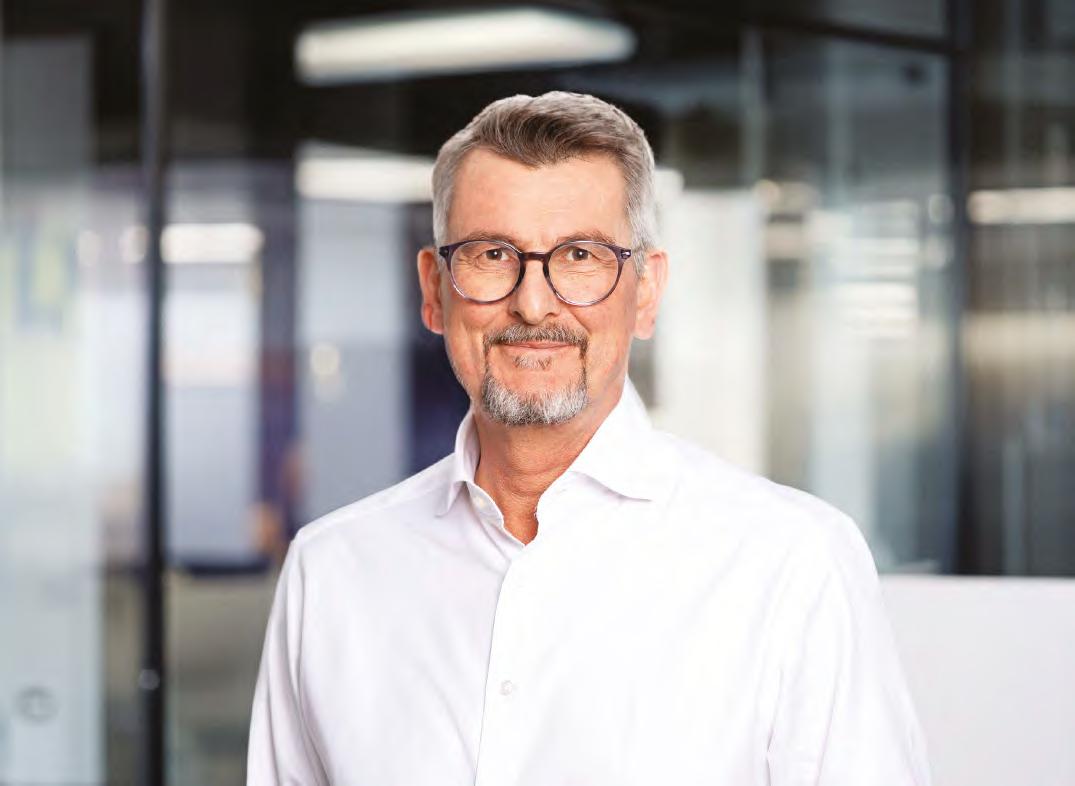
CHRIS is a managing partner at Rewire, a data and AI consulting firm with offices in Amsterdam and Heidelberg. He is responsible for developing client relationships in Germany, Austria and Switzerland. Rewire’s DACH operations provide end-to-end data and AI transformation, from strategy through implementation and change management.
MAXIMISING THE IMPACT OF YOUR DATA & AI CONSULTING PROJECTS
FROM STRATEGY TO EXECUTION: A GUIDE FOR ENTERPRISE LEADERS
Could you share how you got into consulting?
My journey started in data and analytics. My first role was with a utility company where I analysed mainframe data and even helped calculate the company’s first wind park. I was fascinated by analytics, and that passion led me to SAS, a leading data analytics company, where I was involved in building out their professional services division, which ultimately led me into consulting.
With the advent of cloud computing, the consulting landscape shifted dramatically. Previously, we focused on explaining the value of data analytics, but cloud technology has enabled us to operationalise insights in ways that weren’t previously possible. This evolution has been incredibly exciting.
How did you communicate the value of data back when it was relatively new?
The internet was just emerging then, but many industries were already familiar with using data, such as insurance actuarial work. For those in other sectors, we emphasised modernisation and the potential of new algorithms to enhance insights. But with today’s GenAI advancements, there’s far less emphasis on convincing people of data’s value – now the focus is on how to execute and achieve impact.
How did you experience the shift from local to cloud computing?
Cloud computing was a major leap, especially in
handling and integrating data from diverse sources. Thistransformation was also driven by IoT developments, connecting millions of devices and greatly expanding the data we could analyse, from customer behaviours to complex production processes.
In the past two decades, what aspects of enterprise data functions haven’t changed much?
Data management remains a challenge. We’ve evolved from data warehouses – low-tech but high-governance – to data lakes, which brought high-tech but low-governance, often creating data chaos. Now, with concepts like data mesh, we’re seeing a more balanced approach that combines technology with governance. Yet, engaging business leaders in data management is still an ongoing challenge.
How do you see the role of consulting in the data and AI space?
You have to differentiate between types of consulting services – there isn’t just one type, but many different flavours and shapes. It’s a spectrum:
On one end , you have the ‘body leasers’ – you order three units of data scientists, and that’s exactly what you get, but then you have to direct their work. On the other end , you have strategy consulting firms – the peak of the mountain, high-performing and fastmoving consulting firms.
In between , you have system integrators who lean more toward the first category, and boutique firms that position themselves in the middle or closer to the strategy end.
You need to identify the right type of consulting for your current state and engage with the appropriate firm. It’s rarely one provider from start to finish – you’ll likely need different types of services at different points in your maturity journey.
Choosing the right consulting partner is crucial. For instance, strategy consultants excel at high-speed, impactful short-term engagements but may require substantial internal resources to keep pace. In contrast, system integrators operate within very specific project parameters, and body leasing can lead to dependency on individual freelancers. Each approach has its place, but the fit depends on the project’s goals.
Do you view strategy as the starting point for data and AI initiatives?
Yes, I do. However, you need to be aware that data and AI initiatives can originate from different points in organisations:
Sometimes you have an enthusiastic board member who believes in it unconditionally. That’s a brilliant starting point, and your strategy becomes more operational since you don’t need to convince anyone at the board level. You can focus on implementation and achieving impact. If you don’t have that convinced board member, you first need to:
● Analyse your value pools.
● Determine the potential commercial impact.
● Calculate the transformation costs. This requires a different type of consulting service, typically a shorter engagement, but it’s necessary work to gain board-level commitment.
How often does misalignment occur between the developed solution and business goals?
Yes, that does happen and it’s not so much that the overall objectives fall apart, but rather the specific scoping of the solution that can be problematic.
What often happens is that there’s a complex challenge, like developing a ‘copilot’ based on generative AI. A copilot is typically not a single use case, but rather multiple use cases bundled together. Deciding how to slice and scope that effort requires very conscious choices:
● Do I go for maximum immediate impact?
● Do I prioritise speed of deployment?
● Do I aim for breadth in terms of how many functions, processes, or assets the copilot should address?
● Or do I take a more strategic, scaled approach – build the first use case to help develop the necessary infrastructure and data products, which then accelerates subsequent use cases?
These design choices are critical, and sometimes the solution being developed can end up not fully aligned with the organisation’s strategic direction. It’s very important to identify and fix any such misalignment very early on.
With today’s GenAI advancements, there’s far less emphasis on convincing people of data’s value – now the focus is on how to execute and achieve impact.
While less common in data and AI engagements compared to other types of consulting work, this challenge can still arise. The key is to maintain tight alignment between the solution being built and the overarching business goals throughout the project.
What common challenges do organisations face early in their data and AI journeys?
There are very common challenges, particularly around the expectation versus reality gap. The first step in capability building is understanding the art of the possible. There are a lot of expectations, especially now with GenAI, about what it can and cannot do. Spending time understanding the possibilities is crucial to avoid mismatched expectations.
A second major challenge is that organisations frequently underestimate the infrastructure and data management requirements needed to produce an AI use case. Transformations can fail in different directions: Some organisations overshoot by investing heavily in technology and data first. I’ve seen programs spend three years working on data without deploying a single use case. On the other extreme, some programs deploy three use cases without considering how to build a common data foundation or infrastructure.
Both extremes should be avoided. The right approach is to think about all dimensions and develop your infrastructure, data foundation, and organisational capabilities alongside the use cases that create impact. Recently, I posted about the end of the ‘lighthouses’ phenomenon. We’ve seen many organisations building exciting lighthouse projects by different groups, but with no common foundation or connection between them. While individual lighthouses may be stable, they don’t help the organisation scale data and AI because there’s no scaling plan behind them.
Once the partner is chosen, what can be done to ensure effective collaboration between the consulting company and the organisation?
There are several key considerations:
1. When choosing your partner, think about common objectives. We increasingly see performance-based coupling between organisations and consulting partners, working toward shared goals with remuneration tied to reaching these objectives.
2. Establish proper engagement governance with regular feedback loops between the client and consulting organisations.
3. Understand that consulting organisations also have an obligation to develop their people. On longerterm engagements, there will be some rotation of
personnel that needs careful planning. Friction can arise when consultants need to move on after 4-6 months to avoid task repetition and continue their professional growth. This requires open conversations and planning.
Are there procurement or RFP process improvements that would benefit AI consulting projects to ensure they are set up correctly? This is specifically about data and AI rather than general procurement. Data and AI projects have significant dynamics across different dimensions, similar to the early days of online marketing – you often end up somewhere different from where you initially planned. This doesn’t mean you’re not creating impact; you may just take a different path to achieve it.
For procurement, this means you shouldn’t engage data and AI consultancies in the traditional way you would work with a system integrator. Strict, tight statements of work can actually hinder your success.
What other factors are crucial for a successful consulting-client collaboration?
You need alignment across three domains: business, leadership, and technology. Business leaders provide insight into core needs, leadership drives organisational changes, and the tech team delivers solutions. These elements together form a strong foundation for impactful AI projects.
How important is leadership involvement in AI projects?
Essential. Successful AI initiatives integrate AI into processes, often involving workflow changes, capability-building, and sometimes even role redefinitions. Leadership commitment is key to driving these changes. You cannot implement data and AI at scale without senior leadership commitment. Without it, initiatives will remain siloed or isolated in specific departments. Everyone says AI will change the world and transform every process, but that will only happen if your most senior leadership not only supports it, but is convinced that this is a new enterprise capability.
Everyone says AI will change the world and transform every process, but that will only happen if your most senior leadership not only supports it, but is convinced that this is a new enterprise capability.
Looking forward, what trends do you see in the data and AI field?
I see a few key trends emerging:
INFRASTRUCTURE AND TECHNOLOGY:
● The hyperscalers are dominating the infrastructure space, but architectures are becoming more componentised. There’s rapid innovation in data and AI tools, so organisations need the ability to easily swap out components rather than being locked into specific solutions.
INTEGRATING CONVENTIONAL AI AND GENERATIVE AI (AGENT NETWORKS):
There will be a lot of discussion around how to integrate conventional AI models (e.g., for risk scoring) with the new wave of generative AI models (language, image, video). Creating end-to-end processes that leverage both will be an important next step.
DATA MESH:
● The focus is shifting from AI as a separate tool on top of processes to AI becoming an embedded, natural step within processes. This allows for more seamless integration and automation of simple operational decisions.
What major pitfalls should organisations avoid in this space?
Many still underestimate the infrastructure needed to support AI. Additionally, trying to shortcut foundational work in data management or infrastructure will hinder sustainable AI growth.
Why should data & AI practitioners consider consulting as a career?
Consulting offers unparalleled learning opportunities. Every client and project is unique, exposing you to varied business challenges and technologies. It’s an excellent choice for anyone seeking continuous growth and diverse experiences.
While a full data mesh implementation can be highly complex and time-consuming, organisations can take a more pragmatic approach. Focusing on data product-centric thinking and selectively adopting certain data mesh components that can be implemented quickly, is a smart way to progress.
Can you explain the Rewire philosophy and approach?
Rewire not only help clients identify impactful areas but also support them in capability building and integrating AI into business processes. The Rewire approach is a hybrid between strategy consulting and implementation. Our aim is to drive impact collaboratively with clients, adapting engagement models as projects evolve. Our team’s strength is in balancing high-level strategy with hands-on execution and capability building.
FROM PERSONALISATION TO INSPIRATION : HOW IKEA RETAIL (INGKA GROUP) IS PIONEERING THE USE OF AI IN HOME RETAIL
& DESIGN
Can you give us an overview of how data and AI is set up at IKEA?
I work for IKEA Retail (Ingka Group), IKEA’s largest franchise. My work covers approximately 31 countries where IKEA operates throughout Europe, North America, parts of Asia, and South America. The Data & Analytics Team oversees AI within the company’s product teams, and deals with data foundation, governance, management, and insights for IKEA Retail, as a whole. We work alongside the product teams, or sometimes we work independently to build data platforms and other tech that the different product teams can enjoy and reuse. Recently, we opened an AI lab internally, which focuses on GenAI and applied AI functionality. Our goal is to have an R&D function across IKEA as we explore how new technologies can give new experiences to our customers.
Within my team, we have several specialist teams working on different aspects of innovation. One team is dedicated to what we call ‘retail data machinery,’ focusing on personalised recommendations, personalisation engines, and search optimisation. Another team specialises in insights, analysing the broader
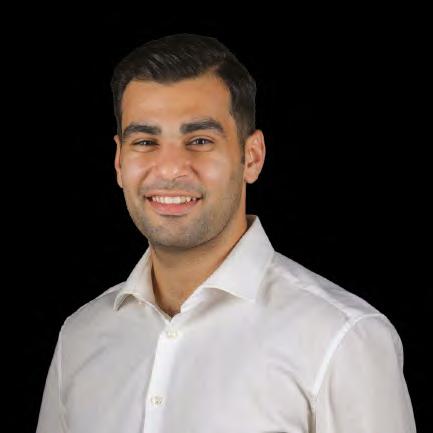
SHAHIN SHAHKARAMI leads a data and AI engineering team at IKEA, driving transformative changes for one billion customers across the globe. His focus is on recommendation systems, multi-modal search and NLP to personalise the omnichannel shopping experience. His team is focused on building end-to-end ML applications. Prior to IKEA, Shahin delivered analytics solutions in the telecommunications industry, enhancing customer satisfaction at scale.
customer journey – both online and across our omnichannel platforms – looking at how people transition between stores, apps, and the web. We’re also investing heavily in building generative AI capabilities that will shape IKEA for years to come.
What are the main areas of the business that your team work with?
We focus on growth, or as we call it, ‘growth and range’, which includes how we present IKEA products to our customers online. That’s an essential part of IKEA – our products and how we personalise them. We’re here to make sure every customer finds the most relevant products.
Another key focus is ensuring IKEA remains a truly unique omnichannel retailer, where the IKEA experience feels seamless and consistent, whether you’re looking at our website, our app or you’re in an IKEA store – the
goal is that these channels work cohesively, while staying true to the IKEA brand.
I also work on home imagination capabilities, which is about helping online customers imagine and better design their homes. We want customers to feel inspired and translate their home furnishing needs online in an interactive and playful way.
What’s the long-term strategy for data and AI at IKEA?
Our vision in IKEA is to develop high-quality data and AI products that enable accurate actionable insight and on the other hand infuses automate intelligence to enhance efficiency and performance. Specifically within AI, we are actively investing to build capabilities to maintain IKEA’s leadership within the home furnishing space.
How do you decide what data and AI use cases to work on? It’s always about having a clear
SHAHIN SHAHKARAMI
strategy of what we want to achieve. Where do we want to be in a few years’ time? Are there areas that we need to address as an online retailer, and also as a leader of life at home within the furnishing industry? The use cases and projects typically stem from two different angles. First, we consider our product with an overall view together with UX and engineering, to then understanding the product’s impact on the business, and the business goals we want to achieve. Then we consider how AI or data can help us attain that goal. That’s how we choose which projects to take on. And then there is an additional angle, which is to consider new capabilities. For example, with the GenAI situation, which opened up a lot of doors for us. We need to understand the tech and assess if it can solve our customer problems more efficiently or create new experiences for us. In those cases we start by experiementing the tech without having a use case in mind to begin with, then we see a realm of possibilities from there.
We do some opportunity sizing from there, and then see if we can embark on these projects moving forward.
How do you ensure that the data and AI teams collaborate well with business stakeholders and other teams in the organisation?
sometimes we take more of a hybrid approach: having a central team that supports the larger product teams.
There’s value to having a standalone function in the organisation; in being able to connect the dots between different product teams that might be operating in silos, due to the company size. We can take a more holistic view, and then collaborate between different teams, acting as the glue – and the voice of reason –for the organisation.
When you’re working with the teams on a specific project, who’s doing the execution? Each product team consists of four parts, as we call them: PDEX (which is product), engineering, data, and UX. These four work together from the start until the end of the project.
Sometimes there are different flavours in the organisation. For example in PDEX there are products that don’t need a data person because you’re building a front-end tooling, but some products are more AI-heavy. And in this instance we’re involved from the ideation to execution.
causal effect testing.
This has helped us to understand the value of the work of AI and our data scientists as well, because when you make a feature, you can always visualise the impact in that moment. But you can also do it holistically, at a larger level.
We also evaluate opportunity sizing – essentially, what the potential impact would be if we scaled a solution across all our global operations or removed certain organisational barriers. From there, we assess the return on investment (ROI). But it’s all about finding the right balance. For example, even if the ROI on a specific product’s search function is low, it’s still essential to have that feature on the website.
But for our large investments, we always ask: does it reduce costs by improving productivity or efficiency? Does it generate revenue, or enhance customer satisfaction? The initiative needs to deliver on at least one of these in the medium or long term. From there, we work backwards to identify specific use cases.
There should be a good balance between projects you know are going to generate revenue, save costs and improve customer satisfaction, whilst at the same time investing in projects that are more exploratory and innovative; asking open-ended questions that could pay off in the future.
The secret to good collaboration is ensuring we all share one goal. And within that common business goal, we have different specialists who work on achieving it.
We have our data and analytic coworkers embedded in different product teams. Sometimes we have our specialists fully embedded in certain product teams, because we know that will ensure the goal alignment and also the delivery of a certain data analytics, inside the product, or AI product. And
How do you measure the return on investment of data and AI initiatives at IKEA?
That’s one of the favourite questions of any C-suite or board member: how do we actually measure the impact of data and AI? Because it can all become a bit abstract. Over the last few years, we’ve built a great experimentation and measurement culture within IKEA, meaning that everything we have for our customers, always gets A/B tests and different types of
A great example of something that’s hard to quantify, is the fact that many customers who visit IKEA don’t use a shopping list, but they’re there for inspiration. They’re not just looking for a list of items to buy, they are seeking an experience. Because of this, we aim to have all these tools available to help customers get inspired, and then measure the impact of that inspiration – for example, if the customer is coming back or if they’re adding things directly. In these instances the ROI isn’t as tangible as you want it to be, but sometimes you need to address it in order to be an industry leader in this area. You want customers to come to you for inspiration as well.
Can you give us an example of a successful data and AI initiative at IKEA? I think one of the most successful
examples has been our journey in recommendation and personalisation. Our product range is at the heart of IKEA: the stores, the website, everything revolves around it. Our vision to help every customer access the most relevant range, the most relevant content, the most relevant services – this has been really important. So, we built an in-house recommendation engine.
This engine addresses a really complex set of recommendation problems, because we’ve got so many different journeys, different channels, different experiences, and you want to build something that’s effective and reusable, and also cost-conscious and scalable at the same time.
Essentially, our recommendation system consists of more than 15 AI models that work together to power different recommendation panels that we have on the website, and in other locations you might not associate with recommendation, like a chat or a 3D setting. The engine can offer the right product for a customer in all these contexts. And today approximately 40% of online visitors to IKEA are using it and it’s generating both direct and indirect revenue across the website.
In the past, we used to buy these recommendations from an external company. It’s been a strategic direction for us to build them all in-house and in a way that IKEA can generate the right content and products for different types of customers.
What prompted you to build these technologies in-house?
The way I see it is this: if the capability we’re developing requires complete control and a clear understanding of everything that goes in and out, then it’s something we need to build in-house. For example, IKEA is different from other retailers because it sells its own product online in many countries (compared to a retailer like Amazon, which has an open-
ended number of products). So, the complexity and challenge is thereby different, and we need to build these things internally to offer the best service to our customers.
What were the challenges you experienced when building this tech, and how did you overcome them?
The main challenges were twofold. First, there were technical challenges: we wanted to build personalisation for our customers, but did we have real-time data for our customers to personalise things in real-time settings? Could we serve these models in a scalable but cost-conscious way? And from an algorithmic perspective, how should we design this system to address all these journeys and channels in a way that’s reusable and functional?
We solved these technical challenges by having really smart people that work endlessly to solve these problems. We focussed on our data foundation, for example, by ensuring we understand our customers and customer data, setting up goods and the ops setup structures. And from an algorithmic perspective we gained a lot of knowledge, whilst solving these problems in an innovative way.
The second challenge was to overcome the organisational issues, in particular the change management side of things. Moving technology is easy because it’s logical: you move from point A to B. But organisationally, it’s always challenging when you’re coming up with new ideas that are changing parts of the company, fundamentally.
But thankfully, we’ve achieved a high level of collaboration within the organisation. We have a clear vision of where we want to go and we can help guide everyone towards that.
What impact has your background and experience had in the way you approach
productionalising tools of this complex nature?
I have a deep technical background in the space, and I’ve been really interested in AI since my academic experience. But when it comes to these complex systems, I always look at how they can be of practical benefit. And that’s where maybe my product owner side comes into play: really looking from the customer perspective. Does this really improve customer satisfaction? Does it really help our customer?
I sometimes feel the role I have is more of a translator: taking some really complex technical concept that might involve, say, the cost function of neural networks, and translating this for a senior leader or different department within the company towards business priorities. And then conversely, explaining some business idea which might sound really abstract to someone who works in data science because there are so many ways of doing it, and translating it back into technical language.
Can you tell us about the next potential use case for the project?
A next potential case is how we can help our customers better imagine their homes. Because the essential problem we’re trying to solve is this: if people buy product X or product Y, does it really fit in their room? Does it fit in with their style? Does it fit within their budget?
That translation is really difficult to achieve, because people’s homes are complex. People are complex as well. And that’s where we’re utilising AI to help customers imagine how the products will look, and how they will match their personal style.
There are different types of capabilities such as scaling designs, which give customers these tools to help them place products in their rooms, or to re-design the room, and to give them different options so they can compare styles. Our ultimate aim is to simplify
the process of designing a room. Interior design might be easy for a group of our customers, but not for most people. On the other hand, people do have a feeling for what they want and what they like, based on their needs and desires.
So in summary, that’s the use case: interior design, toolings, and 3D settings that are very, very interesting. And that’s what the team is focusing on at the moment. We’re still in the early stages of investing in and understanding these capabilities. We’re committed to doing this responsibly, ensuring that everything we create prioritises the safety and well-being of both our customers and our business.
As a GenAI use case the project has huge potential. Why should you buy this pillow that’s white and square versus the other pillow that is also white and square? There’s this whole angle of better storytelling and answering customers’ questions, helping them when they’re deciding and choosing products.
And for certain types of products, you need to help people visualise the end design. Let’s say if you’re buying a kitchen and you’re spending a significant amount of money. Or if you just bought a house and it’s completely empty and you don’t know where to start. It’s all about removing the fear of design for the customer, by helping them imagine what the room could look like with these different styles and sets of products.
So, with these capabilities and technologies we’re building, we are using GenAI to scale that design. But on the other hand, we are also doing a lot of engineering and tech work to create these toolings as well.
What are you most excited about for the future of data and AI at IKEA?
This might sound cliché, but IKEA’s
SHAHIN SHAHKARAMI
mission of creating a better life for many, is honestly something really iconic. And I think AI can help with that. AI has huge potential for helping every single individual from different budgets, from different backgrounds,
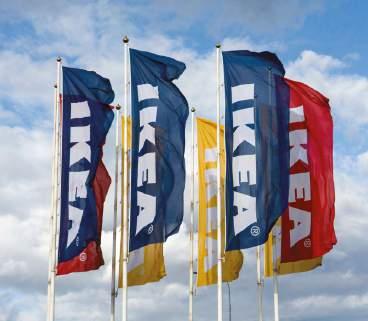
I believe we’re on the verge of a revolution in e-commerce and retail. In five years, we may be embracing experiences we can’t even imagine today – experiences that will soon feel as natural as the ones we rely on now.
different stages of life. You could be a student, you could be a young professional, you could be retired and use these capabilities. That’s really exciting!
And more specifically within my own domain, what really excites me is that there’s a lot of movement in spatial computing, spatial understanding, of the interior space. GenAI capabilities are getting a lot better at that. When exploring that area of spatial computing for IKEA, there are a lot of the models that have become cheaper and better. There’s the option to offer hyper personalisation at scale and give new experiences to our customers. And storytelling our range to our customers is another topic that really excites me.
What future trends in AI and data could impact large enterprises like IKEA?
There are three main trends. One is, of course, AI acts. the EU AI Act, for example. How that impacts an organisation. IKEA has an immense focus on that; we’ve been investing in responsible AI, and we’ve got responsible AI teams organised within the company to become a leader in this space.
The second is the future trends of productivity at work. I think all these productivity tools can really enhance the day-to-day work of a lot of our coworkers in large organisations. At IKEA, for example, even if you move productivity by a few percentages, it will have a massive impact. If your coworkers are more productive in answering customer calls, in answering customer questions in the stores, or maybe even if software engineers are more efficient in the way that they write code – that will drive meaningful transformation.
And the third trend is GenAI. It now has better multimodal capabilities that are cheaper, safer and more secure. It can be used on your device and not in the central clouds. These models are evolving into having better reasoning, and getting better at solving complex tasks. There are a lot of new applications that will come with GenAI. I believe we’re on the verge of a revolution in e-commerce and retail. In five years, we may be embracing experiences we can’t even imagine today – experiences that will soon feel as natural as the ones we rely on now.
HOW TO BUILD AN OPEN-SOURCE COMMUNITY IN BIG PHARMA
PROGRAMMING LANGUAGES:
A TRUE PARADIGM SHIFT IN THE PHARMACEUTICAL INDUSTRY
In recent years, the pharmaceutical industry has experienced a significant paradigm shift in its adoption of opensource programming languages. Up until recently, each young graduate had to choose between open-source and proprietary programming languages. However, the landscape has evolved, with a majority of pharmaceutical companies now embracing a multilingual programming strategy. The goal is to use the right tool for the right job.
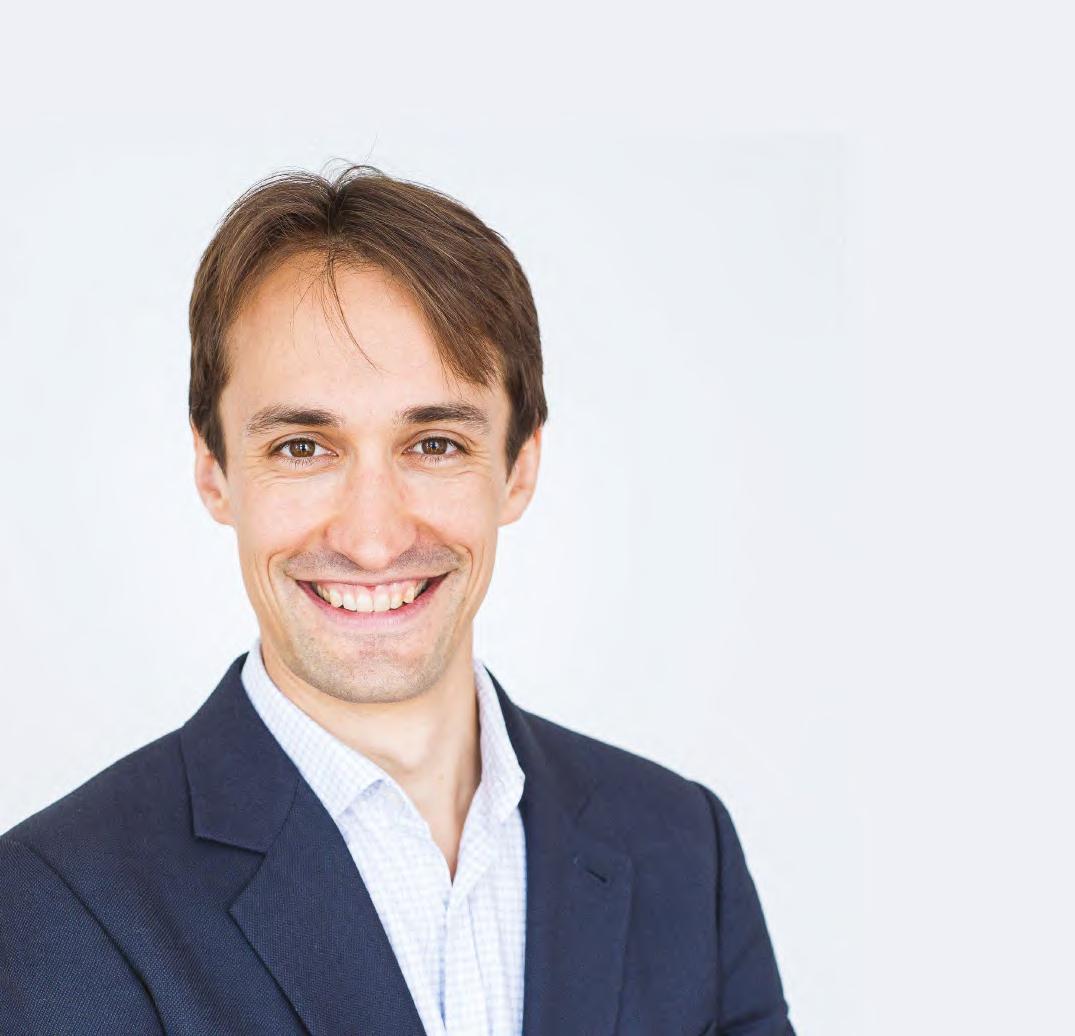
GUILLAUME DESACHY is dedicated to helping bring the right medicine to the right patient by leveraging the power of biometrics.
As Head of Biometrics at Pierre Fabre, he leads a department of 15+ experts in data management, programming and statistics, working collaboratively to drive the success of both clinical trials and real-world evidence studies. The Biometrics Department supports all drugs developed and commercialised by Pierre Fabre. Since his graduation from the French National School of Statistics (ENSAI) in 2011, he has had the privilege of working across all stages of drug development (from pre-clinical research to launch) across diverse landscapes, including academia (UCSF - U.S.), biotech ventures (Enterome - France) and global pharmaceutical industry leaders (BMS, Servier, AstraZeneca & Pierre Fabre - France & Sweden).
AUTHOR : Guillaume Desachy
WORK DONE AS : Statistical Science Director, Biometrics, Late-stage Development, Respiratory and Immunology (R&I), BioPharmaceuticals R&D, AstraZeneca, Gothenburg, Sweden
CURRENT EMAIL ADDRESS : guillaume.desachy@pierre-fabre.com
In early 2021, a group of us at a major pharmaceutical company convened to discuss the growing importance of the open-source programming language R within our organisation and the industry at large. R was not a new thing at AstraZeneca, nor was it a fleeting trend. Despite its established presence, we had limited insight into who the R users were within this large organisation, and there were no formal channels for them to connect, share knowledge, or inspire one another. No forums, gatherings, or events existed to facilitate the collaboration and growth of this community.
OUR OBJECTIVE?
Federate all collaborators using the open-source programming language R, enhancing cross-team collaboration and knowledge-sharing.
We decided it was time to establish a community of R users within AstraZeneca. Since 2016, the company has utilised an internal social media platform called Workplace, akin to Facebook for companies, as the primary communication tool among groups. It was immediately clear that this platform would serve as the foundation for developing our R user community.
HOW TO KICKSTART AN OPEN-SOURCE COMMUNITY IN A BIG PHARMA COMPANY
We decided to steal with pride a successful concept from the external world: the data visualisation contest known as TidyTuesday * , which utilises opensource data. However, we didn’t just want to replicate
it; we aimed to make it uniquely our own. The core principle would remain the same as TidyTuesday, but with a distinctive twist. Each time we promoted a dataset, we aligned it with our corporate values and current global events. And that’s how #azTidyTuesday was born!
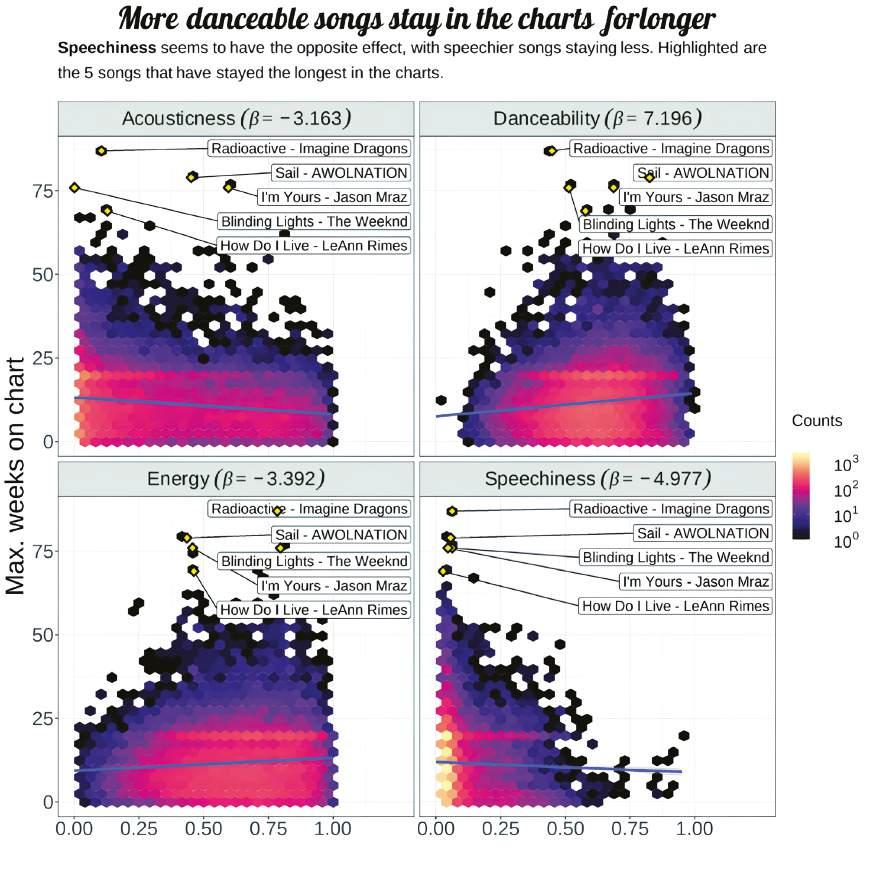
FIGURE 1
Featured entry for the final edition of #azTidyTuesday Dataset: Billboard Hot 100
Scientific question: Is there anything that can explain that a song is going to make it to the top of the charts? Could it be explained by its genre, its danceability, its energy, its loudness, its speechiness, its acousticness, its instrumentalness, its liveliness, its valence, its tempo, its duration?
Graph credit: Alvaro Mendoza Alcala
* TidyTuesday is a weekly initiative by the global R community for practising data analysis skills. Every Tuesday, a new dataset is posted on GitHub, and participants are invited to explore, visualise, and share their analyses using the R programming language and its packages, especially those from the tidyverse. It’s a great opportunity to learn, practise, and improve your R skills, as well as to see how others approach the same dataset.
#azTidyTuesday was just the beginning and proved to be a very popular way to promote and grow the community. It also underscored the need to develop additional initiatives to meet the diverse needs of our community members.
Shortly thereafter, we launched a monthly
initiative showcasing publicly available R packages or functions. This was done through blog posts that showcased both well-known and lesser-known packages and functions. Each blog post included a few lines of code that readers could try out at their own convenience and pace.

It quickly became apparent that we needed to federate this community of R users, and an internal R conference seemed like the perfect way to achieve this. Such an event would send a strong message both internally and externally.
The very first AstraZeneca R Conference, #AZRC2022, was scheduled for February 2022. At the time, our community was growing but still in its early stages, so we were uncertain about the level of interest, the topics that would resonate, and who would be
willing to help organise it.
To our amazement, more than 500 collaborators from 22 countries attended the inaugural AZ R Conference! This conference was a fully virtual, halfday conference featuring Max Kuhn as the keynote speaker, over 30 speakers and nearly 100 poster authors!
The conference was structured into three parallel tracks: governance, machine learning, and reproducibility.
500+ participants from 22 countries!
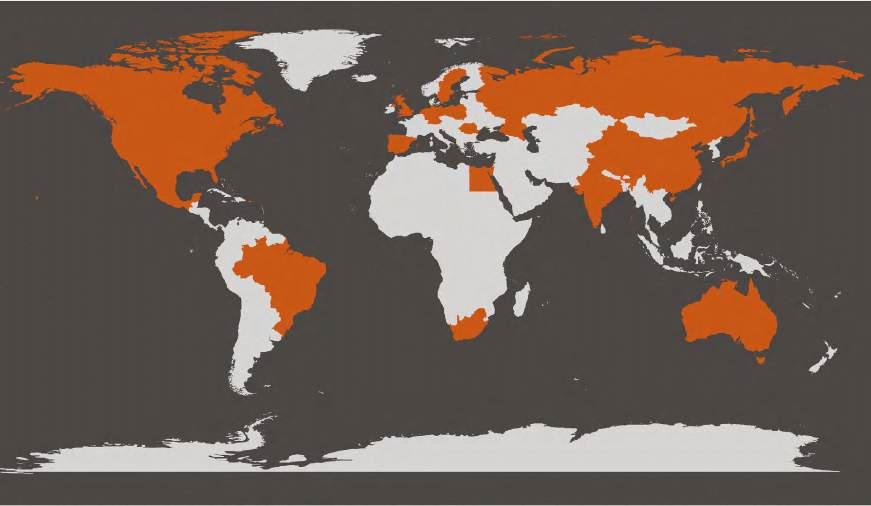
FIGURE 2
Countries highlighted in orange show countries from which AZ collaborators connected from for the AstraZeneca R Conference 2022. Graph credit: Tom Marlow
The overwhelming response to the conference sent a clear message: the community of R users within AstraZeneca was getting definite traction.
Rather than resting on our laurels after the success of this internal conference, we recognised the need to improve inclusion and diversity in addressing the community's needs and learning styles. We decided to evaluate all future initiatives based on two indices: the self-led learning index and the synchronicity index. The self-led learning index differentiates between self-directed learning and structured instruction, while the synchronicity index distinguishes between synchronous and asynchronous initiatives.
For example, #azTidyTuesday and the ‘Function of
the Month’ initiatives were asynchronous by nature, allowing community members to engage at their own pace. In contrast, the AstraZeneca R Conference 2022 was highly synchronous: although all talks were recorded for later viewing, real-time interaction with speakers required live attendance.
Following the inaugural internal R Conference, we knew we needed to continue fostering a sense of belonging and strengthening connections across departments.
We then launched ‘R @ AZ 10:1.’ The idea was to showcase an R user within the organisation and ask them 10 questions, further promoting engagement and collaboration within our growing community.
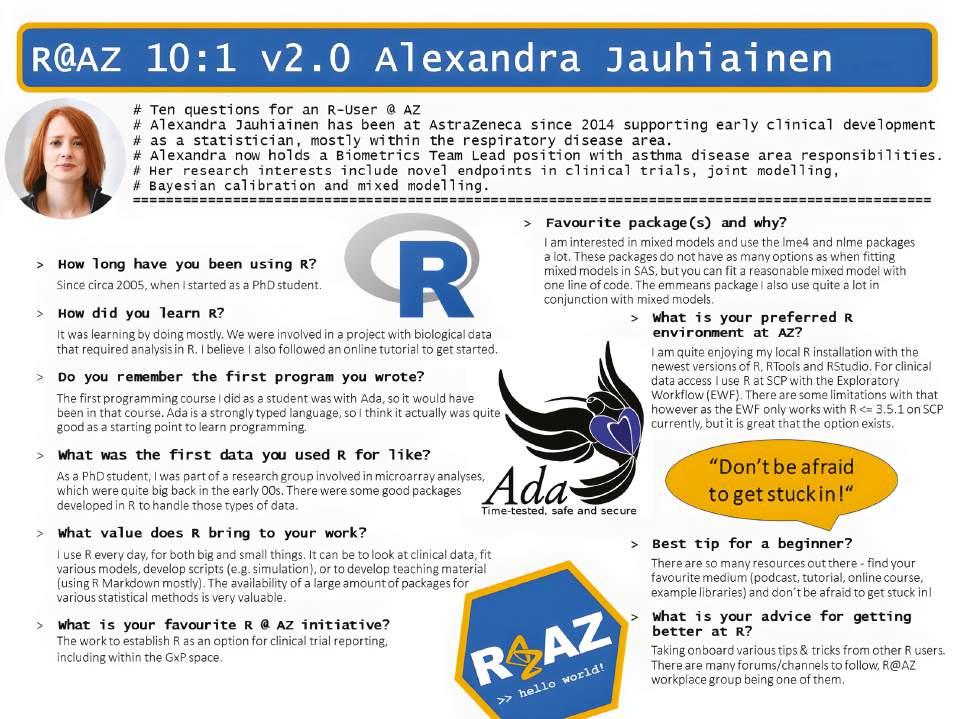
FIGURE 3
Example of R@AZ 10:1, featuring Alexandra Jauhiainen
Some of the feedback we received from the community after #AZRC2022 was a desire for more frequent, conference-like events. We heard this loud and clear, but we also recognised that everyone has busy schedules.
This led to the idea of hosting ‘Lunch & LeaRns’. The concept was simple: invite a member of our community to showcase something cool they did with R, all within a 30-minute session. The only rule was that each Lunch & LeaRn would last exactly 30 minutes, including Q&A.
And again, we kept inclusion & diversity in mind by scheduling these sessions at lunchtime, alternating between Europe and the U.S. to accommodate different time zones.
As more collaborators began returning to office sites, we saw an opportunity to further nurture these virtual connections in real life. This inspired the launch of the ‘R Hot Desk’ at the AstraZeneca Gothenburg site.
The principle is straightforward: once a month, experts in the open-source programming language R meet in an open area of the Gothenburg offices and dedicate two hours to answering R-related questions. This initiative allows for face-to-face interaction and real-time problem-solving, further strengthening the community.
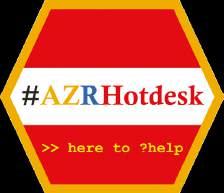
The interest in our face-to-face help desk did not meet our expectations. While there was some definite interest, the format may not have been ideal. Therefore, we decided to
rejuvenate the concept by transitioning to an online help desk instead of a face-to-face one.
WHAT IS THE VALUE OF THIS COMMUNITY? WHAT IS A KEY PERFORMANCE INDICATOR? WHAT IS ITS RETURN ON INVESTMENT?
All of this was based on voluntary involvement: there was no hierarchy between Guillaume Desachy (R@AZ Lead) and the members of the Steering Committee. Given the preciousness of everyone’s time, it’s reasonable to question the time spent building such a community. What is the value of this community? What are the key performance indicators (KPIs) for this community? What is the return on investment (ROI) of this community?
At first, these questions can be puzzling and extremely difficult to answer.
When considering the value of this community, we initially looked at the number of members in our internal social media group. However, this was not a true KPI, as it is not a metric we can directly influence. No matter how hard we worked, our initiatives could only nudge collaborators to join the group. Joining a social media group was never the end goal for this community. The true objective was to foster the community and encourage meaningful connections.
One metric that seems to be a more accurate KPI is the number of ongoing initiatives within the community. The Steering Committee has direct influence over this, as it decides which initiatives to start and run on a regular basis. This metric better reflects our efforts to actively engage and support the diverse needs of the community.
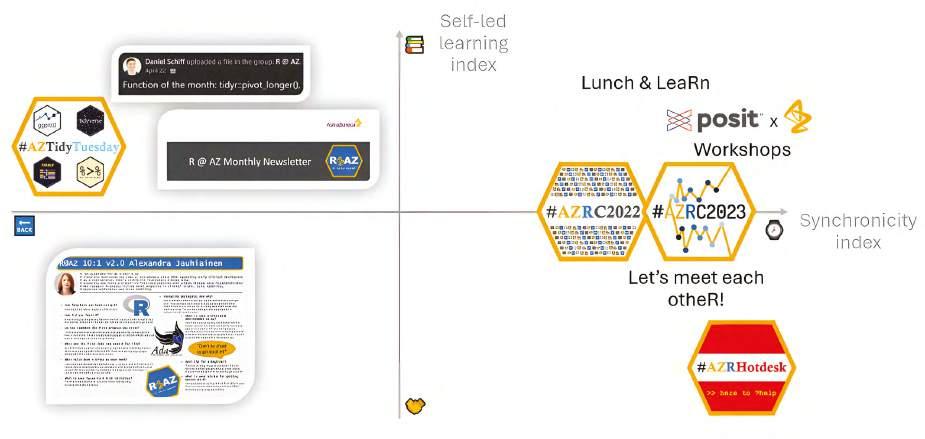
FIGURE 4
This KPI was used to gauge the vitality of this community. Regularly, we assessed additional KPIs for each initiative led by the R@AZ Steering Committee: Is the initiative well-attended? Is it well-received? Is it meeting the needs of the community?
When momentum around an initiative began to ebb, the Steering Committee would pause, reflect, and sometimes decide to retire the initiative. This was the case with #azTidyTuesday, which we decided to retire after 25 editions.
Key performance indicators are one thing, but they still don't fully address the question of return on investment (ROI). This question was particularly challenging to answer until one day when an Executive Director at AstraZeneca reached out to Guillaume Desachy and one of his teammates with a simple query:
‘Have either of you used this R package?’
Neither Guillaume nor his teammate had ever used this R package. However, we knew we could leverage the community. We posted a message in the Hot Desk Teams channel, and within 40 minutes, we had identified someone who had experience with the package.
Reflecting on this, we realised that without the existence of this community, it would have likely taken much longer to identify this subject matter expert. This highlighted the ROI of having a wellconnected and active community.
HAS THIS BENEFITTED THE BROADER ORGANISATION?
From the very beginning, our objective has been to federate all collaborators using the open-source programming language R, thereby strengthening collaboration across teams and enhancing knowledge-sharing. In less than three years, we have built a thriving open-source community of 1,600+ collaborators within a large pharmaceutical company. If we consider that all collaborators in this community are connected, it means there are a total of 1.4 million * connections within this community. By this measure alone, we have certainly met our objective of uniting all collaborators using this opensource programming language in AstraZeneca.
As decision-makers, we value tangible KPIs and a clear ROI. One achievement the Steering Committee is particularly proud of is the creation of a psychologically safe space. The once nascent Hot Desk where community members were hesitant to ask questions, has evolved into a place where collaborators of all skill levels can quickly find solid answers. This community has also enabled collaborators to take on leadership roles, whether leading an initiative, heading the organising committee for the yearly conference (20+ collaborators), or even leading the community’s Steering Committee (15+ collaborators). For those looking to develop professionally, this community has been a platform for honing skills such as leadership, communication, and networking.
This open-source community has been repeatedly recognised as a model for community-building within a company in the pharmaceutical industry and showcased in various forums: useR! 2022, R/ Pharma 2022, Posit blog post [2022], Posit MeetUp [2022], Posit customer story [2023], Boehringer-Ingelheim’s guest blog post [2023], The Effective Statistician Podcast [2023], R-Ladies Paris MeetUp [2024]
Over a three-year period, this once-emerging community evolved into a hub able to attract worldleading experts for exclusive gatherings.
Although the Lead of the R@AZ Steering Committee has now embraced a new career opportunity as Head of Biometrics at Pierre Fabre Laboratories, the R community he leaves behind at AstraZeneca lives on. A new Lead of the R@AZ Steering Committee has since taken over, and the community continues to grow, meet the needs of its members, and challenge itself. This ongoing effort consistently promotes the use of open-source within a large pharmaceutical company.
A true advocate for the importance of building communities and strong networks to enhance collaboration and knowledge-sharing across teams, Guillaume Desachy leverages his experience with R@AZ to foster collaboration across different departments at Pierre Fabre Laboratories. Striving to be as inclusive as possible, he remains mindful of diverse learning styles in all his initiatives and day-today work as Department Head.
HOW TO GET THIS STARTED IN YOUR OWN ORGANISATION
Whether your organisation is large, medium or small, building strong communities in a work environment is crucial. In this article, we have discussed the clear return on investment of such ventures. You too can start your own community of subject matter experts within your organisation. Here are five tips and tricks we’ve learned along the way:
● Start with the end in mind: Clearly define your objectives and what you hope to achieve with the community.
● Put a structure in place: Establish a framework for how the community will operate, including roles, responsibilities, and communication channels.
● Surround yourself with passionate people: Engage individuals who are enthusiastic and committed to the community’s success.
● Ask yourself: Is this initiative sustainable in the long run? Consider not just the first few editions but the next 20. Plan for longevity and continuous engagement.
● Doing things well is important and talking about what you do is equally important as it will help build momentum.
SPECIAL THANKS TO ALL WHO CONTRIBUTED TO BUILDING THIS COMMUNITY, INCLUDING Abhijit Das Gupta, Anna Samsel, Anna Strzeszewska-Potyrała, Antal Martinecz, Bartosz Górnikiewicz, Chuyu Deng, Daniel Schiff, Ewa Klimkowska, Gabriella Rustici, Gustav Söderström, Jamie MacKay, Jasia King, Jasmine Sadler, Kartik Patel, Lindsey Jung, Monika Eliasz-Kowalska, Monika Huhn, Parth Shah, Per-Arne Stahl, Rachel Evans, Sara Nozohouri, Theodosia Salika, Tom Marlow, Tomasz Lebitko, Vera Hazelwood, Yerka Larsson, and Zach Dorman.
For further insights into Guillaume’s career and publications, you can follow him on LinkedIn www.linkedin.com/in/guillaume-desachy.
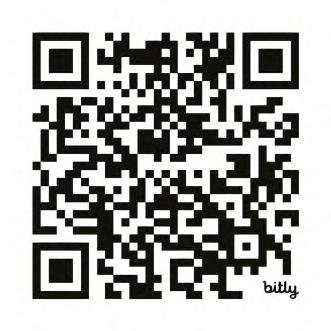
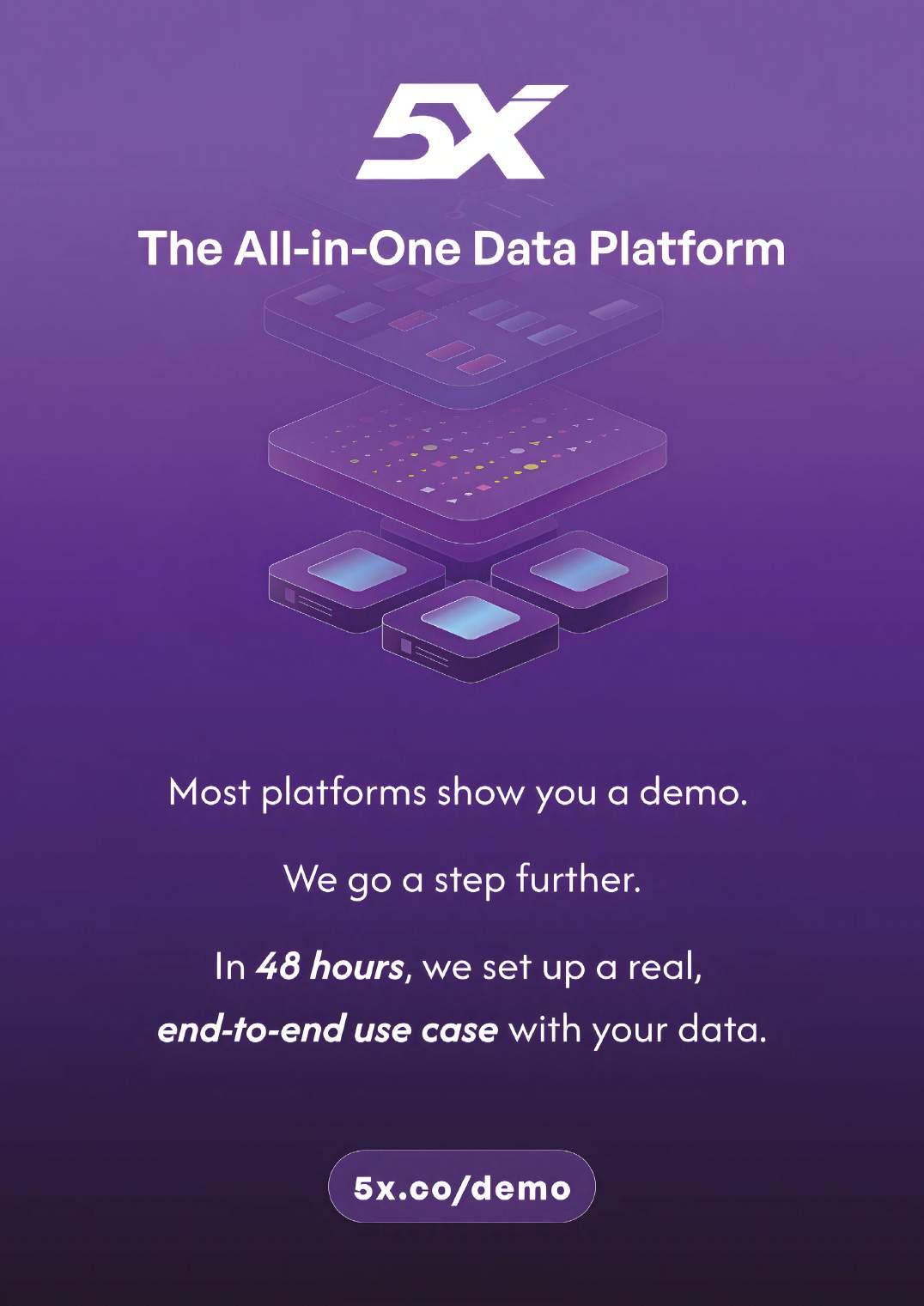
FROM PROBABILITY TO PRECI SION:
RETHINKING AI FOR CRITICAL APPLICATIONS
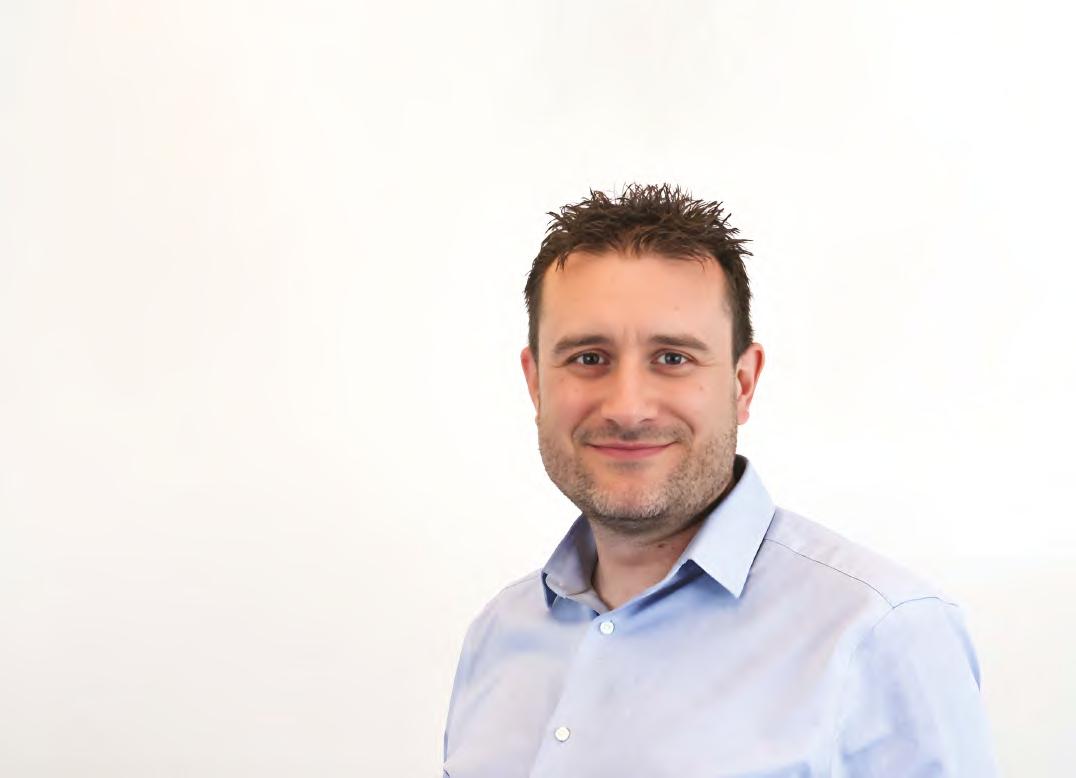
BEN TAYLOR is a pioneer of the causal decision intelligence movement. Before co-founding Rainbird in 2013, he was a computer scientist at Adobe and went on to lead the technical development of an award-winning AI system that revolutionised the insurance industry. Ben is a frequent speaker on the importance of trust in AI and is a member of the All-Party Parliamentary Group on Artificial Intelligence.
In high-stakes AI applications, precision and explainability are paramount, qualities that large language models (LLMs) inherently lack, regardless of prompting strategy or architecture. While LLMs are powerful tools for processing language, their predictive, probabilistic nature makes them unsuitable for tasks requiring deterministic, explainable decision-making.
This article explores the limitations of LLMs, particularly their inability to provide precise, causal reasoning. It introduces the role of causal reasoning platforms, such as Rainbird, to ensure accuracy and accountability in critical decisions.
By combining the strengths of LLMs in knowledge extraction with the deterministic nature of causal reasoning, organisations can achieve transparent and reliable decision intelligence in areas like tax and audit, credit decisioning, and health administration.
By combining the strengths of LLMs in knowledge extraction with the deterministic nature of causal reasoning, organisations can achieve transparent and reliable decision intelligence in areas like tax and audit, credit decisioning, and health administration.
BEN TAYLOR
BEN TAYLOR
THE PRECISION CHALLENGE OF LANGUAGE MODELS
Imagine asking an LLM like GPT4 or Sonnet 3.5, a simple arithmetic question: What is 1 + 1?
You will get the correct answer, not because the LLM is performing a calculation, but because it’s been wellexposed to the question in its training data.
Now, consider a slightly more complex question: What is 1 + 2 × 2?
The correct answer requires an understanding of order of precedence. Again, an LLM will likely give you the correct answer, but not because it understands the order it needs to perform the calculation. It’s not performing a calculation at all, it’s predicting the likely best output based on its training data, which for most LLMs, is the public internet.
But what happens when we scale up the complexity?
Let’s pose a larger number calculation directly to an LLM:
Calculate 5674328923 / 993
The response might surprise you. Instead of computing the exact answer, the LLM will generate an approximation and most likely, an incorrect result. If you ask the same question five times, you’ll likely get five different answers.
This is an inherent consequence of the way LLMs are designed. They predict the next word in a sequence based on patterns learned during training, not through precise mathematical computation.
This limitation highlights a fundamental challenge. LLMs are predictive, not deterministic. In high-stakes applications where precision is paramount, relying solely on predictive models will lead to errors that, depending on the use case, may have significant ramifications.
PRECISION IN HIGH-STAKES DOMAINS
Precision isn’t just crucial in calculating arithmetic; it’s vital across any high-stakes domain where decisions are of high consequence.
For example:
● Tax and Audit: Ensuring compliance with tax regulations requires precise identification of appropriate tax treatments. Auditors must meticulously evaluate financial statements to detect discrepancies or fraud.
● Credit Decisioning: Banks must accurately assess the creditworthiness of applicants to minimise defaults and remain on the right side of financial regulations.
● Healthcare Administration: Accurate management of patient records and billing is crucial for ensuring proper treatment, reimbursement and regulatory compliance. Health administrators need precision when tracking healthcare quality metrics, to improve patient outcomes and meet accreditation standards.
In these areas, decisions aren’t just about numbers –they’re about understanding cause and effect in realworld situations. They require reasoning over knowledge while ensuring compliance with regulations, providing transparent and logical explanations for each outcome.
ADDRESSING SYMPTOMS, NOT ROOT CAUSES
When discussing the shortcomings of LLMs, the conversation often centres around avoiding hallucinations – the term used to describe the tendency of these models to generate plausible-sounding but factually incorrect or nonsensical information.
There are many approaches you might take to ground an LLM and limit the impact of hallucinations. You might, for example, use retrieval augmented generation (RAG) to ground the LLM into referencing your own documented sources. Techniques like RAG and other prompting strategies, such as chain-of-thought (CoT), improve an LLM’s recall and may reduce hallucinations, but don’t address the core issue.
Hallucinations are symptomatic of a broader and more fundamental issue – an inherent lack of precision in LLMs. This is because fundamentally, LLMs operate on probability distributions, not logical reasoning.
While RAG and other similar processing architectures may improve factual accuracy, they cannot turn an LLM into a precise, deterministic reasoning engine. They can never be precise.
All of the strategies implemented to mitigate hallucinations are addressing symptoms rather than root causes. The result is an imprecise attempt to simulate logical reasoning without the benefit of actually being logical. OpenAI’s GPT4 o1 model, previously known as Project Strawberry, is a good example of this.
No grounding or prompting strategies can transform a probabilistic text generator into a deterministic reasoner.
WHEN DETERMINISM MATTERS
Deterministic systems are essential when:
● Precision is required: In fields like finance or healthcare where small errors can have significant consequences.
● Explanations are necessary: When decision-makers need to understand the causal rationale behind outcomes if they are to trust and act upon them.
● Regulatory compliance is mandatory: Where transparent decision-making is crucial for meeting legal and ethical standards.
What’s required to close this gap is a companion technology that can perform the equivalent of a mathematical calculation, only over logical expressions of knowledge.
This encoding approach serves two key purposes: it enables us to derive precise, reproducible answers from
BEN TAYLOR
knowledge representations, and it allows us to trace the reasoning behind the system’s conclusions.
Renowned computer scientist Judea Pearl, a pioneer in the field of causal reasoning argues that, while data can show us correlations, it can never truly tell us why something happens.
After more than two decades of AI being synonymous with data-centric machine learning, this is a critical distinction. In high-stakes decisions, understanding the causal relationships, the why behind an outcome, is just as important as the outcome itself.
Consider a medical diagnosis scenario. An LLM might correctly correlate a condition based on a list of symptoms, but without understanding the causal relationships between a disease and the resulting symptoms. It can’t provide the kind of explanatory power that a human doctor, or a causal reasoning system, could.
For high-stakes decisions, knowing that two variables are linked isn’t enough. We need to comprehend the underlying mechanisms and causes behind their relationship. Understanding the pattern alone is insufficient, so grasping the causal connections is crucial for making informed, reliable decisions that we can trust.
ENTER CAUSAL REASONING
To address these challenges, we must turn to causal reasoning and symbolic models. Unlike LLMs, which generate outputs based on patterns from their training data, symbolic AI operates on explicit rules and logic. In Rainbird’s case, these are structured as weighted knowledge graphs.
This approach enables nuanced but ultimately deterministic outputs. Given the same inputs, a deterministic system will always produce the same results and is able to explain why.
A brief historical perspective helps us to understand the foundations of this approach.
The field of knowledge representation and reasoning (KR&R) has been a cornerstone of AI research since the discipline’s inception. Early expert systems demonstrated the power of encoding domain knowledge in a form that machines could reason over. While these systems had limitations, they laid the groundwork for modern causal reasoning platforms that have now overcome those limitations.
Causal reasoning powered AI systems consist of two parts:
1. Knowledge Representation: Experts encode domain knowledge into the system using logical rules and relationships.
2. Reasoning Engine: The system applies this knowledge to specific data, processing it through logical inference to arrive at evidenced conclusions. Importantly, this approach doesn’t require that all
knowledge be binary or perfectly defined. Where there’s uncertainty or ambiguity in the domain knowledge, this can also be encoded as part of the system. The key is that the reasoning process itself remains deterministic.
This determinism extends to scenarios with sparse or uncertain data. Even when working with incomplete information, a causal reasoning system can provide a perfect chain of reasoning, showing the cause-andeffect relationships that led to its conclusion, accurately propagating any uncertainty and reflecting it in the outcome. When ambiguity or uncertainty exists in the source knowledge or data, it can be explicitly encoded.
This method allows for precise calculations over knowledge and as a result, transparent decisionmaking. Outcomes show the source and implications of all uncertainties.
This isn’t to say that LLMs have no place in highstakes decision-making. While they are a poor proxy for reasoning, they excel at interpreting unstructured data, understanding natural language, and extracting relevant information.
In
high-stakes decisions, understanding the causal relationships, the why behind an outcome, is just as important as the outcome itself.
THE BEST OF BOTH
Consider the messy, unstructured world we live in; emails, customer reviews, doctor’s notes, written regulation, policy, operating procedures, handbooks and so on. LLMs can manipulate these forms of explicit knowledge into more structured forms of knowledge representation that are computable.
LLMs have been proven to be effective at processing unstructured data, including from sources of knowledge. They can identify key concepts, relationships, rules, weights and probabilities – and translate them into symbolic representations of knowledge that can be reasoned over with absolute precision and transparency.
Let’s return to our earlier example of using an LLM to perform calculations over large numbers.
When we give the large-number calculation to ChatGPT, we find it generates an accurate result in stark contrast with using the LLM directly.
ChatGPT is a tool which has been designed to pass off certain functions to companion technologies. It recognises the complexity of a maths problem, and rather than trying to predict the answer using the LLM it translates the input into a simple Python script. It then executes this script to precisely calculate the answer. This is a simple demonstration of how LLMs can create an executable encoding from unstructured natural language.
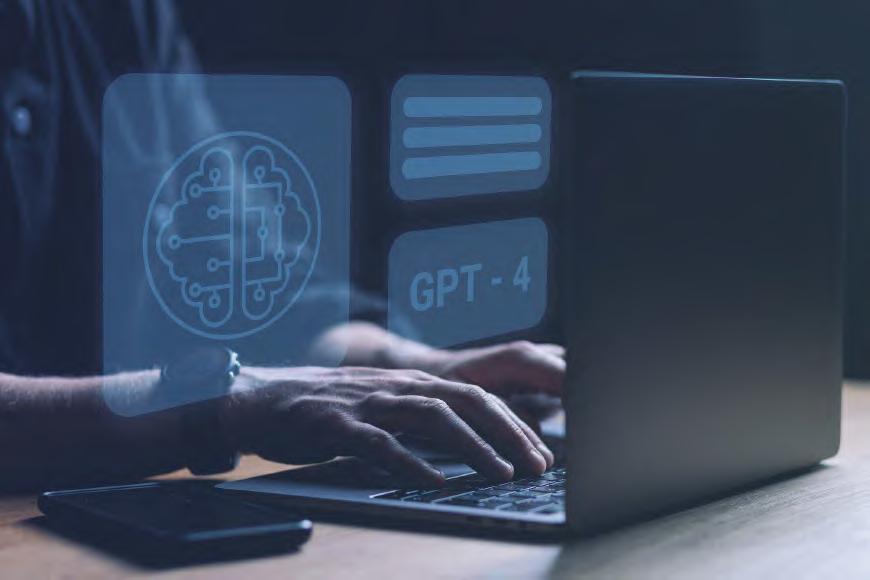
Extending that principle, it’s easy to recognise the power of using an LLM to understand a question being asked, together with any relevant data, and passing this to a causal calculator like Rainbird that can compute over knowledge captured in a knowledge graph to provide a precise answer.
The narrative of generative AI is biased towards the advancement and marketing of artificial general intelligence (AGI) by the large frontier model providers. But, the history of AI tells us that business value lies not in generalisation but in the application of such technologies to narrow domains.
The majority of people attempting to extract value from generative AI are not trying to build generalised tools at all, but seek the application of this technology to build narrower tools that can work precisely in critical domains.
LLMs are playing a vital role in brokering interactions between humans and data, while the reasoning is managed by a causal engine that can do so in the context of a model of what is important, in Rainbird’s case, encapsulated in a computable knowledge graph.
BUILDING THE AI WE CAN TRUST
As we look to the future of AI in high-stakes, precision-
critical applications, it’s clear that LLMs alone are insufficient. We must leverage the strengths of both generative and causal approaches.
LLMs can serve as powerful front-ends, interpreting human intent and translating it into structured forms. Causal reasoning systems can then take over, providing the precision, determinism, and explainability needed for critical decision-making.
This hybrid approach is already well-proven by Rainbird and powering decisions in tax, audit, law, lending, claims, healthcare and more. It offers the best of both worlds: the flexibility, language understanding and seamless user experience of generative AI, combined with the precision and logical rigour of logical systems.
In a world where we are slowly and inextricably yielding decisions to machines, and where AI is increasingly involved in outcomes that affect people’s lives, careers, and well-being, we cannot afford to rely solely on probabilistic models.
The future of AI lies not in choosing between approaches, but in skillfully combining them to create systems that are greater than the sum of their parts.
We are finally moving closer to a future where all AI systems are accountable and can truly do what we want them to do; drive decisions with precision, transparency, and reliability.
BEN TAYLOR
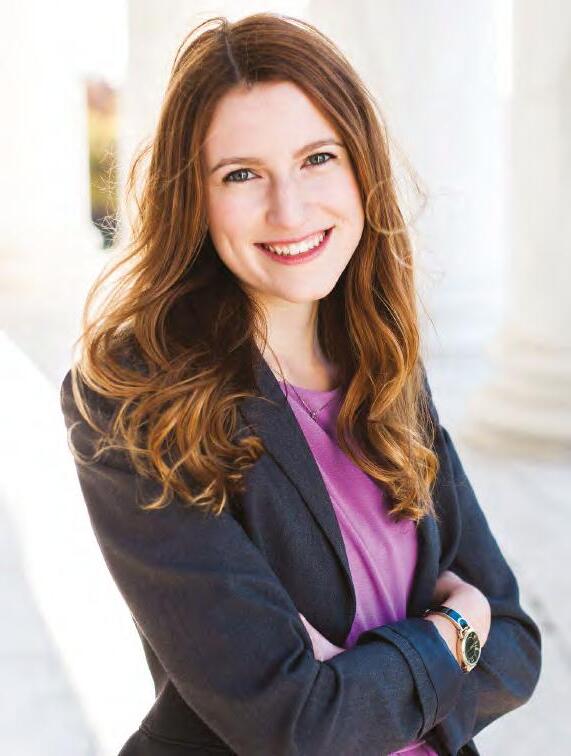
EXPLORING THE FUTURE OF DATA AND AI: FIVE MUST-READ BOOKS FOR TODAY’S DIGITAL LEADERS
A deep dive into the intersection of data quality, human-centred strategy, and the evolving role of AI in reshaping our world.
BY NICOLE JANEWAY BILLS
NICOLE JANEWAY BILLS is the Founder & CEO of Data Strategy Professionals. She has four years’ experience providing training for data-related exams and offers a proven track record of applying data strategy and related disciplines to solve clients’ most pressing challenges. She has worked as a data scientist and project manager for federal and commercial consulting teams. Her business experience includes natural language processing, cloud computing, statistical testing, pricing analysis, ETL processes, and web and application development. She attained recognition from DAMA for a Master-level pass of the CDMP Fundamentals Exam and Data Governance Specialist Exam.
Emergent technologies toe the line between overhyped and revolutionary. Over the last few years, generative AI has played jump rope with that line. Nevertheless, as GenAI graduates from its infancy, it has become clear that this technology will have a lasting impact on the world.
In this article, we look at five books that explore the evolving relationship between data, technology, and human factors, offering insights into data quality, strategy, and the rise of AI.
NICOLE JANEWAY BILLS
MEASURING DATA QUALITY FOR ONGOING IMPROVEMENT
Authors: Laura Sebastian-Coleman
Time to read: 6 hrs 16 mins (376 pages)
Rating: 4.1/5 (39 total ratings)
Measuring Data Quality for Ongoing Improvemen t by Laura Sebastian-Coleman is an excellent resource for data practitioners and organisations grappling with maintaining high-quality data. This guide explores the challenges of measuring and monitoring data quality over time, providing a structured approach that bridges the gap between business needs and IT capabilities.
Sebastian-Coleman begins by laying a solid foundation in the general concepts of measurement before introducing a detailed framework comprising over three dozen measurement types. These are mapped to five objective dimensions of data quality: completeness, timeliness, consistency, validity, and integrity. By focusing on ongoing measurement rather than one-time assessments, the book emphasises the importance of continuous improvement in data quality – a critical factor for organisations aiming to leverage data as a strategic asset.
One of the standout features of this book is its plainlanguage approach. Sebastian-Coleman avoids technical jargon, making the content accessible to both business stakeholders and IT professionals. This fosters more effective communication and collaboration across departments.
The guidance offered is not just theoretical; it provides actionable strategies for applying the Data Quality Assessment Framework within any organisation. Readers will find valuable insights on prioritising measurement types, determining their placement within data flows, and establishing appropriate measurement frequencies. The inclusion of common conceptual models for defining and
PEOPLE AND DATA
Author: Thomas C. Redman
Time to read: 4 hrs 24 mins (264 pages)
Rating: 4.8/5 (22 total ratings)
People and Data by Thomas C. Redman explores the relationship between non-data professionals and data in achieving organisational success. Redman, known as the ‘Data Doc,’ sheds light on why many companies have yet to harness the full value that data offers. He identifies a common misstep – regular employees are often excluded from data-driven initiatives, resulting in structures and processes that fail to capitalise on data’s potential.
The book underscores the importance of prioritising data quality improvement. Redman tackles challenging organisational issues such as departmental silos that impede the effective use of data, and he advocates for upskilling the entire workforce, demonstrating how employees at all levels can leverage data insights to enhance business performance.
1storing data quality results is particularly useful for trend analysis and detecting anomalies over time.
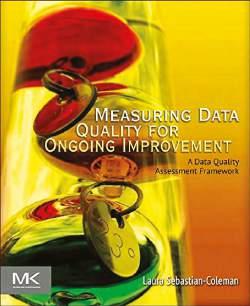
Furthermore, the book addresses generic business requirements for ongoing measuring and monitoring. It details the calculations and comparisons necessary to make measurements meaningful, helping organisations understand trends and make informed decisions based on their data quality metrics.
Laura Sebastian-Coleman’s extensive experience as a data quality practitioner shines throughout the book. With a career spanning over two decades, she brings a wealth of knowledge in implementing data quality metrics, facilitating stewardship groups, and establishing data standards.
Measuring Data Quality for Ongoing Improvement is more than just a guide – it’s a roadmap for organisations seeking to elevate their data quality practices. By leveraging this technology-independent framework, businesses can initiate meaningful discussions about data quality, prioritise improvements, and ultimately achieve a higher level of data integrity and trustworthiness.
TL;DR: Measuring Data Quality for Ongoing Improvement offers a practical, accessible approach to measuring and monitoring data quality over time, providing organisations with the tools to implement continuous improvement across five key dimensions.
2A standout feature of this book is its practical advice, complemented by real-world examples from organisations like AT&T and Morgan Stanley. Redman doesn’t just theorise; he provides resources such as a curriculum for employee training and tools to help companies align their data efforts with business goals.
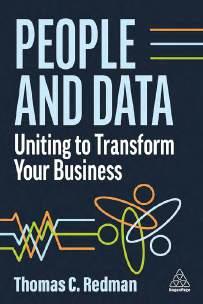
TL;DR: People and Data by Thomas C. Redman emphasises the crucial role of non-data professionals in maximising data quality and organisational success, offering practical guidance on uniting people and data to unlock a company’s full potential.
HUMANIZING DATA STRATEGY
Authors: Tiankai Feng
Time to read: 2 hrs 4 mins (124 pages)
Rating: 4.5/5 (38 total ratings)
In Humanizing Data Strategy , Tiankai Feng offers a refreshing exploration into the often overlooked human elements of data strategy. Drawing from his experience in data analytics, data governance, and data strategy, Feng bridges the gap between technical expertise and the emotional, irrational, yet crucial human behaviours that influence data initiatives.
The book stands out by combining relentless optimism with a candid reflection on common mistakes made in data strategy and practical solutions to address them. Feng introduces the ‘Five Cs’ framework – competence, collaboration, communication, creativity, and conscience – to link human needs with business objectives. This framework provides guidance for C-suite executives, operational staff, data experts, business users, and even students aiming to become change-makers in creating sustainable data strategies.
One of the compelling aspects of Feng’s approach is his acknowledgement of the role that emotions and impulses play in data strategy work. Rather than positioning these elements of the work as obstacles, Feng encourages the reader to see them as opportunities to enhance data strategy by making
CO-INTELLIGENCE
Author: Ethan Mollick
Time to read: 4 hrs 16 mins (256 pages)
Rating: 4.5/5 (1459 total ratings
Co-Intelligence by Ethan Mollick explores AI’s transformative impact on work, learning, and life. As a Wharton professor specialising in innovation and entrepreneurship, Mollick brings a unique perspective to discussing AI as a ‘co-intelligence’ that can augment or even replace human thinking.
Mollick begins by sharing his journey with AI, particularly his experience with the release of ChatGPT in November 2022. He describes the profound realisation that AI technologies are not just tools but entities capable of creative and innovative tasks once reserved for humans. This sets the stage for a deeper examination of how AI is reshaping industries, education, and personal productivity.
Diving into ethical and societal implications, Mollick also addresses concerns about job displacement and the future of human work. He challenges readers to harness AI’s power responsibly – learning from it without being misled and using it to create a better future. His balanced approach encourages proactive engagement with AI, emphasising collaboration over fear.
Mollick explores key themes throughout the book, such
3
it more inclusive and impactful. The author’s focus on empathy and compassion adds a human touch to data-driven decision-making.
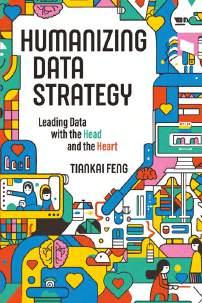
The writing is accessible and engaging, enriched by personal anecdotes and professional wisdom. Feng’s unique use of humour, music, and even memes to explain complex data-related concepts adds an unconventional yet effective dimension to the learning experience.
Humanizing Data Strategy is a call to action for organisations to recognise and leverage their most significant asset – their people. By integrating the Five Cs into data strategy, Feng provides a roadmap for creating a more collaborative, ethical, and effective data culture.
TL;DR: Humanizing Data Strategy by Tiankai Feng delves into the essential human aspects of data strategy, offering an actionable Five Cs framework to create more inclusive and impactful data initiatives by linking human needs with business objectives.
4
as understanding AI fundamentals, its impact on business and education, and navigating the ethical landscape surrounding AI and data privacy.
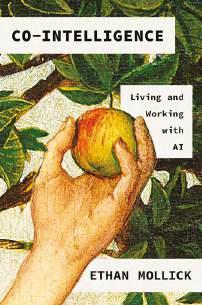
Endorsements from notable figures such as Angela Duckworth and Daniel H. Pink underscore the book’s significance. Duckworth describes it as ‘the very best book I know about the ins, outs, and ethics of generative AI,’ while Pink praises its lucid explanations and insightful analysis.
Co-Intelligence is for anyone curious about how AI is changing our world and what that means for individuals and organisations. Mollick combines expertise, practical advice, and a thought-provoking perspective to help readers understand and thrive in this new era.
TL;DR : Co-Intelligence by Ethan Mollick explores the transformative role of AI as a ‘co-intelligence,’ offering practical guidance on collaborating with intelligent machines to enhance work, learning, and life without losing human identity.
AI & THE DATA REVOLUTION
Authors: Laura Madsen
Time to read: 3 hrs 29 mins (209 pages)
Rating: 5/5 (15 total ratings)
In AI & The Data Revolution , Laura Madsen delivers a practical and insightful guide for data leaders navigating the complexities of today’s technology landscape. Businesses are increasingly relying on data-driven decision-making and AI-powered solutions. In her book, Madsen addresses the challenges and opportunities that come with this rapid evolution.
Drawing from her extensive experience as a global data strategist, Madsen provides a comprehensive roadmap to harness the power of AI while mitigating risks. The book offers effective strategies that data practitioners can implement immediately, making it an excellent resource for practitioners at any stage of their careers.
Key themes explored include understanding the fundamentals of AI and its implications for data leadership, navigating the ethical and regulatory landscape surrounding AI and data privacy, and creating sustainable models for technology disruption within organisations. Madsen also tackles the critical issue of technical debt that can hinder innovation, offering guidance on addressing it effectively.
One of the book’s strengths is its blend of theoretical
frameworks with real-world case studies. This approach equips readers with the knowledge and tools needed to thrive in the age of AI disruption. Madsen emphasises the importance of creating an AI framework with dynamic innovation in mind, ensuring that organisations remain adaptable in a fastpaced environment.
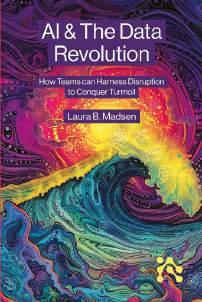
The inclusion of a step-by-step guide on how to get started further enhances the book’s practicality. Madsen’s writing is accessible and engaging, avoiding unnecessary jargon without sacrificing the depth of the content.
In addressing both the opportunities and the challenges, AI & The Data Revolution empowers readers to unlock the full potential of their data assets and drive innovation within their organisations.
TL;DR : AI & The Data Revolution by Laura Madsen offers practical advice and strategic insights for data leaders, providing guidance on leveraging AI, addressing challenges like technical debt, and creating sustainable models for innovation in today’s fast-paced, technological world.
CONCLUSION
From data quality to AI collaboration, these five books cut through the tech hype to reveal a surprising truth: the future of digital leadership isn't about the deployment of better algorithms – it's about better collaboration between humans. Through frameworks for measurement, strategies for teamwork, and hard-won insights about AI adoption, these books offer a masterclass in what really matter s when it comes to pushing forward effectively with emerging technologies. By integrating these insights into their work, leaders can effectively balance technology with human values, ensuring their organisations thrive.
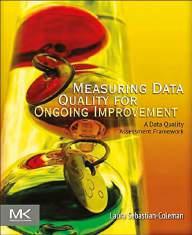
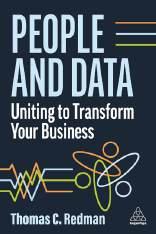
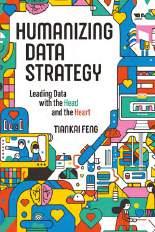
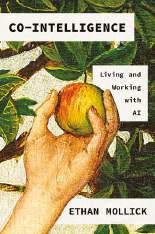
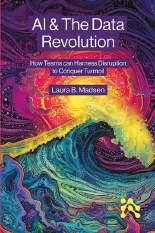
AI VS THE PLANET: THE ENERGY CRISIS BEHIND THE CHATBOT BOOM
AI’S INSATIABLE APPETITE FOR POWER – WHAT’S THE COST OF INTELLIGENCE?
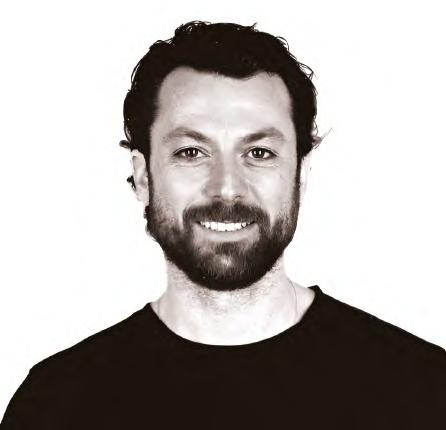
AI’S RAPID GROWTH AND ENERGY DEMANDS
ChatGPT recently hit a massive milestone of 200 million weekly active users – double what it was this time last year. This is incredible! But with rapid growth comes enormous energy demands. On average, ChatGPT alone uses around 453.6 million kilowatt-hours per year, which costs OpenAI about $59.4 million annually. That’s just for answering prompts, not even considering the energy used for model training.
ENERGY COSTS PER QUERY
Each query to ChatGPT reportedly uses around 0.0029 kilowatt-hours of energy, about ten times what a typical Google search requires. This may sound like a drop in the ocean, but when we scale this to ChatGPT’s 3 billion weekly queries, it consumes over a million kilowatthours daily. To put it into perspective, that’s enough energy to power over 43,000 U.S. homes for a day.
FRANCESCO GADALETA is a seasoned professional in the field of technology, AI and data science. He is the founder of Amethix Technologies, a firm specialising in advanced data and robotics solutions. Francesco also shares his insights and knowledge as the host of the podcast Data Science at Home
His illustrious career includes a significant tenure as the Chief Data Officer at Abe AI, which was later acquired by Envestnet Yodlee Inc. Francesco was a pivotal member of the Advanced Analytics Team at Johnson & Johnson. His professional interests are diverse, spanning applied mathematics, advanced machine learning, computer programming, robotics, and the study of decentralised and distributed systems. Francesco’s expertise spans domains including healthcare, defence, pharma, energy, and finance.
CHARGING ELECTRIC CARS OR RUNNING CHATGPT?
Imagine if we redirected all that energy from ChatGPT’s yearly consumption into fully charging electric vehicles. We’d be able to charge the entire fleet of EVs in the U.S. – all 3.3 million of them – almost twice over. These numbers are staggering, and they underscore how AI’s energy demands are approaching the same scale as some national power grids.
TRAINING IS AN ENERGY MONSTER
Training these large models is even more intense. When OpenAI trained GPT-4, it consumed about 62 million kilowatt-hours over 100 days, which is almost 50 times the power needed for GPT-3. And now, models like the OpenAI o1 are developed with even more sophisticated reasoning and training techniques, which may increase energy demands further.
FRANCESCO GADALETA
THE BLOCKCHAIN ANALOGY
FUTURE DIRECTIONS
EFFICIENCY-FOCUSED ALGORITHMS
AI algorithms today, especially deep learning models, consume massive amounts of computational power, particularly during training and inference. For AI to grow sustainably, we need algorithms optimised for efficiency from the outset.
KEY APPROACHES:
Pruning and Quantisation:
Techniques like model pruning and weight quantisation reduce the size and complexity of neural networks, making them faster and less powerhungry without significant accuracy loss. This could be a deep dive into the maths and techniques that make these approaches feasible.
Sparsity in Neural Networks:
Sparse models, where many of the network connections are zeroed out, offer a compelling way to lower computational costs.
Self-Supervised Learning:
Reducing the need for extensive labelled data and resources can lower the cost associated with data curation and processing, directly reducing the energy footprint of AI systems.
CONCLUSION
Efficiency-Focused Algorithms: AI research must prioritise models that consume less energy, especially during inference.
Now, here’s where AI reminds me of blockchain. Both involve massive computing power – think about all those GPUs crunching data nonstop. But while blockchain often offers minimal value and bloated energy costs, AI holds genuine promise. As engineers, we need to avoid following the same energy-intensive pitfalls of blockchain tech. AI has the potential to be a meaningful force for good, but we must carefully balance its benefits with environmental costs.
HARDWARE OPTIMISATION
Traditional processors, including GPUs, aren’t designed with AIspecific tasks in mind. Specialised hardware designed explicitly for AI tasks, like inference and model training, can drastically improve energy efficiency.
KEY INNOVATIONS:
ASICs and TPUs : Companies like Google have developed AIspecific processors (e.g., TPUs) that are highly efficient for machine learning.
Low-Power Edge Devices:
Specialised edge devices and microcontrollers, like NVIDIA’s Jetson Nano or Coral’s Edge TPU, are bringing powerful AI processing to small, low-power devices for local processing, dramatically reducing energy use.
Neuromorphic Chips: This emerging technology, inspired by the human brain, simulates neural activity with lower energy demands. Explain how these could redefine AI’s approach to resource constraints.
DECENTRALISED LEARNING
Training and inference on centralised data centres not only increases energy costs but also poses security and latency challenges. By decentralising AI processes, we can reduce energy costs and bring computation closer to the data source.
KEY METHODS:
Federated Learning: A distributed learning approach that trains AI models on edge devices, allowing data to stay local and reducing the need for extensive data transfers. This can save energy and enhance privacy.
Edge Computing with Local Inference: Moving AI computations to edge devices means models perform tasks on user devices rather than in centralised servers. This approach uses local resources, lowering the cost and energy required for data transmission.
Distributed Architectures: Peer-to-peer training architectures or grid computing setups could spread the computational load, using only nearby nodes as needed and potentially leveraging idle computing power.
Looking to the future, the energy cost of AI can’t continue unchecked. If we want sustainable growth, here are three directions for AI development that make sense:
Hardware Optimisation: We need chips explicitly designed for AI tasks that optimise energy use, like more efficient GPUs or specialised AI hardware.
Decentralised Learning: Moving away from giant centralised models to decentralised, smaller models that handle data locally could dramatically reduce energy consumption.
Need to make a critical hire for your team?
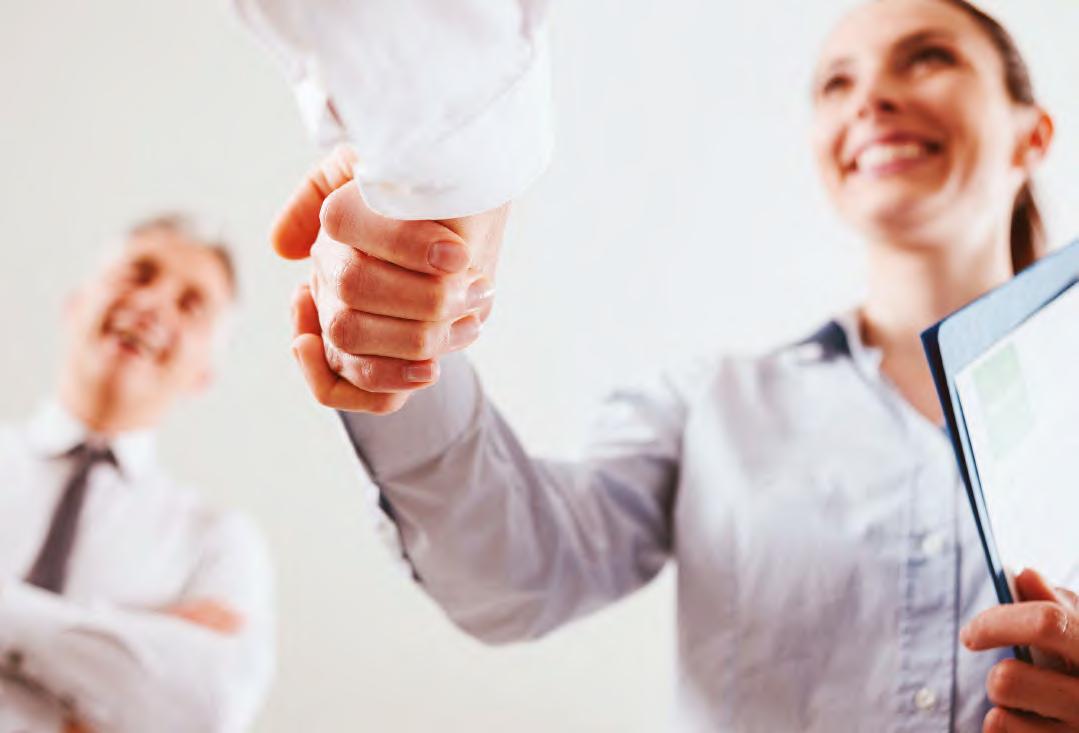
To hire the best person available for your job you need to search the entire candidate pool – not just rely on the 20% who are responding to job adverts.
Data Science Talent recruits the top 5% of Data, AI & Cloud professionals.
OUR 3 PROMISES:
Fast, effective recruiting – our 80:20 hiring system delivers contractors within 3 days & permanent hires within 21 days.
Pre-assessed candidates – technical profiling via our proprietary DST Profiler.
Access to talent you won’t find elsewhere – employer branding via our magazine and superior digital marketing campaigns.
Then why not have a hiring improvement consultation with one of our senior experts? Our expert will review your hiring process to identify issues preventing you hiring the best people.
Every consultation takes just 30 minutes. There’s no pressure, no sales pitch and zero obligation.
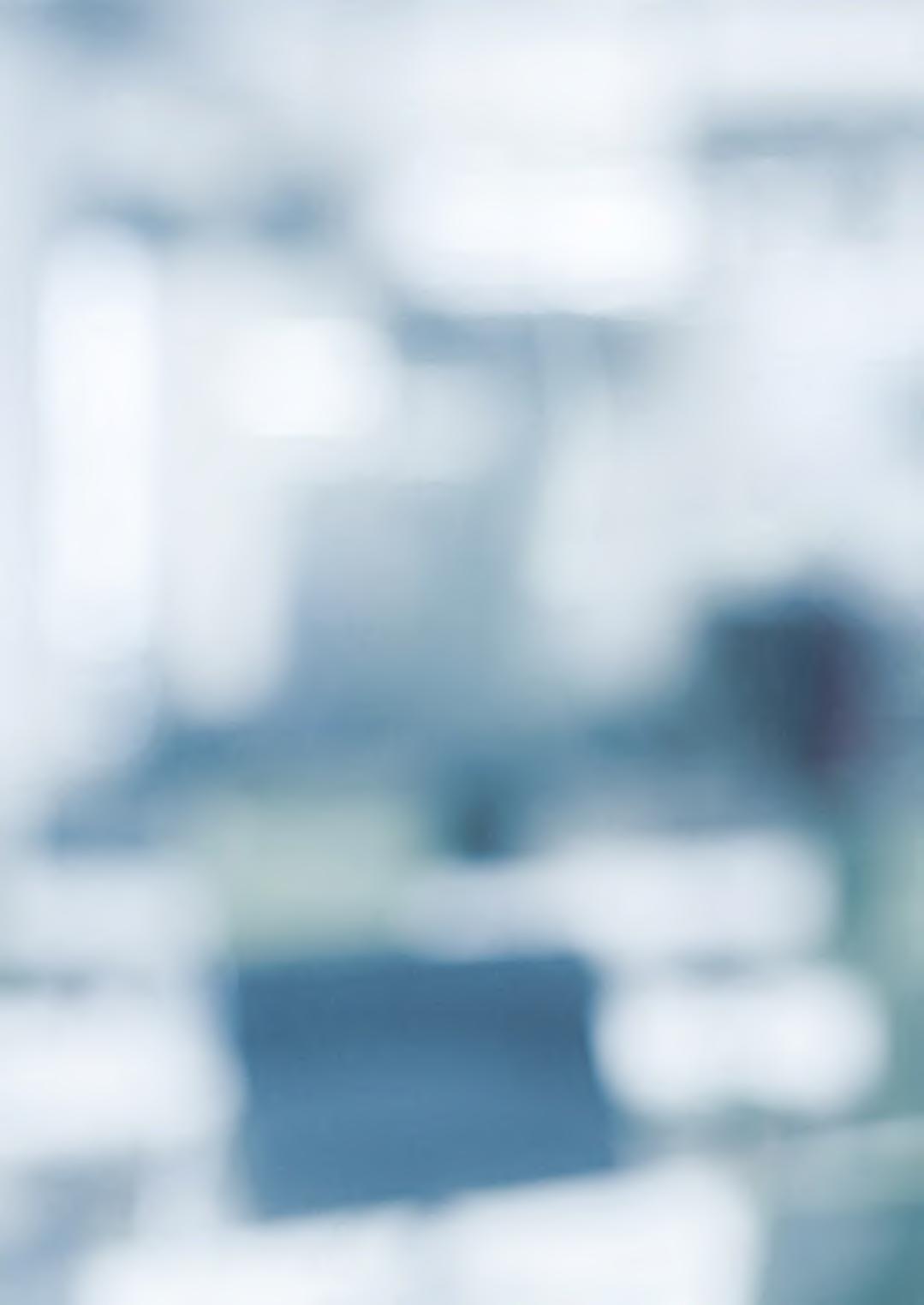
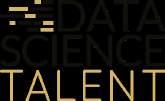
MY JOURNEY:
FROM ELECTRONICS & COMMUNICATION
ENGINEER TO DATA SCIENTIST
I, Jhanani Ramesh, grew up in Chennai, India, and my journey has taken me across the world and into the field of data science. From an early age, I have always been inclined towards mathematics and the latest technologies. This passion guided my academic journey, leading me to pursue a Bachelor’s in Electronics and Communication Engineering (ECE) from Anna University in Tamil Nadu, India. I relished the challenges and complexities that came with this field, and it deepened my curiosity about how technology could be harnessed to
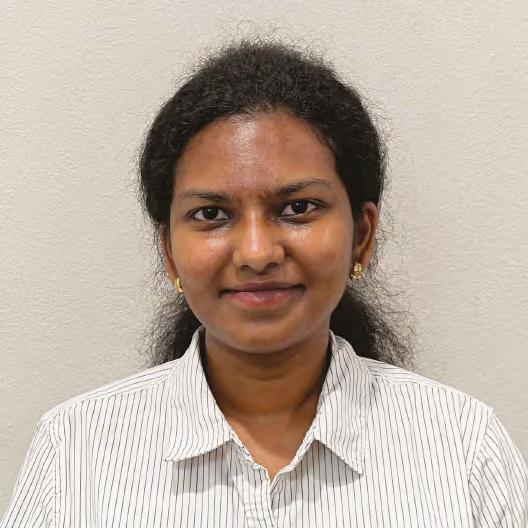
solve real-world problems. My ambitions led me to explore opportunities in higher education, where I could blend technical expertise with business acumen. This search wasn’t a solo journey; my parents played a crucial role in helping me research various programs, tirelessly combing through options to find the best fit for my goals. Together, we discovered the Rutgers Master of Business and Science (MBS) program, a unique combination of an MBA and MS, which was exactly what I was looking for. The high reputation and innovative curriculum of the program made it the perfect choice to fulfil my dreams. The excitement of being
While data scientists are often comfortable speaking in technical terms, these externships taught me the importance of translating technical metrics into actionable insights for business users.
accepted into this prestigious program marked the beginning of my journey in the United States. During my college years, I sought every opportunity to gain practical experience by participating in externships with organisations such as FRA/Conrail, Never Fear Being Different, Ortho Clinical Diagnostics, and the Defense Advanced Research Project Agency (DARPA). These experiences allowed me to apply data science concepts to real-world problems, giving me a deeper understanding of the challenges associated with data cleansing and data gathering. While data scientists are often comfortable speaking in technical terms, these externships taught me the importance of translating technical metrics into actionable insights for business users. I realised that data visualisation is a crucial component in effectively conveying data insights.
JHANANI RAMESH
Initially, I opted for the ECE concentration at Rutgers, given my background and comfort in the field. However, my curiosity soon led me to explore the burgeoning fields of big data analytics. As I delved into these areas through elective courses, I realised that this was where my true passion lay. The ability to derive insights from vast datasets and build predictive models fascinated me, and I decided to switch my concentration to analytics. This decision transformed my academic experience, as I found myself thoroughly enjoying every aspect of the program.
The combination of engineering and MBS training provided me with the best of both worlds. My engineering background strengthened my maths skills and problem-solving abilities, while the MBS program equipped me with a comprehensive understanding of both business and technical aspects. On the business side, I gained insights into finance, marketing, leadership, and communication, while on the technical side, I mastered data science techniques, statistics, AI, ML, regression analysis, Python, R, data structures, database creation, and SQL. This unique blend allowed me to apply data science techniques to make predictions and, more importantly, to convey those insights to business leaders in a language they understood. It helped me view problems from a business perspective and develop technical solutions to address them effectively.
Throughout my time at Rutgers, I developed a strong ability to learn and adapt to new technologies. The rapid pace at which technology evolves today demands constant learning, and I embraced this challenge wholeheartedly. Each semester brought with it the opportunity to master new software and programming languages, culminating in final projects that required me to apply these skills in real-world scenarios. This experience fostered a deep confidence in my ability to learn and master anything within a short time frame.
One of the key lessons I learned during my studies was the importance of basing solutions on the problems at hand, rather than the tools available. This realisation pushed me to step out of my comfort zone and explore various software and technologies, always striving to provide the best solutions for the challenges I encountered.
Today, as a data scientist at Integra LifeSciences, I apply these principles. I am passionate about creating and training models to achieve the desired outcomes, and I find great satisfaction in the process of exploration and innovation. The journey from ECE to data science has been one of continuous learning and adaptation, and I am excited to see where this path will lead me in the future.
I believe data science is like cream that can go on top of any cake. The analysis of data can be
applied across various industries –whether it’s finance, healthcare, life sciences, the energy sector, nonprofit organisations, or technology –data science enhances and elevates the decision-making process. The versatility and impact of data science are what make this field so exhilarating.
Outside of work, I am committed to continuous learning and contributing to the data science community. As an ambassador for Women in Data Science (WiDS), an active speaker and a student mentor, I actively engage in initiatives to share my knowledge. In my free time, I read Kaggle Notebooks and GitHub to stay current with the latest data science techniques. Furthermore, I have had the honour of serving on the jury panel for the Globee Awards.
The world of AI and ML is ever-evolving, and I enjoy staying informed about the latest developments by reading blogs and connecting with others in the community. This exchange of ideas and knowledge constantly inspires and motivates me, reinforcing my commitment to contributing to this dynamic field. My journey has taught me that with curiosity, dedication, and a willingness to learn, it is always possible to turn ambition into achievement. As Albert Einstein once said: ‘The important thing is not to stop questioning. Curiosity has its own reason for existing’. I encourage everyone to stay curious and enjoy finding answers.
The analysis of data can be applied across various industries –whether it’s finance, healthcare, life sciences, the energy sector, non-profit organisations, or technology – data science enhances and elevates the decision-making process.
ROHAN
SHARMA
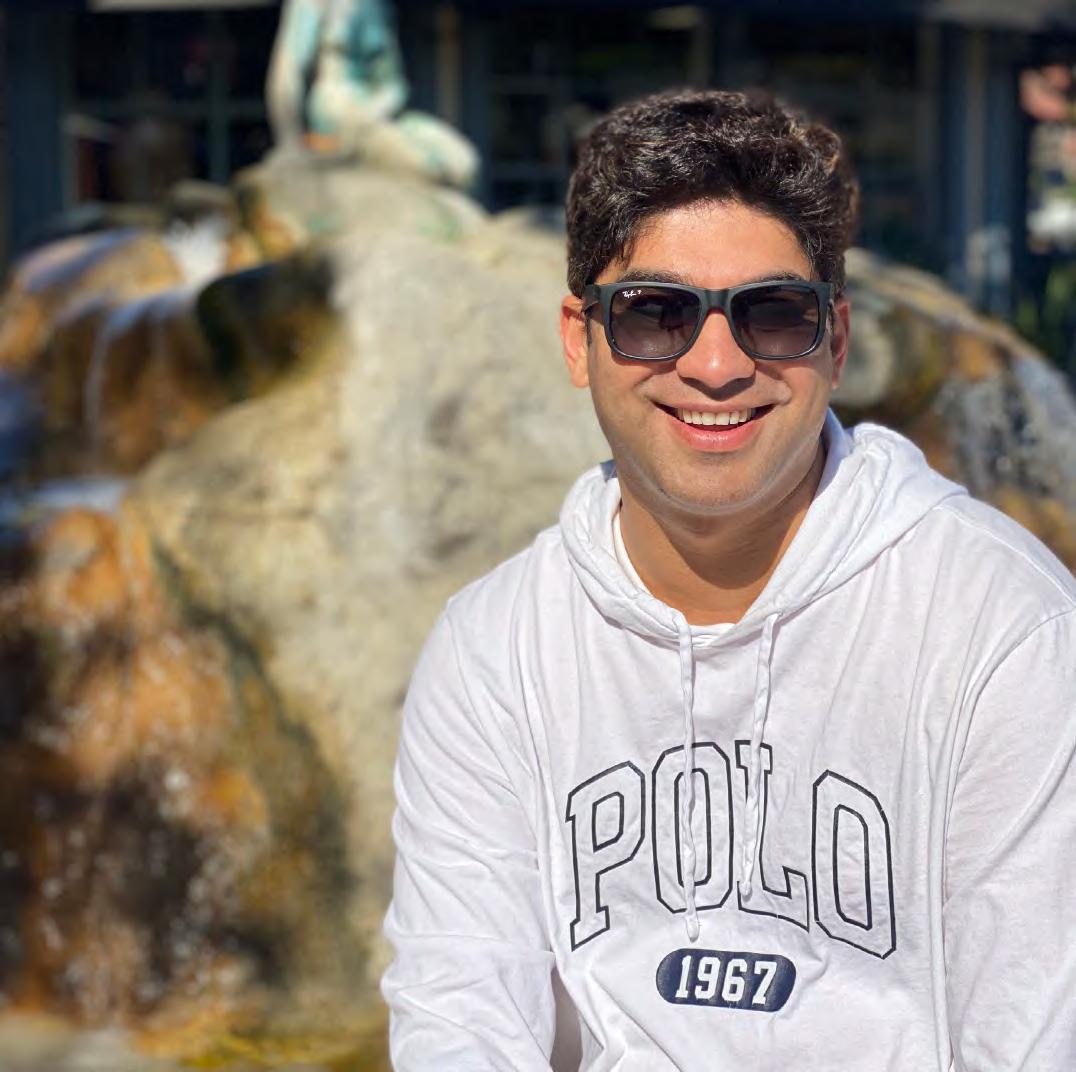
NAVIGATING AI GOVERNANCE:
A PRACTICAL GUIDE FOR ENTERPRISE LEADERS & INVESTORS
BY ROHAN SHARMA, AUTHOR OF AI AND THE BOARDROOM, AND CONSUMER DATA & ANALYTICS LEADER, MATTEL
ROHAN SHARMA is an awardwinning senior technology executive with extensive global experience in AI and digital transformation. His expertise spans automation, data science, and AI, having led initiatives for Fortune 100 companies including Apple, Disney, AT&T, Nationwide, and Honda. Rohan serves on advisory boards for Frost & Sullivan, UCLA Anderson School’s Venture Accelerator, Harvard Business Review, and the CMO Council. He mentors at Techstars,
UC San Diego, and MAccelerator, and consults for Stanford Seed. A USC-trained engineering leader, Rohan is an international speaker on leadership, presenting at TEDx and premier industry conferences. He has authored AI & Boardroom (Springer Nature) and Minds of Machines . Rohan’s multifaceted career encompasses roles as a keynote speaker, strategic advisor, mentor, and board member, showcasing his commitment to innovation and leadership in the tech industry.
ROHAN SHARMA
Anew breed of AI-first startups and business models is creating unprecedented opportunities for investors but is also introducing new risks and challenges. Navigating this complex landscape requires a shift in perspective, moving beyond traditional investment criteria to embrace a more holistic approach that incorporates AI governance as a core component of due diligence.
The biggest risk in AI adoption is fear itself. Scandals and negative headlines create a trust deficit, potentially slowing innovation and progress.
This article provides a practical, action-oriented guide for investors to evaluate AI portfolio companies with a focus on governance and making informed investment decisions. One of the key reasons why AI governance is crucial for startups is that it is essential for the large enterprises to which they sell their products and services.
Beyond the Hype: Asking the Right Questions
The allure of AI can sometimes lead to inflated expectations and overhyped claims. To avoid falling into the trap of ‘AI washing,’ where an AI-first approach in a startup’s core product is touted as a solution without substance, investors must adopt a more discerning approach to due diligence. This starts with asking the right questions to cut through the hype and gain a deeper understanding of the company’s true AI capabilities and governance practices.
This is particularly important because this is the first time in modern history that regulations, legal frameworks, and popular media conversation are going hand in hand with innovation in the field of AI. Furthermore, responsible AI governance in a portfolio company and its products builds trust, accelerating decision-making and technology deployment.
Key Areas of Focus
● Ethical Considerations: Delve into the portfolio company’s approach to addressing ethical challenges inherent in AI, particularly bias and discrimination. Responsible investing demands a proactive approach to mitigating potential harms and ensuring fairness in AI systems. This could also prevent legal challenges against the portfolio company in the future.
● AI Governance Framework: Look for tangible evidence of the portfolio company’s commitment to responsible AI development, such as an AI Principles document or adopted ethical guidelines. This demonstrates a conscious effort to establish guardrails and ensure accountability in AI development and deployment.
The biggest risk in AI adoption is fear itself.
● Regulatory Compliance: Evaluate the portfolio company’s understanding of and preparedness for the evolving regulatory landscape. Determine the company’s risk category under frameworks like the EU AI Act and assess compliance with existing regulations relevant to their industry. Some regulations could outright decimate the business models for many portfolio companies.
● Compliance Personnel: Inquire about the portfolio company’s internal structure for AI governance. Is there a designated compliance role responsible for tracking and interpreting regulatory guidance? This signals a proactive approach to risk management and compliance.
● Intellectual Property (IP): Assess the portfolio company’s IP strategy to protect investor interests and ensure long-term viability. Look for signs of a well-defined IP strategy and address any red flags related to potential IP self-dealing. Since many startups are built on large foundational models like GPT, Llama, etc. and on cloud stacks of these very same technology giants, portfolio companies should have a very clear understanding of IP ownership and potential copyright legal challenges their products and services can expose them to.
From Questions to Action: Deal-Gating with AI Governance
The answers to these due diligence questions provide crucial insights that inform investment decisions. By incorporating AI governance into the deal-gating process, investors can effectively filter opportunities and mitigate potential risks.
Without effective AI governance, companies risk missteps leading to misinformation, bias, IP infringement, and data leakage. These failures can result in financial and reputational damage, including fines, lawsuits, and brand value erosion.
● Red Flags: Certain responses should raise immediate concerns and warrant further investigation or potentially disqualify the investment altogether. These include overhyped AI claims without substantiation, a lack of a designated compliance person, and the absence of a clear IP strategy.
● Yellow Flags: Some answers, while not necessarily deal-breakers, require more cautious evaluation. This could include involvement in high-risk AI categories under the EU AI Act or other regulations, which signals potential legal and reputational risks that need to be carefully considered.
● Green Flags: Conversely, certain responses indicate a strong commitment to responsible AI development and governance, serving as positive indicators for investment. This includes companies that can articulate a clear understanding of AI governance principles, have implemented a robust compliance framework, and demonstrate a proactive approach to addressing ethical considerations.
Aligning with Global Standards: EU AI Act and NIST AI RMF
The global regulatory landscape for AI is rapidly evolving, with frameworks like the EU AI Act and the NIST AI RMF setting new standards for responsible AI development and deployment. Investors should encourage portfolio companies to align their practices with both frameworks, ensuring preparedness for international markets and mitigating future compliance risks.
In extreme cases, governance failures may trigger regulatory backlash, potentially stifling innovation across entire sectors.
Understanding ‘Foundation Models’: Licensing, Dependencies, and Risks
‘Foundation models,’ large-scale AI models trained on massive datasets, are becoming increasingly prevalent in AI applications. While powerful, these models present unique challenges and risks that investors must understand.
● Licensing Risks: Carefully evaluate the licensing agreements associated with foundation models, particularly those developed by third parties.
Understand potential restrictions on usage, modification, and commercialisation.
● Commercial Dependencies: Analyse the portfolio company’s reliance on foundation models and the potential impact of changes in licensing terms or the availability of these models on their business. Over-dependence on third-party foundation models can create vulnerabilities and limit flexibility.
Beyond Checklists: Vigilance, Adaptation, and the Human Element
AI governance is not a static checklist but a dynamic process that demands ongoing vigilance and adaptation. The rapid pace of innovation in AI requires investors to stay informed about emerging regulations, copyright debates, and technological advancements to effectively adjust their investment strategies and due diligence processes.
Beyond regulations and frameworks, AI governance ultimately comes down to people. Assess the portfolio company’s culture and its commitment to ethical AI development. Seek out founders who are open to feedback, guidance, and continuous learning in the realm of AI governance. Prioritise investments in portfolio companies thoughtfully designing systems that foster effective human-AI collaboration, ensuring human oversight and intervention where appropriate.
Additionally, consider how portfolio companies are positioning their AI solutions for adoption by target enterprises. Evaluate their understanding of enterprisespecific AI governance requirements and their ability to meet the stringent standards of large corporate clients. By embracing an informed approach to AI governance, investors can position themselves to capitalise on the transformative potential of AI, while mitigating risks and contributing to a more responsible and ethical AIpowered future.
Beyond regulations and frameworks, AI governance ultimately comes down to people.
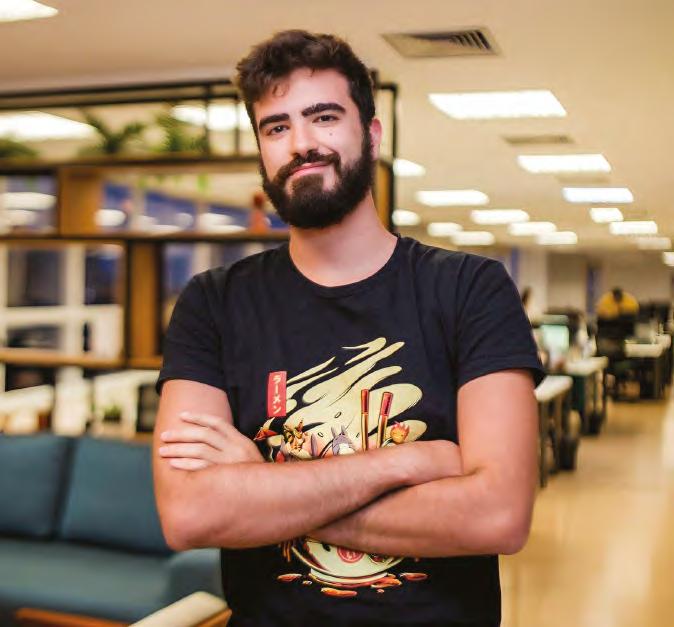
GUSTAVO POLLETI is a Staff Machine Learning Engineer at Trustly North America, where he leads efforts in risk and fraud mitigation for open banking payments across the region. Originally from Brazil, Gustavo holds both an engineering degree and an MSc from the University of São Paulo, where he is also pursuing a PhD. With extensive experience in financial decision-making, credit, and risk management, his current focus is on leveraging open banking data to develop the next generation of financial products. Gustavo’s goal is to realise the promise of open banking by building safer and more accessible products through advanced machine learning solutions.
SCALING AI WITHIN OPEN BANKING:
BUILDING TRUSTLY’S MACHINE LEARNING PLATFORM
If data is the new oil that fuels long-term profitability, North American open banking is a reservoir yet to be discovered, buried deep down within decades of financial system inefficiencies and complexities. Open banking data is both rich and diverse. A lot can be learned by looking at someone’s bank activity data. By knowing someone’s income or how they spend their money, one can draw behaviour models for several purposes. From fighting financial crime and fraud to providing relevant recommendations, open banking data remains one of the most relevant promises towards the democratisation of financial services with machine learning (ML). When it comes down to ML, data is king. The higher the relevance and quality of the data available for your ML models, the better they will perform. ‘It is all about the data!’ This line stuck with
me as I decided to join Trustly as their first machine learning engineer in early 2023. It’s impressive what we have accomplished in such a short timeframe since we started building our in-house machine learning platform.
Data-driven decisions can be a major differentiator in the competitive financial landscape and, ultimately, offer a cutting-edge experience for your customers – if the data is good enough. Trustly has built a comprehensive open banking platform that challenges siloed bank data. By connecting the data from more than 99% of financial institutions in North America, Trustly is pioneering the next generation of intelligent financial services.
If data is gold, machine learning is how we mint and make it shine. Open banking data offers several challenges for machine learning applications, requiring
GUSTAVO POLLETI
strong security guarantees as it handles sensitive and personal identifiable information (PII). Given privacy constraints, such data is subject to strict regulations in terms of when you can consult and for what purposes. Often, you will be granted access for a limited period of time during the customer interaction. As a result, machine learning systems using open banking data usually operate online or near-real time and, thus, deal with all the complexities and challenges that come along. Smart safety guardrails are paramount as the connection with third-party financial institutions can unexpectedly fail or take too long to respond. US financial institutions have diverse service levels. Not all US banks have direct APIs ensuring the most up-to-date data. As a result, our ML models must be robust enough to rely on outdated data or have a reliable fallback strategy in place.
The lack of open banking standardisation presents another big challenge for ML applications. Each financial institution provides information in their own format, while others don’t provide it at all. There is no widely adopted standard for bank activity expense categorisation. One financial institution can tag a transaction made in a pub as ‘Entertainment’ while another as ‘Food & Beverage’. The lack of standards makes it difficult to train models with data across different financial institutions.
At Trustly, we designed and implemented an inhouse machine learning platform to deal with the inherent challenges of open banking by developing a unified standard feature store, so that we could develop models that benefit from data across different financial institutions. We introduced model governance and several pre- and post-deployment safeguards to de-risk online near real-time machine learning systems. We also decreased model response time from seconds to just a few milliseconds. Today, we’re a major player in machine learning and AI in the open banking space and we’re well-positioned to continue leading the pack.
REAL-TIME GUARANTEED PAYMENTS DEPEND ON OPEN BANKING RISK MODELS
In the US, traditional payment rails like ACH take days to process, and it’s been cumbersome and inconvenient for customers to pay with their bank due to poor user experience and long settlement times. Can you imagine purchasing crypto assets and receiving them days later? Through our machine learning platform coupled with open banking data, we made guaranteed payments on the ACH rail available in real time.
Now, you can log into your bank, choose from which bank account you want to pay, and with a few clicks, your payment is complete. The merchant receives their payment, and you receive your digital assets immediately . That’s an entirely different experience from the archaic ACH payment without open banking.
Any customer can pay straight from their account, from any bank, with the click of a button and without needing a credit card or manually inputting account and routing numbers. Additionally, Trustly’s open banking platform helps merchants reduce expensive processing fees (the average credit card processing fee is 2.24%, which adds up to merchants’ payment costs).
Guaranteed Payments Reduce Risk for Merchants
To make the ACH payment happen in real-time, Trustly assumes the risk and pays the merchant directly at the time of the transaction and then later collects the payment from the customer’s account. The risk: Trustly may be unable to collect the payment due to fraud (which is still much lower than card-not-present fraud, thanks to the bank’s multi-factor authentication) or simply because the customer didn’t have sufficient funds during the transaction. That’s where machine learning comes in. ML bolsters the Trustly risk engine to mitigate against risky transactions and ensure we can still provide cost-effective guaranteed payments.
One of the most common ACH return codes is R01: Insufficient Funds. Trustly’s payment product guarantees the transaction instantly, at the moment of the purchase, but will only settle the transaction, i.e. collect the funds from the customer’s account, later after the ACH process has been completed. Even if the customer has funds in their account at the time of purchase, the risk of insufficient funds persists since ACH processing can take up to three days. The customer might spend those available funds elsewhere, causing the transaction to fail.
Why Bank Connectors Matter
Trustly built payment connectors with most banks in North America, covering 99% of financial institutions between Canada and the US. It’s no small feat, especially considering that other open banking providers use screen scraping, which is less reliable and less secure than doing the work to build bank connections from the beginning.
One of the major benefits of proprietary bank connections? We built them, and we maintain them. It’s a big reason our data is so reliable. Trustly needs accurate, bank-grade data that’s so reliable it can power guaranteed payments.
This technology allows Trustly to access and use fresh open banking data to conduct risk analysis and decide whether to approve or decline a transaction. Our platform’s capability is directly tied to the success of our risk models. While the stakes are high, the ability to access fresh consumer financial data gives us a competitive advantage to build data products at scale using machine learning. We’re the only open banking provider using this approach.
Toy example depicting the ‘non-sufficient funds’ risk scenario where the ACH transaction fails, causing financial loss
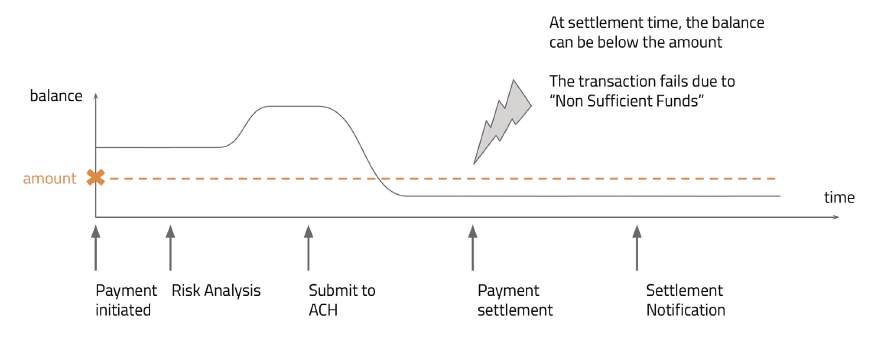
Consider the risk evaluation scenario depicted in Figure 1.
1. When the payment is initiated, the customer authenticates to their financial institution and allows Trustly to retrieve their account information through open banking technology. Only when the customer initiates the payment, are we able to retrieve balances, transaction history, debts, etc.
2. Next, we perform the risk analysis and decide to approve or decline the payment. We check the customer’s account balance to ensure they have the balance to cover the transaction amount. Note that there is a delay between the risk analysis and the transaction settlement, thus, despite the customer’s balance being enough when the payment is initiated, there is no guarantee that it will remain greater than the transaction amount when the payment is settled. In fact, the customer balance
can vary if they receive payouts, transfer money or other pending transactions are settled. This uncertainty whether the balance will still cover the amount during settlement is the ‘non-sufficient funds’ risk we discussed.
3. After Trustly has submitted the payment, ACH will process and try to settle it. If the customer’s balance is not enough to cover the transaction amount value, the transaction fails and Trustly incurs a loss, as it has already guaranteed the payment to the merchant. Up to three business days after the ACH submission, Trustly is notified if the transaction has completed successfully or failed.
As you can see, powerful machine learning models are needed to identify risky transactions and minimise company losses.
chart depicting an example of risk analysis representing the processing times of each step involved, from calling external data providers to the model inference and associated decision policy
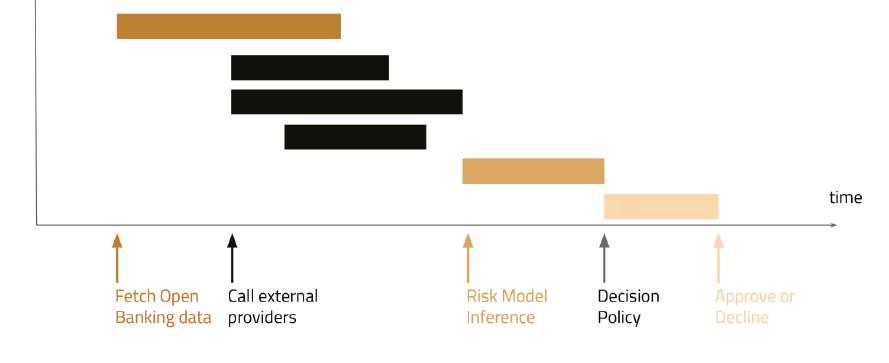
FIGURE 1
FIGURE 2
Gantt
Trustly machine learning risk evaluation has to operate with strict time constraints and be robust enough to cope with potential failures and data inaccuracies from external data providers. Consider the Gantt chart depicting the near-real time machine learning risk inference in Figure 2. Once the risk analysis starts, we retrieve open banking data from the customer’s financial institution and enrich it with API calls to external providers, including our own internal data. External providers, such as the financial institution itself, are prone to incidents causing data disruption, which can degrade the data quality from third parties. However, the risk analysis is robust enough to tolerate potential data lapses. The data sources go through our inhouse processing pipeline to produce rich engineered features. Not all processes are dependent on each other, so they can run in parallel. By processing independent parts of the risk analysis asynchronously, we can drastically reduce the total time. Of course, some steps are dependent, for example, the model requires all its features to be computed before starting the inference. Once we have produced the risk scores, we apply a threshold decision considering the amount and the risk of the transaction not having sufficient funds among other business rules and controls. In simple terms, we balance the trade off between approving more transactions while minimising risk.
Machine Learning Platform: High Stake Decision-Making at Scale
task that takes the longest to run (as opposed to summing up the time of individual tasks). Typically, the model inference time becomes the bottleneck after we employ async processing as it is a slow task and highly dependent on others. Feature selection and regularisation means the process of making the models lighter and leaner. For example, the fewer
Trustly connects businesses to thousands of financial institutions in the US and Canada as an account-toaccount payment method with industry-leading low latency even during peak transaction processing times.
features a model uses, the fewer tasks it depends on and the less time it will take in inference. We applied a backwards feature selection technique to prune the models as much as we can without significant predictive performance loss. Backwards feature selection consists of repeatedly training and evaluating models, reducing the number of features at each iteration until the performance deteriorates. For example, if we have 500 features available we train a model with all features and evaluate its performance. Then, we remove features based on some criteria, like the less relevant features in terms of importance or information value for the original model. We retrain and evaluate the model without the removed features. We repeat the process until we observe a drop in performance when compared to the original model.
We built our machine learning platform to support our risk analysis for Trustly Pay Guaranteed Payments. And when it comes to scale, Trustly connects businesses to thousands of financial institutions in the US and Canada as an account-to-account payment method with industry-leading low latency even during peak transaction processing times.
Our most requested models operate and maintain robust request per second (RPS) performance under regular business conditions, and can demonstrate significantly increased capacity during major events like the Super Bowl for our gaming customers. Additionally, since we operate in the payments business, the risk evaluation must respond under strict time constraints. No customer will wait seconds to have their payment processed.
We’re leading the industry with our feature selection, regularisation, and parallel processing. We reduced up to 10x the response times and have all our new models with p99 latency below 200ms with some models actually responding below 35ms!
When we process tasks in parallel instead of in sequence, the total elapsed time is driven by the
Besides latency, we also introduced several safeguards and fallback strategies to protect us from several failure scenarios. If our models go down or are fed with corrupted data, it presents a major problem! To prevent this risk, we developed strong control measures and processes. Every change in models and policies follows strict governance procedures, like peer review, model cards, automated testing, test coverage checks, stress testing, and several other forms of validations. Additionally, we have safeguards in place to reduce the blast radius and severity of potential incidents. For example, every model is escorted by at least one fallback model that can be activated if the main one becomes unavailable. The fallback model can act as a substitute if a feature group fails to be calculated or the model endpoint starts to timeout, thus allowing us to continue operations without downtime in case of failures.
Imagine the scenario where a given data provider fails. All features dependent on the unavailable source will be affected. The absence of, or worse, the corruption of several features can hurt model performance if it is highly dependent on them. When we develop a model, we also produce alternative versions without specific feature groups. For
example, if a data source fails and a specific set of features becomes unavailable or corrupted, we can redirect the traffic to the fallback model instead. This approach allows us to continue to operate in a more predictable setting.
Even if performance is degraded as the fallback model will always be worse than the main model, since we have validated the fallback model offline, we still have a good estimation about the performance loss. Otherwise, if we simply used the main model with the corrupted features, we can’t anticipate how it will behave. Of course, some failure scenarios can be anticipated and validated offline, but it can be difficult to anticipate failures, so we consider fallback strategies a more reliable approach. We also employ a fallback model as a last resource in case of severe infrastructure failure. If all model services become unavailable, start to timeout, or even a network issue prevents the main application server from calling our models, we still have a fallback model hardcoded in the main application server code.
MACHINE LEARNING PLATFORM: SCALE ACROSS THOUSANDS OF FINANCIAL INSTITUTIONS THROUGH STANDARDISATION
Financial institutions are diverse and, thus, some may be more reliable than others when it comes to data standards. One of the most difficult challenges to overcome in open banking is the difficulty of establishing a single data standard among all financial institutions. Every institution has its particular ways of managing and sharing its data. Trustly consolidates the financial data across financial institutions and categorises it in a single standard data model. Our unified data model allows us to build a Feature Store that centralises data from all the financial institutions connected to Trustly: the same sets of features can, therefore, be used for both major banks and local ones. This high level of standardisation allows us to scale our ML operation across institutions and geographies. We currently have more than 6000 features that can be reused among all our machine learning models to improve decision-making.
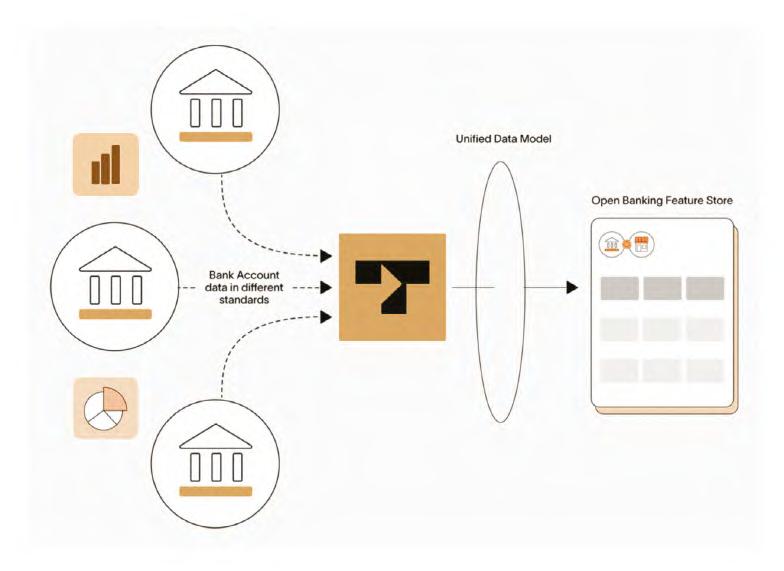
FIGURE 3
Scheme of a unified feature store for open banking data
While features can be plugged into any model, we often need to give specific attention to key merchant partners or financial institutions. For example, a major financial institution in the US may require a specific model, while minor institutions with similar behaviour can be grouped in a single model. Trustly currently operates several individual machine learning models along with other models that challenge the ones in production to ensure a continuous feedback loop. We keep striving to improve our performance.
While in most industries data science teams often operate as craftsmen, where each model development has a dedicated data scientist and its own code, Trustly requires a more industrial approach. As part of our platform, we created a standardised model pipeline covering all aspects from model creation, deployment, and observability, including data preparation, feature selection, hyperparameter tuning, regularisation, features validation, orchestration, artefact tracking, and service endpoint wrapping. This pipeline is versioned in an internal library. It is config-driven and is based on configurable steps.
For example, it is mandatory to apply hyperparameter tuning for model development, but choosing different tuning algorithms through a declarative configuration is possible. Through this effort, we managed to reduce model development time from about three months to a single week. We were able to refresh all our models through automated retraining thanks to having this standardisation in place.
EXPERIMENTATION, FAST ITERATION AND THE FEEDBACK LOOP PROBLEM
The standard training pipeline allows us to keep iterating on our risk models with outstanding agility. It automates most of the development and deployment process to boost our data science team productivity. However, engineered processes and automation are
great but ‘it is all about the data’. The data we have available to retrain models is what really moves the needle and can effectively allow us to evolve our models over time. Let’s recall the ‘non-sufficient funds’ (NSF) problem. As I mentioned earlier, the risk models are key in deciding to approve or decline a transaction. Note that we can only know if a given transaction effectively became a NSF if it was approved. If we decline a transaction, we can never know if it was a good or bad decision. Because the transaction never occurred, we would not know whether the transaction would become a financial loss or not. After I start using a model in production, it is expected that it will affect the data I get for next iterations as I will only know the outcome for transactions the model considers not risky, the ones effectively approved. Note that ‘the transactions the model considers a risk’ is different from ‘the transactions that will become an NSF’. Machine learning models are inherently approximations and imperfect, so part of the transactions it considers safe will become NSF (false negatives) and part of the transactions it considers risky won’t actually become NSF (false positives). In our setup where we only see the outcome for approved transactions, we are actually blind to the false positives. If we use this biased data to retrain a next iteration model and replace the previous one, the new model will likely only learn to discriminate among the false negatives of the previous model. If you launch it and replace the previous model, it will perform poorly as it will have to deal with the transactions that were previously declined and, thus, unseen to it. In the end, the new model will most likely learn the bias of the previous one instead of actually becoming better at the task at hand. We call this phenomenon a ‘degenerative feedback loop’, which is often found in problems like ours where the treatment, the decision of approving or declining a transaction, causes a selection bias in the data affecting the next model iterations.
While the stakes are high, the ability to access fresh consumer financial data gives us a competitive advantage to build data products at scale using machine learning. We’re the only open banking provider using this approach.
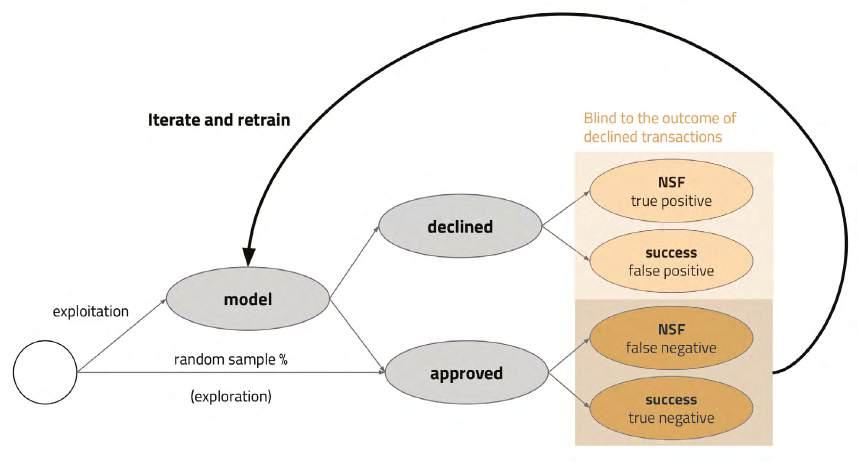
There are many ways to solve this feedback loop problem. The most simple one consists of approving a random sample of transactions without consulting the model. Of course, if we approve transactions that would be otherwise declined by the model, we can retrain and evaluate future iterations, avoiding the feedback loop. Note that the bigger this unbiased sample is, the more NSF you will get as you will approve risky transactions. The balance between letting more transactions in so that you can learn from them and build better models later versus using the current model to block as many risky transactions as possible is not trivial and known as the ‘exploration versus exploitation’ problem. At Trustly, we apply more sophisticated approaches that minimise the cost of exploration with causal inference techniques combined with experimentation designs. For example, instead of assigning the same probability of bypassing the model for every transaction, we could prioritise transactions with lower amounts or even capping high
amount transactions to prevent losses. Of course, this will introduce a selection bias, but it can be later treated accordingly during training. Other approaches may include more sophisticated techniques like building synthetic balanced datasets by combining approved data from different models or experiment designs specific for this purpose.
SCALING AI IN OPEN BANKING
We are a small and young machine learning engineering and data scientists team. There is still plenty of foundational work to do. It may not be today, tomorrow, or next year, but we are confident that our work will set the stage for world-class AI in open banking. Trustly has cracked the surface and dug so deep that it hit the big reservoir of the North American financial system that is open banking data coupled with machine learning. The data is pumping. It is time to continue building our machine learning platform to fuel new open banking applications.
FIGURE 4
Diagram representing the retraining process considering the feedback loop
ANTHONY ALCARAZ
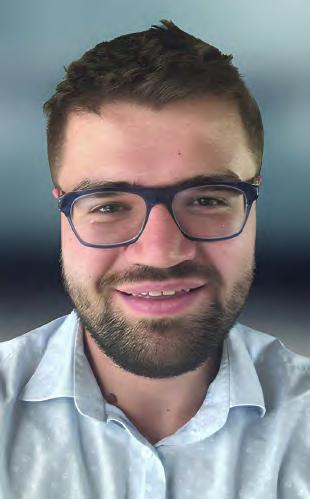
ANTHONY ALCARAZ is AWS’s Senior AI/ML Strategist for EMEA Startups and specialises in helping earlystage AI companies accelerate their development and go-to-market strategies. Anthony is also a consultant for startups, where his expertise in decision science, particularly at the intersection of large language models, natural language processing, knowledge graphs, and graph theory is applied to foster innovation and strategic development.
Anthony’s specialisations have positioned him as a leading voice in the construction of retrievalaugmented generation (RAG) and reasoning engines, regarded by many as the state-of-the-art approach in our field. He’s an avid writer, sharing daily insights on AI applications in business and decision-making with his 30,000+ followers on Medium.
Anthony recently lectured at Oxford on the integration of artificial intelligence, generative AI, cloud and MLOps into contemporary business practices.
GRAPH FOUNDATION MODELS: THE NEXT FRONTIER IN AI FOR BUSINESS?
Anew paradigm is emerging that promises to revolutionise how businesses leverage their interconnected data: graph foundation models. These powerful AI systems are designed to understand and reason about the complex relationships between entities in ways that traditional machine learning models simply cannot match. As businesses across industries grapple with increasingly large and complex datasets, graph foundation models offer a versatile and potent new tool for extracting actionable insights and driving innovation.
At their core, graph foundation models build upon the success of graph neural networks (GNNs) while addressing their limitations. These models employ innovative architectures such as graph mixture-of-experts (MoE) and graph transformers initialised with pretrained language model parameters. This allows them to effectively handle both structural and feature heterogeneity across diverse graph types.
For instance, the AnyGraph model uses a MoE architecture with multiple specialised ‘expert’ networks, each tailored to specific graph characteristics. This enables the model to adapt to various graph structures and feature spaces,
As businesses across industries grapple with increasingly large and complex datasets, graph foundation models offer a versatile and potent new tool for extracting actionable insights and driving innovation.
from social networks to molecular graphs. Similarly, graph language models (GLMs) combine the strengths of transformer-based language models with graph-specific architectural modifications, allowing them to process both textual and graph-structured data seamlessly.
The business value of these models is multifaceted:
Enhanced generalisation: Graph foundation models can make accurate predictions on entirely new types of graphs without additional training, a capability known as zero-shot learning. This allows businesses to quickly adapt to new data sources or changing market conditions.
Improved accuracy: By capturing higherorder relationships in data, these models often outperform traditional machine learning approaches in tasks like link prediction, node classification, and graph classification.
Versatility: A single graph foundation model can be applied across various tasks and domains, from e-commerce recommendations to financial fraud detection, streamlining a company’s AI infrastructure.
Scalability: These models are designed to handle large-scale, complex graphs efficiently, making them suitable for enterprise-level applications.
Importantly, graph foundation models offer relatively easy deployment compared to traditional graph AI approaches. Their zero-shot and few-shot learning capabilities mean they can often be applied to new domains with minimal fine-tuning, reducing the time and resources required for implementation. Additionally, their ability to handle heterogeneous graph data can simplify data preparation processes, as they can work with varied node and edge types without extensive preprocessing.
Furthermore, graph foundation models show promising compatibility with large language models (LLMs), opening up exciting possibilities for multimodal AI systems. For example, GLMs can process interleaved inputs of text and graph data, allowing for seamless integration of structured knowledge graphs with unstructured textual information. This synergy between graph AI and natural language processing could enable more sophisticated question-answering systems, improved knowledge graph construction, and enhanced reasoning capabilities across both textual and graph-structured data.
[...] graph foundation models show promising compatibility with large language models (LLMs), opening up exciting possibilities for multimodal AI systems.
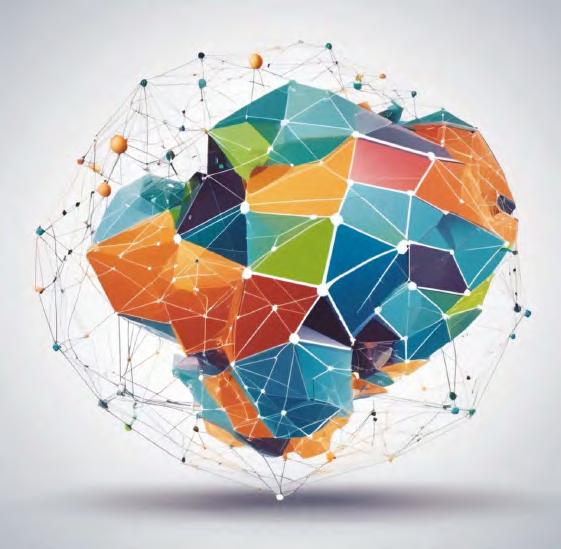
Foundational graph model by AI
ANTHONY ALCARAZ
THE EVOLUTION OF GRAPH AI MODELS
To understand the significance of graph foundation models, it’s helpful to first consider the evolution of graph-based AI. Traditional graph neural networks emerged as a powerful way to learn from graphstructured data, which is ubiquitous in the real world. Social networks, molecular structures, financial transaction systems, and countless other domains can be represented as graphs, with nodes representing entities and edges representing relationships between them.
GNNs work by iteratively aggregating information from a node’s neighbours in the graph, allowing the model to capture both local and global structural information. This approach has proven highly effective for tasks like node classification, link prediction, and graph classification. However, traditional GNNs face several key limitations:
1. Limited generalisation: GNNs trained on one type of graph often struggle to transfer that knowledge to graphs with different structures or from different domains.
2. Reliance on high-quality training data: Like many deep learning models, GNNs typically require large amounts of labelled training data to perform well.
3. Difficulty handling heterogeneous graphs: Many real-world graphs contain nodes and edges of different types, which can be challenging for traditional GNNs to model effectively.
4. Scalability issues: As graphs grow larger and more complex, the computational demands of GNNs can become prohibitive.
Graph foundation models aim to address these limitations by taking inspiration from the success of foundation models in other domains of AI, such as large language models like GPT-3. The key idea is to create a versatile graph AI system that can learn rich, transferable representations from diverse graph data, enabling powerful zero-shot and few-shot learning capabilities.
Two prominent examples of graph foundation models are graph language models (GLMs) and AnyGraph. These models employ innovative architectures and training approaches to achieve unprecedented generalisation and adaptability across different graph domains.
KEY CAPABILITIES OF GRAPH FOUNDATION MODELS INCLUDE:
1. Zero-shot learning: The ability to make accurate predictions on entirely new types of graphs without additional training. This is a game-changer for businesses dealing with constantly evolving data landscapes.
2. Cross-domain generalisation : Graph foundation models can handle diverse graph types from various domains, such as social networks, citation networks, and molecular graphs. This versatility allows them to be applied across different business functions without extensive retraining.
3. Fast adaptation: These models can quickly adjust to new graph datasets and domains, often requiring only a small amount of fine-tuning data to achieve high performance. This agility is crucial in fast-paced business environments.
4. Handling heterogeneous graphs: By employing sophisticated architectures like mixture-of-experts (MoE), graph foundation models can effectively process graphs with varying structures and node feature types.
5. Improved scalability: Through clever design choices and efficient training techniques, these models can handle larger and more complex graphs than their predecessors.
One of the key innovations enabling these capabilities is the way graph foundation models address the challenge of heterogeneous graph data. For example, the AnyGraph model employs a graph MoE architecture that learns a diverse ensemble of graph experts, each tailored to specific structural characteristics [2]. This allows the model to effectively manage both in-domain and cross-domain distribution shifts in graph structures and node features. Similarly, graph language models use a novel approach to unify the strengths of language models and graph neural networks [1]. By initialising a graph transformer with pretrained language model parameters, GLMs can leverage existing language understanding capabilities while also processing graph structures. This enables them to handle interleaved inputs of both text and graph data, opening up exciting possibilities for multimodal graph AI applications.
These advancements represent a significant leap forward in graph AI’s ability to learn and reason about complex, interconnected systems. As we’ll see in the next section, this translates into a wide range of powerful business applications across industries.
BUSINESS APPLICATIONS AND USE CASES
The versatility and power of graph foundation models make them applicable to a vast array of business problems across industries. Let’s explore some of the most promising use cases and how these advanced AI models can drive tangible business value:
E-Commerce and Retail:
1. Product recommendations: Graph foundation models can generate more accurate
and personalised product recommendations by leveraging complex relationships between users, products, and behaviours. By capturing higherorder interactions in the data, these models can uncover non-obvious connections and improve recommendation relevance.
1. Fraud detection: By analysing patterns in transaction networks and user behaviour graphs, graph foundation models can identify suspicious activities with greater accuracy and adaptability than traditional methods. Their ability to generalise across different types of fraud patterns makes them particularly valuable in this ever-evolving challenge.
2. Supply chain optimisation: Graph foundation models can analyse complex supply chain networks to identify bottlenecks, optimise routing, and predict potential disruptions. Their ability to reason about multi-hop relationships in the graph can uncover non-obvious inefficiencies and risks.
Finance:
1. Risk assessment: By modelling the intricate relationships between financial entities, market factors, and historical data, graph foundation models can provide more nuanced and accurate risk assessments for lending, investment, and insurance applications.
2. Market analysis: These models can process vast amounts of interconnected financial data to identify market trends, predict price movements, and uncover trading opportunities that may be missed by traditional analysis methods.
3. Anti-money laundering: The ability of graph foundation models to detect complex patterns in transaction networks makes them powerful tools for identifying potential money laundering activities and other financial crimes.
Healthcare and Life Sciences:
1. Drug discovery: Graph foundation models can accelerate the drug discovery process by analysing molecular graphs and predicting potential interactions between compounds and biological targets. Their zero-shot learning capabilities allow them to make predictions on novel molecular structures.
2. Patient outcome prediction: By modelling the complex relationships between patient data, treatments, and outcomes, these models can provide more accurate predictions of treatment efficacy and potential complications.
3. Disease spread modelling: Graph foundation models can analyse social contact
networks and geographical data to model the spread of infectious diseases with greater accuracy and adaptability than traditional epidemiological models.
Manufacturing:
1. Predictive maintenance: By modelling the relationships between equipment, sensors, and historical maintenance data, graph foundation models can predict equipment failures with greater accuracy and provide more targeted maintenance recommendations
2. Process optimisation: These models can analyse complex manufacturing processes represented as graphs to identify inefficiencies and optimise production flows.
Social Media and Online Platforms:
1. Content recommendation: Graph foundation models can generate more engaging and diverse content recommendations by capturing complex relationships between users, content, and interaction patterns
2. Community detection: These models excel at identifying meaningful communities and subgroups within large social networks, enabling more targeted marketing and community management strategies
3. Misinformation detection: By analysing the spread of information through social networks, graph foundation models can identify potential sources and patterns of misinformation with greater accuracy than traditional methods.
Telecommunications:
1. Network optimisation: Graph foundation models can analyse complex telecommunications networks to optimise routing, predict congestion, and identify areas for infrastructure improvement.
2. Customer churn prediction: By modelling the relationships between customers, usage patterns, and network performance, these models can provide more accurate predictions of customer churn risk.
Energy and Utilities:
1. Smart grid management: Graph foundation models can optimise energy distribution and predict demand patterns by analysing the complex relationships in power grid networks.
2. Fault detection and localisation: These models can quickly identify and localise faults in utility networks by reasoning about the relationships between network components and sensor data.
In each of these applications, graph foundation models offer several key advantages over traditional approaches:
Improved accuracy: By capturing higher-order relationships and generalising across different graph structures, these models often achieve superior predictive performance compared to traditional machine learning methods.
Faster deployment: The zero-shot and fewshot learning capabilities of graph foundation models allow for rapid deployment in new domains with minimal additional training data.
Greater adaptability: As business environments and data distributions change, graph foundation models can quickly adapt without requiring extensive retraining.
Unified modelling approach: Instead of developing separate models for different graph-based tasks, businesses can leverage a single graph foundation model for multiple applications, streamlining their AI infrastructure.
Interpretability: The graph structure inherent in these models often allows for better interpretability of results, as relationships between entities are explicitly modelled.
As an example of the performance gains possible with graph foundation models, the AnyGraph model demonstrated superior zero-shot prediction accuracy across various domains compared to traditional GNNs and other baseline methods. In experiments on 38 diverse graph datasets, AnyGraph consistently outperformed existing approaches in both link prediction and node classification tasks.
Similarly, graph language models showed improved performance over both language model and graph neural network baselines in supervised and zero-shot settings for tasks like relation classification. This demonstrates the power of combining language understanding with graph structure awareness. These results highlight the transformative potential of graph foundation models across a wide range of business applications. As we’ll explore in the next section, however, there are still challenges to overcome and exciting future directions to pursue in this rapidly evolving field.
CHALLENGES AND FUTURE DIRECTIONS
While graph foundation models represent a major advance in graph-based AI, they are still an emerging technology with several challenges to address and promising avenues for future research. Understanding these challenges and future directions is crucial for businesses looking to leverage these powerful models effectively.
Current Limitations:
1. Computational resources: Training largescale graph foundation models requires significant computational resources, which may be prohibitive for some organisations.
2. Data quality and preparation: The performance of these models still depends on the quality and consistency of the input graph data, which can be challenging to ensure across diverse data sources.
3. Interpretability: While graph structures offer some inherent interpretability, the complex nature of foundation models can still make it challenging to fully explain their decisionmaking processes.
4. Handling dynamic graphs: Many real-world graphs are dynamic, with nodes and edges changing over time. Improving the ability of graph foundation models to handle temporal aspects of graphs is an ongoing challenge.
5. Balancing specificity and generalisation: There’s often a trade-off between a model’s ability to generalise across diverse graph types and its performance on specific, specialised graph tasks.
Ongoing Research Areas:
1. Scaling laws: One of the most exciting areas of research is exploring how the performance of graph foundation models scales with increases in model size and training data. Early results from the AnyGraph study suggest that these models exhibit scaling law behaviour similar to that seen in large language models [2]. As models grow larger and are trained on more diverse graph data, their zero-shot prediction accuracy continues to improve, even as performance on in-domain tasks begins to saturate.
2. Emergent abilities: Researchers are investigating whether graph foundation models demonstrate emergent abilities – capabilities that appear suddenly as model size increases, which were not present in smaller versions. Understanding and harnessing these emergent properties could lead to breakthrough applications.
3. Multimodal integration : There’s growing interest in developing graph foundation models that can seamlessly integrate multiple modalities of data, such as text, images, and tabular data alongside graph structures. This could enable more powerful and flexible AI systems that can reason across different types of information.
4. Efficient architectures: Researchers are exploring novel model architectures and training
ANTHONY ALCARAZ
techniques to improve the efficiency of graph foundation models, allowing them to scale to even larger and more complex graphs.
5. Few-shot and zero-shot learning: While current graph foundation models show impressive zero-shot capabilities, there’s ongoing work to further improve their ability to generalise to new tasks and domains with minimal additional training.
6. Causal reasoning: Incorporating causal reasoning capabilities into graph foundation models could enhance their ability to identify root causes and make more robust predictions in complex systems.
ETHICAL CONSIDERATIONS: As with any powerful AI technology, there are important ethical considerations surrounding the development and deployment of graph foundation models:
1. Data privacy: Graph data often contains sensitive information about individuals and their relationships. Ensuring the privacy and security of this data throughout the model training and deployment process is crucial.
2. Bias and fairness: Graph foundation models may inadvertently perpetuate or amplify biases present in their training data. Developing techniques to detect and mitigate such biases is an important area of ongoing research.
3. Transparency and accountability: As these models are deployed in high-stakes business applications, ensuring transparency in their decision-making processes and establishing clear lines of accountability becomes increasingly important.
4. Environmental impact: The computational resources required to train and run large-scale graph foundation models have significant environmental implications. Developing more energy-efficient approaches is an important consideration.
INTEGRATION WITH OTHER AI PARADIGMS:
One of the most exciting future directions for graph foundation models is their potential integration with other advanced AI technologies:
1. Large language models: Combining the strengths of graph foundation models with the natural language understanding capabilities of large language models could enable powerful new applications in areas like knowledge graph construction, question-answering systems, and multimodal reasoning.
2. Computer vision: Integrating graph foundation models with advanced computer vision systems could enhance tasks like scene understanding, object relationship modelling, and visual reasoning.
3. Reinforcement learning: Combining graph foundation models with reinforcement learning techniques could lead to more sophisticated AI agents capable of reasoning about complex, graph-structured environments.
CONCLUSION
From enhancing e-commerce recommendations and detecting financial fraud to accelerating drug discovery and optimising manufacturing processes, graph foundation models have the potential to drive innovation and create competitive advantages across industries. Their ability to uncover non-obvious relationships and patterns in large-scale graph data can lead to deeper insights, more accurate predictions, and more efficient decision-making processes.
Key takeaways for business leaders and data scientists include:
Graph foundation models offer a versatile and powerful approach to leveraging interconnected data, with applications across numerous industries and business functions.
The zero-shot and few-shot learning capabilities of these models enable rapid deployment and adaptation to new domains, potentially reducing time-to-value for AI initiatives.
As research progresses, we can expect to see continued improvements in the scalability, efficiency, and capabilities of graph foundation models, making them increasingly accessible and valuable to businesses of all sizes.
The integration of graph foundation models with other AI paradigms like large language models and computer vision systems holds exciting potential for future innovations.
As businesses continue to grapple with increasingly large and complex datasets, the ability to effectively model and reason about interconnected systems will become a critical competitive differentiator. Graph foundation models provide a powerful new tool for harnessing this interconnected data, enabling organisations to uncover deeper insights, make more accurate predictions, and drive innovation in ways that were previously not possible.
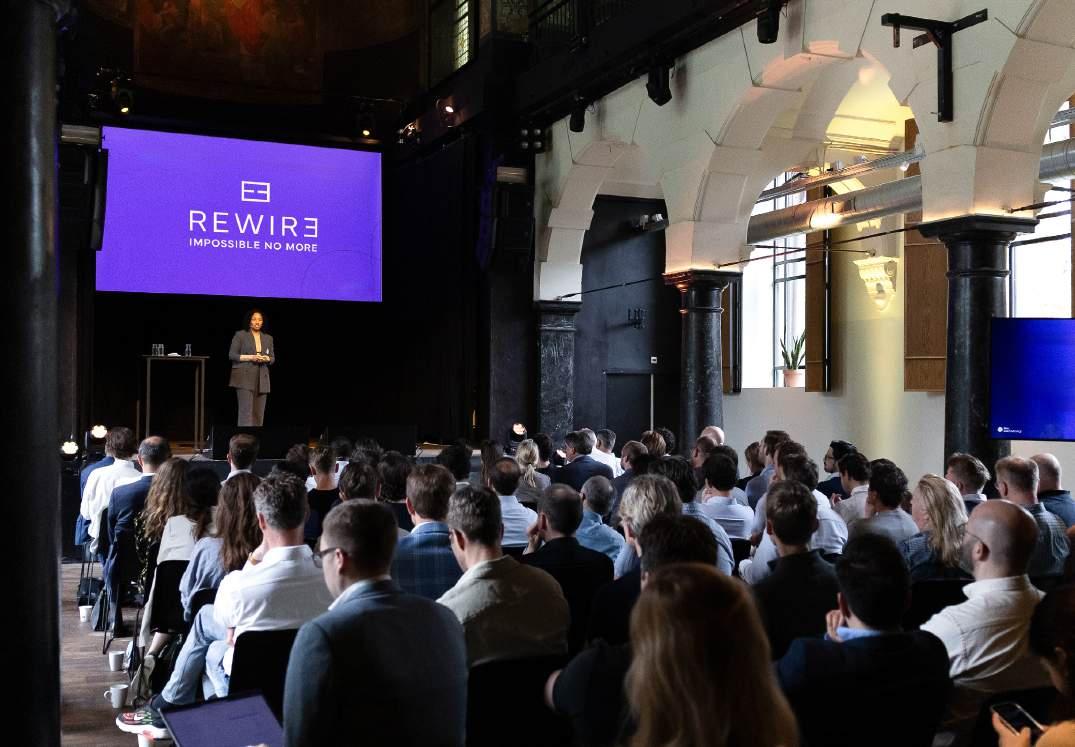
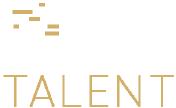
N AVIGATING COMPLEXITY: A LOOK AT THE SHIFTING LANDSCAPE OF CLINICAL DEVELOPMENT
By PHILIPP DIESINGER, GABRIELL FRITSCHE-MÁTÉ, ANDREAS THOMIK AND STEFAN STEFANOV
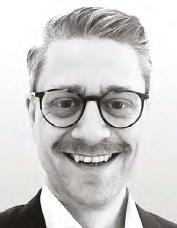
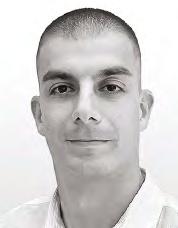
PHILIPP DIESINGER
is a data and AI enthusiast, having built a career dedicated to serving clients in the life science sector across diverse roles. Philipp is driven by his passion for leveraging datadriven decision-making to deliver tangible results with real-world impact.
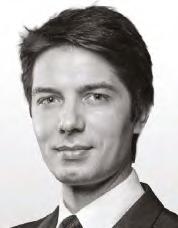
GABRIELL FRITSCHE-MÁTÉ works as a data and technology expert in the pharma industry, solving problems across the pharma value chain. He has a PhD in Theoretical Physics and a background in physics, computer science and mathematics.
STEFAN STEFANOV is a seasoned software engineer with over seven years of experience in leading and developing data and AI solutions for the life science sector. He is passionate about transforming intricate data into user-friendly, insightful visualisations.
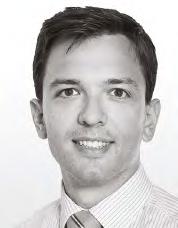
ANDREAS THOMIK is a senior data scientist driven by a passion for leveraging data and AI to generate business value, and has done so across multiple industries for close to a decade, with a particular focus on the life sciences field.
Over the last twenty years, clinical development has been transformed by advances in technology, patient-centric approaches, regulatory changes, and precision medicine. Digital technologies, big data, and AI have streamlined trial design, patient recruitment, and data analysis, while virtual trials have improved access and efficiency.
Patient-centric approaches have led to adaptive trial designs and better patient-reported outcomes [1] , enhancing relevance and tailoring therapies, especially in oncology and rare diseases [2]. Regulatory pathways like the FDA’s Breakthrough Therapy designation have expedited approvals for high-need conditions, and collaborations have increased to address global health challenges [3] .
Precision medicine, powered by genomics, has enabled targeted therapies, particularly in cancer, resulting in
more personalised treatment and better success rates. However, clinical trials have also become significantly more complex [4]. Broader inclusion criteria now ensure diverse representation, encompassing different genders, ethnicities, and age groups, which necessitates broadening recruitment across multiple regions [5]. This globalisation of clinical research requires managing diverse regulatory requirements and logistical challenges, particularly as trials are conducted across more countries than ever before [6]. The rapid expansion in the number of trial sites has necessitated sophisticated coordination to maintain consistency [7]
The shift towards personalised medicine, focusing on targeted therapies tailored to specific genetic markers, demands intricate trial designs and precise patient selection, adding new layers of complexity. Adaptive trial designs, which require ongoing adjustments based on interim results, further contribute to the challenge [8]
Advanced technologies, such as wearables and digital health tools, have also added to the complexity of clinical trials. The collection of data from diverse sources presents challenges in data integration, monitoring, and privacy maintenance. Additionally, the use of real-world evidence from electronic health records and patient-reported outcomes imposes further demands on data management and analytics [9] .
Furthermore, a growing focus on rare disease treatments, the rise of combination therapies, and the use of advanced endpoints have also played roles in adding complexity. Rare disease trials often require creative methodologies to address small patient
populations, while combination therapies necessitate extensive evaluation of potential drug interactions. Moreover, the sophisticated endpoints and patientreported outcomes require nuanced measurement and analysis [10]
To quantify changes in clinical development and gain insights into trends, diversity, and the growth of clinical research across various disease areas and regions, we analysed 617k interventional clinical studies initiated since 2000, using data from 17 trial registries. These studies spanned 186 countries, involved approximately 680k clinical sites, and were supported by 200k unique sponsors, enrolling a total of 121 million participants.
Figure 1 shows an increase of reach and complexity of interventional clinical studies.
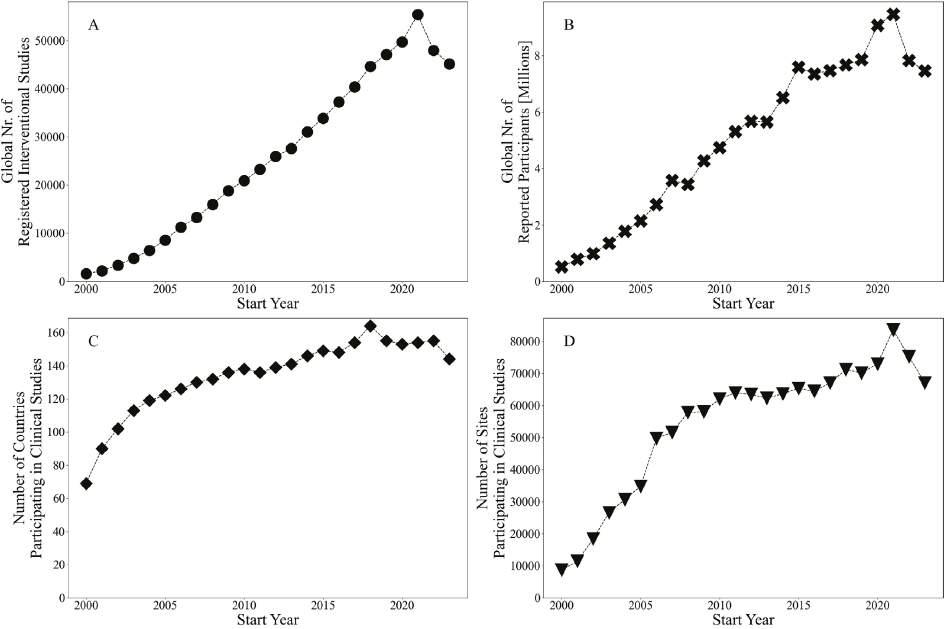
Figure 1A illustrates the significant rise in the number of clinical studies registered globally from 2000 to the present. This growth reflects not only stricter regulatory policies that require registering clinical studies in public study registries but also the increasing demand for new drugs, innovations in treatments, and a wider acceptance of transparent clinical research as a crucial component of medical progress.
Figure 1B highlights the parallel growth in the
number of registered participants involved in clinical studies. This increase aligns with the expanding number of studies and reflects efforts by researchers to ensure diverse and representative participant samples. Such diversity enhances the robustness of study results and supports a better understanding of drug efficacy across different populations.
Figure 1C demonstrates a twofold increase in the number of countries participating in clinical studies.
FIGURE 1
TRENDS AND INCREASED COMPLEXITY IN GLOBAL CLINICAL DEVELOPMENT SINCE 2000
This trend emphasises the globalisation of clinical research, with expansion beyond traditionally dominant regions like North America and Europe. The inclusion of new countries broadens patient diversity, making clinical findings ethnically and globally more relevant. Additionally, extending research into emerging markets has facilitated access to new patient populations, expertise, and potentially lower operational costs,
ultimately accelerating pharmaceutical innovation.
Figure 1D focuses on the growing number of clinical sites involved in trials worldwide. This increase reflects the expanded infrastructure needed to accommodate the rising volume of studies and participants. More clinical sites contribute to better patient access and enhance the capacity to test diverse therapeutic interventions.
FIGURE 2
DRILL-DOWN INTO CLINICAL DEVELOPMENT ACTIVITY OF NEOPLASMS AND DIABETES MELLITUS STUDIES
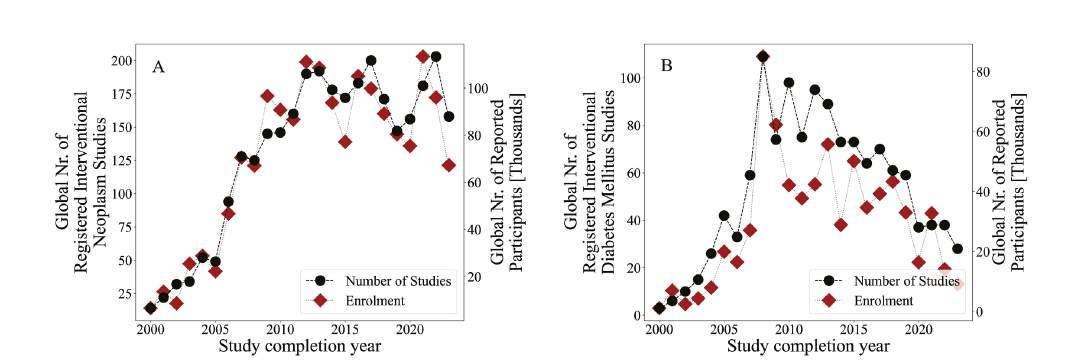
Globally, clinical development has intensified. However, examining individual medical conditions reveals more nuanced trends in concluded clinical trials. For instance, while studies related to neoplasms (Figure 2A) have seen an increase in both volume and participant numbers, diabetes mellitus studies (Figure 2B) have shown a recent decline in these metrics. This highlights how clinical research priorities can shift over time, reflecting changing needs, constraints and focuses across different medical conditions and therapeutic areas.
The increasing focus on neoplasm research can be attributed to several factors. Cancer remains one of the leading causes of death worldwide, and there are still many types of cancer with limited treatment options, which drives continued investment in oncology research. Advances in precision medicine, particularly genomics and immunotherapy, have made oncology a promising field with breakthroughs in targeted and personalised treatments, attracting significant funding and patient interest. Furthermore, governments and private organisations provide substantial incentives for
cancer research, and regulatory bodies often expedite approval processes for cancer drugs. Strong patient advocacy also fuels this momentum, ensuring that cancer remains a priority in clinical research and driving ongoing innovation.
In contrast, diabetes mellitus research has seen a decline in recent years. Diabetes, especially type 2 diabetes, has been extensively researched, leading to the development of numerous effective treatments, which reduces the immediate demand for new diabetes drugs [11]. The focus has increasingly shifted toward prevention and lifestyle interventions rather than new pharmacological treatments. Additionally, diabetes trials are often long and complex due to the chronic nature of the condition and the presence of comorbidities, making recruitment challenging and costly for sponsors [12]. As the market for diabetes medications has matured, pharmaceutical companies may have reallocated resources to emerging areas, such as obesity, metabolic syndromes, and other novel conditions, where there is more opportunity for innovation and growth.
Figure 2 analyses 3100 Phase 3 neoplasm studies and 1200 diabetes mellitus studies.
FIGURE 3
GROWING DIVERSIFICATION IN THE SCOPE OF CLINICAL DEVELOPMENT OF NEOPLASMS AND DIABETES MELLITUS STUDIES
Figure 3A shows an analysis of 3132 completed phase 3 neoplasm studies. Figure 3B shows an analysis of 1275 completed phase 3 diabetes mellitus studies. Studies were carried out by industry sponsors since the year 2000.
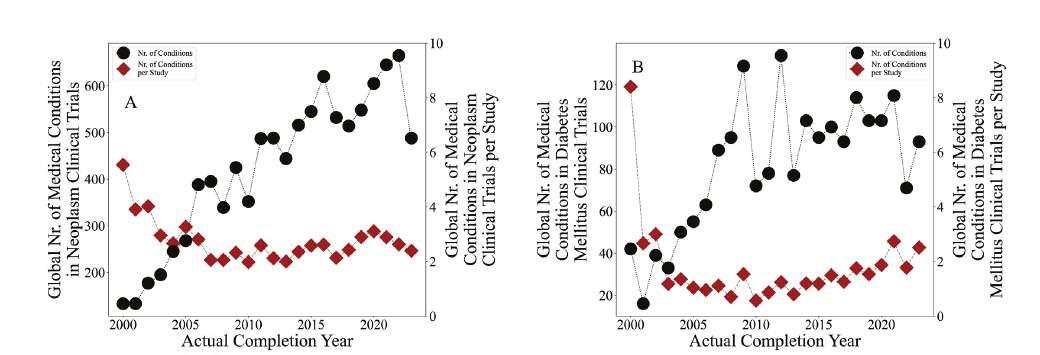
Figure 3A depicts how the number of unique medical condition terminologies used to tag phase 3 neoplasm clinical studies has increased over time as reported in global clinical study registries. This steady increase in unique terms suggests a growing diversification in the scope of clinical research. At the same time, the average number of medical conditions per study has stabilised at 2-3 conditions per trial, indicating a consistent focus on multi-faceted approaches while keeping study designs manageable. The expanding medical condition vocabulary reflects the increasingly complex nature of neoplasm studies and points to an evolving landscape where trials are designed to address a growing range of specific cancer types and subtypes.
Figure 3B shows a similar trend for diabetes mellitus. The number of unique medical condition terms has grown steadily despite the decline in the number of studies, indicating a diversification of study focus in diabetes research. Although the number of conditions tagged per study remains stable, this trend reflects the evolving complexity of diabetes trials, with researchers targeting more nuanced subgroups of the disease.
The findings in both figures highlight a broader strategy
REFERENCES
[1] Mullins CD, Vandigo J, Zheng Z, Wicks P. Patient-centeredness in the design of clinical trials. Value Health. 2014 Jun;17(4):471-5. doi: 10.1016/j. jval.2014.02.012. Epub 2014 May 5. PMID: 24969009; PMCID: PMC4497570
[2] Grant, M.J., Goldberg, S.B. Precise, pragmatic and inclusive: the modern era of oncology clinical trials. Nat Med 29, 1908–1909 (2023). doi.org/10.1038/s41591-023-02466-6
[3] Shea, M., Ostermann, L., Hohman, R. et al. Impact of breakthrough therapy designation on cancer drug development. Nat Rev Drug Discov 15, 152 (2016). doi.org/10.1038/nrd.2016.19
[4] Martin, L., Hutchens, M. & Hawkins, C. Clinical trial cycle times continue to increase despite industry efforts. Nat Rev Drug Discov 16, 157 (2017). doi.org/10.1038/nrd.2017.21
[5] National Academies of Sciences, Engineering, and Medicine; Policy and Global Affairs; Committee on Women in Science, Engineering, and Medicine; Committee on Improving the Representation of Women and Underrepresented Minorities in Clinical Trials and Research; BibbinsDomingo K, Helman A, editors. Improving Representation in Clinical Trials and Research: Building Research Equity for Women and Underrepresented Groups. Washington (DC): National Academies Press (US); 2022 May 17. Appendix B, Key Trends in Demographic Diversity in Clinical Trials Available from: www.ncbi.nlm.nih.gov/books/NBK584392/
within clinical development to refine and specialise the focus of studies, aiming for more precise interventions and a better understanding of specific conditions.
In summary, the evolution of clinical development over the past two decades has been marked by increasing complexity, patient-centric innovations, and a move towards precision medicine. The diversification in study design and medical condition focus demonstrates an industry adapting to address the needs of various diseases and patient populations. Moving forward, further advances in digital health, adaptive trial methods, and an emphasis on diversity and inclusion in clinical research are likely to shape the field. These ongoing changes may influence the pace and direction of clinical development, ultimately affecting how treatments are brought to patients worldwide.
In today’s increasingly complex clinical research landscape, AI and data sources like real-world evidence, patient registries, and study databases are essential tools for addressing and managing emerging challenges. To prevent a potential slowdown in medical breakthroughs, clinical studies must be optimised, with processes streamlined to enhance efficiency and effectiveness.
[6] Kristina Jenei, Fabio Y Moraes, Bishal Gyawali - Globalisation of clinical trials in oncology: a double-edged sword?: BMJ Oncology 2023;2:e000163
[7] Carlos H. Barrios et al., The Global Conduct of Cancer Clinical Trials: Challenges and Opportunities. Am Soc Clin Oncol Educ Book 35, e132-e139(2015). DOI:10.14694/EdBook_AM.2015.35.e132
[8] Duan, XP., Qin, BD., Jiao, XD. et al. New clinical trial design in precision medicine: discovery, development and direction. Sig Transduct Target Ther 9, 57 (2024). doi.org/10.1038/s41392-024-01760-0
[9] Khosla S, Tepie MF, Nagy MJ, Kafatos G, Seewald M, Marchese S, Liwing J. The Alignment of Real-World Evidence and Digital Health: Realising the Opportunity. Ther Innov Regul Sci. 2021 Jul;55(4):889-898. doi: 10.1007/ s43441-021-00288-7. Epub 2021 Apr 29. PMID: 33914297; PMCID: PMC8082742
[10] May, M. Rare-disease researchers pioneer a unique approach to clinical trials. Nat Med 29, 1884–1886 (2023) doi.org/10.1038/s41591-023-02333-4
[11] Eriksson JW, Eliasson B, Bennet L, Sundström J. Registry-based randomised clinical trials: a remedy for evidence-based diabetes care? Diabetologia. 2022 Oct;65(10):1575-1586. doi: 10.1007/s00125-022-05762-x. Epub 2022 Jul 29. PMID: 35902386; PMCID: PMC9334551
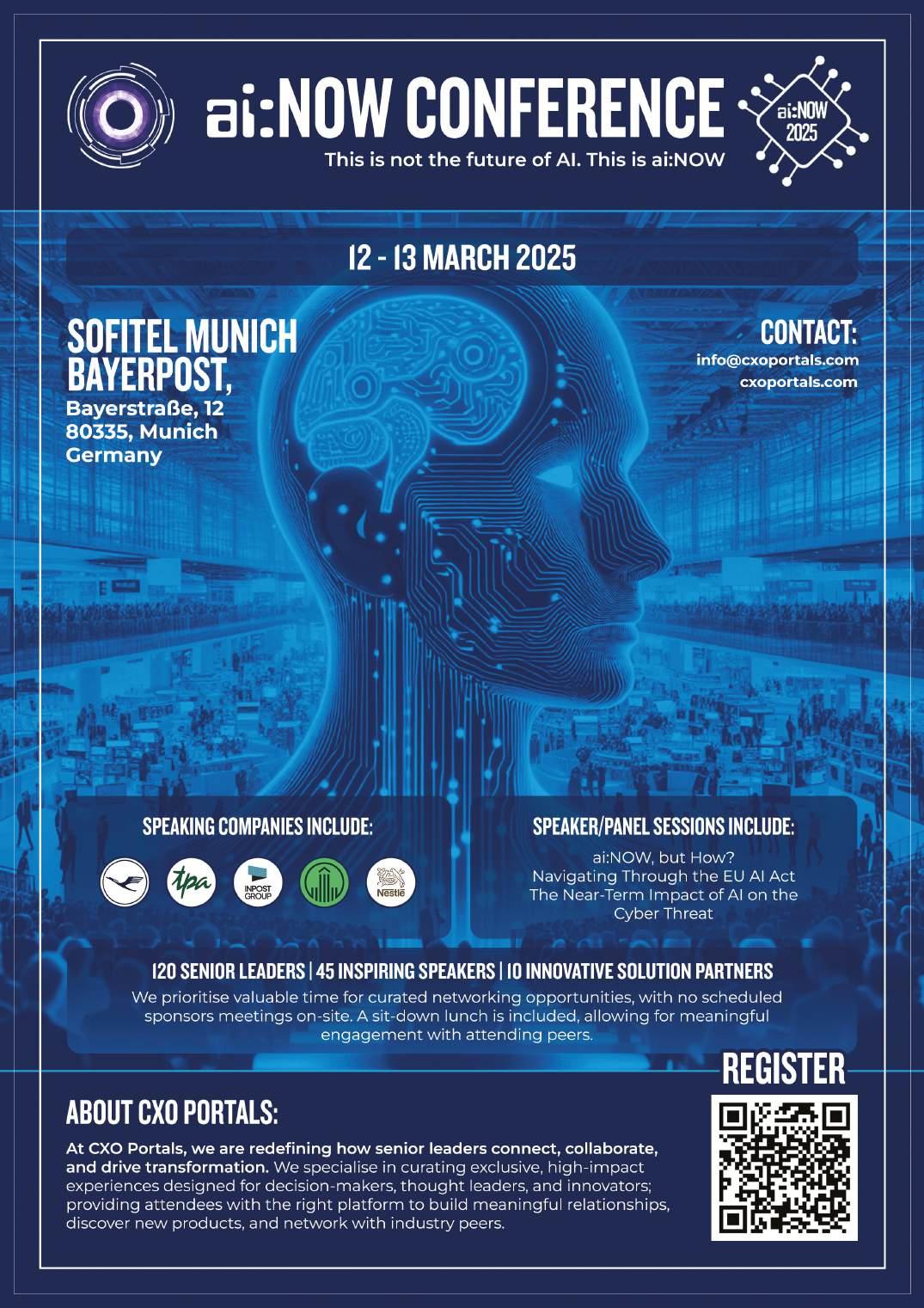
A CONVERSATION ON THE EVOLUTION OF GENAI: INSIGHTS FROM MARTIN MUSIOL
MARTIN’s academic background is in engineering and computer science, and he’s been coding since 2006. His professional experience includes working for some of the world’s leading companies, including household names such as IBM, Airbus and Mphasis. He’s been involved in the startup world and once created his own NLP startup. Martin has been working in the field of generative AI since 2016, which is an absolute lifetime in this discipline. He’s the creator of the world’s first online course in generative AI, and his book on the subject was published by Wiley.
Martin is also the organiser of the Python Machine Learning Meetup in Munich, and the creator of the influential newsletter Generative AI - Short & Sweet , which now has over 40,000 subscribers.
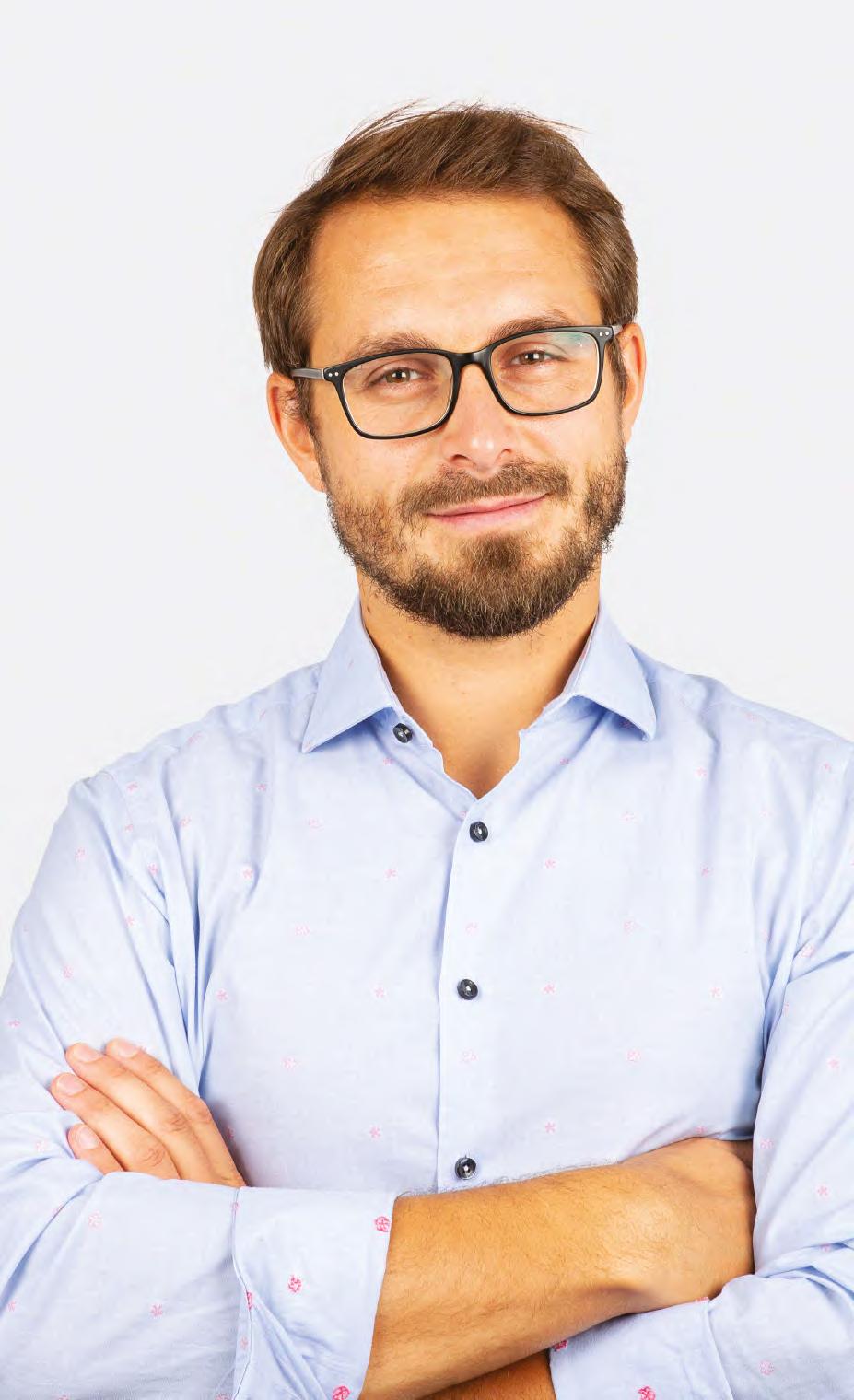
MARTIN MUSIOL
MARTIN MUSIOL
So, Martin, let’s go back to 2016. That was well before anyone was really talking about generative AI. What first got you interested in this field back then?
In 2016, I actually gave a talk at a conference in Milan titled ‘Generative AI: How the New Milestones in AI Improved the Products and Services We Built’. At that time, I was working as a data scientist at Flock Design, a consultancy. I had just come out of university, and one paper I came across in 2014 really stuck with me. It was on generative adversarial networks (GANs), by Ian Goodfellow – the original ‘vanilla’ GAN. That paper planted a seed, and it kept coming back to me.
In the design space I was working in, the concept of generating visuals through AI was very intriguing. Early GAN-generated images were rough, to say the least, but I could see the potential. I thought: ‘Someday, this technology might be able to create images that are indistinguishable from reality.’ The exact timeline wasn’t clear, but the potential was there. That’s what really drove me to explore how 3D object generation and other generative capabilities could fit into design.
What happened after those early years? When did you start to see the technology mature? At first, generative AI created some buzz, especially at that conference on data-driven innovation. There were engaging conversations, but it quickly faded because there was no clear business value back then. The tech was mostly focused on visuals, and the language models weren’t advanced enough yet. In 2017, though, things started to shift with Google’s release of the Bard model. I was working at IBM at the time, and we used Bard in a project for a geological client. That’s when I saw the first truly impressive applications of a language model.
Then in 2018, I noticed an increase in research on GANs, and more language model use cases were appearing in papers. I decided to launch a website called GenerativeAI.net and create an online course to explain the technology. I shared it on Reddit and Hacker News, and it gained traction – nothing huge, but enough to tell me there was real interest out there. Later, when DALL-E launched in the summer of 2022, I saw the first big spike in my site’s traffic. But the real explosion happened with ChatGPT’s release that December. That’s when interest in generative AI went truly mainstream.
How would you define generative AI? The term has evolved significantly: what, in your perspective, are the core components that make up generative AI?
There are many ways to look at it, but I’d describe it in contrast to what we call discriminative AI. Traditional AI has been largely about classifying or identifying data – whether through regression, reinforcement
Discriminative AI still drives value, of course, such as in recommendation engines, but generative AI is really just beginning to reveal its potential.
learning, dimensionality reduction, and so forth. These are discriminative tasks, where the model essentially ‘judges’ or ‘selects’ from existing options.
Generative AI, however, is about creation. Instead of analysing existing data, it generates new content: text, images, videos, even 3D objects. It’s a more complex task. Judging between options is relatively easy, but generating coherent, high-quality content from scratch is much harder. That’s why we’ve seen this shift, where generative AI is now taking the lead, especially as we find its applications in so many areas. Discriminative AI still drives value, of course, such as in recommendation engines, but generative AI is really just beginning to reveal its potential.
You’ve been part of generative AI’s evolution from the start. Could you walk us through some key moments? How did these developments shape generative AI, and how did you experience it?
I’d divide it into two main areas: sequential data generation and parallel data generation. Sequential data is where you’re working with text, music, or code –basically anything where the order matters. Parallel data generation, like image creation, is different.
Before transformers, we relied on models like LSTMs and sequence-to-sequence models for sequential data, and they worked pretty well. But then the ‘Attention Is All You Need’ paper came out in 2017, introducing transformers. It was revolutionary. Around that time, Google released the Bard model based on transformer architecture, and we implemented it in a project to identify specific words in data. Initially, we used regular expressions with about 80% accuracy, then an LSTM model improved that to around 90%. But when we used the transformer, accuracy jumped to 99% – a massive leap.
This new architecture was a game-changer. If Google had pursued it aggressively back then, they could have had their ‘ChatGPT moment’ sooner. But instead, they open-sourced it, and OpenAI ran with it. It’s interesting
because Ilya Sutskever, a co-founder of OpenAI, has mentioned that early conversations with Geoffrey Hinton on the potential of scaling these models were met with scepticism. But over time, the data and the improvements made the case undeniable.
It sounds like this combination of advancements and open collaboration really fuelled generative AI’s progress. Where do you see it going from here?
Generative AI has come a long way, but we’re still just scratching the surface. The possibilities are endless, whether in creative fields, scientific research, or even healthcare. I see it transforming how we create and interact with content, whether it’s personalised education or innovative medical applications. As models continue to improve, generative AI will reshape entire industries.
Looking back, it’s been fascinating to witness how something I first encountered as a paper on GANs has grown into this powerhouse that’s now a core part of modern AI. And as we see more breakthroughs, generative AI’s potential will only keep expanding.
In your view, what are some of the key technologies driving generative AI forward today?
Until recently, I was leading generative AI projects for Infosys across EMEA. About 99% of our clients wanted to incorporate some form of AI that could query a knowledge base. The core architecture behind these systems is called retrieval augmented generation (RAG). Essentially, you break down large knowledge sources into manageable chunks and use a semantic search to retrieve the most relevant pieces based on the query.
For example, we worked with a global transportation company that frequently received emails asking about regulations in various countries. Traditionally, answering these emails involved manually searching through extensive documentation. We created a chatbot where they could simply paste in the email content. The chatbot extracts the intent and then searches a vector database of the document chunks, retrieving the most relevant pieces of information. The result is then compiled and presented in a coherent, accurate response – powered by models like GPT-4. RAG architectures like this are fundamental in enterprise applications today.
Did you notice any significant technological shifts in generative AI driven by advancements in hardware, like GPUs or new chip designs?
Hardware is a big part of it, yes. Nvidia, for instance, has been at the forefront, creating more advanced chips tailored for AI workloads. Most of the investments in training infrastructure flow through hyperscalers straight to companies like Nvidia. Besides that, we’re
Generative AI has come a long way, but we’re still just scratching the surface. The possibilities are endless, whether in creative fields, scientific research, or even healthcare.
seeing new architectures emerge, like language processing units (LPUs), designed specifically for language tasks. Groq, for example, is a newer company competing with Nvidia in this space, though on a smaller scale. They focus on achieving lower latencies, which is crucial for real-time AI applications. Overall, improvements in processing power have accelerated generative AI development, making complex tasks more feasible.
You mentioned enterprise use cases, especially in regulatory or document-heavy environments. What are some other real-world applications where generative AI is making an impact?
Customer service is one area ripe for AI-driven transformation. A year ago, McKinsey mapped AI’s disruption potential across industries, and marketing topped the list. In marketing, we’re seeing AI applications in content creation tools like Grammarly and copywriting platforms. But customer service is where enterprise applications are really taking off. Consider routine tasks like address changes or account updates. Today’s language models can handle these efficiently, extracting the required information and updating it in a database, even if it’s provided in an email or as an image.
In fact, we’ve developed systems where AIs crosscheck each other’s work to ensure accuracy before updating customer records automatically. This process can even extend to phone interactions. Imagine calling your internet provider to update your address. With current models, you could speak directly to an AI that would make the update seamlessly. The potential to automate these interactions is enormous.
It sounds like generative AI could disrupt many sectors. Beyond marketing and customer service, where else do you see the biggest potential impact?
Software development stands out as a huge opportunity. I’m actually building an app that can parse financial documents, extract a company’s hierarchy, and visually map it out. I just started this project today, and it’s already 90% done! With a basic front-end, a back-end, and some connections to a language model and RAG system, you can create a functional proof of concept surprisingly quickly.
Generative AI tools like Claude 3.5 allow you to build projects with minimal coding. I simply described my idea
in natural language, iterated a few times, and was able to develop a working model. This might mean that in the future, learning traditional coding languages like Python and C++ won’t be necessary for many developers, which is quite a paradigm shift.
What advice would you give to large corporations looking to integrate generative AI into their existing AI strategies?
Good question. It doesn’t have to be a massive investment right from the start. I often advise companies to start small. A proof of concept (POC) is a great way to get started without overwhelming resources or budgets. You can set up a cloud-based environment, maybe on Azure or AWS, and create a basic semantic search application with your internal documents.
For example, Azure offers a service called Azure AI Search, which lets you upload documents and quickly build a powerful, front-facing semantic search engine. With services like this, concerns about data security can be managed effectively. Companies can design their systems to protect data from external access or misuse, especially in sensitive regulatory environments like Europe.
Could you give us an overview of small language models versus large language models? How do they compare in enterprise settings?
Small language models have far fewer trainable parameters than their large counterparts. For instance, GPT-3.5 has 175 billion parameters, whereas a smaller model like Microsoft’s Phi-3 has only 3 billion. Despite the size difference, well-optimised small models can perform remarkably well in specific use cases.
One common belief is that larger models are more prone to ‘hallucinations,’ or generating inaccurate responses, since they’re trained on such a vast amount of data. Smaller models, on the other hand, can be more efficient and are often suitable for tasks where latency or computational cost is a factor. Many small models are even open-source, allowing organisations to run them on local hardware.
From your experience, what are the most common hurdles companies face when implementing or fine-tuning these models?
Training large models is extremely resource-intensive. Fine-tuning a frontier model can cost millions. Opensource models like Llama 3 aim to make AI more accessible, but even those require significant resources for fine-tuning, which isn’t exactly ‘democratised’ yet. Another critical challenge is ensuring the integrity of the models being used. There’s a growing concern about ‘sleeper agents’ – backdoors or behaviours embedded into models during training. Anthropic researchers discovered that certain character sequences
can trigger hidden behaviours in a model, causing it to bypass guardrails. You don’t want that in a professional, customer-facing AI, so it’s essential to vet models carefully.
That’s a bit concerning. Could you elaborate more on the sleeper agent concept?
A sleeper agent is essentially a hidden function embedded in a model. Anthropic found that when a specific character sequence appears, the model switches into an unfiltered, unrestricted mode – ignoring any safety protocols. In an enterprise setting, this could lead to reputational damage if, for instance, a model starts generating inappropriate content based on an unknown trigger.
Even after fine-tuning, some of these behaviours can persist. So, companies should verify their models’ origins and use robust vetting processes before deploying them in production.
Designing generative AI systems clearly involves significant planning. Do you have any cautionary tales from your experience?
Definitely. We had a client during the early days of GPT-4 who implemented an application allowing users to interact with the model freely. Within a month, API usage skyrocketed, leading to a bill of half a million dollars – just for one trial month! That was a costly lesson in controlling access and managing user interactions.
Another issue is bots. The internet is full of automated bots, so if you have an open system, it’s essential to secure it, perhaps by requiring logins. Otherwise, you could be paying for automated spam interactions. Thoughtful design and user access management are crucial for any organisation looking to integrate generative AI effectively.
How does data quality and quantity impact generative AI models? You mentioned that smaller language models can sometimes work with less data, but what’s your experience here?
Working with well-trained models like ChatGPT or Claude-3 actually gives you some leeway with noisy data. If you set the context for the model – letting it know the data might be noisy and guiding it to focus only on relevant parts – it can manage pretty well. This is where prompt engineering becomes essential. Crafting the right prompts is a science in itself, and there was a time when papers on prompt engineering were coming out rapidly.
In RAG (retrieval augmented generation) applications, especially where you’re querying a large knowledge base, having clean data is crucial. Although models handle some level of noise, removing irrelevant information can streamline the response quality, especially when dealing with vast data sets.
MARTIN MUSIOL
How do you measure the performance of generative AI systems? What metrics do you find useful?
Performance measurement in generative AI is complex. There are benchmarks like MMLU and questionanswering tests, but sometimes these benchmarks are included in the training data, blurring the lines between training and testing results. Comparing models directly, however, can be insightful. Take the Elo rating system, originally from chess, which Hugging Face uses on their LMSys Leaderboard. They update this daily, showing how different models perform against each other. The leaderboard reveals the top models, like GPT-4.
Another interesting metric is the Hallucination Leaderboard, also on Hugging Face, which measures how often models generate ‘hallucinations’ or inaccurate responses. GPT-4 currently has one of the lowest hallucination rates. For those exploring model performance, these leaderboards are a good place to start.
Are there any misconceptions about generative AI you’d like to clarify? Or perhaps common questions you get?
Lately, I’ve been exploring Claude 3.5 Sonnet and its upcoming version, Claude 3.5 Opus, which are packed with tools. They’re remarkable in their ability to integrate code directly into documents. You can even build and publish web apps within the platform itself, just by outlining your ideas in natural language. It’s pretty wild! I highly recommend people try it out if they haven’t yet.
We’re touching on future trends in generative AI. Are there any emerging developments or technologies you’re particularly excited about? Absolutely. One trend I’m really interested in – and I write about it in my book, The Agentic Future – is the rise of AI agents. Imagine language models at the core of multiple agents, each with specialised functions, like a project manager agent, a quality assurance agent, and so on, all working together in a multi-agent framework. There’s a framework called CrewAI that’s pioneering this. Andrew Ng recently said that we’re already at GPT5 levels in performance with GPT-4o when used in a multi-agent setup, and I agree. These agents working in tandem could be game-changers, acting like executive assistants, planning our trips, or managing tasks in both our professional and personal lives.
And this agent-to-agent communication goes beyond individual assistance. My agents could interact with yours, negotiating prices or scheduling meetings. It’s
an exciting vision. Another development I’m watching is the rise of humanoid robots, which we’re seeing with projects like Elon Musk’s Optimus, which incorporates GPT-4o as its ‘brain.’ In 2024, we’ll see more of these technical convergences – AI models integrated into physical embodiments, bringing us closer to AGI.
Could you elaborate on how these developments relate to AGI?
The journey to AGI, or artificial general intelligence, hinges on bridging the gap between understanding the world through language and truly experiencing it. Text alone is just an approximation. If I describe living in a jungle for three months, you’ll get a sense of it, but it’s not the same as actually being there.
True AGI needs to connect with the world on multiple levels: visual, auditory, maybe even tactile through robotic embodiment. Multimodal and multi-sensor AI models, capable of interpreting and interacting with various data streams in real-time, are key. I cover this more in my book, discussing how multimodality, multi-sensory input, and multitasking capabilities –essentially imitating the human brain’s functions – are essential to AGI development.
That’s incredibly thought-provoking! Speaking of your book, could you tell us a bit more about it?
Certainly. The book, Generative AI: Navigating the Course to the AGI Future , is split into three parts. The first part is a brief history of AI, with examples of early generative AI, like ELIZA, a chatbot from 1965 that could simulate conversations.
The second part dives into the current landscape, exploring various applications and the vast potential that’s still untapped. I guide readers through a framework to identify opportunities for using generative AI in their own fields. The third part, which is about 35% of the book, looks forward. I discuss the future of generative AI, the evolution of autonomous AI agents, multi-agent frameworks, and the potential merger of these technologies with humanoid robots. The book concludes with an exploration of AGI and how we might prepare for its arrival.
You also have a popular generative AI newsletter. Could you tell us a bit about that?
The newsletter, Generative AI - Short & Sweet , comes out twice a week, on Tuesdays and Fridays. Fridays are a recap of the week’s top AI news, while Tuesdays dive into a specific topic. We’ve covered everything from small language models to applications of Llama agents and different AI frameworks.
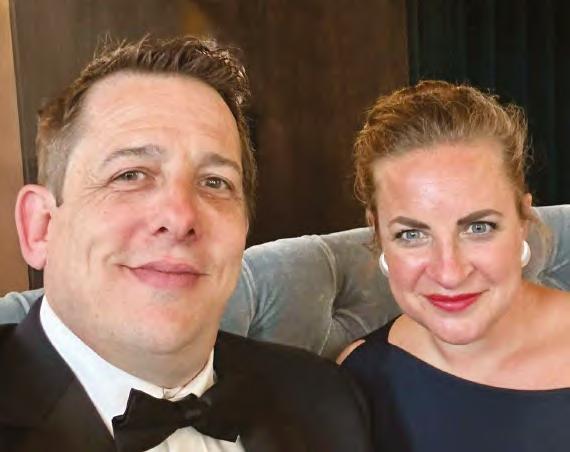
AI NOW CONFERENCE:
REVOLUTIONISING PROFESSIONAL EVENTS
THE AI NOW C ONFE RENCE is not just another gathering of AI enthusiasts. From its inception, the conference has sought to redefine the industry standard by fostering meaningful connections, delivering actionable insights, and creating an environment where participants genuinely enjoy learning and networking.
In this interview, we talk to the founders, CHARMAINE BAKER and GLENN HAPGOOD about their vision for AI Now, and why its unique approach has created a world-class event.
CXO PORTALS , founded by Charmaine Baker and Glenn Hapgood, is an events company with over a decade of experience. We support senior leaders both professionally and personally, focusing on audience management. Our virtual and physical platforms help partners and attendees build business relationships, discover new products, and network with industry peers. We connect senior leaders and businesses globally, fostering growth and integrity.
ai:NOW partnered with CXO Portals is a platform created to assist individuals and organisations with the implementation and understanding of AI.
When implemented correctly, AI delivers business value, and with more companies planning to incorporate AI technologies in the future, the growth will continue to rise.
For most enterprises, true innovation comes not from experimentation or pilots but from industrialising AI at scale.
Moving from one-off pilots to enterprise-wide adoption carries risks and challenges and many organisations lack clarity, the right infrastructure, processes, and skills in place to introduce actionable AI solutions.
The ai:NOW community of senior leaders and partners, as well as the upcoming ai:NOW Conference will all provide the support needed for individuals and organisations to truly understand and implement AI practically.
What was the inspiration behind setting up the AI Now conference?
We wanted to create something unique – an educational platform where end users could learn from one another, exchange ideas, and leave with actionable insights. Unlike many sponsor-driven conferences, our focus is on the attendee experience. We’ve intentionally minimised sponsor meetings to prioritise networking and relevant sessions.
The conference was designed to span two days, allowing executives to collaborate and explore all facets of AI – from current developments to future possibilities.
One of the key aspects here is the idea of creating ‘events to be enjoyed, not endured.’
CONTENT, STRUCTURE AND SELECTING THE RIGHT SPEAKERS
What makes the AI Now conference stand out from other conferences in terms of content or structure?
What sets us apart is our collaborative approach. We rely heavily on our advisory board, network, and community to identify real challenges in AI. Unlike other events that focus on buzzwords, we help companies implement AI effectively by starting with the basics – data readiness, team building, and pilot programs.
For companies excelling in implementation, the conference provides advanced tracks to refine their strategies. This tiered approach ensures value for participants at any stage of their AI journey.
Can you talk about how you choose the right speakers to ensure the sessions address the challenges people are facing? After identifying potential speakers, we conduct in-depth calls to refine session topics and formats
– whether interactive discussions or presentations – to ensure relevance to our diverse audience. We also evaluate speakers’ ability to present engagingly. A high-profile title isn’t enough if the speaker can’t hold an audience’s attention. With over 30 years of combined experience in event planning, we have learned the importance of aligning the right speaker with the audience’s expectations.
DELIVERING ACTIONABLE OUTCOMES, BUSINESS CASE STUDIES AND PILOT SESSIONS
How do you ensure that the sessions deliver actionable outcomes for your participants rather than just the theoretical knowledge common at other conferences? We review session synopses and presentations, providing feedback to ensure they’re tailored to the audience’s needs. Every session must be outcome-based, with actionable takeaways relevant to participants at different stages of AI maturity.
Speakers also contribute to a post-event report with key insights and summaries, ensuring attendees have tangible resources to implement professionally.
Can you explain how business case studies and pilot sessions work? What are the practical benefits for participants? These sessions are designed for mutual learning. Attendees submit use cases they’d like discussed, enabling tailored discussions. Business case sessions provide concrete examples attendees can adapt and present to decisionmakers. Pilot sessions detail the implementation journey, including challenges and solutions, providing a roadmap for attendees to launch their own pilots effectively.
The post-event document compiles these cases, serving as a valuable guide for attendees’ AI initiatives.
ENSURING PARTICIPANT ENGAGEMENT
How do you engage participants and ensure they stay actively involved throughout the two days?
Retention is a key metric for us, and we’ve consistently designed processes to maintain excitement and ensure engagement. It’s about the small details. From personalised calls to discuss expectations to offering a luxurious venue like the Sofitel, we ensure participants feel valued and comfortable. This personalised approach fosters a sense of belonging, encouraging attendees to engage fully.
Regular updates on new speakers and sessions maintain excitement, while feedback ensures we meet participants’ needs.
Networking is a central focus of AI Now. Attendees have ample time for meaningful interactions, whether during extended coffee breaks or sit-down lunches which allow participants to step away from the conference atmosphere and engage in high-quality conversations over a meal. This relaxed setting fosters deeper connections and meaningful networking.
Can you give us insight into how the size of the conference impacts the outcomes?
This is a purposefully small and niche conference to maintain highlevel, engaging discussions. It’s an invite-only event, though we do receive organic interest through our website, social media, and LinkedIn. Every registration is carefully vetted to ensure the attendees will benefit from and contribute to the event.
This selectivity ensures personalised care for attendees. With fewer participants, the team can accommodate dietary needs, tailor sessions, and provide seamless logistics for guest speakers. The smaller size also
fosters better engagement and higher-quality conversations.
Smaller events are far more effective for networking and interaction. At massive conferences, like CES in Vegas, meaningful connections are nearly impossible due to the sheer number of attendees. In contrast, our event – limited to about 130–140 participants – ensures every individual is approachable and relevant. It’s focused and intimate, facilitating deep, high-value engagement.
Large conferences can feel intimidating, especially for first-time attendees. By offering a personal touch, we ensure participants feel comfortable and
prepared. This personal connection starts with early communication, continues with tailored agendas, and extends to on-site assistance. When participants feel at ease, they’re more likely to engage openly and contribute to discussions.
SPOTLIGHT ON INNOVATION: SHOWCASING AI PRODUCTS
What AI-related products or solutions showcased at the event this year are you particularly excited about? While we hosted major players like Microsoft, Intel, and Dell, we placed equal emphasis on AI startups. Many startups offer groundbreaking solutions but
struggle to afford the sponsorship fees at traditional conferences. We wanted to give these innovators a platform to present their ideas directly to our high-level audience. The innovation stage hosted a competition that allowed startups like Deepdub, Gen8, and Aferis to pitch their solutions in an engaging format. By providing startups with a 10–15 minute pitch opportunity in front of decisionmakers, we help open doors that might otherwise remain closed. The winner, Uziel from Deepdub, stood out for presenting a solution that wasn’t just enterprisefocused but also inclusive and practical, resonating deeply with the audience.
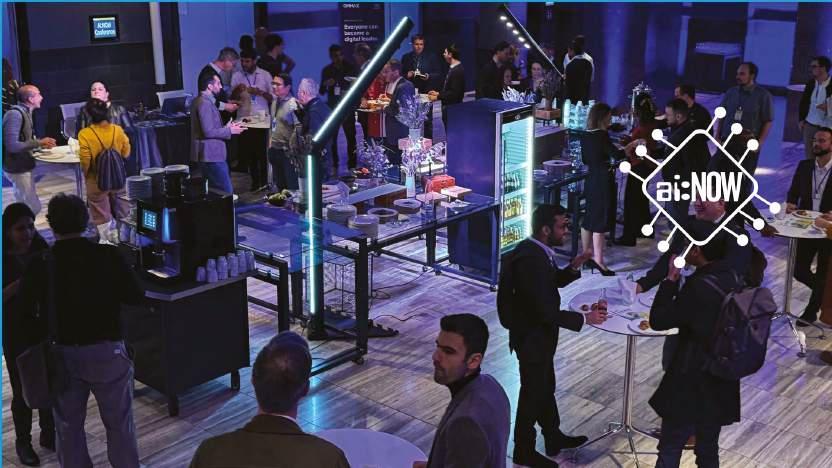
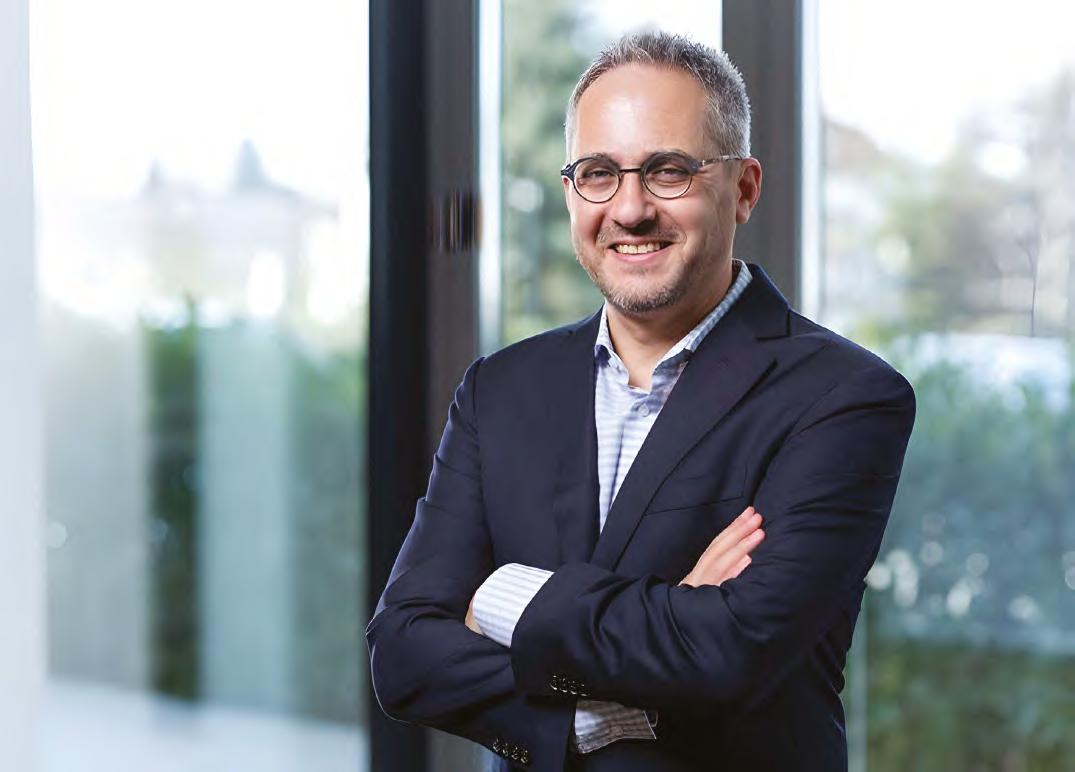
AI NOW COMPETITION WINNER SPOTLIGHT UZIEL ZONTAG - DEEPDUB
Can you tell us what Deepdub does?
Deepdub is advancing generative AI into new dimensions, specifically in the realm of speech. Our goal is to provide an easy-to-use yet highly professional tool for dubbing conversations or content into any language.
Basic dubbing – taking something spoken in English and converting it to Chinese, for example – already exists and isn’t the challenge. The real challenge lies in achieving high-quality dubbing in professional settings. Imagine dubbing a famous James Bond scene where Sean Connery’s
Scottish voice is rendered in Chinese. It might sound nice, but does it truly feel authentic?
Deepdub provides tools that go beyond simple translation, accounting for the surrounding context, environment, and cultural nuances. This allows users to deliver speeches or dialogues in another language with unparalleled accuracy and realism.
Our primary audience isn’t casual TikTok creators or everyday users. We cater to professionals – dubbing houses, filmmakers, and creators of professional training content. These are the people who need advanced,
industry-standard solutions.
Our primary use case is in the professional content creation industry. For instance, filmmakers and TV series producers use Deepdub extensively. Beyond that, consider a sports setting – say, a beautiful Maradona goal. With Deepdub, you could instantly dub that clip into Turkish or any other language in seconds.
Marketing is another key area. Most of the content we consume today combines video and voice. Deepdub integrates these elements into a seamless and professional platform, making it an ideal tool for various industries.
AI NOW Comompetition winner Uziel Zontag
The world of AI and localisation is evolving rapidly. What future advancements is Deepdub working on?
The first is real-time dubbing, which is incredibly exciting. Right now, we already have the capability for real-time dubbing of short clips, and soon, it will be possible for full discussions or entire environments in real-time. This opens up amazing possibilities. For example, you could speak to me in your language, and I wouldn’t need to respond in English. You’d hear my words seamlessly translated into perfect English, as if I had spoken it myself. This is just the beginning.
In the future, subtitles may no longer be necessary. People will experience content naturally, in their own languages, with no additional effort. This is a major direction Deepdub is pursuing.
The second direction is equally exciting: improving cultural and
STRATEGIC SPONSOR INTEGRATION
How are sponsors integrated into the conference in a way that enhances the experience for attendees?
We carefully select sponsors based on their alignment with our attendees’ needs. For example, at our last event, we partnered with Equinix, a data centre solution provider, to address challenges related to running AI on-prem or off-prem. Their presence wasn’t about hard selling but about offering valuable advice in a consultative, collaborative way. To maximise sponsor impact, we provide them with detailed attendee insights through our proprietary AI Now Matrix. This tool, developed by our co-founder Slava, benchmarks AI maturity across enterprises, industries, and individuals. The insights help sponsors identify potential partnerships and tailor their approach before the event even begins.
contextual harmonisation. One of the biggest challenges is ensuring that the dubbed content feels natural and culturally appropriate. With the rise of AI agents, the potential here is enormous. Imagine an AI agent specialised in, say, American cartoons. It could swiftly and accurately handle dubbing while understanding the cultural nuances, humour, and specific vocabulary required for that context.
This localisation-driven approach – using AI agents tailored to different content industries –represents the future. It ensures that vocabulary, tone, and jokes align perfectly with the cultural context, making the entire dubbing process smoother and more precise. Deepdub is investing heavily in this area, and it’s a major step forward for the industry.
Entertainment is a significant area where new use cases are emerging. For instance, we are
in discussions with companies organising large international events and fairs. These events attract audiences from diverse nationalities, and there’s a growing need for real-time translations into multiple languages simultaneously. This is definitely an area where Deepdub will make a big impact.
Another area is training. Training currently poses a significant challenge for large corporations – not just in creating translations but in ensuring accuracy. A critical issue is liability. For example, if a translation error leads to someone not following proper guidance, it can create serious problems. Companies need high-quality, precise dubbing to ensure their instructions are clear and error-free. Whether it’s learning how to operate a washing machine or following complex technical guidelines, well-dubbed training materials can greatly reduce such risks.
It’s also crucial to maintain the right balance. We limit the number of vendors and carefully select them to ensure the focus remains on end users. Attendees don’t want to be overwhelmed by vendors, and sponsors prefer not to compete with direct competitors. This approach fosters meaningful, mutually beneficial conversations.
THE FUTURE GROWTH OF AI NOW
How do you envision the conference evolving next year and beyond?
We’re planning to expand across Europe, targeting regions like the UK and Benelux. As attendees’ AI maturity grows, we’ll introduce tracks tailored to different stages – beginner, intermediate, and advanced – to ensure relevance and value. We also plan to revisit and evolve key topics. For example, regulations like the EU AI Act will
continue to develop, requiring updated sessions. Bringing back speakers to expand on their previous topics ensures continuity and deeper insights.
Another major development is the launch of an online community, enabling year-round engagement. This platform will allow participants to share stories, collaborate, and influence the framework of future conferences.
The AI Now Conference is redefining the professional event experience. By prioritising highquality interactions, actionable content, and inclusivity, it has become a leading platform for advancing AI knowledge and collaboration. With plans to expand geographically, adapt to attendees’ evolving needs, and integrate an online community, AI Now is poised to shape the future of AI events for years to come.
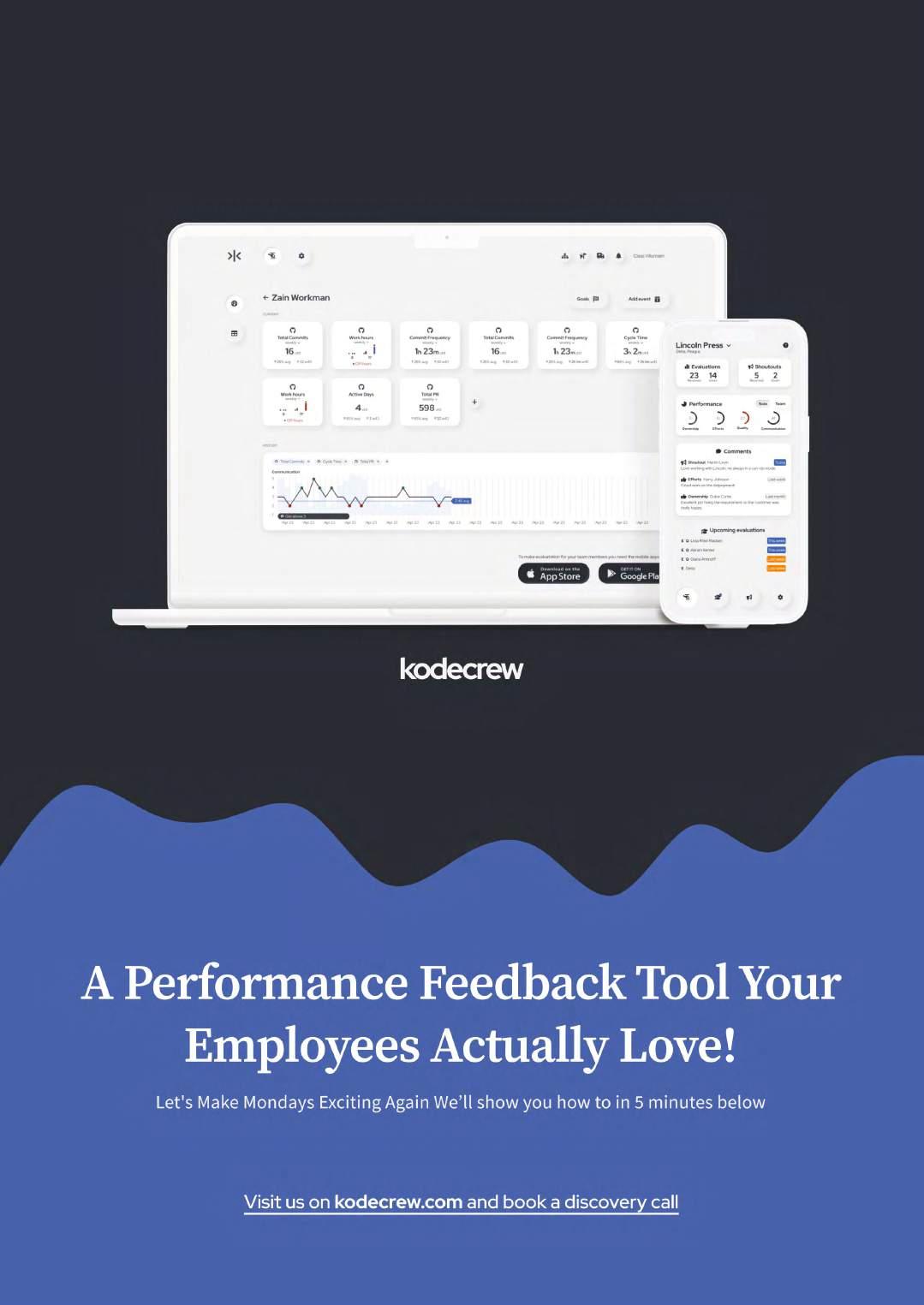
S3 Connected Health
The specialist partner for the design and development of digital health solutions and connected medical devices.
Our Pharma and MedTech Services and Capabilities
Digital health strategy consulting
Solution & service design
Medical device software & connectivity
Solution & device integration and evolution
Life cycle management
Digital health platform
Regulated healthcare software & SaMD development
Solution management & data services
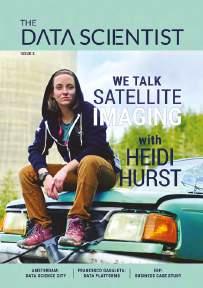
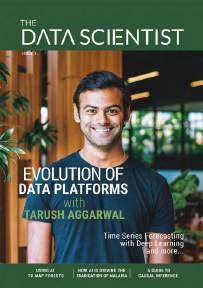
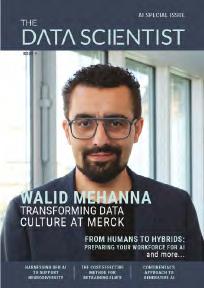
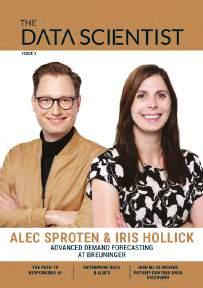
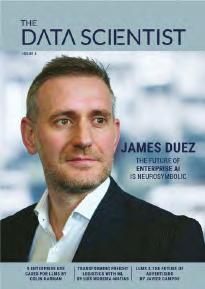
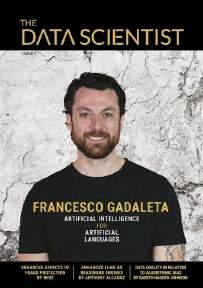
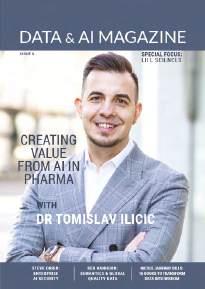
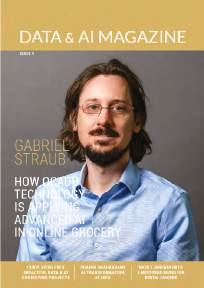