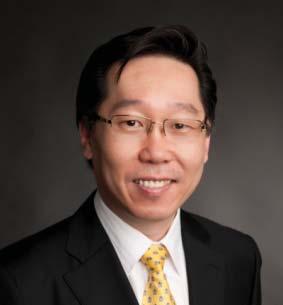
10 minute read
ArƟfi cial Intelligence transforms engineering Harnessing the power of compuƟ ng, Big Data and advanced algorithms, ArƟfi cial Intelligence presents new possibiliƟ es for today’s engineers
ARTIFICIAL INTELLIGENCE
TRANSFORMS ENGINEERING
by William Yong, Managing Director, Southeast Asia, Water and Narsingh Chaudhary, ExecuƟ ve Vice President, Asia Power Business, Black & Veatch Harnessing the power of compuƟ ng, Big Data and advanced algorithms, ArƟfi cial Intelligence presents new possibiliƟ es for today’s engineers.
Governments in Asia perceive Artificial Intelligence (AI) as a core pillar of their smart city strategies and growing investments in the concept indicate its vast potential. Singapore launched its National Artificial Intelligence Strategy and committed over SGD 500 million to fund activities related to AI under the Research, Innovation and Enterprise 2020 Plan. The Philippines is working with the Asian Institute of Management (AIM) Aboitiz School of Innovation, Technology and Entrepreneurship (ASITE) to prepare a roadmap which will position the country as an AI leader in Southeast Asia. Malaysia has set up a USD 1 billion AI park to boost research and development in the country. AI is not a new concept. What is new, however, is its value proposiƟ on. Rapid developments in compuƟ ng power, Big Data, Machine Learning (ML) and advanced algorithms have boosted the potenƟ al of AI. AI and ML technologies off er the ability to process and uƟ lise vast amounts of data. That ability allows power and water faciliƟ es, for example, to move beyond the descripƟ ve analyƟ cs in use currently. DescripƟ ve analyƟ cs uses data to understand past incidents and trends. Over the years, data mined from different power and water equipment has largely been unstructured. Today, power and water leaders can leverage AI technology by layering ML capabilities over the data collected, manipulate it, find patterns, and transform the data into insights that can anticipate required action. Automating business processes, gaining insight through data analysis, and engaging with customers and employees are some business priorities that can be addressed by AI. AI can enable and enhance predictive analytics which establishes what is likely to happen, and move towards prescriptive analytics which suggests actions based on the predictions. One example of predictive analytics is predictive maintenance. AI can predict the state of the equipment in advance so that maintenance can be scheduled. This addresses sustainability and reliability issues of facil
Mr William Yong Mr Narsingh Chaudhary
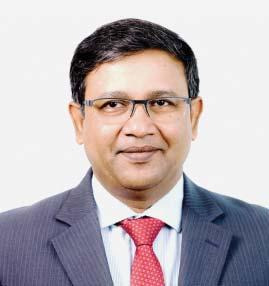
ities like water networks and power grids, while mitigating costly outages and other equipment failures. AI can also be trained to support prescripƟ ve analyƟ cs. PrescripƟ ve analyƟ cs enables autonomous management, where machines act on the informaƟ on the AI has extracted. Autonomous management conƟ nuously refi nes and amends the way it responds to the need to act.
First steps Industry response to AI has been positive. Pressured by limited capital, rising customer expectations, and growing commitments to sustainability and reducing resource consumption, asset-heavy facilities, like utilities, are adopting data analytics to improve different stages of the infrastructure lifecycle. Simplifying Asset Performance Management (APM) is one priority Asian utilities are considering. They are teaming up with software partners, like Black & Veatch’s subsidiary, Atonix Digital, to implement APM software that improves processes ranging from health-monitoring of critical assets, preventing failures, and improving the operational efficiency of facilities such as power generation, industrial, and water and wastewater treatment plants. By focusing on core areas including risk assessment, investment planning, data management, performance analysis, and monitoring and diagnostics, software such as Black & Veatch’s ASSET360 platform can promote innovation across the entire infrastructure lifecycle, through its modular, extensible architecture, and its seamless integration of functions and tasks across all specialised modules.
Engineers lead the change AI technology is bringing new opportunities and changing roles. AI relies on data, complemented by the insights of engineers who are intimately knowledgeable on how assets have been designed, built and behave. The engineers understand what the assets need to do and need not do, to present meaningful and relevant insight, advice or control action. Expertise from a variety of disciplines in the organisation will be necessary to provide insights into the data patterns to teach the computer how to recognise failure mode data signatures and understand how to optimise performance. Today’s engineers have the opportunity to think of AI as another component of the industry workforce. It can learn, but it needs to be taught. AI needs to gain experience, while operating under close supervision, from those who know the assets well. The result will be a blended workforce of artificial and human intelligence - Engineer 2.0. The close involvement of asset experts in the planning, implementation, training and operation of AI will help them develop trust in the system. The process will take time. According to one industry estimate, only about 20% of AI-aware companies are using AI technology in a core business process. A signifi cant amount of clean data is required to determine what opƟ mum performance looks like. Once performance baselines are established, anomalies can be detected with enough confi dence to provide expert advice or perform AI control. Most uƟ liƟ es do not yet have data in suffi cient quanƟ ty and quality to reach this point. While it may exist in pockets, there is sƟ ll much to do to in terms of data collecƟ on and structuring to support an eff ecƟ ve enterprise-level AI system. It will take a few years to achieve the full potential of an enterprise-level AI system. This sort of time scale will help foster the level of cultural change required to garner the requisite trust in AI’s ability to perform effectively and safely. Trust in AI is key if we are ultimately to delegate elements of control to it. Without trust, this will not happen. Creating the right level of trust requires the ability to explain. If the logical basis of a decision can be explained, that decision is more likely to be trusted. In other words, AI systems’ ‘thinking’ must be transparent and readily understood. This is our current challenge. The supercharged pace of technological change means that it may take a shorter Ɵ me to gather the requisite levels of suitable data and embed a successful, enterprise-wide AI system. What is certain, however, is that, with the support of AI, Engineer 2.0 is becoming a reality.
AI elevates producƟ vity in digital water networks Asian companies, faced with limited capital, rising customer expectaƟ ons, and growing commitments to sustainability, are adopƟ ng AI applicaƟ ons to improve different components of the water infrastructure lifecycle. Risk assessment, investment planning and performance analysis are some key business objectives that AI applications are addressing in the water sector.
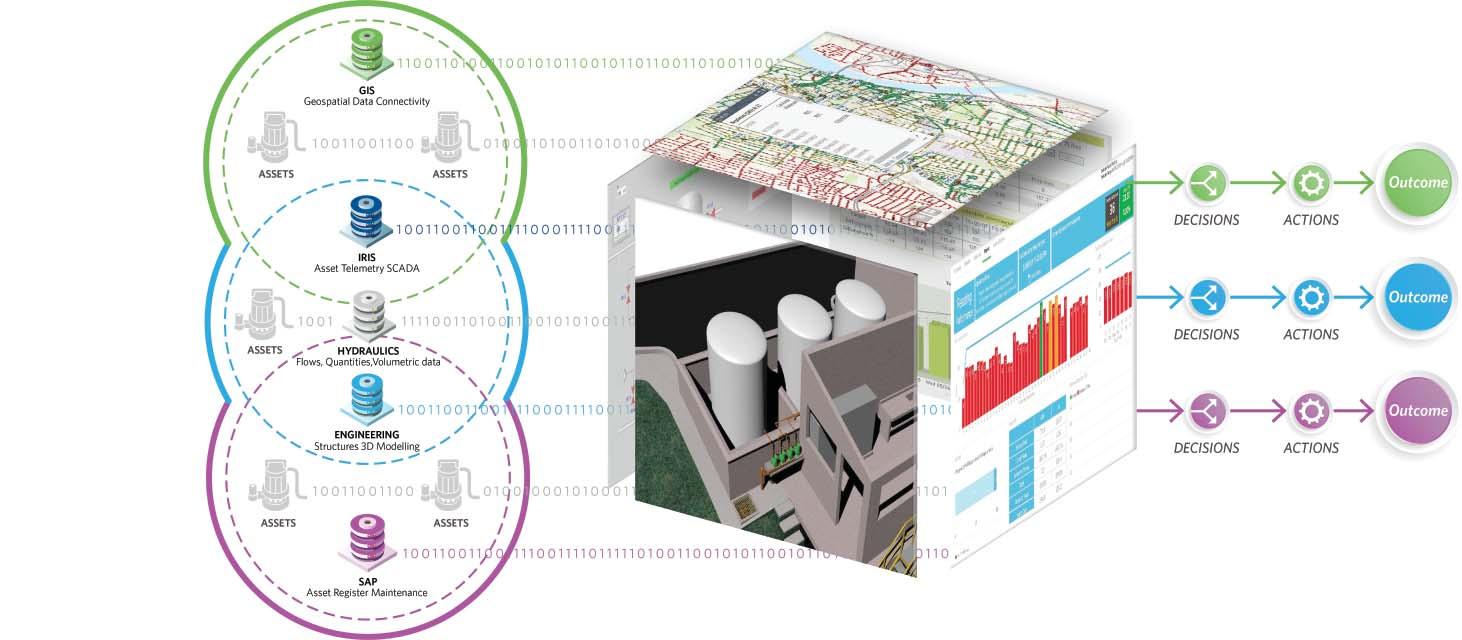
One AI applicaƟ on that has been considered is the creaƟ on of a digital representaƟ on of water treatment and distribuƟ on infrastructure, and embedding AI into a digital twin. By enabling predicƟ ve capabiliƟ es and intuiƟ ve decision support and intervenƟ on, digital twins address mulƟ ple business objecƟ ves. The twin is in constant dialogue with its physical counterpart, enabling uƟ liƟ es to simulate and test scenarios and opƟ ons before implemenƟ ng them in the real world. A water uƟ lity digital twin off ers the prospect of assistance to enhance customer experience, without increasing the bills to fund improvements, by opƟ mising performance of exisƟ ng assets and increasing the effi ciency with which they are operated and maintained. The twin supports this by facilitaƟ ng systems thinking - combining mulƟ ple internal and external data sources across the asset base with predicƟ ve analyƟ cal techniques served through mulƟ ple funcƟ onal views. This enables improved insights that support beƩ er decisions, leading to beƩ er outcomes in the physical world.
AI opƟ mises output potenƟ al in the next-gen power grid Governments across Asia are tapping integrated power infrastructures to address growing energy demand generated by rising incomes, industrialisaƟ on and a rapidly growing urban populaƟ on. An integrated power infrastructure takes advantage of diff erent generaƟ on, transmission and distribuƟ on technologies to help uƟ liƟ es overcome the piƞ alls of ageing infrastructure assets while meeƟ ng customer demand for energy that is renewable and reliable. While integrated power infrastructures off er opportuniƟ es to improve the way energy is produced, transmiƩ ed and consumed, they also introduce operaƟ onal complexiƟ es to the supply and demand cycle. One way to miƟ gate these operaƟ onal complexiƟ es is through autonomous management. An autonomous interface can integrate core components of the grid to opƟ mise power output. Core components of the grid include renewable and convenƟ onal energy, energy storage and microgrids. AI, with its capability to support analyƟ cs, forecasƟ ng and automated decision-making, is key to the success of Asia’s energy transiƟ on. AI-generated insights can improve renewable energy generaƟ on forecasƟ ng, grid stability and reliability, demand forecasƟ ng, demand-side management effi ciency and opƟ mised energy storage operaƟ on. The large quanƟ ty of data collected by smart sensors is parƟ cularly useful for renewable energy applicaƟ ons. As wind and sunlight aff ect power generaƟ on producƟ on, sensors and smart grids ensure that renewable energy plants are operaƟ ng to their opƟ mal potenƟ al. This promotes grid stability while addressing the intermiƩ ent nature of renewables. At the same Ɵ me, digital transformaƟ on iniƟ aƟ ves such as predicƟ ve asset maintenance off er forecasƟ ng and real-Ɵ me monitoring. PredicƟ ve maintenance uses ML, paƩ ern recogniƟ on and advanced analyƟ cs to opƟ mise, manage and deliver intervenƟ ons. These intervenƟ ons give power uƟ liƟ es in Asia the opportunity to prioriƟ se mission criƟ cal investments through idenƟ fying equipment and processes that could result in the highest cost benefi ts.
Black & Veatch to create water uƟ lity digital twins One of the world’s fi rst water uƟ lity digital twins will be created for Anglian Water in the UK, by Black & Veatch. The uƟ lity’s ‘future water company, today,’ iniƟ aƟ ve uses the Newmarket region of its operaƟ ng area as a proving ground for innovaƟ ons promising the greatest benefi ts. As part of this, Black & Veatch is creaƟ ng a digital representaƟ on of the region’s water treatment and distribuƟ on infrastructure and embedding Hybrid AdapƟ ve Real-Ɵ me Virtual Intelligence (HARVI), an arƟfi cial intelligence (AI) provided by its strategic partner EMAGIN, into the digital twin. This enables predicƟ ve capabiliƟ es and intuiƟ ve decision support and intervenƟ on. Anglian Water describes the Newmarket region as a ‘shop window’ for its cuƫ ng-edge approach to delivering water services. The ‘future water company, today,’ iniƟ aƟ ve has set seven ambiƟ ous goals for Newmarket - zero leakage and bursts, 100% customer saƟ sfacƟ on, water consumpƟ on of 80 litres per person per day, zero polluƟ on and fl ooding, 100% compliant and chemical-free drinking water, carbon neutrality, and building a circular economy that eliminates the concept of waste from the processes. The digital twin is a signifi cant step towards meeƟ ng those goals. A water uƟ lity digital twin off ers the prospect of helping enhance customer experience, without increasing bills to fund improvements, by opƟ mising performance of exisƟ ng assets and increasing the effi ciency with which they are operated and maintained. The twin supports this by facilitaƟ ng systems thinking - combining mulƟ ple internal and external data sources across the asset base with predicƟ ve analyƟ cal techniques served through mulƟ ple funcƟ onal views. This enables improved insights that support beƩ er decisions, leading to beƩ er outcomes in the physical world. The interface between Anglian Water’s asset monitoring and control networks, EMAGIN’s AI HARVI and Black & Veatch’s analyƟ cs and visualisaƟ on tools, will be managed using Black & Veatch’s ECO-X digital ecosystem environment. ECO-X facilitates the seamless interacƟ on of common water industry tools, uƟ liƟ es’ enterprise IT systems and Black & Veatch’s own AI-enabled data gathering, analyƟ cs and management tools.