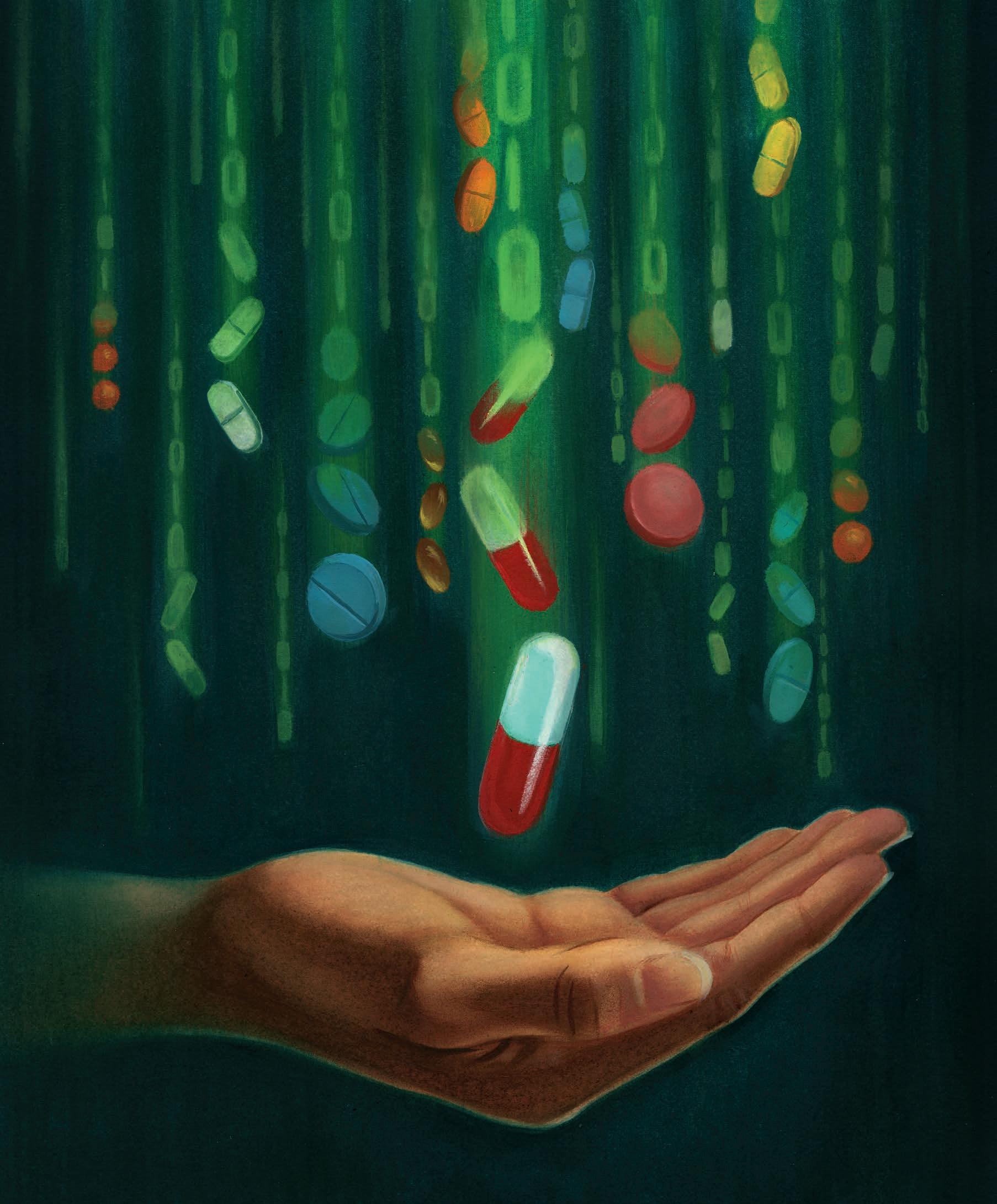
3 minute read
Collecting Clean Data
Natural-language processing is critical for better AI.
By CASSIE TOMLIN Illustration by CHRIS BUZELLI
Advertisement
ONLY 12% OF HEALTHCARE ORGANIZATIONS operate mature artificial intelligence (AI) programs. The AI gold standard integrates algorithms into a health system’s framework that are consistently vetted for bias and methodically monitored for compliance. The lack of trustworthy AI tools that translate between institutions can breed imbalanced, incomplete and skewed data.
Clean, AI-derived data is hard to come by. Less than half of 1% of studies that mine information from electronic health records (EHRs) harvest anything other than structured data fields, such as dates or diagnostic codes. This narrow approach excludes valuable context found in unstructured clinical notes and risks study results that don’t reflect actual population health.
“When data is not analyzed correctly, and the wrong conclusions are reached and the wrong actions are taken, that defeats the purpose of research and renders it harmful,” says Graciela Gonzalez-Hernandez, PhD, vice chair of Research and Education in the Department of Computational Biomedicine.
One way to achieve clean, widely applicable data, according to Dr. Gonzalez-Hernandez: Train AI programs with natural-language processing, which mines meaning from large sets of fluid text. Instead of keyword matching—rigid scouring for specific terms— natural-language processing analyzes large sets of sentences or phrases (on social media, in health records or from literature) to uncover more nuanced insights.
Analyzing unstructured data is costly and time consuming, whereas pulling codes is, in theory, less ambiguous and less prone to error. But the more egregious error in studying, for example, a set of people diagnosed with heart disease lies in ignoring variables only found in unstructured notes. Without accessing such text, researchers ignore people with heart disease who went undiagnosed and can also miss valuable insights into how symptoms differ across gender, race or age.
Natural-language processing can make all the difference in establishing a real understanding of the actual landscape of disease. Dr. Gonzalez-Hernandez points to a 2016 paper published in Diabetes Research and Clinical Practice comparing big-data strategies in the study of how often Type 2 diabetes patients experienced hypoglycemia. Researchers at Optum Epidemiology and Merck & Co. found that AI that utilized natural-language processing methods to read EHRs, combined with standard approaches, revealed a much higher prevalence of hypoglycemia than standard AI approaches alone.
A 2009 federal mandate requires physicians to use EHRs to report clinical data and quality measures. The rule was intended to standardize the capture of such information, facilitate its exchange, and improve research and care. But the effort has fallen far short, in part, because large-scale population health studies ignore unstructured EHR data, Dr. Gonzalez-Hernandez says.
“We’ve just begun to tap into this promise of using many records together to take advantage of cumulative knowledge to uncover patterns and come up with better ways to treat people,” she says. “After all these years, we’re still barely using EHRs, even though the data is all there.”